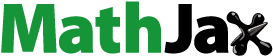
ABSTRACT
We investigated the relationships between cognitive change following stroke, awareness of cognitive impairments, and mood to further understanding of change processes influencing psychological outcomes post-stroke in line with the “Y-shaped” process model. Patients (n = 143; Mage = 73 years, SD = 13.73; 74 males) were assessed at 3-weeks (T1) and 6-months (T2) post-stroke and had completed the Oxford Cognitive Screen (T1 and T2), the Cognitive Failures Questionnaire (CFQ; T2), and the Hospital Anxiety and Depression Scale (HADS; T2). An ANCOVA controlling for disability relating to activities of daily living (ADL) revealed that awareness of cognitive impairment was significantly lower in participants with moderate-severe cognitive impairment. Regression analysis indicated that greater awareness of cognitive impairment and reduced independence in ADL were associated with greater emotional distress at T2. Cognitive improvement was associated with lower emotional distressat T2. Contrary to the awareness hypothesis, moderation analyses suggest that this effect was largest for those most cognitively impaired at T1. Findings emphasize the importance of monitoring stroke patients’ capacity to be self-aware when assessing and formulating long-term post-stroke distress and have potential implications for improving long-term emotional status in those most cognitively impaired post-stroke, e.g., through psychoeducation, cognitive rehabilitation, and emotional support.
Introduction
Stroke is the fourth most common cause of death and the leading cause of complex disability in the UK (Adamson et al., Citation2004; Office for National Statistics, Citation2018). Post-stroke depression and anxiety are common psychological consequences of stroke, with prevalence estimated to be 15–40%, and 18–24% respectively (Knapp et al., Citation2020; Mitchell et al., Citation2017; Wu et al., Citation2019). Long-term emotional distress in stroke survivors has been shown to impact quality of life, physical recovery, mortality, and cognitive impairment (Ayerbe et al., Citation2013; Cai et al., Citation2019; Donnellan et al., Citation2010; Ezema et al., Citation2019; Kim et al., Citation2018; Williams & Demeyere, Citation2021). The number of individuals living with the long-term physical and psychological effects of stroke in the UK is predicted to increase by >60% by 2035, with the cost of social care for stroke survivors projected to increase by 250% in this period (King et al., Citation2020). Consequently, it is imperative to identify patients at risk of adverse emotional and functional outcomes after stroke to optimize support from services through early detection and treatment. In this, it is necessary to develop our understanding of the psychological change processes following stroke that may increase the risk of adverse outcomes in the stroke population, as this could lead to tailored preventative interventions that target such processes in these patients.
The “Y-shaped” process model (subsequently referred to as the Y-shaped model; Gracey et al., Citation2009) indicates how biological, psychological, and social factors can interact to determine outcomes following acquired brain injury (ABI). The model suggests that following ABI, individuals undergo a process of adaptation and reintegration into society, which initially involves them developing awareness, understanding, and adaptive resolution of social and psychological discrepancies in their sense of self. Gracey et al. (Citation2009) set out three key discrepancies that survivors may experience following ABI: social, interpersonal, and personal discrepancies. It is proposed that when a stroke survivor is aware of such discrepancies, they may experience psychological threat regarding feared and actual catastrophic meanings associated with their post-injury situation. This may lead them to experience automatic emotional reactions and to adopt safety-seeking behaviours to cope with such threats. The model hypothesizes that safety-seeking behaviours can reduce threat-to-self in the short term. However, over time this can prevent the individual from resolving discrepancies, increasing their risk of experiencing poor long-term mental health and psychosocial outcomes.
The Y-shaped model draws on evidence regarding an individual’s “awareness” of changes to their sense of self post-injury. “Awareness” in the context of disability is understood as one’s ability to recognize deficits, understand the functional implications of these deficits, and set realistic goals accordingly (Ownsworth et al., Citation2007). Awareness can relate to an individual’s insight into a specific deficit in their sensory, motor, perceptual, behavioural, or cognitive functioning (Orfei et al., Citation2009). Awareness typically increases in the years following brain injury, with awareness of physical changes typically preceding awareness of cognitive and behavioural changes (Hart et al., Citation2004, Citation2009). In the literature, “awareness” in the context of brain injury is commonly operationalized as the difference between an individual’s subjective account of their deficits and either an objective test of their deficits or a second rater of that individual’s deficits (Boosman et al., Citation2014; Hoofien et al., Citation2004; Ownsworth et al., Citation2007). However, there is currently no gold-standard measure of awareness (Bogod et al., Citation2003; Geytenbeek et al., Citation2017).
Evidence suggests that awareness is not a unitary or “all or nothing” construct and that the level and direction of self-awareness will depend on the interaction of neurological, psychological, and socio-environmental factors at any one time (Fleming & Ownsworth, Citation2006; Gracey et al., Citation2009; Ownsworth et al., Citation2007). Literature exploring the epidemiology of awareness of cognitive impairment indicates that under-reporting cognitive impairment following stroke may be primarily due to organic changes in the brain, which inhibits one’s ability to understand the extent of one’s cognitive impairment (Boosman et al., Citation2014; Ownsworth et al., Citation2007). However, evidence suggests that psychological defence mechanisms, such as denial, may also contribute to the long-term under-reporting of stroke-related impairments in this population (Ownsworth et al., Citation2002). In contrast, subjectively over-reporting the extent of one’s cognitive impairment may be primarily due to psychological mechanisms such as attentional bias, which may contribute to hyper-awareness (Boosman et al., Citation2014; Ownsworth et al., Citation2007). Evidence suggests that those who are hyper-aware of cognitive impairment are more likely to experience clinically significant psychological distress (Boosman et al., Citation2014; Carroll & Coetzer, Citation2011; Geytenbeek et al., Citation2017; Morton & Barker, Citation2010; Ownsworth et al., Citation2007). In line with this, literature indicates that subjective awareness of cognitive impairment influences emotional wellbeing in individuals with long-term disabilities, such as stroke, more than objective cognitive impairment (OCI) alone (Mitrushina & Tomaszewski, Citation2020; Van Rijsbergen et al., Citation2019). Evidence suggests that this relationship between emotional distress and perceived cognitive impairment is likely to be bi-directional, in that emotional distress can undermine cognitive abilities and maintain or exacerbate negative attentional bias to cognitive impairment (Chamelian & Feinstein, Citation2006; Wallace & Bogner, Citation2000).
Based on the Y-shaped model, we can hypothesize that the emotional consequences of cognitive change in the first 6-months following stroke may differ depending on the level of acute cognitive impairment. Specifically, we expect that individuals with greater cognitive impairment present with lower levels of awareness immediately post-stroke in line with previous literature (Boosman et al., Citation2014; Ownsworth et al., Citation2007). Following this reasoning, we would then also expect that if these individual’s cognition improves, they may experience, and report higher levels of distress compared to those who are not improving and to those whose cognition has remained relatively intact. Specifically, they may gain access to the cognitive skills which enable them to process their situation, potentially leading to greater self-discrepancy. On the other hand, individuals with less acute cognitive impairment and higher levels of initial distress may report lower levels of distress following cognitive improvement, due to a perceived reduction in self-discrepancy.
The present study thus set out to answer the following research questions:
Preliminary cross-sectional research questions and hypotheses:
1. What is the relationship between cognitive impairment and awareness of cognitive impairment at 6-months post-stroke?
H1: There will be a significant difference in awareness of cognitive impairment between the 'moderate-severe cognitive impairment' and 'mild-no cognitive impairment' groups at 6-months post-stroke.
2. What is the relationship between awareness of cognitive impairment and emotional distress at 6-months post-stroke?
H2: There will be a significant association between awareness of cognitive impairment and emotional distress at 6-months post-stroke.
Primary longitudinal research question and hypothesis:
3. Does severity of acute post-stroke cognitive impairment moderate the relationship between cognitive change and emotional distress in the first 6-months months following stroke?
H1: The effect of cognitive change between subacute and 6-months post-stroke on mood at 6-months post-stroke will be moderated by subacute cognitive impairment.
Findings may have important implications for psychological intervention. For instance, through supporting resource-based decision-making with regards to targeted cognitive behavioural interventions for stroke survivors at risk of long-term adverse mood outcomes.
Materials and methods
Design and participants
The study used both a cross-sectional and longitudinal design. A secondary data analysis was conducted on a subset of data originally collected from acute stroke units at the John Radcliffe Hospital between February 2015 and February 2020 as part of two studies of the Oxford Cognitive Screen (OCS; Demeyere et al., Citation2015), the OCS-Tablet and OCS-Recovery studies (NHS Research Ethics Committee references: 14/LO/0648 and 18/SC/0550). Participants were included in those larger studies if they were adults recruited within 3-weeks of confirmed ischemic or haemorrhagic stroke, able to give informed consent, and able to concentrate for the duration of OCS administration, as judged by the multidisciplinary team.
Participants were included in the current analysis if they had completed at least 9 of out 12 subtests of the OCS within 3-weeks of confirmed ischemic or haemorrhagic stroke (T1) and again approximately 6-months later (T2). Participants were required to have complete data for (i) the Cognitive Failures Questionnaire at T2 (CFQ; Broadbent et al., Citation1982), (ii) the Hospital Anxiety and Depression scale at T2 (HADS; Snaith & Zigmond, Citation1986), (iii) demographic information including age, sex, and years of education, (iv) the National Institutes of Health stroke scale at admission (NIHSS; Lyden et al., Citation1994), (v) stroke hemisphere, (vi) the Barthel Index at T2 (BI; Mahoney & Barthel, Citation1965). The T1 measures were administered within the first few days and weeks following stroke whilst the participants were still inpatients in hospital. At this acute time-point, to reduce burden on participants, only a brief assessment was completed. Data for 253 participants with complete CFQ, HADS, and Barthel Index scores were screened in line with the remaining inclusion criteria. One-hundred and ten participants were excluded during the screening process: 13 participants did not have complete follow-up OCS scores, 2 participants completed less than 9 OCS subtests at T1, 2 participants completed less than 9 OCS subtests at T2, 1 participant did not have age recorded, 48 participants did not have years of education recorded, 21 participants did not have stroke hemisphere recorded, and 23 participants did not have NIHSS scores recorded. Consequently, 143 participants were included in the current analysis (see ).
Measures
Cognitive measurement
Objective cognitive impairment was measured using the OCS, a short cognitive screen designed for use with stroke patients (Demeyere et al., Citation2015). Domain-specific subtest scores relating to apraxia, unilateral neglect, memory, language, executive function, and number abilities are generated. Impairments on the OCS for each subtest are indicated by a score lower, or in some cases a score higher, than the normative “cut-off” (5th or 95th centile respectively; see https://www.ocs-test.org/ocs-background/how-to/ for a video demonstration on how to score the OCS). It has good test-retest reliability, content validity, specificity, and high sensitivity (Demeyere et al., Citation2015).
Self-awareness measurement
Subjective cognitive impairment was assessed with the Cognitive Failures Questionnaire (CFQ), a 25-item self-report questionnaire whereby subjects rate the frequency of everyday cognitive errors on a 5-point Likert scale ranging from 0 (never) to 4 (very often; Broadbent et al., Citation1982). The total score is computed by summing all scores, with a maximum score of 100. This measure is frequently used in stroke samples and has good psychometric properties (Barker-Collo et al., Citation2010; Bridger et al., Citation2013; Westerberg et al., Citation2007). This measure was not administered at T1 as participants were inpatients in hospital, which may make it difficult for them to estimate the functional impact of their cognition, as they would not have been able to experience this in their usual environments.
Emotional distress measurement
Emotional distress was assessed with the HADS, a 14-item questionnaire examining symptoms of anxiety and depression on a 4-point scale, whereby higher scores indicate greater symptomology (Snaith & Zigmond, Citation1986). Caseness is defined by a score of >8 on both subscales (Bjelland et al., Citation2002). It has been used with stroke survivors and reports good internal consistency (Cronbach α = 0.8), with high specificity and sensitivity in this population (Aben et al., Citation2002). Total HADS score was used as a measure of emotional distress in the main analyses. Given natural reactions to a significant life event and early post-stroke emotionalism, a mood measure was not administered at T1 as it was felt that this would not be indicative of clinically significant mood symptoms.
Participant and stroke characteristic measurements
Participant age, sex, and years of education were recorded. The NIHSS, a proxy measure of stroke severity (Lyden et al., Citation1994), and stroke hemisphere were extracted from participant medical records. Participants also completed the Barthel Index, a measure of disability relating to activities of daily living (ADL). It has a maximum score of 20 and higher scores indicate greater ability to carry out ADL’s independently (Mahoney & Barthel, Citation1965).
Procedure
Participants were initially assessed with the OCS during admission to an acute stroke unit, up to 3 weeks after their stroke (T1, M = 3.5 days, SD = 2.93). A 6-month follow-up assessment was planned (T2), although due to delays in contacting and reaching participants following discharge, the actual time interval differed across participants (5–9 months, M = 6.7 months, SD = 0.78). Questionnaires were administered at T2 in addition to the OCS. Stroke characteristic data were extracted from medical records (NIHSS; hemisphere). Assessments took place at the hospital (T1) and at the patient’s home (T2). illustrates which assessments were administered at each time-point.
Table 1. Summary of assessments administered at T1 and T2.
Statistical analyses
Statistical analyses were conducted using the SPSS 27.0 statistical package. Power analyses in GPower 3.1 determined the power for a sample size of 143 participants in each analysis (Faul et al., Citation2009). All hypotheses, methods and the analysis plan were pre-registered on OSF prior to data analysis (https://osf.io/87kjv).
Computing variables
Objective cognitive impairment
Raw scores on the OCS at T1 and T2 were used to compute objective cognitive impairment severity scores at T1 and T2 respectively. These scores were quantified by summing the number of subtests (n = 12) on which a participant scored outside the norm-based cut-off values on the OCS (range = 0–12; Demeyere et al., Citation2015), following the approach of others (e.g., Van Rijsbergen et al., Citation2020). As a small number of subtests were missing for some participants, the percentage of tests failed was calculated from the number of attempted subtests at each time-point (range 0–12 subtests at T1 and 0–12 subtests at T2). The percentage of tests “failed” comprised the objective cognitive impairment severity scores (range 0–100%) at T1 and T2, whereby higher scores indicate greater cognitive impairment ( ).
In order to explore whether previously reported findings identifying a negative association between severity of post-stroke cognitive impairment and awareness of cognitive impairment apply to the present data, participants were grouped into a “moderate-severe cognitive impairment” group (defined as those with a T2 objective cognitive impairment score ≥1 SD from the sample objective cognitive impairment mean; cut-off ≥ 39.73% subtests failed) and a “mild-no cognitive impairment" group (defined as those with a T2 objective cognitive impairment score <1 SD from the sample objective cognitive impairment mean).
Cognitive change
Existing studies report a range of criteria for indicating cognitive change in stroke samples, thus there is no widely agreed “gold standard” for calculating this (Brainin et al., Citation2015). Here, cognitive change was quantified as the difference between the patient’s objective cognitive impairment scores over time (, range −100% to 100% whereby positive scores indicate cognitive decline).
Awareness
Awareness scores were generated to represent participants’ awareness of their cognitive impairment, following a similar procedure used by Mitrushina and Tomaszewski (Citation2020). First, psychometric Z-scores were computed from objective cognitive impairment scores at T2. Secondly, psychometric subjective cognitive impairment Z-scores were computed for each participant using raw total scores on the CFQ. Then, OCI Z-scores were subtracted from CFQ Z-scores (). Awareness scores were computed by transforming the answer into a T-score. This created a distribution of awareness scores centred around a mean of 50 (accurately reporting level of cognitive impairment), with lower scores representing lower levels of awareness (under-estimating cognitive impairment) and higher scores representing hyper-awareness (over-estimating cognitive impairment). Measuring awareness of cognitive impairment in this way provided a more objective proxy of awareness than comparing participant’s perceived awareness to the perceptions of a family member or carer (Hoofien et al., Citation2004). Moreover, this measure perhaps reflects the approach to assessing self-awareness of cognitive impairment in clinical practice, whereby patients are asked about changes in their functional abilities day-to-day, as opposed to being directly asked about changes in specific, and likely unfamiliar, cognitive domains. For example, a patient may not identify as having “executive impairments” but may notice that they often “say something and realize afterwards that it might be taken as insulting” when asked (CFQ, Q8; Broadbent et al., Citation1982).
Minimizing bias
To determine whether any selection bias occurred between participants who were re-examined at follow-up and those who were not, and between those who were excluded due to missing data and those who were not, a series of binary logistic regression analyses were carried out whilst correcting for multiple comparisons using the Benjamini and Hochberg (Citation1995) false discovery rate method. Demographic variables, stroke characteristics, and objective cognitive impairment at T1 were predictor variables (see Supplementary 1).
Main analyses
To identify possible covariates, a series of univariate correlations for continuous predictors and t-tests/ANOVAs for categorical predictors were carried out to explore the association between demographic/stroke characteristic variables and the dependent variable (DV) in each analysis (see Supplementary 2). These identified that Barthel Index was significantly associated with awareness (rs(141) = .14, p = .090) and HADS (rs(141) = −.37, p < .001) so this was included as a covariate in all main analyses.
Prior to the main analyses, data were examined to check whether necessary statistical assumptions had been violated. With regards to analysis one, there was an absence of extreme outliers, the dependent variable was normally distributed, and Levene’s test indicated that there was homogeneity of variance. However, the assumption of homogeneity of regression slopes was not met, as the covariate (BI) had a significantly different relationship with the "moderate-severe cognitive impairment" group vs the "mild-no cognitive impairment" group F(2, 140) = 40.27, p < .001 Therefore, a robust ANCOVA was carried out (Mair & Wilcox, Citation2020). This produced the same findings as an ANCOVA, therefore unadjusted (ANCOVA) data are reported.
Assumptions were explored for the regression models in analyses two and three. Assumptions for the moderator analysis were examined by firstly centring the means for the predictor and moderator variable and computing an interaction variable by multiplying scores of the centred variables 'cognitive change' and 'objective cognitive impairment T1'. A forced-entry regression with the centred predictor, centred moderator, the interaction variable, and BI as predictors was carried out in order to inspect sources of bias in the model. None of the regression models presented suggested high residual deviations from normality, Cook’s distances >1, or Durbin-Watson statistics <1 or >3. The variance of residuals in both models was constant. Checks for multicollinearity of predictors found no problems, with VIF scores well below 10 and tolerance scores >0.2.
To investigate whether there was a difference in awareness between the “moderate-severe cognitive impairment” group and the “mild-no cognitive impairment" group, an ANCOVA was carried out whilst controlling for BI. To explore the relationship between awareness and emotional distress, a hierarchical multiple linear regression analysis was performed with emotional distress as the DV. BI was entered into the first step as a covariate (Model 1) and awareness was entered into step two (Model 2). To investigate whether objective cognitive impairment at T1 has a moderating effect on the relationship between cognitive change and emotional distress, the PROCESS plug-in (Hayes, Citation2017) was used to conduct a moderation analysis. Emotional distress was entered as the DV, cognitive change was entered as the predictor, objective cognitive impairment at T1 was entered as the moderator, and awareness and BI were entered as covariates.
Follow-up analyses
Exploratory logistic regression follow-up analyses were conducted to ascertain the effects of BI, awareness, and objective cognitive impairment at T2 on the likelihood that participants would report clinically significant symptoms of anxiety and depression. The logistic regression models were built systematically to choose the most parsimonious models as the final ones. The final models indicated that BI and awareness significantly contributed to the models, but adding an interaction term had virtually no effect on the fit of the model, so the final model did not include this. The models demonstrated that the assumption of linearity of the logit was met, the Durbin-Watson statistic was close to two, and there was an absence of multicollinearity.
Results
One-hundred and forty-three participants met criteria for inclusion. Descriptive statistics for clinical and demographic variables for participants are shown in . Binary logistic regression analyses indicated that completion of follow-up (yes/no) and exclusion from the present study (yes/no) were not predicted by demographic variables, stroke characteristics, or objective cognitive impairment at T1 at p < .1 (see Supplementary 1). These results suggest that dropout and outcome data are missing at random, resulting in a low risk of selective attrition.
Table 2. Clinical and demographic characteristics of included and excluded participants.
Main analyses 1. What is the relationship between cognitive impairment at 6-months post stroke and awareness of cognitive impairment at 6-months post stroke?
In this sample, 23 participants were considered to be in the “moderate-severe cognitive impairment” group at T2 and 120 participants were considered to be in the “mild-no cognitive impairment” group. The mean OCI T2 score for the “moderate-severe cognitive impairment” group was 54.02% (SD = 13.41) and the mean OCI T2 score for the “mild-no cognitive impairment” group was 17.65% (SD = 8.17). A one-way ANCOVA was carried out to explore whether there were any significant differences in awareness score between the “moderate-severe cognitive impairment” group and the “mild-no cognitive impairment" group at T2, whilst controlling for BI (see for adjusted descriptive statistics). The covariate BI was not significantly related to awareness (F(1, 140) = 0.58, p = .449). There was a significant effect of severity of objective cognitive impairment at T2 on awareness after controlling for the effect of BI, (F(1, 140) = 104.81, p < .001, partial η2 = .43) suggesting that awareness was significantly lower in the “moderate-severe cognitive impairment” group (see ).
Figure 2. Scatterplot illustrating the relationship between awareness and OCI at T2.
Note: The vertical line through the x-axis denotes the cut-off OCI score (39.73) that defines the two groups, whereby OCI scores ≥ 39.73 indicates “moderate-severe cognitive impairment”.

Table 3. Unadjusted and covariate adjusted descriptive statistics for awareness.
2. What is the relationship between awareness of cognitive impairment at 6-months post stroke and emotional distress at 6-months post stroke?
In the sample, 28% and 22% of participants met the clinical threshold for anxiety and depression on the HADS respectively. A hierarchical multiple regression analysis was carried out whereby potential predictors of HADS Total were entered in blocks. BI was entered first as a covariate, then awareness was added in the second block. The final model indicated that higher BI scores were associated with lower emotional distress (b = −.63, CI [−.86, −.40], t = −5.33, p < .001), and higher awareness scores were associated with greater emotional distress (b = .18, CI [.11, .25], t = 5.03, p < .001), together explaining 25.2% variance in the model (R2Adjusted = .252, F(2, 140) = 24.98, p < .001), with a large effect size of f2 = .34.
3. Does severity of acute post-stroke cognitive impairment moderate the relationship between cognitive change and emotional distress in the months following stroke?
The model indicates that cognitive change was a positive predictor of HADS, whereby a reduction in impairment over time was associated with a lower HADS score (b = .19, 95% CI [.09, .30], t = 3.57, p < .01), and this was moderated by objective cognitive impairment at T1, suggesting that this effect was strongest for those with greater cognitive impairment at T1 (b = .0020, 95% CI [.0002, .0038], t = 2.21, p < .05). However, this interaction between acute severity and cognitive change only explained 2.2% of variance in the model (R2 Change = .022, F(1, 137) = 4.88, p < .05). Together, predictors explained 36.9% variance in the model (R2Adjusted = .369, F(5, 137) = 17.57, p < .001; see ), with a large effect size of f 2 = .58.
Table 4. Hierarchical multiple regression analyses of objective cognitive impairment at T1 as a moderator of the relationship between cognitive change and emotional distress (HADS total score) controlling for the effect of BI and awareness.
The Johnson–Neyman method (Citation1936) was used to explore the directionality of the moderation effect. shows how the relationship between cognitive change and HADS changes at different values of objective cognitive impairment at T1. This indicates that those with more severe cognitive impairment at T1 may be more sensitive to the effects of cognitive change on HADS.
Figure 3. The conditional effect of cognitive change on HADS score as a function of objective cognitive impairment score at T1.
Note: Conditional effect of cognitive change represents the gradient between cognitive change and HADS score for a given OCI score at T1. Those with a higher OCI score at T1 are more sensitive to the effects of cognitive change on HADS. Upper and lower 95% confidence intervals are indicated by the dashed lines.

Exploratory analyses
Further exploratory analyses were conducted to examine whether BI, awareness, and objective cognitive impairment at T2 were differentially related to anxiety and depression symptomology. Two forced-entry logistic regression analyses were performed with BI and awareness entered as predictors in the first, and BI, awareness, and objective cognitive impairment at T2 entered as predictors in the second. Objective cognitive impairment at T2 did not significantly improve the anxiety model so was excluded.
Increasing awareness was associated with an increased likelihood of reporting clinically significant anxiety (b = .046, 95% CI [.012, .087], p < .01, OR = 1.05, 95% CI [1.01, 1.08]) and depression symptomology (b = .097, 95% CI [.054, .154], p < .001, OR = 1.10, 95% CI [1.05, 1.20]). Higher BI scores were associated with a reduction in the likelihood of clinically significant anxiety (b = −.147, 95% CI [−.252, −.051], p < .01, OR = 0.863, 95% CI [0.79, 0.95]) and depression symptomology (b = −.150, 95% CI [−.261, −.063], p < .01, OR = 0.861, 95% CI [0.78, 0.96]). Higher objective cognitive impairment T2 scores were associated with an increase in the likelihood of clinically significant depression symptomology (b = .07, 95% CI [.02, .12], p < .01, OR = 1.07, 95% CI [1.02, 1.12]).
The anxiety model explained 18% (Nagelkerke R2) of the variance in symptoms of anxiety. The model correctly classified 72.7% of cases, however, it was more accurate at classifying cases not reporting clinically anxious symptoms (93.2% correct) than cases reporting clinically anxious symptoms (20%). The depression model explained 32% (Nagelkerke R2) of the variance in symptoms of depression. The model correctly classified 83% of cases, however, it was more accurate at classifying cases not reporting clinical symptoms of depression (97.3% correct) than cases reporting clinical symptoms of depression (34%).
Discussion
To the authors’ knowledge, this is the first study to test aspects of the Y-shaped model in the early months after a stroke. This study primarily aimed to test the hypothesis that the relationship between cognitive change and mood outcomes in the months following stroke would be moderated by the severity of acute post-stroke cognitive impairment, to further understanding of change processes influencing psychological outcomes post-stroke in line with the Y-shaped model. Preliminary research questions aimed to establish whether previously well-documented relationships between the severity of cognitive impairment and awareness of cognitive impairment, and between awareness of cognitive impairment and emotional distress, were also present in this sample.
Results of this study of stroke participants with well characterized cognitive impairment suggest a strong relationship between awareness of cognitive deficits and cognitive functioning, with participants reporting accurate awareness when only mildly cognitively impaired and participants with more severe or global cognitive impairment under-estimating their cognitive deficits. This supports evidence that poor awareness of cognitive deficits is associated with organic changes sustained by brain injury through stroke (Boosman et al., Citation2014; Ownsworth et al., Citation2007). In line with the Y-shaped model, those with more severe or global cognitive impairment may consequently lack the capacity to process, retain, understand, or accept the cognitive consequences of their stroke (Gracey et al., Citation2009). Moreover, findings suggest that this strong relationship between cognitive impairment and awareness exists several months after the stroke itself. This indicates that this awareness deficit may persist despite possible exposure to interpersonal feedback from others and personally experiencing the impact of cognitive impairments on functional tasks, although this should be explored in future studies. This finding suggests that stroke patients with more severe or global cognitive impairment may benefit from tailored psychoeducation and targeted feedback interventions provided over time, which both explain and normalize changes to cognition in a context of a safe therapeutic relationship, whilst also giving space to reflect on changes noticed and the meaning of these changes to the patient.
With regards to the second hypothesis, findings suggest that greater awareness of cognitive impairment is strongly associated with increased distress, in line with previous work in various ABI samples (Boosman et al., Citation2014; Geytenbeek et al., Citation2017; Mitrushina & Tomaszewski, Citation2020; Morton & Barker, Citation2010). Moreover, findings suggest that BI is a negative predictor of emotional distress in accordance with previous research, indicating that the more independent an individual is with their activities of daily living, the less likely they are to report clinically significant symptoms of anxiety or low mood (Huang et al., Citation2014; Park et al., Citation2012). These findings may be explained by the relationship between “threat-to-self”, “self-discrepancy”, and emotional distress prescribed by the Y-shaped model. Specifically, it is possible that an individual with greater awareness of their cognitive deficits and higher levels of disability in carrying out ADLs following stroke may experience a threatened pre-injury identity, accompanied by an increase in emotional distress (Gracey et al., Citation2009) although pre-injury identity was not specifically measured in this study. Alternatively, it is possible that increased emotional distress heightens one’s attentional bias towards “cognitive failures” following stroke, thus increasing awareness of one’s experience of self-discrepancy. This should be investigated in a future longitudinal study which measures the dynamic influence of variables at both 3-week and 6-month time-points.
The final analysis explored the effect of cognitive change on emotional distress, and whether this was moderated by the severity of objective cognitive impairment at T1. Our findings suggest that cognitive improvement in the months following stroke is significantly associated with lower reported emotional distress across all levels of acute objective cognitive impairment. It is possible that participants may report low levels of anxiety and depression symptomology as they are experiencing fewer cognitive difficulties. When considering the mechanisms underlying this finding through the lens of the Y-shaped model, one could hypothesize that improvement in cognitive performance may reduce the discrepancy between pre- and post-injury cognitive ability enabling the individual to progress through a process of post-stroke adjustment, associated with decreased distress (Gracey et al., Citation2009).
The moderation model indicated that the effect of cognitive change was moderated by acute cognitive impairment (see ). To the best of the authors’ knowledge, this is the first observation that emotional outcomes in the months following stroke depend, partially, on the interaction between cognitive change and the severity of acute post-stroke cognitive impairment. Notably, the direction of the moderating effect of acute cognitive impairment on the relationship between cognitive improvement and emotional distress is opposite to the one we expected. Specifically, those with more severe acute cognitive impairments reported lower emotional distress as a function of cognitive improvement. This finding appears incongruent with the awareness hypothesis (Boosman et al., Citation2014; Ownsworth et al., Citation2007). One may expect that an individual with a greater acute cognitive impairment would have lower levels of awareness, and thus low emotional distress. If an under-aware individual’s cognitive function were subsequently to improve, one may expect that they may gain the capacity to become aware of any discrepancy in their pre- and post-stroke self, which may then lead to increased emotional distress. This incongruence would suggest that awareness of cognitive impairment is not the dominant factor in determining the relationship between acute cognition and anxiety and depression symptomatology in this particular stroke sample at 6-months, which is supported by the R2 = .39 of the model. The sample in this study primarily comprised participants with mild stroke. Mild stroke has been associated with better long-term functional outcomes and it is possible that those experiencing fewer or more focal deficits may have greater success with identifying and implementing cognitive and behavioural compensatory strategies, which may have a notable impact on their everyday functioning and mood (Kwakkel & Kollen, Citation2013). This raises the question of whether the results of this study would follow the expected direction in a sample comprising moderate-severe strokes, whereby participants may be more likely to experience more limited cognitive and functional recovery as their awareness improves and this should be investigated in future research. Moreover, as the mechanism and direction of the association between change in cognition and mood cannot be established in this study, due to the absence of a measure of threat-to-self and adjustment, this should also form the basis for future research.
Findings from the exploratory analyses suggest that subjective awareness of cognitive impairment and disability affecting ADL are significant predictors of clinically significant anxiety and depression symptomology. These findings differ from those of Geytenbeek et al. (Citation2017), who reported that depression was not associated with awareness in a TBI sample. This may be a consequence of methodological differences including sample characteristics, or different measures used. Current findings also suggest that cognitive impairment is a significant predictor of depression symptomology. This is in line with previous research, which suggests that deficits in attention, memory, processing speed, and executive functioning, are commonly experienced by stroke survivors and are also associated with symptoms of depression (Broomfield et al., Citation2011; Nakling et al., Citation2017; Nys et al., Citation2006). This finding may thus reflect stroke-induced damage to the frontal subcortical circuits, which are responsible for both executive skills and emotional regulation (Alexopoulos et al., Citation1997; Flaster et al., Citation2013). Moreover, changes to executive skills may also interact with psychological mechanisms to further maintain low mood, for example, through exacerbating difficulties with flexible thinking and problem solving (Broomfield et al., Citation2011). However, due to the exploratory nature of these findings, future studies should use a hypothesis-driven approach to explore these further.
Clinical implications
Findings emphasize the importance of adopting a biopsychosocial approach to neurorehabilitation by monitoring stroke patients’ cognitive deficits and their capacity to be self-aware when assessing and formulating long-term post-stroke distress and adjustment (Mitrushina & Tomaszewski, Citation2020; Van Rijsbergen et al., Citation2019). Findings suggest that patients with more severe or global cognitive impairment 6-months post-stroke are less likely to understand, retain, or accept post-stroke cognitive change. Although low awareness appears to be protective against emotional distress at 6-months, whether that be due to a low level of self-discrepancy, the absence of negative attentional bias, or denial, present findings suggest that cognitive improvement over the first 6-months post-stroke is associated with lower reported emotional distress. Moreover, this effect is largest for those most cognitively impaired in the days following stroke. This has possible implications for the provision of psychological intervention in this sub-group. Namely, targeting this sub-group of patients with psychoeducation and feedback regarding cognitive change and cognitive rehabilitation interventions, alongside emotional support in the early stages post-stroke may have a positive impact on their long-term emotional adjustment. This approach would support these patients to attend to evidence of changes to their cognition, appraise these in a way that is less threatening to their sense of self, and learn compensatory strategies that enable them to engage in meaningful activities and adjust to post-stroke changes. In contrast, cognitive improvement over the first 6-months appears to have a much smaller impact on the emotional wellbeing of those with less cognitive impairment in the weeks following stroke. Psychological interventions such as cognitive behavioural therapy may be better suited to this group, to support them with the process of personal reconciliation.
Strengths and limitations
A methodological advantage of this study is that participants’ self-evaluations of their cognitive abilities were compared to their neuropsychological performance, as opposed to scores based on evaluations provided by family members or clinicians, thus yielding a more objective measure of awareness (Hoofien et al., Citation2004). A further strength of this study is the large sample size and inclusion of participants with communication difficulties, which makes findings more generalizable to clinical populations.
Some limitations of this study should be noted. Firstly, the measure of awareness used in this study perhaps reflects intellectual awareness, in that participants were asked to rate the implications of cognitive changes post-injury. However, “online awareness”, defined as the ability to self-monitor and modify behaviour during performance, was not measured (Toglia & Kirk, Citation2000). Additional research is required to determine a gold-standard measure for detecting multiple aspects of impaired self-awareness, according to normalized cut-off scores. Secondly, emotional distress was considered as an outcome in this study, however, it could also be a potential risk factor for awareness and cognitive impairment, and future studies should measure these variables at multiple time-points over the course of 1-year post-stroke to establish the mechanism and order of change over time. Thirdly, the study sample consisted of primarily participants with a mild stroke (median NIHSS score 5), good cognitive functioning (120 participants were in the “mild-no cognitive impairment” group), and high levels of functional independence in personal care and mobility (median BI score at 6-months was 18/20), which limits the generalizability of the findings to the entire stroke population. Fourthly, data was not collected regarding premorbid psychological history, despite evidence that this variable is associated with self-awareness, post-stroke depression, and psychosocial stroke outcomes (Hurst et al., Citation2020; Taylor-Rowan et al., Citation2019).
Conclusion
This study suggests that emotional outcomes in the months following stroke depend partially on the interaction between cognitive change and the severity of stroke-induced cognitive impairment. Specifically, cognitive improvement over time was associated with lower HADS scores at 6-months post-stroke and this effect was largest for those most cognitively impaired in the days following stroke. These results have potential implications for improving long-term emotional adjustment in this sub-group of patients through psychoeducation and cognitive rehabilitation interventions, alongside emotional support.
Supplementary_Information_2.docx
Download MS Word (17.8 KB)Supplementary_Information_1.docx
Download MS Word (21.8 KB)Acknowledgements
The authors would like to express sincere gratitude to Dr Georgie Boothroyd, Dr Aonghus Ryan, and Dr Nigel King in helpful early discussion of this research.
Disclosure statement
No potential conflict of interest was reported by the author(s).
Data availability statement
A minimal dataset that supports the findings of this study is openly available in OSF at https://osf.io/87kjv/10.17605/OSF.IO/87KJV
Additional information
Funding
References
- Aben, I., Verhey, F., Lousberg, R., Lodder, J., & Honig, A. (2002). Validity of the beck depression inventory, Hospital Anxiety and Depression scale, Scl-90, and hamilton depression rating scale as screening instruments for depression in stroke patients. Psychosomatics, 43(5), 386–393. https://doi.org/10.1176/appi.psy.43.5.386
- Adamson, J., Beswick, A., & Ebrahim, S. (2004). Is stroke the most common cause of disability? Journal of Stroke and Cerebrovascular Diseases, 13(4), 171–177. https://doi.org/10.1016/j.jstrokecerebrovasdis.2004.06.003
- Alexopoulos, G. S., Meyers, B. S., Young, R. C., Kakuma, T., Silbersweig, D., & Charlson, M. (1997). Clinically defined vascular depression. American Journal of Psychiatry, 154(4), 562–565. https://doi.org/10.1176/ajp.154.4.562
- Ayerbe, L., Ayis, S., Wolfe, C. D., & Rudd, A. G. (2013). Natural history, predictors and outcomes of depression after stroke: Systematic review and meta-analysis. British Journal of Psychiatry, 202(1), 14–21. https://doi.org/10.1192/bjp.bp.111.107664
- Barker-Collo, S. L., Feigin, V. L., Lawes, C. M., Parag, V., & Senior, H. (2010). Attention deficits after incident stroke in the acute period: Frequency across types of attention and relationships to patient characteristics and functional outcomes. Topics in Stroke Rehabilitation, 17(6), 463–476. https://doi.org/10.1310/tsr1706-463
- Benjamini, Y., & Hochberg, Y. (1995). Controlling the false discovery rate: A practical and powerful approach to multiple testing. Journal of the Royal Statistical Society: Series B (Methodological), 57(1), 289–300. https://doi.org/10.1111/j.2517-6161.1995.tb02031.x
- Bjelland, I., Dahl, A. A., Haug, T. T., & Neckelmann, D. (2002). The validity of the Hospital Anxiety and Depression scale: An updated literature review. Journal of Psychosomatic Research, 52(2), 69–77. https://doi.org/10.1016/S0022-3999(01)00296-3
- Bogod, N. M., Mateer, C. A., & Macdonald, S. W. (2003). Self-awareness after traumatic brain injury: A comparison of measures and their relationship to executive functions. Journal of the International Neuropsychological Society: JINS, 9(3), 450. https://doi.org/10.1017/S1355617703930104
- Boosman, H., Van Heugten, C., Winkens, I., Heijnen, V., & Visser-Meily, J. (2014). Awareness of memory functioning in patients with stroke Who have a good functional outcome. Brain Injury, 28(7), 959–964. https://doi.org/10.3109/02699052.2014.888763
- Brainin, M., Tuomilehto, J., Heiss, W. D., Bornstein, N. M., Bath, P. M., Teuschl, Y., Richard, E., Guekht, A., Quinn, T., & Group, P. S. C. S. (2015). Post-stroke cognitive decline: An update and perspectives for clinical research. European Journal of Neurology, 22(2), 229–e216. https://doi.org/10.1111/ene.12626
- Bridger, R. S., Johnsen, SÅK, & Brasher, K. (2013). Psychometric properties of the cognitive failures questionnaire. Ergonomics, 56(10), 1515–1524. https://doi.org/10.1080/00140139.2013.821172
- Broadbent, D. E., Cooper, P. F., FitzGerald, P., & Parkes, K. R. (1982). The cognitive failures questionnaire (CFQ) and its correlates. British Journal of Clinical Psychology, 21(1), 1–16. https://doi.org/10.1111/j.2044-8260.1982.tb01421.x
- Broomfield, N. M., Laidlaw, K., Hickabottom, E., Murray, M. F., Pendrey, R., Whittick, J. E., & Gillespie, D. C. (2011). Post-stroke depression: The case for augmented, individually tailored cognitive behavioural therapy. Clinical Psychology & Psychotherapy, 18(3), 202–217. https://doi.org/10.1002/cpp.711
- Cai, W., Mueller, C., Li, Y.-J., Shen, W.-D., & Stewart, R. (2019). Post stroke depression and risk of stroke recurrence and mortality: A systematic review and meta-analysis. Ageing Research Reviews, 50, 102–109. https://doi.org/10.1016/j.arr.2019.01.013
- Carroll, E., & Coetzer, R. (2011). Identity, grief and self-awareness after traumatic brain injury. Neuropsychological Rehabilitation, 21(3), 289–305. https://doi.org/10.1080/09602011.2011.555972
- Chamelian, L., & Feinstein, A. (2006). The effect of major depression on subjective and objective cognitive deficits in mild to moderate traumatic brain injury. The Journal of Neuropsychiatry and Clinical Neurosciences, 18(1), 33–38. https://doi.org/10.1176/jnp.18.1.33
- Demeyere, N., Riddoch, M. J., Slavkova, E. D., Bickerton, W.-L., & Humphreys, G. W. (2015). The Oxford Cognitive Screen (Ocs): Validation of a stroke-specific short cognitive screening tool. Psychological Assessment, 27(3), 883–894. https://doi.org/10.1037/pas0000082
- Donnellan, C., Hickey, A., Hevey, D., & O'Neill, D. (2010). Effect of mood symptoms on recovery one year after stroke. International Journal of Geriatric Psychiatry, 25(12), 1288–1295. https://doi.org/10.1002/gps.2482
- Ezema, C. I., Akusoba, P. C., Nweke, M. C., Uchewoke, C. U., Agono, J., & Usoro, G. (2019). Influence of post-stroke depression on functional independence in activities of daily living. Ethiopian Journal of Health Sciences, 29(1), 841–846. https://doi.org/10.4314/ejhs.v29i1.5
- Faul, F., Erdfelder, E., Buchner, A., & Lang, A.-G. (2009). Statistical power analyses using G* power 3.1: Tests for correlation and regression analyses. Behavior Research Methods, 41(4), 1149–1160. https://doi.org/10.3758/BRM.41.4.1149
- Flaster, M., Sharma, A., & Rao, M. (2013). Poststroke depression: A review emphasizing the role of prophylactic treatment and synergy with treatment for motor recovery. Topics in Stroke Rehabilitation, 20(2), 139–150. https://doi.org/10.1310/tsr2002-139
- Fleming, J., & Ownsworth, T. (2006). A review of awareness interventions in brain injury rehabilitation. Neuropsychological Rehabilitation, 16(4), 474–500. https://doi.org/10.1080/09602010500505518
- Geytenbeek, M., Fleming, J., Doig, E., & Ownsworth, T. (2017). The occurrence of early impaired self-awareness after traumatic brain injury and its relationship with emotional distress and psychosocial functioning. Brain Injury, 31(13-14), 1791–1798. https://doi.org/10.1080/02699052.2017.1346297
- Gracey, F., Evans, J. J., & Malley, D. (2009). Capturing process and outcome in complex rehabilitation interventions: A “Y-shaped” model. Neuropsychological Rehabilitation, 19(6), 867–890. https://doi.org/10.1080/09602010903027763
- Hart, T., Seignourel, P. J., & Sherer, M. (2009). A longitudinal study of awareness of deficit after moderate to severe traumatic brain injury. Neuropsychological Rehabilitation, 19(2), 161–176. https://doi.org/10.1080/09602010802188393
- Hart, T., Sherer, M., Whyte, J., Polansky, M., & Novack, T. A. (2004). Awareness of behavioral, cognitive, and physical deficits in acute traumatic brain injury. Archives of Physical Medicine and Rehabilitation, 85(9), 1450–1456. https://doi.org/10.1016/j.apmr.2004.01.030
- Hayes, A. F. (2017). Introduction to mediation, moderation, and conditional process analysis: A regression-based approach. Guilford Publications.
- Hoofien, D., Gilboa, A., Vakil, E., & Barak, O. (2004). Unawareness of cognitive deficits and daily functioning among persons with traumatic brain injuries. Journal of Clinical and Experimental Neuropsychology, 26(2), 278–290. https://doi.org/10.1076/jcen.26.2.278.28084
- Huang, H. C., Huang, L. K., Hu, C. J., Chang, C. H., Lee, H. C., Chi, N. F., Shyu, M. L., & Chang, H. J. (2014). The mediating effect of psychological distress on functional dependence in stroke patients. Journal of Clinical Nursing, 23(23-24), 3533–3543. https://doi.org/10.1111/jocn.12606
- Hurst, F. G., Ownsworth, T., Beadle, E., Shum, D. H., & Fleming, J. (2020). Domain-specific deficits in self-awareness and relationship to psychosocial outcomes after severe traumatic brain injury. Disability and Rehabilitation, 42(5), 651–659. https://doi.org/10.1080/09638288.2018.1504993
- Johnson, P. O., & Neyman, J. (1936). Tests of certain linear hypotheses and their application to some educational problems. Statistical Research Memoirs. https://psycnet.apa.org/record/1936-05538-001
- Kim, E.-S., Kim, J.-W., Kang, H.-J., Bae, K.-Y., Kim, S.-W., Kim, J.-T., Park, M.-S., Cho, K.-H., & Kim, J.-M. (2018). Longitudinal impact of depression on quality of life in stroke patients. Psychiatry Investigation, 15(2), 141–146. https://doi.org/10.30773/pi.2017.10.11
- King, D., Wittenberg, R., Patel, A., Quayyum, Z., Berdunov, V., & Knapp, M. (2020). The future incidence, prevalence and costs of stroke in the UK. Age and Ageing, 49(2), 277–282. https://doi.org/10.1093/ageing/afz163
- Knapp, P., Dunn-Roberts, A., Sahib, N., Cook, L., Astin, F., Kontou, E., & Thomas, S. A. (2020). Frequency of anxiety after stroke: An updated systematic review and meta-analysis of observational studies. International Journal of Stroke, 15(3), 244–255. https://doi.org/10.1177/1747493019896958
- Kwakkel, G., & Kollen, B. J. (2013). Predicting activities after stroke: What is clinically relevant? International Journal of Stroke, 8(1), 25–32. https://doi.org/10.1111/j.1747-4949.2012.00967.x
- Lyden, P., Brott, T., Tilley, B., Welch, K., Mascha, E., Levine, S., Haley, E., Grotta, J., & Marler, J. (1994). Improved reliability of the NIH stroke scale using video training. NINDS TPA stroke Study Group. Stroke, 25(11), 2220–2226. https://doi.org/10.1161/01.STR.25.11.2220
- Mahoney, F. I., & Barthel, D. W. (1965). Functional evaluation: The Barthel Index: A simple index of independence useful in scoring improvement in the rehabilitation of the chronically ill. Maryland State Medical Journal, 14, 61–65. https://psycnet.apa.org/record/2012-30334-001
- Mair, P., & Wilcox, R. (2020). Robust statistical methods in R using the Wrs2 package. Behavior Research Methods, 52(2), 464–488. https://doi.org/10.3758/s13428-019-01246-w
- Mitchell, A. J., Sheth, B., Gill, J., Yadegarfar, M., Stubbs, B., Yadegarfar, M., & Meader, N. (2017). Prevalence and predictors of post-stroke mood disorders: A meta-analysis and meta-regression of depression, anxiety and adjustment disorder. General Hospital Psychiatry, 47, 48–60. https://doi.org/10.1016/j.genhosppsych.2017.04.001
- Mitrushina, M., & Tomaszewski, R. (2020). The effect of subjective perception of cognition on emotional functioning in adults with long-term neurological and neuropsychiatric disorders. Disability and Rehabilitation, 42(22), 3135–3141. https://doi.org/10.1080/09638288.2019.1585490
- Morton, N., & Barker, L. (2010). The contribution of injury severity, executive and implicit functions to awareness of deficits after traumatic brain injury (TBI). Journal of the International Neuropsychological Society, 16((06|6)), 1089–1098. https://doi.org/10.1017/S1355617710000925
- Nakling, A. E., Aarsland, D., Næss, H., Wollschlaeger, D., Fladby, T., Hofstad, H., & Wehling, E. (2017). Cognitive deficits in chronic stroke patients: Neuropsychological assessment, depression, and self-reports. Dementia and Geriatric Cognitive Disorders Extra, 7(2), 283–296. https://doi.org/10.1159/000478851
- Nijboer, T., Van de Port, I., Schepers, V., Post, M., & Visser-Meily, A. (2013). Predicting functional outcome after stroke: The influence of neglect on basic activities in daily living. Frontiers in Human Neuroscience, 7, 182. https://doi.org/10.3389/fnhum.2013.00182
- Nys, G., Van Zandvoort, M., Van Der Worp, H., De Haan, E., De Kort, P., Jansen, B., & Kappelle, L. (2006). Early cognitive impairment predicts long-term depressive symptoms and quality of life after stroke. Journal of the Neurological Sciences, 247(2), 149–156. https://doi.org/10.1016/j.jns.2006.04.005
- Office for National Statistics. (2018, October 23). Deaths registered in England and Wales (Series Dr): 2017. https://www.ons.gov.uk/peoplepopulationandcommunity/birthsdeathsandmarriages/deaths/bulletins/deathsregisteredinenglandandwalesseriesdr/2017
- Orfei, M., Caltagirone, C., & Spalletta, G. (2009). The evaluation of anosognosia in stroke patients. Cerebrovascular Diseases, 27(3), 280–289. https://doi.org/10.1159/000199466
- Ownsworth, T., Fleming, J., Strong, J., Radel, M., Chan, W., & Clare, L. (2007). Awareness typologies, long-term emotional adjustment and psychosocial outcomes following acquired brain injury. Neuropsychological Rehabilitation, 17(2), 129–150. https://doi.org/10.1080/09602010600615506
- Ownsworth, T. L., McFarland, K., & Young, R. M. (2002). The investigation of factors underlying deficits in self-awareness and self-regulation. Brain Injury, 16(4), 291–309. https://doi.org/10.1080/02699050110103986
- Park, E.-Y., Shin, I.-S., & Kim, J.-H. (2012). A meta-analysis of the variables related to depression in Korean patients with a stroke. Journal of Korean Academy of Nursing, 42, 4. https://doi.org/10.4040/jkan.2012.42.4.537
- Snaith, R., & Zigmond, A. (1986). The Hospital Anxiety and Depression scale. British Medical Journal, 292(6516), 344. https://doi.org/10.1136/bmj.292.6516.344
- Taylor-Rowan, M., Momoh, O., Ayerbe, L., Evans, J. J., Stott, D. J., & Quinn, T. J. (2019). Prevalence of pre-stroke depression and its association with post-stroke depression: A systematic review and meta-analysis. Psychological Medicine, 49(4), 685–696. https://doi.org/10.1017/S0033291718002003
- Toglia, J., & Kirk, U. (2000). Understanding awareness deficits following brain injury. NeuroRehabilitation, 15(1), 57–70. https://doi.org/10.3233/NRE-2000-15104
- Van Rijsbergen, M. W., Mark, R. E., Kop, W. J., de Kort, P. L., & Sitskoorn, M. M. (2019). Psychological factors and subjective cognitive complaints after stroke: Beyond depression and anxiety. Neuropsychological Rehabilitation, 29(10), 1671–1684. https://doi.org/10.1080/09602011.2018.1441720
- Van Rijsbergen, M. W., Mark, R. E., Kop, W. J., De Kort, P. L., & Sitskoorn, M. M. (2020). Course and predictors of subjective cognitive complaints during the first 12 months after stroke. Journal of Stroke and Cerebrovascular Diseases, 29(3), 104588. https://doi.org/10.1016/j.jstrokecerebrovasdis.2019.104588
- Wallace, C. A., & Bogner, J. (2000). Awareness of deficits: Emotional implications for persons with brain injury and their significant others. Brain Injury, 14(6), 549–562. https://doi.org/10.1080/026990500120457
- Westerberg, H., Jacobaeus, H., Hirvikoski, T., Clevberger, P., Östensson, M.-L., Bartfai, A., & Klingberg, T. (2007). Computerized working memory training after stroke–A pilot study. Brain Injury, 21(1), 21–29. https://doi.org/10.1080/02699050601148726
- Williams, O. A., & Demeyere, N. (2021). Association of depression and anxiety with cognitive impairment 6 months after stroke. Neurology, 96(15), e1966–e1974. https://doi.org/10.1212/WNL.0000000000011748
- Wu, Q., Zhou, A.-m., Han, Y.-p., Liu, Y.-m., Yang, Y., Wang, X.-m., & Shi, X. (2019). Poststroke depression and risk of recurrent stroke: A meta-analysis of prospective studies. Medicine, 98(42). https://doi.org/10.1097/MD.0000000000017235