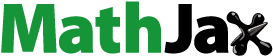
Abstract
Purpose
Health protection agencies require scientific information for evidence-based decision-making and guideline development. However, vetting and collating large quantities of published research to identify relevant high-quality studies is a challenge. One approach to address this issue is the use of adverse outcome pathways (AOPs) that provide a framework to assemble toxicological knowledge into causally linked chains of key events (KEs) across levels of biological organization to culminate in an adverse health outcome of significance to regulatory decision-making. Traditionally, AOPs have been constructed using a narrative review approach where the collection of evidence that supports each pathway is based on prior knowledge of influential studies that can also be supplemented by individually selecting and reviewing relevant references.
Objectives
We aimed to create a protocol for AOP weight of evidence gathering that harnesses elements of both scoping review methods and artificial intelligence (AI) tools to increase transparency while reducing bias and workload of human screeners.
Methods
To develop this protocol, an existing space-health AOP in the workplan of the Organisation for Economic Co-operation and Development (OECD) AOP Programme was used as a case example. To balance the benefits of both scoping review tools and narrative approaches, a study protocol outlining a screening and search strategy was developed, and three reference collection workflows were tested to identify the most efficient method to inform weight of evidence. The workflows differed in their literature search strategies, and combinations of software tools used.
Results
Across the three tested workflows, over 59 literature searches were completed, retrieving over 34,000 references of which over 3300 were human reviewed. The most effective of the three methods used a search strategy with searches across each component of the AOP network, SWIFT Review as a pre-filtering software, and DistillerSR to create structured screening and data extraction forms. This methodology effectively retrieved relevant studies while balancing efficiency in data retrieval without compromising transparency, leading to a well-synthesized evidence base to support the AOP.
Conclusions
The workflow is still exploratory in the context of AOP development, and we anticipate adaptations to the protocol with further experience. To further the systematicity, future iterations of the workflow could include structured quality assessment and risk of bias analysis. Overall, the workflow provides a transparent and documented approach to support AOP development, which in turn will support the need for rigorous methods to identify relevant scientific evidence while being practical to allow uptake by the broader community.
1. Introduction
Regulatory-based decision-making requires evidence from high-quality empirical studies. However, due to the sheer volume of research published daily and barriers to accessibility, such as siloed information storage, collecting and organizing relevant studies and information that represent the current state of research can constitute a hurdle for timely policy development. The need to organize scientific information to understand toxicological effects is one of the catalysts of the Organisation for Economic Co-operation and Development (OECD) Programme on the adverse outcome pathway (AOP) knowledge framework. First conceptualized over a decade ago, the AOP framework (Ankley et al. Citation2010) is a structure for assembling knowledge using studies that can inform regulatory decision-making (OECD Citation2018). Stored as living documents in the web-based and crowd-sourced AOP Wiki (aopwiki.org), the pathways can be continuously updated to reflect the ever-changing state of scientific knowledge. AOPs represent a hypothetical chain of events occurring across levels of biological organization that lead from a molecular initiating event (MIE) to an adverse health outcome of regulatory significance (Ankley et al. Citation2010). Key events (KEs) in each pathway are connected by causal key event relationships (KERs) that are supported by a weight of evidence in the form of the modified Bradford Hill criteria (B-H criteria) (Becker et al. Citation2015). In the context of AOP development, the relevant B-H criteria are biological plausibility, dose-response concordance, temporal concordance, essentiality of key events, and consistency. The process of collecting and reviewing literature to inform the weight of evidence across KERs in a proposed AOP is at the discretion of the AOP developer and can be dependent on the application of the AOP. Case studies using different methodologies with lessons learned are needed to help standardize data collection in AOP development.
Originally, AOPs were used to organize data for chemical and ecological risk assessment. Recent work by Chauhan et al. (Citation2021) and Preston et al. (2021) showed the benefits of expanding AOPs for use in the field of radiation, where AOPs are now being explored for research and regulation to support low dose and low dose-rate exposures. Using this study, we continue to expand AOP framework application by building a collection of pathways that explore the health effects of space travel. The human body is finely tuned for life on earth, and travel to space comes with exposure to multi-faceted physiological stressors including chronic low-dose ionizing radiation exposure and microgravity. To assess associated risks and identify countermeasures to protect future space travelers, the current state of knowledge on molecular, cellular, tissue, organism and population level effects need to be better characterized. Indeed, international radiation governing bodies (e.g. the International Commission on Radiological Protection (ICRP), National Council on Radiation Protection and Measurements (NCRP), United Nations Scientific Committee on the Effects of Atomic Radiation (UNSCEAR)) have directed efforts to understanding low dose and low dose-rate effects, and some are considering AOPs as a valuable tool to integrate knowledge from the molecular to population level (Laurier et al. Citation2021). For this reason, harmonization of approaches for evidence gathering that ensures transparency of data collection would benefit the process of AOP construction. This in turn will help facilitate the identification of the most relevant studies in the radiation field that could support the development of quantitative AOPs, whereby the empirical evidence is used for risk-model development.
Conventionally, AOP building is completed through a narrative review approach based on prior knowledge and expert opinion. Adopting systematic review (SR) tools for AOP weight of evidence collection has been proposed in order to improve objectivity, comprehensiveness, reproducibility, and transparency of the process and to create a set of standardized best practices for AOP developers of the future (de Vries et al. Citation2021). This new structure could also help with those new to AOP development since it could serve as a step-by-step guide through the process.
Although there are a number of methodologies to explore for AOP development (de Vries et al. Citation2021), for data-rich areas like the broad preliminary AOP network of the current project, a scoping review methodology may be most suitable (Arksey and O’Malley Citation2007; Munn et al. Citation2018). While full SRs are considered the highest standard in evidence search, selection, and synthesis, they are built around a focused Population, Exposure, Control, and Outcomes (PECO) question. Scoping reviews, on the other hand, have a wider scope with more expansive inclusion criteria. Scoping reviews frequently precede SRs and allow the research team to identify knowledge gaps, that a PECO-focused SR would be feasibly address. Scoping reviews do not aim to produce critically appraised and synthesized results and/or answer a particular question (Peters et al. Citation2015) instead they are used for mapping the evidence on a particular topic and identifying key concepts. Furthermore, the assessment of methodological limitations or risk of bias of the individual studies is not required (Peters et al. Citation2015). While scoping reviews are a methodology through which literature is screened, SR methodologies also contain systematic evidence maps (SEMs) that are used to visually represent the results of literature screening. SEMs are useful for at-a-glance identification of trends in broad collections of evidence and synthesizing review results in a user-friendly product (Miake-Lye et al. Citation2016; Wolffe et al. Citation2019). Our aim is to include the benefits of both scoping literature review and data summary using SEMs in AOP development.
We tested several workflows and considered steps that can be automated by exploring the inclusion of natural language processing (NLP) artificial intelligence (AI) software with the goal to accelerate literature review and alleviate the burden on human screeners. The data management aspects of the software tools have successfully been tested and validated by others (van der Mierden et al. Citation2019), but the automated screening features were not found to accurately identify relevant studies (Gartlehner et al. Citation2019; Gates et al. Citation2019). With these considerations, we opted to test the use of Distiller SR and SWIFT Review in AOP development with the following specific objectives:
Create a methodology that optimizes the inclusion of elements of scoping review tools and text mining software in the evidence collection process required for AOP construction.
Demonstrate the process using a case study that is being developed related to the radiation field.
Here, we outline a practical protocol, and present results from the screening process involved in the development of an AOP relevant to the radiation field that is comprised four health outcomes and 20 (19 KEs + 1 MIE) KEs. Additionally, we present the workflows that have been tested and discuss their merits and drawbacks. A major effort of our group is to advance radiation risk assessment applications and engage the community in the use of AOPs for this purpose. Thus, the overarching objectives are both to build radiation AOPs as well as to evaluate the use of elements of SR tools in AOP development.
2. Methods
2.1. Resources
Tools and resources used in collecting the weight of evidence for the AOP were: SWIFT Review ((Sciome Workbench for Interactive computer-Facilitated Text-mining) www.sciome.com/swift-review/ released 08.28.2019: version 1.43) and DistillerSR (Evidence Partners. www.evidencepartners.com/products/distillersr-systematic-review-software released 12.06.2020 version 2.34.0). The statistical text-mining and machine learning features of SWIFT review (such as the SWIFT tag browser feature) were used to prioritize the results of literature searches prior to human screening. Distiller was used to create structured screening forms for reviewers that facilitated reference evaluation while tracking reviewer responses and data-management. Additionally, three AOP-reference collection workflows (henceforth referred to as simply ‘workflow’ or ‘flow’) were evaluated to determine the most efficient iteration of the protocol. Lastly, the machine learning-based automated reviewer feature (Distiller Artificial Intelligence System [DAISY]) was tested for efficacy in assisting with literature screening.
2.1.1. High-level project overview
The project is comprised of three phases, as outlined in . In the completed first phase of the project, the work focused on the creation of a preliminary AOP network that is being used as a case-example and predominantly informed by studies relevant to the space environment. Phase II, which is the focus of the current work, is the creation and validation of a protocol to collect a weight of evidence for preliminary AOP developed in Phase I. The detailed results of Phase III will be published in the future as a narrative that summarizes the weight of evidence and will be input into the AOP Wiki (www.aopwiki.org), as well as visualized in SEMs.
Figure 1. Overview of the three phases of the AOP project. The current publication focuses on the second phase; the process of developing and validating a weight of evidence collection protocol. After deploying the first iteration of the reference screening workflow, changes were made to the methodology resulting in versions 2 and 3 of the scoping review literature screening workflow. Differences between the workflows are detailed in results.

2.1.2. Preliminary AOP network – Phase I
A case-study AOP network related to health outcomes relevant to space stressors was the basis for testing elements of scoping review methodologies and also developing appropriate filtering criteria to identify relevant studies that could support causal linkages to the AO. This network was initially built by screening ∼100 expert-selected articles. Studies were retrieved manually by study authors using a variety of search engines (e.g. Google Scholar and PubMed) and literature databases from the National Aeronautics and Space Administration (NASA), Canadian Space Agency (CSA), as well as from authoritative reports from these agencies. The retrieved studies and agency reports guided the development of an AOP network for the following adverse outcomes (AOs): cardiovascular disease, impaired learning and memory, bone loss, and cataracts. While cancer is another well-studied outcome of ionizing radiation, due to the chronic low dose nature of the space exposure scenario, the present work is focused on non-cancer health effects for which there is growing concern (Patel et al. Citation2020). The preliminary network contained a total of 40 adjacent KERs relationships and 20 non-adjacent KERs (Supplementary Figure 1).
2.1.3. Creation of protocol – Phase II
Following the completion of Phase I and procurement of SR software (Distiller SR), a modified scoping review protocol was developed with the aim of identifying relevant evidence to support each KER in the AOP as well as to identifying any additional KEs. The study protocol was developed based on the guidelines and principles outlined in the ENVINT PRISMA-SM-P report (available at (Elsevier Citation2017). The protocol has been registered at osf.io/t9amw.
2.1.4. Weight of evidence gathering – Phase III
The project then continued to Phase III Step 1, with the outlined study protocol being tested to collect the appropriate AOP weight of evidence. As practical experience was gained through the literature screening process, there was a return to Phase II Step 2 with amendments being made to the protocol. All amendments were logged and are detailed in the results. Throughout the testing and amending of the protocol, three distinct workflows ( of Results) were produced. The first test of the Phase II Step 2 workflow (hereafter referred to as flow 1) was the first iteration process, the two subsequent versions (flow 2 and flow 3) were modified from flow 1 to address inefficiencies of the first approach.
2.2. Information retrieval
All literature searches to support Phase II of the AOP construction were developed by a Health Canada librarian (RH) on Ovid Medline, with no language or date restrictions. A Preferred Reporting Items for Systematic Reviews and Meta-Analyses (PRISMA) (Moher et al. Citation2009) study flow diagram (Supplementary Figure 2) was created to track the number and source of the references collected. Any additional articles sourced outside of the literature searches (e.g. passed along from subject matter experts or the reference sections of review articles) were marked as ‘Other Sources’ on the study flow diagram.
To identify relevant studies, a series of keywords, assays/endpoints, and applicable Medical Subject Headings (MeSH) terms were collected to describe each of the components (MIE, KE, and AO) of the AOP network (lists of keywords and endpoints representing each AO are available in Supplementary Material). The list of endpoints defined the measurement technique for each of the KEs, which ensured consistency in study identification across multiple screeners. The literature searches were conducted by a librarian and were divided into three sets: 1) MIE to AO searches; 2) independent KER searches; 3) KER searches that were pooled together along with MIE to AO searches. MIE to AO searches were broad, overarching searches that captured any studies discussing each of the AOs in the context of relevant exposure conditions and allowed the identification of KEs related to the network. In contrast, the KER searches focused on studies that explored the relationships between specific KEs. To manage the number of studies and retrieve relevant articles of significance to the space field all searches were filtered using space exposure terms. If KER searches returned insufficient references, the scope was broadened to include references from chemical stressors.
Set 1 – MIE to AO searches (used for Workflow 1 only)
Set 2 – KER searches (used for Workflow 2)
Set 3 – Pooled searches (used for Workflow 3)
A full list of the completed search strategies is available in the supplementary material. The search strategy was validated using the ∼100 articles manually curated for creation of the preliminary AOP network. When the pilot searches retrieved articles from the manually collected list, it provided confidence in the search workflow.
2.3. Eligibility criteria
To be deemed eligible for the workflow, studies had to be published, peer-reviewed articles written in English. Letters to the editor, opinion pieces, editorials, press releases, advertisements, books, book chapters, theses, conference abstracts, or proceedings and posters were not considered. Additionally, study eligibility was the same for all three workflows and was determined by the PEOE criteria outlined in . Meaning, to be eligible, a study must have explored one type of evidence from the population, exposure, and outcomes or endpoints element (P and E and (O or E)). One type of evidence per element was sufficient; a study did not need to consider every type of evidence in each element (e.g. a study needed to only consider one population of interest in the listed items shown in ). These PEOE criteria specify the defined space-relevant stressors in the context of the current work.
Table 1. The PEOE statement (Population, Exposure, Outcomes and Endpoints) used to inform the inclusion and exclusion criteria for the scoping review.
2.4. Data management
Although many tools are available for data management (van der Mierden et al. Citation2019), after consultation with experts in systematic methodologies, Distiller was selected as a reliable tool for the this project. Structured literature screening forms were created (Supplementary Figure 3) that allowed references to either continue through the workflow or be excluded. Metadata for each reference was tracked including publication information, inclusion/exclusion decisions, and responses to form questions. Distiller was also used to create PRISMA diagrams that tracked the progression of all the references through the workflow (Supplementary Figure 2).
2.5. Selection and data collection process
In selecting data, filtering criteria were employed that prioritized radiation studies of all qualities and stressors relevant to the space environment. Additionally, by prioritizing searches, it facilitated identifying relevant articles, and limiting the burden of review for human screeners.
Following the literature database search, the results were imported into SWIFT Review (SWIFT) where the SWIFT-generated tags were used to triage studies according to the following levels of priority:
Evidence stream: human > in vivo > in vitro > in silico
Dose: low > moderate > high
Exposure: space environment > ionizing radiation stressors > non-ionizing radiation stressors > chemical.
Articles triaged using SWIFT to identify the highest priority references (e.g. were tagged by SWIFT with the greatest simultaneous number of high priority tags) were exported into Distiller where they were screened in a three-level process. The DAISY re-rank setting was enabled for all include/exclude levels of Distiller screening; this feature learns from the decisions of human screeners and continuously ranks unscreened articles to bring more relevant articles to the top of the screening queue.
Level 1 – Title and Abstract were screened by two screeners (JK, SS, DF, VL, MA, NA, and TK) who evaluated adherence to the PEOE criteria. Screener consensus was required for progression or exclusion; conflict resolution was completed by a discussion between the reviewers and resolved by a third reviewer (RW and VC) as necessary. The screening was piloted to ensure conflicts did on exceed 10% of the total number of articles screened.
Level 2 – Full text was screened by two screeners (JK, SS, DF, VL, NA, and TK) to verify that the full text was a peer-reviewed published article, available in English, and adhered to the PEOE criteria. Screener consensus was required for progression or exclusion; conflict resolution was accomplished by a discussion between the reviewers and resolved by a third reviewer (RW and VC) as necessary.
Level 3 – Full text was used for data extraction.
2.6. Data extraction
Data extraction was completed in Distiller at Level 3 of the screening workflow using customized template forms. Key data points collected at this level were which KE/KER(s) were investigated, what element of the BH criteria is examined, as well as whether the study investigated any KE/KER(s) that were not currently in our proposed network, and if any confounding factors were studied. The full list of data extraction and coding questions is available in Supplementary Figure 3(c,d).
2.7. Testing distiller automated screener
KER searches completed for the cardiovascular disease AOP were used to test the efficacy of the Distiller automated screener. Individual KER searches were triaged in SWIFT then imported to Distiller. Screening at the first two levels was completed by one human screener and the Distiller Automated Screener feature replaced the second screener (semi-automated screening). Any conflicts between the decisions of the human screener and the AI were logged.
3. Results
3.1. Phase I: preliminary AOP development
Phase I of AOP construction began with a preliminary screening of the literature to identify the authoritative and relevant review articles for the stressor(s) and AO(s) of interest. This was achieved via a nonsystematic approach using search terms relevant to the stressor(s) and AO(s) as the anchoring points. In this stage, it was important to identify the relevant review articles in the field, including documents generated by the international radiation governing bodies. Assembly of these documents facilitated identification of the mechanistically well-defined KEs/KERs for the preliminary network (Supplementary Figure 1). This preliminary screen was managed without the use of specialized software.
3.2. Phase II: study protocol development
The methods used for assembling knowledge to support the AOP network developed in Phase I were documented in the form of a study protocol. This protocol defined the study question, the SR software employed, information sources, databases, search strategy, and inclusion/exclusion criteria. These design features and the technical input (e.g. assay glossary Supplementary Table 1) are an essential aspect for an effective and reliable process. The format of the protocol was developed using Pelch et al. (Citation2019) as the example. In total, as of January 2022 over 34,000 articles were retrieved using the protocols search strategy, with over 3300 over them being reviewed by human reviewers. A PRISMA flow diagram was generated to track the screening process (Supplementary Figure 2).
3.3. Phase III: evidence gathering
Phase III of the process involved identifying studies that met B-H criteria through the rigorous evidence-gathering protocol outlined in Phase II. This required the development of search logic; with the help of the librarian (RH), a database of studies was identified using search strategies for KEs, KERs, MIE, and AOs.
Each of the databases was then taken through level 1 () of screening to determine which best-identified studies met the PEOE statement. In the process of testing the scoping review protocol, three iterations of the workflow evolved (). Once studies passed level 2 screening in Distiller they went into full data extraction. This process extracted the relevant information from each study to help develop the causality statements for AOP development.
Figure 2. Overview of scoping review protocol. References retrieved by a literature search were prioritized in SWIFT (shown in side panel) to identify the most relevant references before being imported to Distiller for a three-level literature screen (outlined in blue). ‘Other Sources’ refers to references acquired outside of the literature searches (e.g. passed along from subject matter experts or the reference sections of review articles).

3.4. Flow 1 – screening the MIE to AO search
The first flow (Flow 1, ) used search terms specific to only the MIE and AO (MIE to AO search). Results from this search were uploaded to Distiller and screened at all three levels. This search strategy yielded thousands of references for each of the four pathways (Cognitive: 6645, Cardiovascular: 3923, Cataracts: 1671, and Bone Loss: 3154). After the second level of screening, the majority were found to not support any B-H criteria. A benefit of this method was the identification of a collection of literature that was later used to validate the inclusion of later proposed KEs and novel KERs ().
Figure 4. Validation of alternative KEs using the MIE to AO literature search. Following expert suggestion of alternative KEs for the cardiovascular disease pathway, SWIFT tag browser feature was used to explore the titles and abstracts of the cardiovascular MIE to AO literature search. Values represent the number of references returned when using combinations of given KEs, highlighting potential for novel KERs.

3.5. Flow 2 – individual KER searches using SWIFT
To address the challenges of flow 1, adjustments were made and flow 2 was generated in which relevant literature was retrieved for each of the KERs in the AOP (). The results of the KER-specific literature searches were then uploaded to SWIFT review, where the statistical text mining and machine learning tools are used to prioritize references based on their association to the PEOE statement (Side panel – ). Following the AI prioritization, human screeners reviewed the list to select the top group of relevant references to then imported into Distiller. Inputting references through SWIFT allowed us to triage references based on tags, such as type of exposure, model tested, and health outcome. This ensured human attention could be prioritized for references that hit the greatest number of categories of interest first. This flow greatly reduced the number of full-text articles requiring review by human screeners, and thereby saved human resources for articles of greatest relevance. Furthermore, it was flexible in that it allowed reviewers to return to a specific KER search and broaden criteria in SWIFT if the original references identified were insufficient. Finally, this flow also simplified project management since screening could be completed in a methodical fashion, with one KER being taken from screening to data extraction at a time.
3.6. Flow 3 – Pool of all KER searches using SWIFT
The last third flow shared many similarities with the second flow; however, the results from literature searches for all KERs were combined and screened collectively (). Individual literature searches were completed for each KER and then all resulting files were collectively uploaded to SWIFT. In SWIFT, references were prioritized based on exposure and model tags before being uploaded to Distiller. Overall, using the scoping review approach was found to have a number of advantages as highlighted through the example of the cardiovascular pathway. By using this approach, we have been able to transparently adapt the preliminary pathway to accurately represent the data informing the weight of evidence. This is exemplified in the SEMs of that show the quantity as well as qualitative attributes of the weight of evidence collected and subsequent changes reflected to the pathway (). For example, KE23 ‘Altered NO levels’ was added following screening (). The addition of this KE was facilitated through screener responses to the question ‘Does the study support a KE or KER not currently included in the proposed AOP?’ in the screening form (Supplementary Figure 3(a,b)), as well as through expert recommendation that was validated using the MIE to AO literature search database (). Using these methods provided documentation and support for the additions made to the network, thereby increasing the transparency of the process. Furthermore, KE14 (protein modification/expression changes) and the original AO (cardiovascular disease) were removed from the network (). Removal of a KE from the network does not negate biological relevance; instead, as the SEM of highlights, it shows that empirical evidence in the form of time, dose, and incidence concordance (demonstrating the essential and causal connectivity) was not identified. Another hurdle was clearly defining the scope of each KE in terms of the endpoints and translating this to screeners through training and detailed protocols to ensure consistency in data retrieval with minimal conflicts.
Figure 5. (a) Systematic evidence map (SEM) illustrating quantity of evidence across all the KERs considered for the cardiovascular pathway. Arrow size indicates relative evidence weight, while the size of the KE circles represents relative degree of connectivity (as determined by number of connections up and downstream from the KE). Values represent the number of references supporting each KER; in order the support a KER a reference must demonstrate at least one of the Bradford–Hill (BH) criteria. (b) Systematic evidence map (SEM) illustrating qualitative aspects of the weight of evidence supporting the cardiovascular pathway. i) illustrates the taxonomic applicability breakdown, ii) the Bradford-Hill criteria supported and iii) the stressors considered by the studies. ‘Multiple stressors’ encompasses studies that explored more than one stressor simultaneously, while ‘other’ includes any other exposures from the PEOE table not otherwise listed (hydrogen peroxide (radiation mimetic), environmental CO2, atmospheric gas, and space environment/conditions).

Figure 6. Updates to the preliminary cardiovascular AOP. Changes to the preliminary pathway were made following literature screening to reflect the contents of the weight of evidence. Any KEs/KERs with insufficient empirical evidence or no additional evidence from that already in the AOP Wiki (www.aopwiki.org) are grayed out, KE titles that have been updated are highlighted in red with original titles faded out, and any newly added KEs/KERs are represented in green. The MIE is in yellow, while the AO in dark blue.

3.7. Results for testing the distiller automated screener
In using the Distiller automated screening feature to assist with the screening of cardiovascular KER searches, we noted that the feature performed better for the title and abstract level than the full-text level. The first KER screened with the automated reviewer (KE16 (vascular remodeling) + AO) generated conflicts at Level 1 (title and abstract screening) with the automated screener disagreeing with the human screener’s inclusion/exclusion choice 39% of the time (). However, for all other KERs screened, the automated screener and human screener agreed for all inclusion/exclusion decisions (). In Level 2 (full-text screening), there was a noticeable increase in conflicts between the human and automated reviewer (ranging all the way from 0 to 100%), with full agreement only occurring for one single KER.
Table 2. Percentage of conflicts in inclusion/exclusion decisions between human screener and Distiller Automated Screener in screening KER searches for the cardiovascular disease pathway.
4. Discussion
In this work, a suggested workflow is described for the development of AOPs using SR tools. This includes a clearly documented path to identify a strategy for assembling the appropriate evidence to support the KERs that ensures transparency, objectivity, and reproducibility in data retrieval. The fundamental principles for AOP development provided by the OECD Users’ Handbook Supplement (OECD Citation2018) were followed throughout the development process. With the addition of elements of SR tools, we produced a methodology that enables efficient and transparent study retrieval, harmonized reporting, and the eventual selection of relevant studies for the development of quantitative AOPs in the future.
Increasing the standardization of AOP development has been an ongoing discussion, from a development of best practices for AOP descriptions (Villeneuve et al. Citation2014), to suggestions of systematic collection of evidence as a next step for the framework (Leist et al. Citation2017; Svingen et al. Citation2021). Bridging the fields of SRs and AOP development and the inclusion of AI techniques to facilitate literature screening have also been identified as methods to increase certainty and confidence in the AOP framework (de Vries et al. Citation2021). To our knowledge, this is the first time that scoping review protocols have been tested for the development of a case study AOP network.
In selecting methods most efficient in retrieving relevant articles to support AOP development, three workflows evolved. The simplest workflow was built from search terms related to the MIE and AO (Flow 1). It was envisioned that this workflow would identify new KEs that were not considered in the preliminary AOP as well as studies to support the modified BH criteria. This workflow, however, retrieved the least number of relevant studies, despite having the greatest number of articles to review. The number of references retrieved was in the thousands, and many did not include empirical data to evaluate the B-H criteria. A specific challenge was that references that explored KEs (but not KERs) would fulfill the PEOE statement at Level 1 and pass forward for full-text review, overwhelming screeners with hundreds of non-AOP relevant full-text articles to review. Modifications of the PEOE statement were discussed, but it was determined that any changes would inadvertently exclude relevant references. However, this method alongside expert consultation did identify a collection of literature that was used to propose new KEs that were not present in the preliminary network. The SWIFT tag browser feature was then used to identify the number of studies that, in their title and abstracts, referred to combinations of these alternative KEs. The representative example is provided in the heat map () that highlights the number of studies within the MIE to AO search that discuss novel KERs. Overall, the methodology of flow 1 is not recommended for identifying studies that support the causality of KERs, as more efficient workflows were identified. However, the search strategies used in this workflow are a viable option for identifying a body of literature that is useful for validating novel or alternative KEs for further exploration.
Flow 2 () involved independent KER searches, with SWIFT prioritization. This flow was both resource- and time-efficient for the reviewers. However, this method requires significantly more investment from information specialists enlisted in reference retrieval. No quantitative measures of efficiency improvements were taken; however, the number of references for human screening was reduced from thousands in flow 1 to 20–30 articles, representing a considerable decrease in human resource demand. Despite increased efficiency through inclusion of SWIFT, a drawback was the training requirements for use of this new software tool. Additionally, there was reference redundancy; there were instances where articles that measured multiple KERs would appear in multiple literature searches and would need to be triaged in SWIFT multiple times. Nonetheless, this workflow is recommended for those working in a broad literature space as focused KER searches were efficient for identifying relevant articles, and SWIFT was an effective tool for prioritizing references for human review.
Flow 3 can be viewed as an alternative approach to Flow 2. It has all the same benefits of increased efficiency (for the screeners) and a high success of finding appropriate articles. Pooling the literature was beneficial, since many relevant references discuss multiple KERs or KEs in one article and articles were being retrieved by numerous searches. By pooling all the results together, duplicates were removed in one step rather running the Distiller de-duplicate feature for each search. Overall, flow 3 was a variation on flow 2 that marginally increased efficiency through removing redundant references earlier in the workflow. However, it does require more training for incoming screeners since it combines the use of Distiller as well as a more in-depth use of SWIFT. Additionally, like flow 2, flow 3 requires greater time investment from library or information specialists. Both flow 2 and flow 3 are equally efficient in the identification of appropriate studies.
Distiller’s automated screener was qualitatively tested and found to have variable accuracy, especially outside of title and abstract screening levels (). This is in line with the work done by Gates et al., which found that semi-automated use of Distiller semi-automated had no improvement on a single reviewer (Gates et al. Citation2019). Overall, Distiller was found to be most useful for its unique literature review project management features. This does not negate the potential for AI inclusion to the AOP development workflow. Teams have had success incorporating a variety of AI approaches (approaches outside the scope of the present project) to their construction of AOP pathways (Carvaillo et al. Citation2019; Jeong et al. Citation2019; Rugard et al. Citation2020). AOP developers working on pathways with a more focused question and defining the technical terminology, such as assay names that delineate the endpoints, may have better success in incorporating current tools in their process.
Our methods of evidence collection also lend themselves to the summary through SEMs that will enable exploration of the weight of evidence that supports our AOP network (). The structured screening forms (Supplementary Figure 3) contained questions that give important information about the nature of the studies screened. SEMs have been created () that demonstrate how the collected data collected can be visually represented and used to justify changes to the network as well as identify knowledge gaps.
Each of the tools and approaches discussed herein has the potential to contribute to AOP weight of evidence collection. The use of these tools relies on training, personnel, and time. As such, we recommend a fit-for-purpose approach (Supplementary Figure 4) based on availability of resources. Our recommendation is for the base-level literature screen to contain the following: structured and recorded literature searches, pre-determined inclusion/exclusion criteria, creation of PRISMA diagrams, and SWIFT triage of references in data-rich areas. These tools are all free and would already vastly improve upon the narrative review approach. Resource permitting, we then recommend supplementing the base-level approach with the addition of structured screening/data extraction forms and project management tools of Distiller, as well as multiple reviewers at all inclusion/exclusion levels, and search strategies in more than one database. Future efforts may include validating our methodology further using a previously well-defined AOP. Teams with the resources to go beyond what was explored in our work could include an additional structured risk of bias analysis step to their workflow. This can be accomplished through using tools, such as the Office of Health Assessment and Translation (OHAT) Risk of Bias Rating framework (Office of Health Assessment and Translation [OHAT] Citation2019) to systematically evaluate weight of evidence quality. Lastly, in all cases, we recommend considering literature retrieval logistics during the project planning phase as it is important for managing project timelines.
In the broader context, AOPs have been gaining momentum as a framework to support the radiation field, particularly in low dose and low dose-rate research. To continue advancing adoption from the community, the process of transparent data collection and documentation will be a critical step. Particularly, if AOP-based approaches are to be used by the international radiation governing bodies and relevant stakeholders, the approach needs to be recognized as a means to produce transparent and objective literature-based narratives, where data-retrieval can be verified. We propose that the standardization of AOP development, including the identification of the optimal AOP-reference collection workflow described in this work is valuable to transparent data retrieval. It helped identify the approach which that yielded the most relevant studies within broad literature spaces, a process that is central to the development of quantitative AOPs and an area of great interest to the radiation field.
In conclusion, our work supports larger efforts to standardize AOP development. We believe that the use of elements of scoping review methodologies present value and can be incorporated in a number of ways for screening articles for AOP weight of evidence collection. We outline three tested workflows and discuss the benefits and hurdles. Additionally, we have provided detailed recommendations for future AOP builders looking to define their methodology. Overall, the most flexible approach applied the use of filtering and screening software such as SWIFT and Distiller, as well as structured literature searches across each of the KERs in the AOP with a predetermined inclusion and exclusion criteria, multiple screening levels, and documentation of reference progression through the screening. The addition of these systematic tools is an improvement to the traditional narrative approach of AOP weight of evidence collection as it reduced bias, increased transparency, and a method for processing large volumes of primary research.
4._Supplementary_Material_2_-_Search_Strategies.docx
Download MS Word (630.6 KB)0.2_Supplementary_Figures_and_Tables.docx
Download MS Word (1.7 MB)Disclosure statement
No potential conflict of interest was reported by the author(s).
Additional information
Funding
Notes on contributors
Tatiana Kozbenko
Tatiana Kozbenko, M.Sc. student in Biology and Bioinformatics at the University of Ottawa, and a Research Affiliate with the Consumer and Clinical Radiation Protection Bureau at Health Canada.
Nadine Adam
Nadine Adam, M.Sc., is a Laboratory Biologist at Health Canada, and previously worked with the Consumer and Clinical Radiation Protection Bureau. She completed her M.Sc. studies in Biochemistry at the University of Ottawa.
Vita Lai
Vita Lai, M.Sc., is a contract research technician at the Ionizing Radiation Health Sciences Division of the Consumer and Clinical Radiation Protection Bureau at Health Canada.
Snehpal Sandhu
Snehpal Sandhu is a student at the University of Ottawa studying Biomedical Sciences with an option in Neuroscience and is working in a co-op placement at Health Canada.
Jacqueline Kuan
Jacqueline Kuan is a University of Ottawa student studying Biopharmaceutical Science, former co-op student, and current part-time employee of the Health Canada Consumer and Clinical Radiation Protection Bureau.
Danicia Flores
Danicia Flores is a Carleton University student studying Biology with a concentration in Health Science and was in a co-op placement with Health Canada before continuing on as a laboratory technician.
Meghan Appleby
Meghan Appleby is a Carleton University student studying Neuroscience and Mental Health and is in a co-op placement with Health Canada
Hanna Parker
Hanna Parker is a University of Ottawa undergraduate student majoring in Biomedical Science and completing an Honour’s Thesis project.
Robyn Hocking
Robyn Hocking, MLIS, is a Research Librarian at the Health Canada Library.
Katya Tsaioun
Katya Tsaioun, Ph.D., Executive Director, Evidence-based Toxicology Collaboration at Johns Hopkins Bloomberg School of Public Health (EBTC). She is a systematic review methodologist, who leads multistakeholder working groups aiming to bring evidence-based approaches, like Systematic Literature Reviews and Systematic Maps, to the field of toxicology.
Carole Yauk
Carole Yauk, Ph.D, is a Professor in the Department of Biology, University of Ottawa, where she holds the Canada Research Chair in Genomics and the Environment. Dr. Yauk serves as a Canadian delegate to the OECD's Extended Advisory Group on Molecular Screening and Toxicogenomics. Within this group, she contributed to the development of the AOP Users' Handbook and is an AOP developer and reviewer.
Ruth Wilkins
Ruth Wilkins, Ph.D, is the Division Chief of the Ionizing Health Sciences Division at the Consumer and Clinical Radiation Protection Bureau. of Health Canada. She is the Canadian alternate representative of the United Nations Scientific Committee on the Effects of Atomic Radiation (UNSCEAR).
Vinita Chauhan
Vinita Chauhan, Ph.D, is a Senior Research Scientist at the Consumer and Clinical Radiation Protection Bureau of Health Canada. She is a Canadian delegate of the HLG-LDR and Extended Advisory Group on Molecular Screening and Toxicogenomics (EAGMST) of the OECD. She chairs the HLG-LDR Rad/Chem AOP Joint Topical Group and is the co-founder of Canadian Organization of Health Effects from Radiation Exposure (COHERE) initiative.
References
- Ankley GT, Bennett RS, Erickson RJ, Hoff DJ, Hornung MW, Johnson RD, Mount DR, Nichols JW, Russom CL, Schmieder PK, et al. 2010. Adverse outcome pathways: a conceptual framework to support ecotoxicology research and risk assessment. Environ Toxicol Chem. 29(3):730–741.
- Arksey H, O’Malley L. 2007. Scoping studies: towards a methodological framework. 10.1080/1364557032000119616.
- Becker RA, Ankley GT, Edwards SW, Kennedy SW, Linkov I, Meek B, Sachana M, Segner H, Van Der Burg B, Villeneuve DL, et al. 2015. Increasing scientific confidence in adverse outcome pathways: application of tailored bradford-hill considerations for evaluating weight of evidence. Regul Toxicol Pharmacol. 72(3):514–537.
- Carvaillo JC, Barouki R, Coumoul X, Audouze K. 2019. Linking bisphenol S to adverse outcome pathways using a combined text mining and systems biology approach. Environ Health Perspect. 127(4):47005.
- Chauhan V, Sherman S, Said Z, Yauk CL, Stainforth R. 2021. A case example of a radiation-relevant adverse outcome pathway to lung cancer. Int J Radiat Biol. 97(1):68–84.
- de Vries RBM, Angrish M, Browne P, Brozek J, Rooney AA, Wikoff DS, Whaley P, Edwards SW, Morgan RL, Druwe IL, et al. 2021. Applying evidence-based methods to the development and use of adverse outcome pathways. ALTEX - Alternat Anim Exp. 38(2):336–347.
- Elsevier. 2017. Guidance notes for authors of systematic reviews, systematic evidence maps and related manuscript types. Amsterdam: Elsevier.
- Gartlehner G, Wagner G, Lux L, Affengruber L, Dobrescu A, Kaminski-Hartenthaler A, Viswanathan M. 2019. Assessing the accuracy of machine-assisted abstract screening with DistillerAI: a user study. Syst Rev. 8(1):277.
- Gates A, Guitard S, Pillay J, Elliott SA, Dyson MP, Newton AS, Hartling L. 2019. Performance and usability of machine learning for screening in systematic reviews: a comparative evaluation of three tools. Syst Rev. 8(1):163.
- Jeong J, Garcia-Reyero N, Burgoon L, Perkins E, Park T, Kim C, Roh JY, Choi J. 2019. Development of adverse outcome pathway for PPARγAntagonism leading to pulmonary fibrosis and chemical selection for its validation: toxcast database and a deep learning artificial neural network model-based approach. Chem Res Toxicol. 32(6):1212–1222.
- Laurier D, Rühm W, Paquet F, Applegate K, Cool D, Clement C, International Commission on Radiological Protection (ICRP). 2021. Areas of research to support the system of radiological protection. Radiat Environ Biophys. 60(4):519–530.
- Leist M, Ghallab A, Graepel R, Marchan R, Hassan R, Bennekou SH, Limonciel A, Vinken M, Schildknecht S, Waldmann T, et al. 2017. Adverse outcome pathways: opportunities, limitations and open questions. Arch Toxicol. 91(11):3477–3505.
- Miake-Lye IM, Hempel S, Shanman R, Shekelle PG. 2016. What is an evidence map? A systematic review of published evidence maps and their definitions, methods, and products. Syst Rev. 5(1):1–21.
- Moher D, Liberati A, Tetzlaff J, Altman DG, Altman D, Antes G, Atkins D, Barbour V, Barrowman N, Berlin JA, et al. 2009. Preferred reporting items for systematic reviews and meta-analyses: the PRISMA statement. PLoS Med. 6(7):e1000097.
- Munn Z, Peters MDJ, Stern C, Tufanaru C, McArthur A, Aromataris E. 2018. Systematic review or scoping review? Guidance for authors when choosing between a systematic or scoping review approach. BMC Med Res Methodol. 18(1):1–7.
- OECD. 2018. Users’ handbook supplement to the guidance document for developing and assessing adverse outcome pathways. (OECD Series on Adverse Outcome Pathways No. 1). Paris, France: OECD.
- [OHAT] Office of Health Assessment and Translation. 2019. Handbook for conducting a literature-based health assessment using OHAT approach for systemic review and evidence integration. Washington (DC): U.S. Department of Health and Human Services.
- Patel ZS, Brunstetter TJ, Tarver WJ, Whitmire AM, Zwart SR, Smith SM, Huff JL. 2020. Red risks for a journey to the red planet: the highest priority human health risks for a mission to mars. NPJ Microgravity. 6(1):1–13.
- Pelch KE, Reade A, Wolffe TAM, Kwiatkowski CF. 2019. PFAS health effects database: protocol for a systematic evidence map. Environ Int. 130:104851.
- Peters MDJ, Godfrey CM, Khalil H, McInerney P, Parker D, Soares CB. 2015. Guidance for conducting systematic scoping reviews. Int J Evid Based Healthc. 13(3):141–146.
- Preston RJ, Rühm W, Azzam EI, Boice JD, Bouffler S, Held KD, Little MP, Shore RE, Shuryak I, Weil MM. 2021. Adverse outcome pathways, key events, and radiation risk assessment. Int J Radiat Biol. 97(6):804–814.
- Rugard M, Coumoul X, Carvaillo JC, Barouki R, Audouze K. 2020. Deciphering adverse outcome pathway network linked to bisphenol F using text mining and systems toxicology approaches. Toxicol Sci. 173(1):32–40.
- Svingen T, Villeneuve DL, Knapen D, Panagiotou EM, Draskau MK, Damdimopoulou P, O’Brien JM. 2021. A pragmatic approach to adverse outcome pathway development and evaluation. Toxicol Sci. 184(2):183–190.
- van der Mierden S, Tsaioun K, Bleich A, Leenaars CHC. 2019. Software tools for literature screening in systematic reviews in biomedical research. ALTEX. https://doi.org/10.14573/altex.1902131
- Villeneuve DL, Crump D, Garcia-Reyero N, Hecker M, Hutchinson TH, LaLone CA, Landesmann B, Lettieri T, Munn S, Nepelska M, et al. 2014. Adverse outcome pathway development II: best practices. Toxicol Sci. 142(2):321–330.
- Wolffe TAM, Whaley P, Halsall C, Rooney AA, Walker VR. 2019. Systematic evidence maps as a novel tool to support evidence-based decision-making in chemicals policy and risk management. Environ Int. 130:104871.