ABSTRACT
We aimed to identify the relationship between variations in metabolic genes and human urinary changes in mercapturic acids (MAs), including CEMA, HMPMA, SPMA, HPMA and HEMA, before and after air pollution exposure. Genotype detection for 47 relevant single nucleotide polymorphisms (SNPs) collected by literature research was performed. Five MAs expression levels in the urinary samples of 50 young healthy individuals with short-term exposure to clean, polluted and purified air at five time points were detected by targeted online solid-phase extraction liquid chromatography tandem mass spectrometry (SPE-LC-MS/MS), followed with associations of SNPs with MAs changes. Difference in MAs between polluted and clean/purified air was significantly associated with 21 SNPs mapped into 9 genes. Five SNPs in GSTP1 showed the most prominent association with the changes in SPMA expression, indicating that those SNPs in GSTP1 and SPMA might serve as biomarkers for susceptibility and the prognosis of lung cancer.
Introduction
Air pollution has been recognized as one of the major health threats worldwide and especially in China, which has air pollution at a much higher level than in developed countries (Guan et al. Citation2016). Fine particulate matter (PM2.5) plays an important role in air pollution and refers to particulate matter with a diameter of less than 2.5 μm (Lin et al. Citation2016), and PM2.5 can be suspended in the air for a long time and thus indicates the degree of air pollution. Numerous epidemiological and toxicological studies have confirmed that exposure to polluted outdoor air is closely associated with an increased risk of cardiovascular (Ma et al. Citation2017; Laeremans et al. Citation2018; Mannucci et al. Citation2019), respiratory (Guan et al. Citation2016; Xing et al. Citation2016; Li et al. Citation2017; Horne et al. Citation2018), neurodegenerative (Babadjouni et al. Citation2017; Cacciottolo et al. Citation2017) and kidney diseases (Bowe et al. Citation2018) and various cancers (Guo et al. Citation2016; Gharibvand et al. Citation2017). The International Agency for Research on Cancer (IARC) has officially designated outdoor air pollution and particulate matter as human carcinogens (Wei et al. Citation2017). There is a causal relationship between PM2.5 and the incidence of lung cancer, asthma, allergic rhinitis and other allergic diseases, especially the deterioration of lung cancer (Takizawa Citation2011). Moreover, PM2.5 was proven to induce microenvironmental and epigenetic alterations in lung cancer, involving the activation of tumor‑associated signaling pathways mediated by DNA methylation, microRNA dysregulation, and elevated cytokine and inflammatory cell levels (Li et al. Citation2017, Citation2018). Since single nucleotide polymorphisms (SNPs) in metabolic genes, such as rs1001179, rs1007991, rs1048943 and rs1050450 (Raaschou-Nielsen et al. Citation2007; Liu et al. Citation2016; Baszuk et al. Citation2021), were reported to be associated with lung cancer, people carrying the SNPs of metabolite genes might be more prone to be affected by PM2.5, which further leads to metabolic changes and high risks in developing lung cancer.
Exposure to polluted air or toxic compounds can be assessed through biological monitoring by using suitable specimens to observe changes in metabolites of interest, including invasive blood samples or noninvasive urinary samples. Metabolites could reflect functional changes induced by external exposure as the end points of many proteins in vivo. Mercapturic acids (N-acetyl-L-cysteine S-conjugates, MAs) are urinary metabolites of environmental and occupational toxicants (Frigerio et al. Citation2019), which are derived from the catabolism of some GSH S-conjugates composed of five MAs. Previous studies have proven that these five metabolites serve as important index factors after exposure to volatile toxicants and carcinogens (Chen et al. Citation2020). Specifically, N-acetyl-S-(2-cyanoethyl)-L-cysteine (2-cyanoethylmercapturic acid, CEMA) is one of the major urinary metabolites derived from acrylonitrile exposure (Bowe et al. Citation2018). N-acetyl-S-(3-hydroxypropyl)-L-cysteine (3-hydroxypropylmercapturic acid, HPMA) (Lindon et al. Citation2010) molecules, apart from 2-carboxyethylmercapturic acid, have been identified as a main urinary metabolite of acrolein (Chen et al. Citation2019). N-acetyl-S-(phenyl)-L-cysteine (S-phenylmercapturic acid, SPMA) is a specific urinary metabolite of benzene and has been commonly recommended as a urinary biomarker for benzene exposure (Schwedler et al. Citation2021). The levels of these urinary biomarkers are considered carcinogenic to humans. N-acetyl-S-(2-hydroxyethyl)-L-cysteine (2-hydroxyethylmethacrylate, HEMA) is a common urinary metabolite of many structurally different xenobiotic chemicals (Vermeulen et al. Citation1989). 3-Hydroxy-1-methylpropylmercapturic acid (HMPMA) is a urinary metabolite of crotonaldehyde (Carmella et al. Citation2013).
Therefore, it would be beneficial for public health researchers to determine if there were intrinsic PM2.5 relationships or greater lung cancer susceptibilities with genetic variations and metabolite changes in humans. The aim of this study was to observe urinary MA changes in 50 healthy young adults with short-term exposure to outdoor air pollution over a time course of polluted and purified air recycling using targeted online solid-phase extraction liquid chromatography tandem mass spectrometry (SPE-LC–MS/MS) analysis. Simultaneously, 47 SNPs in metabolic genes were selected for genotyping assays, according to previous reports where those SNPs were shown to be associated with lung cancer. Next, we conducted a correlation analysis between metabolite changes and SNPs of those subjects to (Nicholson and Lindon Citation2008) discover potential biomarkers for the early monitoring of air pollution as risk factors for lung cancer.
Materials and methods
Chemicals and reagents
The standards of N-acetyl-S-(2-cyanoethyl)-L-cysteine (2-cyanoethylmercapturic acid, CEMA), 3-hydroxy-1-methylpropylmercapturic acid (HMPMA), N-acetyl-S-(phenyl)-L-cysteine (S-phenylmercapturic acid, SPMA), N-acetyl-S-(3-hydroxypropyl)-L-cysteine (3-hydroxypropylmercapturic acid, HPMA) and N-acetyl-S-(2-hydroxyethyl)-L-cysteine (2-hydroxyethylmethacrylate, HEMA) were purchased from Toronto Research Chemicals Inc. (Toronto, Canada) with >98% purity. Formic acid (48–52%) was obtained from Acros Organics Inc. (New Jersey, USA). HPLC-grade methanol and acetonitrile were obtained from TEDIA Company Inc. (Ohio, USA).
Study design and subjects
The study design is shown in detail in . All healthy subjects were recruited from Guang’anmen Hospital, Chinese Academy of Chinese Medical Sciences (Beijing, China). Our study has been approved by the Ethics Committee of Guang’anmen Hospital. Age, height and body weight were obtained from all the subjects. Subjects with one of the following conditions were excluded: (i) subjects who had a smoking history, any pre-existing physical diseases, psychiatric comorbidities or other mental disorders; (ii) pregnant or lactating subjects; and (iii) subjects who suffered from air pollution in the last 4 weeks. This cohort study was approved by the Ethics Committee of Guang’anmen Hospital. All recruited subjects provided written informed consent. The information on the content of daily PM2.5 in 2015 was obtained from the Beijing Meteorological Bureau, which determined the date of sample collection.
Figure 1. Workflow of the research. a total of 50 healthy subjects were recruited according to the enrollment criteria. Peripheral blood and urinary samples of all participants were collected at five different time points, including Point C, Po1, Pu1, Po2 and Pu2. Then, SNP genotyping detection of genomic DNA from peripheral blood at Point C was conducted by an SNP panel, and the expression levels of five mercapturic acids in urinary samples at five points were detected by SPE-LC-MS/MS. Finally, association analysis between SNPs and changes in five mercapturic acids was carried out to discover potential biomarkers for the early monitoring of air pollution as risk factors for lung cancer. Point C: the clean time point; Point Po1: the first polluted time point; Point Pu1: the first purified time point; Point Po2: the second polluted time point; Point Pu2: the second purified time point.
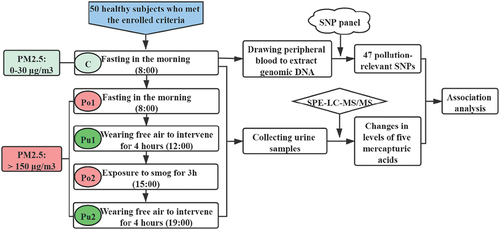
Sample collection
Urine and peripheral blood samples from 50 fasting healthy subjects at the clean time point (Point C) were collected at 8:00 am with PM2.5 ranging from 0 to 30 μg/m3. Urinary samples at the first polluted time point (Point Po1) were collected at 8:00 am with air pollution exposure when the PM2.5 was greater than 150 μg/m3. Next, urinary samples at the first purified time point (Point Pu1) were obtained after the subjects wore positive pressure protective masks (named PPM masks, provided by Hongxinghaoyang Technology Co., Beijing, China) for 4 h at 12:00 am. Subsequently, urinary samples at the second polluted time point (Point Po2) were received after re-exposure to polluted outdoor air for 3 h at 15:00 pm. Finally, urinary samples at the second purified time point (Point Pu2) were gathered after repurification by PPM masks for another 4 h at 19:00 pm. All participants wore or took off their PPM masks according to the sample collection procedure and consumed the same amount of nutrients (Pediasure, 200 g = 900 kJ each time) and distilled water (1500 ml each time) two times throughout the day (from 8:00 am to 19:00 pm). The PPM masks that the participants used were positive pressure respirators, which could quickly reduce PM2.5 to less than 10 μg/m3 and maintain it at 0 μg/m3 in a stable environment, as reported by our previous study.
Genotyping assay
To date, many metabolism-related SNPs have been reported to be related to air pollution and to be significantly associated with the occurrence of lung cancer. A total of 47 SNPs corresponding to 20 metabolic genes were selected through a literature search to detect genotypes in the participants in the present study. General information about these SNPs is given in Supplementary Table S1–1. Genomic DNA from peripheral blood at Point C of 50 healthy subjects was isolated by a TIANamp Blood DNA Midi Kit (Tiangen, Beijing, China). An SNP panel for genotyping by the MassARRAY genotyping platform (Sequenome) based on distinguishing allele-specific primer extension products by mass spectrometry (MALDI-TOF) was selected for SNP genotyping detection. After the iPLEX reactions in a 5 μl final PCR volume of 384-well plates, including the initial denaturation, annealing, extension and final extension steps, the products were desalted and dispensed following the manufacturer’s protocol. Finally, the array was introduced into a MassARRAY Compact 96 mass spectrometer. Spectra were acquired using SpectroAcquire software for data analysis with MassARRAY Typer software, version 4.0.5. PCR primers for each SNP investigated were designed using MassARRAY design software, version 3.1 (Sequenom Inc., San Diego, CA), as shown in Supplementary Table S2. The optimal amplicon size was set to 80–120 bp for the target sequences.
Targeted on-line SPE-LC–MS/MS analysis
The urinary samples of 50 healthy subjects at different points were quickly transferred to sterile tubes and centrifuged at 1500 g for 10 min at room temperature. The supernatants were prepared to detect five MAs (CEMA, HEMA, SPMA, HPMA and HMPMA) using an online solid-phase extraction (SPE)-LC–MS/MS system including an online SPE column and an ultra-performance liquid chromatography/electrospray ionization (ESI)-based mass spectrometer. The online SPE column was a Cartridge C18 MGII (S3) (4.0 × 10 mm) washed with a gradient mobile phase containing 0.1% formic acid and 0.1% formic acid-acetonitrile. Analyte separation was performed using an Ultimate 3000 system (Thermo Fisher Scientific, USA) consisting of an RS Autosampler, a TCC-3000SD Column Oven and an RSLCnano system according to the following conditions: Shiseido ADME column (2.1 mm × 150 mm, 3 μm); flow rate: 0.3 ml/min; column temperature: 30°C mobile phase A: 0.1% formic acid; mobile phase B: 0.1% formic acid–acetonitrile; gradient elution. The LC system was coupled to an electrospray ionization (ESI)-based mass spectrometer (Thermo Fisher Scientific, USA) equipped with a Turbo V™ ion spray source operating in negative ion mode under the following conditions: electrospray voltage, 4000 V; capillary temperature, 375°C capillary voltage, 11 V; sheath gas (N2) pressure, 34 arb; auxiliary gas (N2) pressure, 17 arb; and purge gas (N2) pressure, 0 arb. Selective reaction monitoring (SRM) was used for data acquisition.
Time series analysis
Through targeted online SPE-LC–MS/MS analysis, the expression levels of five MAs (CEMA, HMPMA, SPMA, HPMA and HEMA) in 50 biological replicate urinary samples were obtained at 5 different time points of Point C, Po1, Pu1, Po2 and Pu2. Next, we performed time-series analysis to understand their dynamic patterns and functional relationships. Therefore, the mean expression values of each mercapturic acid at each time point could be calculated, followed by Z-score normalization. Finally, trends in the changes of those five metabolites in a time course could be visualized by using the R package to plot normalized Z-scores.
Correlation analysis
After the datasets were obtained from online SPE-LC–MS/MS and genotyping assays, an integrative analysis was conducted to identify SNPs that were likely to affect the expression of MAs leading to the etiology of lung cancer through air pollution. First, the fold change (FC) value of either Po1/C or Pu1/Po1 for each mercapturic acid was calculated with their logarithmic values. Second, the correlation analysis of the FC values with each genotyping data for a total of 47 SNPs of 50 healthy subjects was performed by using the R program to acquire the Pearson correlation coefficient (represented by r) and a significant P value.
Kyoto Encyclopedia of Genes and Genomes (KEGG) pathway enrichment analysis
After obtaining gene datasets that had a significant correlation with mercapturic acid changes, plus a total of 47 SNPs corresponding to 20 metabolic genes for genotyping, pathway enrichment analysis was carried out using the KEGG database to draw pathway maps for the two gene datasets.
Statistical analysis
Assessment of physiological factors, including age, sex and body mass index (BMI), for the participants was performed using Student’s t-test and presented as the mean ± SEM. Correlation analysis of SNP genotyping and changes in MAs was conducted using the R package to obtain Pearson’s correlation coefficient and the P value. A P value ≤.05 was considered to be a significant difference.
Results
Clinical characteristics of healthy subjects
Based on the inclusion and exclusion criteria, a total of 50 young healthy subjects were recruited for the study. As shown in , all the participants were in the range of 22–28 years old, and the distribution of males and females was relatively balanced. The peripheral blood and urinary samples of all participants were successfully collected at Point C, Po1, Pu1, Po2 and Pu2 according to the sample collection procedure. The flowchart of the procedure in this study is shown in . Specifically, after short-term exposure to outdoor polluted or purified air carrying positive pressure protective masks, peripheral blood and urinary samples were collected at five different time points from all healthy participants who were instructed according to the sample collection procedure throughout the testing day .
Table 1. General characteristics of 50 healthy participants.
Table 2. Genotype of 47 pollution-relevant SNPs selected on the basis of their biological literature search.
Genotyping of the 47 SNPs in 50 healthy subjects
Through a literature search, a total of 47 SNPs corresponding to 20 metabolic genes were selected for genotyping detection. Genotyping of 47 SNPs in 50 subjects were summarized in Table 2and Supplementary Table S3. As a result of KEGG pathway analysis, those 20 mapped genes were mainly involved in metabolic and cancer-related pathways, including the metabolism of xenobiotics by cytochrome P450, tryptophan metabolism, glutathione metabolism, chemical carcinogenesis, proteoglycans in cancer and the Toll-like receptor signaling pathway and related diseases caused by pathogens, bacteria and viruses (Supplementary Table S1–2).
Dynamic changes in the expression levels of five MAs over a time course
The mean values of the expression levels of five MAs (CEMA, HMPMA, SPMA, HPMA and HEMA) in urinary samples of 50 subjects at five time points were obtained by targeted online SPE-LC–MS/MS after Z-score normalization. The expression levels of these five MAs showed a sharp increase in the urinary samples of participants when the participants were exposed to a polluted air environment (Point Po1) compared to a clean airborne ambient environment (Point C), indicating significant changes in metabolic levels of humans affected by polluted air. After the participants wore PPM-masks for 4 h (Point Pu1), the expression levels of MAs dropped and had a similar level to that of Point C. With additional 3 hours of continuous re-exposure to outdoor polluted air (Point Po2), the content of all the MAs in urine changed very little except for SPMA (as shown in ), whereas SPMA levels reached up to that at Point Po1. Finally, the expression levels of all five MAs at Point Pu2 were even lower than those at Point C with air repurification when the participants wore PPM masks for the last 4 h at the end of the study (, Supplementary Table S4). This suggests that the change intendency of those four metabolites (CEMA, HMPMA, HPMA and HEMA) showed a trend of up-low-up-plateau. However, the expression levels of SPMA in urine showed the most sensitive changes during those five time points. SPMA could be an early responsive indicator in the human body to airborne PM pollution with real-time alteration accompanied by a changing trend in the constituents of the outdoor environment. Consequently, our findings demonstrated that the intensity of changes in the five metabolites might reflect different healthy states under different conditions of clean (Point C, Pu1 and Pu2) and polluted air (Point Po1 and Po2) ambient exposure.
Figure 2. Expression levels of five mercapturic acids in urinary samples at five different time points. Expression abundance represented the value after taking the average expression of mercapturic acids in 50 samples and normalizing by Z-score. CEMA: N-acetyl-S-(2-cyanoethyl)-cysteine; HMPMA: 3-hydroxy-1-methylpropylmercapturic acid; SPMA: N-acetyl-S-phenyl-L-cysteine; HPMA: N-acetyl-S-(3-hydroxypropyl)-l-cysteine; HEMA: N-acetyl-S-(2-hydroxyethyl)-L-cysteine.
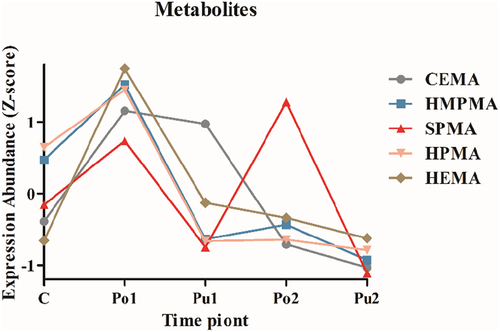
Correlation analysis between the changes of MAs and SNPs
The ratios of log2 (Po1/C) and log2 (Pu1/Po1) for CEMA, HMPMA, SPMA, HPMA and HEMA had significant relationships with 21 SNPs that were mapped into 9 genes, including GSTP1, GSTT1, SOD3, TP63, CYBA, CAT, EPHX1, TLR2 and TLR4 (and Supplementary Table S5). There were significant relationships between log2 (Pu1/Po1) values of SPMA and rs1695, rs1871042, rs4891, rs749174 and rs762803 in the GSTP1 gene, indicating that subjects with these SNPs were more sensitive to alterations in urinary SPMA levels accompanied by a shift in airborne PM levels from polluted air to purified air by PPM masks. Furthermore, significant associations of rs1927911 and rs10759932 in the TLR4 gene with either log2 (Po1/C) or log2 (Pu1/Po1) of SPMA, HPMA or HEMA were also observed. In addition, rs2695231, rs8192287 and rs699473 in the SOD3 gene were significantly correlated with log2 (Po1/C) and log2 (Pu1/Po1) of HPMA, respectively.
Table 3. A total of 21 SNPs mapped to 9 genes had significant relationships with changes in expression levels of five mercapturic acids (P < .05).
In summary, the correlation was highly significant between SNPs in the GSTP1, SOD3 and TLR4 genes and fold changes of Po1/C and Pu1/Po1 for SPMA, HPMA and HEMA (and Supplementary Table S5). Interestingly, the five SNPs in GSTP1 showed the most prominent associations with changes in SPMA expression. Moreover, the 21 SNPs corresponding to 9 genes were mainly involved in leishmaniasis, the metabolism of xenobiotics by cytochrome P450, chemical carcinogenesis and the phagosome and malaria pathways and with P values no more than 0.05 in the KEGG pathway analysis (). The above results indicate that the combination of these SNPs with metabolic changes in SPMA, HPMA and HEMA would be a potential index for assessing the possibility of lung cancer occurrence.
Discussion
Lung cancer is a common malignant cancer, accounting for 27% of the global malignant cancers. Its incidence and mortality rate ranks first among all cancers (Siegel et al. Citation2017, Citation2018). Although smoking is the leading risk factor for lung cancer, increasing attention has been focused on the correlation between environmental pollution, genetic variation, or their interactions and lung cancer occurrence (Parkin et al. Citation2005; Carmella et al. Citation2009). In the present study, we found that a total of 21 SNPs corresponding to 9 genes were significantly related to changes in the five MAs in urine, implying the importance of the genes that function in metabolic pathways involved in SPMA, HPMA and HEMA.
Glutathione S transferase Pi 1 (GSTP1), a widely studied member of the glutathione S transferase (GST) family, has many physiological functions, including metabolism, detoxification and elimination of a variety of carcinogenic compounds and the protection of cells against DNA damage or cancer (Cui et al. Citation2020). Previous studies have shown that people who smoke tobacco and carry the GSTP1-rs1695 polymorphism are susceptible to lung cancer (Kudhair et al. Citation2020). Polymorphisms, such as rs1695 and rs1871042, could increase the incidence of asthma by five fold (Joubert et al. Citation2011). The polymorphisms of rs1695, rs4891, rs749174 and rs762803 showed a significant association with lung cancer susceptibility in smokers (Gu et al. Citation2014). Prior research suggests that GSTP1 polymorphism may be associated with the detoxification of polycyclic aromatic hydrocarbons and lung cancer susceptibility (Li et al. Citation2015). Therefore, GSTP1 polymorphisms may be considered a potential biomarker for cancer risk and a prognostic marker for cancer patients (Wang et al. Citation2015). SPMA is a specific urinary metabolite of benzene, which has been commonly recommended as a urinary biomarker for carcinogenic benzene exposure (Schwedler et al. Citation2021). Our work hence revealed that the GSTP1 polymorphism represented a significant association with the log2 (Pu1/Po1) of SPMA in urine, indicating that subjects with these SNPs might be prone to be affected in a timely manner by changes in urinary SPMA expression attributed to carcinogenic benzene in airborne ambient conditions. Therefore, the combination of these SNPs and SPMA might be considered a more precise biomarker for the susceptibility and prognosis of lung cancer.
Toll-like receptor 4 (TLR4) was the first discovered human homolog of the Dorsophila Toll protein, which is responsible for selective recognition of the endotoxin LPS (Shetab Boushehri and Lamprecht Citation2018). The TLR4 signaling pathway is involved in cancer progression and inflammatory response. Previous studies have emphasized that active TLR4 can increase IL-8, IL-6 and nitric oxide production and the expression of VEGF and TGF-β1. The release of various inflammatory mediators, cytokines and chemokines by active TLR4 could contribute to cancer progression (Chen et al. Citation2018). We found that SNPs in TLR4 were closely related to Po1/C- or Pu1/Po1-fold changes in urinary SPMA, HPMA or HEMA. These findings further confirmed that TLR4 polymorphisms had impacts on the changes in the level of MAs in urine after exposure to polluted air, which were considered carcinogenic biomarkers in humans.
Superoxide dismutase 3 (SOD3) is able to eliminate reactive oxygen species (ROS) that are produced to characterize oxidative stress by metabolism in the human body and to resist the damage of superoxide ions in both the internal and external environments (Gaurav et al. Citation2017; Sun et al. Citation2019). ROS are well known to contribute to chronic airway inflammation, bronchial asthma, chronic obstructive pulmonary disease (COPD), bronchiectasis, interstitial lung disease and other lung diseases. As mentioned above, rs2695231, rs8192287 and rs699473 in SOD3 were obviously associated with log2 (Po1/C) or log2 (Pu1/Po1) of HPMA in urine as a main urinary metabolite of acrolein. Our findings also demonstrated that these SNPs in the SOD3 gene would greatly affect HPMA levels after polluted air exposure, considered carcinogenic biomarkers in humans.
Taken together, our study highlighted the important correlations between metabolic gene polymorphisms and the expression changes of MAs in urine after short-term exposure to polluted air or purified air. The combination of the SNPs in GSTP1, TLR4 and SOD3 with SPMA, HPMA and HEMA might be utilized to generate a prediction model for the human health effect of air pollution and might be considered more precise biomarkers for the early screening of lung cancer susceptibility. Inevitably, our work clearly has some limitations. (1) This study focused only on 47 SNPs corresponding to 20 metabolic genes and five MAs in urine as metabolic biomarkers obtained only by a recent literature search, which is not enough to cover all environmentally relevant genes and metabolites. Therefore, more genetic variations of metabolic genes and more metabolites in urine should be detected on genomic and metabolomic scales, and further research is needed to illustrate the relationships between them. (2) The sample size of healthy young adults was small in our study due to the budget, but a larger number of samples, including human subjects aged 18–65 years, might be more beneficial for designing a validation study in the future.
Conclusions
This study indicated that subjects with these SNPs in GSTP1 were more likely to be altered in urinary SPMA levels, which was accompanied by a shift in airborne PM levels from ambient pollution to purification. This suggests that those SNPs might be predictive biomarkers for air pollution and risk factors in the occurrence of lung cancer and that SPMA could be an early responsive indicator to monitor the effect of airborne pollution on human health. The combination of those SNPs in GSTP1 and SPMA expression might be further considered as a more precise biomarker for susceptibility and prognosis of lung cancer.
Ethics approval and consent to participate
The study was approved by the Institutional Review Board of Guang’anmen Hospital, Chinese Academy of Chinese Medical Sciences, Beijing, China.
Supplemental Material
Download MS Word (119.3 KB)Acknowledgments
We thank all the young participants in the study.
Disclosure statement
No potential conflict of interest was reported by the author(s).
Data availability statement
All the datasets in the current study are available from the corresponding author upon reasonable request.
Supplementary Materials
Supplemental data for this article can be accessed here.
Additional information
Funding
References
- Babadjouni RM, Hodis DM, Radwanski R, Durazo R, Patel A, Liu Q, Mack WJ. 2017. Clinical effects of air pollution on the central nervous system; a review. J Clin Neurosci. 43:16–24. eng. doi:10.1016/j.jocn.2017.04.028.
- Baszuk P, Janasik B, Pietrzak S, Marciniak W, Reszka E, Białkowska K, Jabłońska E, Muszyńska M, Lesicka M, Derkacz R, et al. 2021. Lung cancer occurrence-correlation with serum chromium levels and genotypes. Biol Trace Elem Res. 199(4):1228–1236. eng. doi:10.1007/s12011-020-02240-6.
- Bowe B, Xie Y, Li T, Yan Y, Xian H, Al-Aly Z. 2018. Particulate matter air pollution and the risk of incident CKD and progression to ESRD. J Am Soc Nephrol. 29(1):218–230. eng. doi:10.1681/ASN.2017030253.
- Cacciottolo M, Wang X, Driscoll I, Woodward N, Saffari A, Reyes J, Serre ML, Vizuete W, Sioutas C, Morgan TE, et al. 2017. Particulate air pollutants, APOE alleles and their contributions to cognitive impairment in older women and to amyloidogenesis in experimental models. Transl Psychiatry. 7(1):e1022. eng. doi:10.1038/tp.2016.280.
- Carmella SG, Chen M, Han S, Briggs A, Jensen J, Hatsukami DK, Hecht SS. 2009. Effects of smoking cessation on eight urinary tobacco carcinogen and toxicant biomarkers. Chem Res Toxicol. 22(4):734–741. eng. doi:10.1021/tx800479s.
- Carmella SG, Chen M, Zarth A, Hecht SS. 2013. High throughput liquid chromatography-tandem mass spectrometry assay for mercapturic acids of acrolein and crotonaldehyde in cigarette smokers’ urine. J Chromatogr B Analyt Technol Biomed Life Sci. 935:36–40. eng. doi:10.1016/j.jchromb.2013.07.004.
- Chen M, Carmella SG, Li Y, Zhao Y, Hecht SS. 2020. Resolution and quantitation of mercapturic acids derived from crotonaldehyde, methacrolein, and methyl vinyl ketone in the urine of smokers and nonsmokers. Chem Res Toxicol. 33(2):669–677. eng. doi:10.1021/acs.chemrestox.9b00491.
- Chen M, Carmella SG, Sipe C, Jensen J, Luo X, Le CT, Murphy SE, Benowitz NL, McClernon FJ, Vandrey R, et al. 2019. Longitudinal stability in cigarette smokers of urinary biomarkers of exposure to the toxicants acrylonitrile and acrolein. PloS One. 14(1):e0210104. eng. doi:10.1371/journal.pone.0210104.
- Chen CY, Kao CL, Liu CM. 2018. The cancer prevention, anti-inflammatory and anti-oxidation of bioactive phytochemicals targeting the TLR4 signaling pathway. Int J Mol Sci. 19(9):2729. eng. doi:10.3390/ijms19092729.
- Cui J, Li G, Yin J, Li L, Tan Y, Wei H, Liu B, Deng L, Tang J, Chen Y, et al. 2020. GSTP1 and cancer: expression, methylation, polymorphisms and signaling (Review). Int J Oncol. 56(4):867–878. eng. doi:10.3892/ijo.2020.4979.
- Frigerio G, Mercadante R, Polledri E, Missineo P, Campo L, Fustinoni S. 2019. An LC-MS/MS method to profile urinary mercapturic acids, metabolites of electrophilic intermediates of occupational and environmental toxicants. J Chromatogr B Analyt Technol Biomed Life Sci. 1117:66–76. eng. doi:10.1016/j.jchromb.2019.04.015.
- Gaurav R, Varasteh JT, Weaver MR, Jacobson SR, Hernandez-Lagunas L, Liu Q, Nozik-Grayck E, Chu HW, Alam R, Nordestgaard BG, et al. 2017. The R213G polymorphism in SOD3 protects against allergic airway inflammation. JCI Insight. 2(17):e95072. eng. doi:10.1172/jci.insight.95072.
- Gharibvand L, Shavlik D, Ghamsary M, Beeson WL, Soret S, Knutsen R, Knutsen SF. 2017. The association between ambient fine particulate air pollution and lung cancer incidence: results from the AHSMOG-2 study. Environ Health Perspect. 125(3):378–384. eng. doi:10.1289/EHP124.
- Gu JD, Hua F, Mei CR, Zheng DJ, Wang GF, Zhou QH. 2014. HapMap-Based study on the association between MPO and GSTP1 gene polymorphisms and lung cancer susceptibility in Chinese Han population. Acta Pharmacol Sin. 35(5):636–644. eng. doi:10.1038/aps.2014.11.
- Guan WJ, Zheng XY, Chung KF, Zhong NS. 2016. Impact of air pollution on the burden of chronic respiratory diseases in China: time for urgent action. Lancet (London, England). 388(10054):1939–1951. eng. doi:10.1016/S0140-6736(16)31597-5.
- Guo Y, Zeng H, Zheng R, Li S, Barnett AG, Zhang S, Zou X, Huxley R, Chen W, Williams G. 2016. The association between lung cancer incidence and ambient air pollution in China: a spatiotemporal analysis. Environ Res. 144(Pt A):60–65. eng. doi:10.1016/j.envres.2015.11.004.
- Horne BD, Joy EA, Hofmann MG, Gesteland PH, Cannon JB, Lefler JS, Blagev DP, Korgenski EK, Torosyan N, Hansen GI, et al. 2018. Short-term elevation of fine particulate matter air pollution and acute lower respiratory infection. Am J Respir Crit Care Med. 198(6):759–766. eng. doi:10.1164/rccm.201709-1883OC.
- Joubert BR, Reif DM, Edwards SW, Leiner KA, Hudgens EE, Egeghy P, Gallagher JE, Hubal EC. 2011. Evaluation of genetic susceptibility to childhood allergy and asthma in an African American urban population. BMC Med Genet. 12(1):25. eng. doi:10.1186/1471-2350-12-25.
- Kudhair BK, Alabid NN, Taheri-Kafrani A, Lafta IJ. 2020. Correlation of GSTP1 gene variants of male Iraqi water pipe (Hookah) tobacco smokers and the risk of lung cancer. Mol Biol Rep. 47(4):2677–2684. eng. doi:10.1007/s11033-020-05359-w.
- Laeremans M, Dons E, Avila-Palencia I, Carrasco-Turigas G, Orjuela JP, Anaya E, Cole-Hunter T, de Nazelle A, Nieuwenhuijsen M, Standaert A, et al. 2018. Short-term effects of physical activity, air pollution and their interaction on the cardiovascular and respiratory system. Environ Int. 117:82–90. eng. doi:10.1016/j.envint.2018.04.040.
- Li G, Lan H, Liu Z, Rui T, Lu J, Bian L, Wang Y, Wang S, Zhang H, Bian Y, et al. 2017. The association between short-term exposure to fine particulate matter and outpatient visit in Beijing, China. Iran J Public Health. 46(11):1486–1494. eng.
- Li J, Li WX, Bai C, Song Y. 2017. Particulate matter-induced epigenetic changes and lung cancer. Clin Respir J. 11(5):539–546. eng. doi:10.1111/crj.12389.
- Li XM, Yu XW, Pu MZ, Zhang HX, Wang KJ, Han XD. 2015. Glutathione S-transferase P1, gene-gene interaction, and lung cancer susceptibility in the Chinese population: an updated meta-analysis and review. J Cancer Res Ther. 11(3):565–570. eng. doi:10.4103/0973-1482.163788.
- Li R, Zhou R, Zhang J. 2018. Function of PM2.5 in the pathogenesis of lung cancer and chronic airway inflammatory diseases. Oncol Lett. 15(5):7506–7514. eng. doi:10.3892/ol.2018.8355.
- Lin YC, Hsu SC, Chou CK, Zhang R, Wu Y, Kao SJ, Luo L, Huang CH, Lin SH, Huang YT. 2016. Wintertime haze deterioration in Beijing by industrial pollution deduced from trace metal fingerprints and enhanced health risk by heavy metals. Environ Pollut. 208(Pt A):284–293. doi:10.1016/j.envpol.2015.07.044.
- Lindon JC, Holmes E, Bollard ME, Stanley EG, Nicholson JK. 2010. Metabonomics technologies and their applications in physiological monitoring, drug safety assessment and disease diagnosis. Biomarkers. 9(1):1–31. doi:10.1080/13547500410001668379.
- Liu HX, Li J, Ye BG. 2016. Correlation between gene polymorphisms of CYP1A1, GSTP1, ERCC2, XRCC1, and XRCC3 and susceptibility to lung cancer. Genet Mol Res. 15(4). LID - eng. doi:10.4238/gmr15048813.
- Ma Y, Zhao Y, Yang S, Zhou J, Xin J, Wang S, Yang D. 2017. Short-Term effects of ambient air pollution on emergency room admissions due to cardiovascular causes in Beijing, China. Environ Pollut. 230:974–980. eng. doi:10.1016/j.envpol.2017.06.104.
- Mannucci PM, Harari S, Franchini M. 2019. Novel evidence for a greater burden of ambient air pollution on cardiovascular disease. Haematologica. 104(12):2349–2357. eng. doi:10.3324/haematol.2019.225086.
- Nicholson JK, Lindon JC. 2008. Systems biology: metabonomics. Nature. 455(7216):1054–1056. eng. doi:10.1038/4551054a.
- Parkin DM, Bray F, Ferlay J, Pisani P. 2005. Global cancer statistics, 2002. CA Cancer J Clin. 55(2):74–108. eng. doi:10.3322/canjclin.55.2.74.
- Raaschou-Nielsen O, Sørensen M, Hansen RD, Frederiksen K, Tjønneland A, Overvad K, Vogel U. 2007. GPX1 Pro198Leu polymorphism, interactions with smoking and alcohol consumption, and risk for lung cancer. Cancer Lett. 247(2):293–300. eng. doi:10.1016/j.canlet.2006.05.006.
- Schwedler G, Murawski A, Schmied-Tobies MIH, Rucic E, Scherer M, Pluym N, Scherer G, Bethke R, Kolossa-Gehring M. 2021. Benzene metabolite SPMA and acrylamide metabolites AAMA and GAMA in urine of children and adolescents in Germany - human biomonitoring results of the German Environmental Survey 2014-2017 (GerES V). Environ Res. 192:110295. eng. doi:10.1016/j.envres.2020.110295.
- Shetab Boushehri MA, Lamprecht A. 2018. TLR4-based immunotherapeutics in cancer: a review of the achievements and shortcomings. Mol Pharm. 15(11):4777–4800. eng. doi:10.1021/acs.molpharmaceut.8b00691.
- Siegel RL, Miller KD, Jemal A. 2017. Cancer statistics, 2017. CA Cancer J Clin. 67(1):7–30. eng. doi:10.3322/caac.21387.
- Siegel RL, Miller KD, Jemal A. 2018. Cancer statistics, 2018. CA Cancer J Clin. 68(1):7–30. eng. doi:10.3322/caac.21442.
- Sun SQ, Gao N, Hu XQ, Luo H, Peng J, Xia Y. 2019. SOD3 overexpression alleviates cerebral ischemia-reperfusion injury in rats. Mol Genet Genomic Med. 7(10):e00831. eng. doi:10.1002/mgg3.831.
- Takizawa H. 2011. Impact of air pollution on allergic diseases. Korean J Intern Med. 26(3):262–273. doi:10.3904/kjim.2011.26.3.262.
- Vermeulen NP, Jong JF, Bergen EJ, Welie RT. 1989. N-Acetyl-S-(2-Hydroxyethyl)-L-Cysteine as a potential tool in biological monitoring studies? a critical evaluation of possibilities and limitations. Arch Toxicol. 63(3):173–184. eng.
- Wang Y, Ren BU, Zhang L, Guo Z. 2015. Correlation between metabolic enzyme GSTP1 polymorphisms and susceptibility to lung cancer. Exp Ther Med. 10(4):1521–1527. eng. doi:10.3892/etm.2015.2666.
- Wei H, Liang F, Cheng W, Zhou R, Wu X, Feng Y, Wang Y. 2017. The mechanisms for lung cancer risk of PM(2.5) : induction of epithelial-mesenchymal transition and cancer stem cell properties in human non-small cell lung cancer cells. Environ Toxicol. 32(11):2341–2351. eng. doi:10.1002/tox.22437.
- Xing YF, Xu YH, Shi MH, Lian YX. 2016. The impact of PM2.5 on the human respiratory system. J Thorac Dis. 8(1):E69–E74. eng. doi:10.3978/j.issn.2072-1439.2016.01.19.