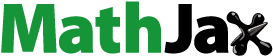
ABSTRACT
Integrating multiple hyperspectral linear-array cameras into a Linear-array Multi-camera System (LMS) enables an expanded Field of View (FOV) while preserving high spatial resolution. However, the constrained capabilities of tie points are weakened by the limited overlap and short baseline between sub-cameras, hindering the attainment of reliable solutions for the theoretically ideal relative calibration model. Additionally, the absence of stereoscopic imaging in the LMS introduces the challenge of acquiring dense Ground Control Points (GCPs) throughout the FOV during calibration. In this paper, for calibrating an advanced airborne hyperspectral LMS named Arial Hyperspectral System-1000 (AHS-1000), we propose an equivalent geometric model that transforms the three-camera configuration of the LMS into a single-camera representation on the master camera’s focal plane, which was successfully applied to the data processing system of AHS-1000. We extracted dense GCPs by matching each Level-1 sub-image of the LMS with the bundled images of an existing three-line-scanner aerial image dataset, whose orientation parameters have been accurately solved by bundle adjustment with sparse GCPs; the matched points of each sub-image were consistently projected onto the master focal plane as image observations for our equivalent model. Finally, we established a collinearity equation system and performed a combined strip adjustment to calibrate the distortion terms for each pixel of the equivalent camera. The results demonstrate the effectiveness and robustness of our equivalent modeling method, which outperforms the classic modeling method by 2.83 pixels in interior accuracy and 0.86 pixels in relative accuracy, as well as outperforming another similar equivalent modeling method by 1.22 pixels in interior accuracy and 0.18 pixels in relative accuracy. Additionally, our comparison utilizing bundled images, public DOM (Digital Orthophoto Maps) /DEM (Digital Elevation Models), and high-precision DOM/DEM from aerial three-line-scanner demonstrates that bundled images can achieve higher calibration accuracy.
1. Introduction
To enhance the efficiency of data collection and processing, airborne hyperspectral sensors are anticipated to possess high spectral resolution, high spatial resolution, and a large Field of View (FOV) concurrently (Jia et al. Citation2020). As an alternative to intricate and challenging solutions in optical design aimed at overcoming spatial limitations of individual hyperspectral sensors, the assembly of multiple hyperspectral linear-array cameras into a Linear-array Multi-camera System (LMS) is often considered a more favorable approach for expanding the FOV while maintaining high spatial resolution (Hu et al. Citation2005; Zhang et al. Citation2019). Due to independent data collection, the sub-cameras often suffer from geometric misalignments, necessitating the accurate recovery of their Relative Orientation Parameters (ROP) for seamless image stitching. Furthermore, to ensure both seamless stitching and precise georeferencing, it is essential to perform calibration for the LMS, taking into account the variations in the Interior Orientation Parameters (IOP) of the sub-cameras caused by their inherent geometric tolerances.
Achieving overall optimization of both interior accuracy and relative accuracy during the LMS calibration is a challenging task. Theoretically, accomplishing reliable LMS calibration involves establishing relative orientation constraints between sub-cameras based on corresponding points, as well as imposing interior accuracy constraints on each sub-camera using Ground Control Points (GCPs) (Gruen and Zhang Citation2002; Hartley Citation1997; Nister Citation2004). Nonetheless, the limited overlap between adjacent sub-cameras presents difficulties in accurately matching corresponding points, and uncertainty in the object-space coordinates of tie points increases due to the small baseline, prompting the development of equivalent geometric models in many studies (Cheng et al. Citation2018; Jiang et al. Citation2017). These models treat the misalignments caused by ROP as part of errors in IOP and compensate for them by utilizing the constraints provided by GCPs. Despite this progress, quantitative comparisons between these equivalent models and ideal models remain scarce. Additionally, it is essential to simultaneously formulate and solve the distortion parameters for all sub-cameras, rather than pursuing separate step-by-step approaches. In the case of the 5-camera system’s geometric stitching on the HY-1C satellite, Cao et al. (Citation2020) first calibrated the IOP and the Exterior Orientation Parameters (EOP) of the master camera and then determined the IOP of the slave cameras. However, there is often a strong correlation between the IOP and EOP for linear-array imagery (e.g. the position of the array along the flight direction correlates with the pitch angle); consequently, this sequencing approach can result in the EOP obtained from bundle adjustment being locally optimal only for the master camera, rather than representing the overall optimal solution for the multi-camera system.
Another challenge in LMS calibration is the specific requirements for GCP density and distribution. Unlike Three-Line-Scanner (TLS) imaging systems, which can perform interior orientation calibration with a limited number of GCPs and utilize matching points from multi-view images, LMS lacks stereoscopic imaging conditions, necessitating denser GCP coverage across the entire FOV for accurate calibration (Leprince, Muse, and Avouac Citation2008). An alternative approach is to establish calibration strips with large overlaps; however, this method could significantly increase the complexity of data acquisition and calibration processing (Lenz et al. Citation2014). Therefore, in situations where high-cost ground calibration fields are unavailable, leveraging existing data or products with comparable or higher resolution imagery to generate dense digital GCPs becomes a natural consideration. While digital GCPs have been widely employed in the on-orbit calibration of spaceborne sensors (Chen et al. Citation2015; Tao et al. Citation2014; Wang et al. Citation2017), their utilization in aerial LMS calibration remains underexplored. The effectiveness and comparative analysis of different strategies for obtaining digital GCPs, such as extracting them from original images after bundle adjustment or from Digital Orthophoto Maps (DOM) and Digital Elevation Models (DEM) products, still lack research and clarity.
In this paper, we focus on the calibration problem of the Arial Hyperspectral System-1000 (AHS-1000), which is a recently modified version of a Chinese-made airborne hyperspectral LMS (Wang et al. Citation2016). Building upon the concept of equivalent geometric modeling, we transform the three-camera configuration of the LMS into an equivalent representation of a single camera by designating the intermediate camera as the master and projecting the slave cameras’ linear arrays onto its focal plane. Furthermore, since there was a recent aerial photogrammetry mission conducted by a TLS, we set the calibration data collection area to be consistent with the range of the TLS mission. This allows us to utilize the TLS images after bundle adjustment as geo-referencing data. Specifically, we first project the original sub-images onto the plane with the mean terrain height of the imaging area using the Position and Orientation System (POS) data, resulting in Level-1 images that overcome the geometric deformation caused by airborne platform jitter. Then, we extract dense corresponding points from TLS images for each Level-1 sub-image by image matching, the corresponding points located at forward, nadir, and backward images of TLS are further utilized to generate digital GCPs. Under the constraints of these GCPs, we reproject the matched points for the three Level-1 sub-images consistently onto the master focal plane and use their projected coordinates as image observations for the equivalent geometric model. Finally, we establish a collinearity equation system based on these observations and perform a combined strip adjustment, solving the EOP of the LMS and calibrating the distortion terms for each pixel of the equivalent camera. The proposed method has been applied to the AHS-1000 data processing system and validated for its effectiveness.
The experimental results validate the effectiveness and robustness of our proposed approach, which outperforms the ideal relative orientation calibration method by 2.83 pixels in interior accuracy and 0.86 pixels in relative accuracy, as well as outperforms the equivalent modeling method used by Cao et al. (Citation2020) by 1.22 pixels in interior accuracy and 0.18 pixels in relative accuracy. Furthermore, we performed a confirmatory analysis between the proposed georeferencing strategy, which utilizes existing bundled images, and the strategy employing DOM/DEM from public data sources or aerial TLS data. The comparison revealed that the proposed strategy exhibited a more concentrated distribution of GCP residuals, resulting in a more accurate calibration outcome. Additionally, we evaluated the georeferencing accuracy of the LMS after calibration, showing a reduction in checkpoint error along the sample direction from the original 1.52 pixels to 0.66 pixels. Our main contributions can be summarized as follows:
By projecting the linear arrays of the slave cameras onto the focal plane of the master camera, we model the LMS as an equivalent single camera for geometric calibration, which facilitates holistic optimization of both interior and relative accuracy.
We apply the proposed equivalent geometric model to a newly developed airborne hyperspectral LMS, AHS-1000, and experimentally compare it with the ideal relative calibration model and a similar equivalent modeling method, thus validating its advantages and stability.
By utilizing the existing bundled images and their orientation parameters for LMS calibration, we qualitatively demonstrate that this georeferencing strategy outperforms the accuracy obtained from relying on DOM and DEM.
The remainder of this paper is organized as follows: Section 2 reviews the related work. Section 3 introduces the imager AHS-1000 and the experimental datasets. Section 4 details the proposed calibration method. Section 5 presents and discusses the results. Section 6 draws the conclusion.
2. Basic theory of algorithms
2.1. LMS calibration
LMS calibration primarily addresses internal distortions in sub-cameras and relative misalignments between sub-images resulting from boresight angles and lever-arm effects. Generally, recent research has placed greater emphasis on enhancing relative accuracy to ensure the production of seamless and accurate geo-rectified image products. Researchers have proposed two main categories of approaches: the misalignment compensation methods from image space and the misalignment calibration methods from object space. The image-space methods typically employ the shift model (Li, Liu, and Wang Citation2010), affine transformation model (Jacobsen Citation1999), or piecewise polynomial model (Meng et al. Citation2013) for image alignment and seamless stitching. However, this approach heavily relies on establishing reliable correspondences between adjacent sub-images, which is challenging when dealing with limited overlap or poorly textured regions.
The object-space misalignment calibration methods can be further classified into the classic relative orientation modeling method and the equivalent modeling method. The classic method is typically performed after completing the internal distortion calibration of sub-cameras. It focuses on rigorously calibrating the accurate angles and spatial offsets between adjacent cameras by simultaneously utilizing image-space correspondences and constraints from object space (Lichti et al. Citation2020). This method is achieved by minimizing the reprojection error of correspondences, which are projected from the original image coordinate system into the image coordinate system of reference camera based on initial relative parameters. It has been proved effective for frame multi-camera system calibration (Guo et al. Citation2021; Rameau et al. Citation2022). However, the narrow overlap design of LMS systems makes it difficult to obtain correspondences as reliably as frame cameras do, and the small baseline makes it even harder to calibrate the exact relative parameters under high flight altitude. While this method has been reported to achieve good calibration results on simulated aerial hyperspectral LMS data (Li et al. Citation2021), the challenges in obtaining reliable and evenly distributed matching points within narrow overlap areas could still introduce calibration biases.
In comparison, the equivalent modeling method aims to represent multiple imagers as a unified approximate imaging model, bypassing the need for rigorous ROP calibration between adjacent imagers. Instead, it focuses on indirectly addressing the internal distortions and relative orientation of each image based on this approximate model. Drawing upon the calibration research of spaceborne sensors with multiple linear arrays mounted on the same focal plane, such as ALOS PRISM (Kocaman and Gruen Citation2008) and ZY-3 (Jiang et al. Citation2014), some researchers constructed the approximate model based on considering the pointing angle of each pixel on a normalized plane. Jiang et al. (Citation2017) conducted calibration on dual cameras using the pointing angle model and compensated for internal distortions and misalignment using a polynomial model. Nevertheless, this study overlooked the resolution changes brought about by tilting as the angle between the two camera optical axes was only 2°.
In a recent study conducted by Cao et al. (Citation2020), which reported on the calibration of the HY-1C ultraviolet imager, they also employed the concept of equivalent modeling by representing the 5-camera system as a distorted virtual camera. However, there are significant disparities between their work and ours in terms of the calibration model and adjustment approach. Firstly, we diverge from their assumption of a shared optical center for the sub-cameras and incorporate the lever arm in our LMS modeling. Secondly, instead of adopting a two-step calibration process for the master camera and slave cameras, we integrate all sub-image GCPs into a single bundle adjustment procedure, which offers a more advantageous means of obtaining a globally optimal solution for the EOP.
2.2. Control data for sensor calibration
Control data, which is a set of GCPs, plays a crucial role in sensor calibration (Shan et al. Citation2023; Wang et al. Citation2012). Traditional control data are typically measured manually from the calibration field (Aguilar, Saldaña, and Aguilar Citation2013). However, collecting a sufficient number of GCPs to cover the entire range of an LMS, can be a time-consuming and labor-intensive task (Cheng et al. Citation2018). To overcome these limitations, other researchers propose to use internal constraints created by tie points among multiple overlapped images (Pi et al. Citation2019; Pi, Li, and Yang Citation2020; Yang et al. Citation2020). However, relying solely on tie points compromises the accuracy of the exterior calibration results and may still require sparse GCPs to achieve optimal external accuracy (Cao et al. Citation2021).
Another commonly used control data for sensor calibration is digital GCP obtained through matching with digital photogrammetric products. Many spaceborne sensor calibration researches derived digital GCPs from public geographic sources, such as the Shuttle Radar Topography Mission (SRTM) DEM or images from map service providers like Google Earth (Cao et al. Citation2022; Tao et al. Citation2014). However, the public geographic data contain local geometric deformation (Ruzgienė, Xiang, and Gečytė Citation2011), leading to inaccuracies in high-resolution airborne LMS calibration. Other studies requested large-scale and more accurate DOM and DEM from official geographic institutions, such as the National Geomatics Center of China (Jiang et al. Citation2017; Zhang et al. Citation2011; Zheng et al. Citation2015). However, height error would be introduced since many digital GCPs are typically feature points located on ground objects that may have changed or been removed in DEM. While the 0.5 m satellite DOM and the 1” Advanced Spaceborne Thermal Emission and Reflection Radiometer (ASTER) DEM have been reported to succeed in supporting calibration on simulated aerial hyperspectral LMS data (Li et al. Citation2021), the practical efficacy of this georeferencing strategy on the AHS-1000 requires validation.
Considering that the existing DOM and DEM products may be limited in terms of spatial resolution or data quality, instead of directly using these final products to extract GCPs, the original images and the intermediate block bundle adjustment results can provide more originated and precise information for georeferencing (Zhang and Tao Citation2017). proposed to use the bundle adjustment result of existing images to obtain GCPs for aerial triangulation of newly collected images. However, the application of this idea is still limited to improving automatic triangulation efficiency and has not been studied and applied to LMS calibration yet. Besides, they only evaluated the advantage of existing bundled images over DOM/DEM based on experience without performing a comparison experiment.
3. Materials
3.1. AHS-1000 imaging system
We apply the proposed calibration method to AHS-1000, a modified version of the airborne imaging spectrometer introduced by Wang et al. (Citation2016). As shown in , following the previous design, AHS-1000 comprises three subsystems designed to collect data in three spectral ranges: Visible-Near Infrared (VNIR), Short-Wave Infrared (SWIR), and Long-Wave Infrared (LWIR). Each subsystem consists of three cameras of the same spectral range, assembled using a parallel light tube optical system to ensure that their primary optical axes intersect at the same point.
The main parameters of the three types of sub-camera in AHS-1000 are shown in . The AHS-1000 is equipped with a total of 1400 spectral bands, allowing for comprehensive spectral coverage. The VNIR, SWIR, and LWIR subsystems achieve Ground Sampling Distances (GSDs) of 0.5 m, 1 m, and 1.2 m, respectively, at a flight altitude of 2000 m. Each sub-camera within the subsystems has a unique viewing angle while maintaining a consistent FOV of approximately 14.7°, forming a composite FOV of around 40°. The VNIR sub-camera utilizes Charge-Coupled Device (CCD) sensor arrays, while the SWIR and LWIR sub-cameras employ Mercury-Cadmium-Telluride Focal-Plane Arrays (MCT FPA).
Table 1. Main parameters of the three types of sub-camera of AHS-1000 (Wang et al. Citation2016).
The AHS-1000 incorporates the POS/AV 610, a system produced by Applanix, which records positions and attitudes at a rate of 100 Hz during data collection. The POS achieves horizontal and vertical positioning accuracies of approximately 1.5 m and 3 m, respectively, while demonstrating an attitude angle determination accuracy of around 0.005 deg. Given the identical assembly design of the sub-cameras in the three subsystems of AHS-1000, we have employed the proposed calibration method to calibrate each subsystem. Our specific focus was on the VNIR subsystem to showcase the effectiveness of the proposed calibration method. In the VNIR subsystem, the middle camera’s images overlap with approximately 140 pixels from the right camera and 100 pixels from the left camera. Considering that the 512 bands of the VNIR image share the same optical mechanism and the image texture should be clear for matching or measuring, we selected the 72nd band with a wavelength of 0.56 µm for the experiments.
3.2. Experimental dataset
In formulating the calibration plan for the AHS-1000, we scheduled a data collection mission in July 2020 to cover the Yushu area in Jilin Province, China, with the goal of minimizing the cost of acquiring dense GCPs. This decision was made based on the availability of an existing image dataset from Yushu that we had performed bundle adjustment in May 2020. This existing dataset was collected by another airborne mapping camera called AMS-3000 (Xi and Duan Citation2020), which is similar to the three-line-scanner ADS series (Hinsken et al. Citation2002).
shows the coverage and overview of the experimental datasets. Three distinct AHS-1000 datasets, labeled as datasets A, B, and C, are presented. Each dataset consists of six image strips. The blue rectangle in illustrates the coverage of these datasets, while provides an overview. Dataset A is designated as the calibration dataset and is used for performance comparisons with different methods. Datasets B and C serve for extension georeferencing validation of the proposed method. Datasets A and B primarily cover urban and rural areas, whereas dataset C predominantly encompasses fields and rivers. The image GSDs for datasets A, B, and C are 0.7 m, 0.7 m, and 1.0 m, respectively. For georeferencing validation experiments, we measured 28 GCPs using a Global Navigation Satellite System (GNSS) receiver in Real Time Kinematic (RTK) mode, which were called RTK GCPs. The locations of these GCPs are shown in , 16 of them are designated as circular markers and are utilized as checkpoints to evaluate the accuracy of the bundle adjustment process.
Figure 2. (a) Coverage of the experimental datasets and GCPs locations; (b) overview of the AHS-1000 datasets.

In , the green rectangle represents the coverage of the existing bundled images obtained from the AMS-3000. These images consist of four strips, each comprising three images captured in the forward, nadir, and backward directions. To enhance the accuracy of the exterior orientation parameters, 8 RTK GCPs were collected, followed by a bundle adjustment process by using 5 of the RTK GCPs as control points while the rest as checkpoints. The resulting checkpoints demonstrate horizontal and vertical accuracies of 0.06 cm and 0.14 cm, respectively, satisfying the requirements for 1:1000 scale production (Xi and Duan Citation2020).
4. Methodology
The proposed method revolves around the core concept of constructing an equivalent geometric model based on a master focal plane to perform a joint calibration of the LMS. In this method, the middle camera is designated as the master, while the other cameras serve as slaves. The linear arrays of the slave cameras are projected onto the focal plane of the master camera. By employing a post-compensation of the reprojection errors of image point observations after bundle adjustment, the internal distortion of the sub-cameras and the misalignment, coupled with resolution changes induced by the boresight angles and lever arm, are jointly calibrated as equivalent distortion on the master focal plane. The overall technical process, as illustrated in , can be divided into three key modules: 1) Level-1 sub-image generation, 2) GCP matching, and 3) Calibration based on master focal plane.
4.1. The equivalent single-camera representation
To ensure the stability of geo-referencing accuracy, an imaging system consisting of three cameras needs to follow two requirements: synchronized exposure and rigid assembly. These two requirements determine that the sub-images have the same lines and the boresight angles and spatial offsets are constant between sub-cameras. Due to the boresight angle and lever arm between adjacent cameras, ground objects in overlapping areas are observed at different times. We label the left, middle, and right cameras as 1, 2, 3, in that order, and the strict geometric model can be described by EquationEquation (1)(1)
(1) :
where is the Earth-Centered Earth-Fixed (ECEF) coordinate of a ground point;
represents the perspective center of LMS in the ECEF frame at time
;
is the rotation matrix from the camera coordinate system to the ECEF coordinate system at time
;
is the rotation matrix between the LMS and the Inertial Measurement System (IMS);
is the rotation matrix from the
-th sub-camera to the master camera,
;
is the translation vector from the
-th camera to the master camera;
is the coordinate of the ground point in focal plane frame of the
-th camera;
is the coordinate of principal point of camera
;
is the internal distortion parameters of the
-th camera;
is the focal length of camera
;
is the scale factor. The 2nd camera is set as the master camera, and the 1st and 3rd cameras serve as slave. Thus,
should be set as the identity matrix and
should be equal to 0.
The translational offsets between the LMS and the GNSS antenna, and the boresight misalignment between the axes of the IMS and the LMS are estimated during bundle adjustment (Gruen and Zhang Citation2002; Zhang et al. Citation2014). Our method aims at calibrating the internal distortions of LMS. Traditionally, to calibrate the LMS, control points on each sub-image and corresponding points serving as constrain between master and slave images are required. However, for airborne LMS like AHS-1000, the jitter of the platform and the limited overlapping area make it difficult to match adequate and accurate corresponding points. Besides, the baseline between the adjacent imagers is too small to support reliable forward intersection for getting coordinates of tie points. To avoid matching points between the master and slave cameras, an equivalent geometric model based on the master focal plane is established.
As shown in , and
are the master camera coordinate system and slave camera coordinate system, respectively. The transformation from the slave camera to the master camera is defined by
and
.
and
are the principal point of the master camera and slave camera, respectively.
and
are the focal length of the master camera and slave camera, respectively. At the exposure time
, pixel Q of the slave camera can be projected to point
in the master focal plane. Thus, the coordinate
of each projected pixel of slave camera in the focal plane of master camera can be expressed as:
Figure 4. The sketch map of the imaging geometry between the master and slave cameras: (a) overview of imaging geometry, (b) enlarged rotation relations between camera S and M.

where are the elements of rotation matrix
;
is the coordinate of a pixel in slave camera focal plane, and
.
Since the slave and master cameras are assembled using a parallel light tube optical system to ensure that their primary optical axes intersect at the same point and their X axes parallel, we only consider the roll and a small yaw angle between adjacent cameras. Thus, the rotation matrix can be expressed as EquationEquation (3)
(3)
(3) :
where and
are the roll angle and small yaw angle between the
-th camera and master camera, respectively.
For the linear-array camera, ; EquationEquation (2)
(2)
(2) can be written as EquationEquation (4)
(4)
(4) after combining EquationEquation (3)
(3)
(3) and simplification:
where ;
is the look angle of each pixel of slave camera.
As an empirical mathematical model, the look angle model is widely used in the internal parameter calibration of line array cameras (Breton et al. Citation2002; Cao et al. Citation2021; Jiang et al. Citation2014). It unifies lens distortion, principal point offset and focal length bias with high-order polynomials to avoid over-parameterization. Based on the laboratory calibration values, internal parameter of each sub-camera can be expressed as EquationEquation (5)
(5)
(5) .
where is obtained from laboratory calibration values;
is the index number of the pixel;
are the model parameters for absorbing the influence of various geometric distortion factors, including lens distortion, principal point offset and focal length bias.
EquationEquations (4)(4)
(4) and (Equation5
(5)
(5) ) establish the relationship between the internal parameters of each sub-camera and the coordinates in the master focal plane, but they are relatively complex and are not conducive to effective calibration. Considering that EquationEquation (4)
(4)
(4) is an inverse proportional function with respect to
, we use Taylor expansion principle to simplify EquationEquation (4)
(4)
(4) into a polynomial with respect to
. In the case of AHS-1000, we found that sub-pixel approximation level can be achieved by performing first-order and third-order Taylor expansions on
and
at the principal point, respectively. Thus, EquationEquation (4)
(4)
(4) can be written as:
where ,
,
,
,
and
are the coefficients after Taylor expansion, and they can describe the geometric deformation caused by misalignment between adjacent cameras;
is the look angle of principle point.
In EquationEquation (5)(5)
(5) , the order of
depends on the accuracy of laboratory calibration values, as well as the severity of radial and the decentering distortions. When
takes the 5th order, it will lead to terms up to the 15th order in EquationEquation (6)
(6)
(6) , which may lead to over-parameterization. In the case of AHS-1000, taking first-order for
is enough since the laboratory calibration values can achieve sub-pixel level. Thus, EquationEquation (6)
(6)
(6) can be further simplified as EquationEquation (7)
(7)
(7) :
where is the projected coordinate of each pixel of slave camera in the master focal plane;
,
,
,
,
and
are compensation parameters for misalignment between adjacent cameras and various geometric distortion factors of each sub-camera.
Thus, we can obtain the internal calibration terms for our equivalent geometric model, which can be expressed as EquationEquation (8)(8)
(8) :
where is the equivalent distortion of each sub-camera in the master focal plane;
,
,
,
,
and
are the calibration parameters;
is the number of sub-camera.
In EquationEquation (8)(8)
(8) ,
is the focal length of master camera, which is only used for setting a reference plane. Since the master camera is also calibrated based on EquationEquation (8)
(8)
(8) , and its focal length bias is absorbed in
,
,
,
,
and
,
will not be calibrated.
The influence of can be seen in . The projected sensor array of the sub-camera mainly suffers three system errors: 1) an offset of the projected principal point
, expressed by
; 2) a rotation angle
with respect to the y-axis in master focal plane, caused by
; 3) a resolution change along the y-axis, indicating variations in the size of the projected pixels due to
and
. To make EquationEquation (8)
(8)
(8) more concise, we partially define the calibration parameters, which is written as EquationEquation (9)
(9)
(9) :
where is the projected principal point offset of camera
in the master focal plane;
is the rotation angle of the
-th camera’s projected linear-array with respect to the y-axis in the master focal plane;
,
and
are the y-axis coordinate of the projected pixel and that of projected principal point, respectively;
and
are the deformation compensation coefficients.
It should be noted that EquationEquation (9)(9)
(9) is accurate enough for compensating deformations in AHS-1000 caused by misalignment between adjacent cameras and various geometric distortion factors, because the yaw angle
(about 0.0004 rad) and baseline (below 9 cm) are small between adjacent camera. If the laboratory calibration values are not accurate, or the assembly process is not accurate enough, higher order in EquationEquation (9)
(9)
(9) should be considered.
If the boresight angles and spatial offsets between adjacent cameras are not measured in laboratory, the initial projected coordinates of each pixel of sub-camera in the master focal plane can be obtained by only considering the roll angle and set the spatial offsets as 0. The roll angle can be estimated according to the number of overlapping pixels. As shown in , we applied a central symmetry transformation to the camera focal plane along the optical axis. The initial projected coordinate of
can be expressed as EquationEquation (10)
(10)
(10) :
where is y-coordinate of
;
is y-coordinate of the principal point of slave camera; FOV is the field of view angle of the sub-camera;
is the y-coordinate of the last overlapping pixel;
is y-coordinate of the principal point of master camera.
Based on EquationEquation (1)(1)
(1) , EquationEquation (7)
(7)
(7) and EquationEquation (9)
(9)
(9) , the equivalent geometric model is established as EquationEquation (11)
(11)
(11) :
where is the projected coordinate of image point in master focal plane;
is the equivalent distortion terms defined by EquationEquation (9)
(9)
(9) .
4.2. Geometric correspondence determination
4.2.1. Level-1 sub-image generation
As shown in , the original Level 0 sub-images exhibit significant geometric deformation, causing the ground objects in images to lose their shapes. Using these Level 0 sub-images directly for image matching would result in a low matching success ratio. To address this issue, data preprocessing is performed to generate rectified Level 1 sub-images by resampling the original sub-images onto a mean elevation plane.
During the geo-rectification process, all sub-images, including both the master and slave images, are processed using the same filtered EOP recorded by the POS. The geo-referencing of the images is performed using EquationEquation (1)(1)
(1) . As depicted in , the derived Level 1 sub-images do not exhibit the significant deformation caused by the jitter. It is important to note that the Level 1 sub-images are only used for matching corresponding points, and subsequent processes like bundle adjustment rely on the coordinates of the matching points on the master focal plane.
4.2.2. GCP matching
To estimate distortion terms at each pixel, a TLS utilizes stereo geometric constraints derived from tie points between multi-view images, often relying on a few sparsely distributed GCPs. However, in the case of the AHS-1000 VNIR subsystem, which functions as a one-line scanner, the availability of dense GCPs becomes crucial for achieving precise distortion estimation. The objective of GCP matching is to obtain dense digital control points that cover the entire FOV of the subsystem using the existing images.
A sequential matching process is used to obtain evenly distributed control points across the images. Given the average elevation, the range in the existing images corresponding to each AHS-1000 strip is first determined through forward and back-projection. As shown by the blue arrows in , the second strip of AHS-1000 images corresponds to the yellow areas in existing bundled images, and the nadir images of the TLS dataset it covers will be matched to the test image sequentially. The matched points on the nadir images are further matched with the forward and backward images and selected as GCPs. The object-space coordinates of the GCPs are calculated through space intersection using the orientation parameters of the bundled images.
A dense point matching algorithm with two steps of expansion (Zhang et al. Citation2016) is adopted to acquire dense corresponding points which can smoothly operate on linear-array images without prior information. The number of GCPs can be adjusted by setting different matching intervals. 25,357 GCPs are obtained with a 33-pixel interval on dataset A in our case. Additionally, matching tie points between strip images of AHS-1000 is crucial for establishing constraints on strip relative orientation and aiding the bundle adjustment process, as shown by the red arrows in .
4.3. Calibration based on master focal plane
After obtaining dense digital GCPs, a strip bundle adjustment is performed to refine the EOP of all scan lines and calculate the reprojection errors of the GCPs. To calibrate the equivalent distortion on the master focal plane, the image coordinates of the GCPs on the Level 1 images need to be transformed to the master focal plane as observations. This transformation process begins by mapping the points from the Level 1 images back to their corresponding pixels on the original sub-images. Subsequently, these points on the slave images are projected onto the slave focal plane and, ultimately, onto the master focal plane using EquationEquation (11)(11)
(11) . To reduce the complexity of bundle adjustment, the Lagrange Interpolation Model (Gruen and Zhang Citation2002; Hinsken et al. Citation2002), which selects certain scan lines as orientation fixes at regular time intervals, is employed and only the EOP of those orientation fixes are solved in the least squares adjustment.
Since the equivalent distortion parameters defined by EquationEquation (9)(9)
(9) are not self-calibrated during the bundle adjustment, the reprojection errors at GCP observations can provide valuable information about image distortion. By analyzing the distribution pattern of the reprojection errors, a post-compensation can be applied to fit the image distortion parameters for each sub-camera based on EquationEquation (9)
(9)
(9) .
Given that the accuracies of the matched control points may vary, we incorporate a strategy in the adjustment process to calculate the reprojection errors of each control point after each iteration and update their respective weights accordingly. This approach ensures that control points with higher accuracy have a greater influence on the adjustment results, effectively improving the overall accuracy and reliability. Based on EquationEquation (11)(11)
(11) , the distortion terms for each pixel on the master focal plane are determined by individually fitting curves to the residual errors of the GCPs from each sub-camera. To improve the accuracy of the curve fitting process, an additional step of noise point filtering can be applied, which is inspired by a density analysis method (Zhu et al. Citation2015). Our filtering approach is to divide the residual points into grids and analyze the point density in each. Noise grids have densities below a specific threshold, determined by the median of point density in each grid column, while grids exceeding this threshold are considered valid. Subsequently, we calculate the distance between the points in the noise grids and their nearest points in the valid grids. Points with distances less than half of the grid height are labeled as valid, while any remaining noise points within the noise grids are removed.
5. Results and discussion
5.1. Experimental settings
To evaluate the performance of the proposed calibration method, we conducted a comparative analysis with two methods: the classic relative orientation modeling method as utilized by Li et al. (Citation2021), and the similar equivalent modeling method described by Cao et al. (Citation2020). The classic method solves boresight angles and spatial offsets between sub-cameras during bundle adjustment, with the geometric constraint of the corresponding points between adjacent sub-images. Cao et al.’s method is performed based on the assumption that all the sub-cameras share the same optical center and a two-step calibration process for solving look-angles of each pixel of the master camera and slave cameras. For the corresponding point matching, a two-step expansion algorithm was employed (Zhang et al. Citation2016). In both the proposed method and the comparison methods, the same set of digital GCPs matched from the existing bundled images was used for calibration.
To assess the interior calibration accuracy of the calibrated images, we used geo-referencing accuracies of the final directly stitched image in the sample direction (), line direction (
), and planimetry (
) as evaluation indicators. Meanwhile, the following steps were performed for evaluating the relative orientation accuracy. Firstly, the corresponding points were matched between adjacent sub-images after rectification; secondly, using the points on the master image as references, the corresponding points on slave images were projected to the ground and subsequently reprojected onto the master image. The Root Mean Square Error (RMSE) of the reprojection errors of these corresponding points in sample direction (
), line direction (
) and planimetry (
) were calculated as the relative accuracy.
Furthermore, we compared our geo-referencing strategy using bundled images with two others. One group inspired by the strategy of Li et al. (Citation2021) utilized a 0.5 m public DOM from Google Earth with approximately 2 m geo-referencing accuracy (Goudarzi and Landry Citation2017; Pulighe, Baiocchi, and Lupia Citation2016) and the public 1” ASTER DEM with around 13 m vertical accuracy (Okolie et al. Citation2024; Tachikawa et al. Citation2011). The other group employed products of AMS-3000, including the 0.2 m DOM with 0.2 m geo-referencing accuracy and 15 m DEM with 2 m height accuracy, verified based on RTK GCPs.
Both the proposed method and the comparison methods were implemented using the C++ language. The experiments were conducted on a computer with an Intel i7 -10,750 H CPU and 32GB RAM.
5.2. Overall comparison
As shown in , 25783 GCPs are obtained from AMS-1000 bundled images. These GCPs are uniformly distributed across the primary range of dataset A, facilitating pixel-by-pixel calibration for the AHS-1000. The distribution of tie points prepared for the classic modeling method is shown in . The lengths of the six strips in dataset A all exceed 60,000 rows, and we have cropped a portion of each strip to clearly show the distribution of tie points. These tie points are distributed within the narrow overlap region of adjacent cameras, and the matching accuracy achieves sub-pixel level. The numbers of tie points in each strip image between adjacent cameras are listed in .
Figure 6. The distributions of GCPs and tie points in dataset A: (a) distribution of GCPs, (b) distribution of tie points in portions of the six strip images.

Table 2. The number of tie points between adjacent cameras in each strip image of dataset A.
5.2.1. Quantitative evaluation
The overall comparison of interior georeferencing accuracy and relative georeferencing accuracy is shown in . Cameras L, M, and R correspond to the left, middle, and right cameras, respectively.
Table 3. Comparison of interior and relative orientation accuracies.
Our equivalent modeling method exhibited superior relative accuracy when compared to the other two methods. Specifically, the relative accuracy between camera L and M in our method was 0.99 pixels, whereas the classic method achieved 2.64 pixels and Cao et al.’s method achieved 1.06 pixels. Furthermore, our method consistently achieved sub-pixel level accuracies for both camera L-M and camera R-M, while Cao et al.’s method did not reach sub-pixel level accuracy for camera L-M. The performance of the classic method varied greatly, with a planimetry relative accuracy of 0.78 pixels for camera R-M but 2.64 pixels for camera L-M. This discrepancy can be attributed to the differing number of overlapping pixels between the sub-cameras, with camera R-M having approximately 40 more overlapping pixels than camera L-M. Consequently, the classic method had approximately 1734 fewer corresponding points between camera L and M compared to the 4119 points between camera R and M in total. Furthermore, the baseline between the adjacent imagers is very small (below 9 cm). The base-height ratio can not support high-precision relative calibration which is based on the stereo constraints of tie points. It is worth noting that the relative accuracy of 0.43 pixels along the line direction of the classic method for camera R-M was the best, which was 0.01 pixels better than ours. Considering that our method was better than the classic method on relative accuracy along line direction for camera L-M by 1.21 pixels, the 0.01 pixels improvement furtherly indicates that the performance of the classic method heavily depends on the reliability of correspondences in overlapping area and is limited by the small baseline. On average, our method outperformed the classic method by 0.86 pixels and Cao et al.’s method by 0.18 pixels in relative accuracy. The higher average relative accuracy achieved by both our method and Cao et al.’s method compared to the classic method underscores the effectiveness of the equivalent modeling approach in attaining consistent calibration results for LMS among sub-cameras. This advantage is particularly notable because it does not depend on correspondences between sub-cameras and can even benefit from a small baseline, distinguishing it from the classic method. According to EquationEquation (4)(4)
(4) , the smaller the baseline is, the higher the approximate accuracy the polynomials can achieve. On the other hand, it can be seen that all the metrics of our method were better than Cao et al.’s method. This can be attributed to our one-step calibration process, which can achieve global optimal calibration result especially for aerial LMS suffering from significant platform jitter. The strong correlations between interior parameters and exterior parameters may result in different calibration result if using the two-step calibration process as utilized by Cao et al.’s method when different calibration images are employed.
Regarding interior georeferencing accuracy, our method also outperformed the classic method and Cao et al.’s method, achieving a planimetry accuracy of 0.95 pixels compared to 3.78 pixels and 2.17 pixels. Indeed, over 25,000 GCPs were matched from the existing images for bundle adjustment of the three methods. For the classic method, due to the fact that the tie points between adjacent sub-images were much less than digital GCPs, the tie points had 100 times more weight than those of the GCPs to make the constraint between sub-images works. However, the uneven distribution of tie points along the sample or line direction, coupled with the challenge of determining the precise object-space coordinates due to the small baseline, resulted in a suboptimal relative calibration outcome. Differently, our proposed method and Cao et al.’s method mitigated the dependence on tie points in the overlapping area. The 1.22-pixel improvement in interior accuracy achieved by our method compared to Cao et al.’s method demonstrates that our approach effectively addressed the misalignment caused by boresight angles and spatial offsets. Therefore, our master focal plane equivalent model and one-step calibration process have proven effective in obtaining more precise and reliable calibration results.
5.2.2. Visual alignment performance in overlapping areas
To further illustrate the effectiveness of our method in improving relative accuracy, we visually compared and analyzed the alignment performance in overlapping areas of the rectified sub-images produced by the three methods. We performed the rectification based on the three methods with the same strip image and superimposed the left and right sub-images onto the middle sub-image, as shown in . For ease of comparison, the middle images of the three methods are displayed in the red channel while the left and right images are displayed in green. Four blocks of the partially enlarged images, denoted as areas 1, 2, 3, and 4, are shown in . Among the four areas, areas 2 and 4 lie in the overlapping area of camera R and M, while areas 1 and 3 lie in that of camera L and M.
Figure 7. Visual alignment performance comparison in overlapping areas of different methods: (a) overview of the test strip image and location of comparison blocks, (b) enlarged comparison blocks.

In , it is evident that the classic method exhibits an offset of around 3 pixels along the line direction in areas 1 and 3, which is more significant than the approximately 1-pixel offset observed in areas 2 and 4. This suggests that the ROP solved between camera L and M had lower accuracy compared to those between camera R and M. As previously discussed in Section 5.2.1, the narrower overlap between camera L and M and the small baseline resulted in poorer relative calibration results. The performance of Cao et al.’s method is better than the classic method in areas 1 and 3 but slightly worse in areas 2 and 4. These observations indicate that Cao et al.’s method reduces the influence of narrow overlapping areas, but its achievable accuracy is limited due to the two-step calibration process and the assumption of a shared optical center.
For enlarged block images of ours, the visual performances in all four areas were better than that of the comparison methods; the offset was barely visible to the naked eye. The misalignment between the sub-cameras was effectively reduced, which indicated that the proposed method succeeded in compensating for errors caused by the boresight angles and spatial offsets between adjacent cameras. Furthermore, our method outperformed Cao et al.‘s method in mitigating misalignment in areas 2, 3 and 4, underscoring the advantages of our one-step calibration process and stringent equivalent assumption. Additionally, our method demonstrated consistent performance across cameras R, L, and M, showing resilience to narrow overlaps.
5.3. Advantage of using bundled images for geometric control
depict a comparison of residual error distributions at GCP observations obtained from public DOM/DEM, AMS-3000 DOM/DEM and bundled images before and after calibration. To facilitate comparison, the residual errors before and after the calibration process are represented by red and blue colors, respectively.
Figure 8. Residual error distributions at GCP observations obtained from public DOM/DEM (top row), AMS-3000 DOM/DEM (middle row), and bundled images (bottom row) before calibration. Residual errors of using public DOM/DEM along sample direction (a) and line direction (b). Residual errors of using AMS-3000 DOM/DEM along sample direction (c) and line direction (d). Residual errors of using bundled images along sample direction (e) and line direction (f).

Figure 9. Residual error distributions at GCP observations obtained from public DOM/ASTER DEM (top row), AMS-3000 DOM/DEM (middle row), and bundled images (bottom row) after calibration. Residual errors of using public DOM/DEM along sample direction (a) and line direction (b). Residual errors of using AMS-3000 DOM/DEM along sample direction (c) and line direction (d). Residual errors of using bundled images along sample direction (e) and line direction (f).

In , the x-axis is the sample coordinate of the projecting sensor array on the master focal plane, while the y-axis represents the residual errors in sample or line directions after the bundle adjustment. To ensure clear visibility of trends and prevent the blending of residual errors in the overlapping area, pixels from both the master camera and the slave cameras are equally sampled in the overlapping region. Regarding the distribution of residual errors at GCP observations of the DOM/DEM and bundled images before calibration, depicted in , a polynomial distorted pattern in the sample direction and a linear distortion pattern in the line direction are observed. These patterns can be divided into three segments corresponding to the left, middle, and right cameras, resulting from the inaccurate initial projection coordinates of pixels on the master focal plane.
Comparing the residual error distributions before and after calibration, our bundle images group and the AMS-3000 DOM/DEM group show significant advantages over the public DOM/DEM group. Firstly, our bundle images group and the AMS-3000 DOM/DEM group demonstrate a higher concentration of residual errors near the piecewise curves, whereas the public DOM/DEM group falls short in this aspect. Secondly, they avoid the distorted curve pattern observed in the public DOM/DEM-based method after calibration. The discrete distribution pattern of the public DOM/DEM leads to inaccurate curve fitting parameters, resulting in poor calibration accuracy using the post-compensation method. Although the misalignment error between sub-cameras is reduced in public DOM/DEM group, as shown in ), the bending trend of the distribution at the ends indicates that the scanners at the ends of the linear-array are not accurately calibrated. This discrepancy arises from calculating the coordinates of GCPs in the presence of non-negligible elevation errors, which means the 0.5 m satellite DOM and the 1” ASTER DEM are not accurate enough for AHS-1000 calibration.
As shown in ), distortions are generally eliminated after calibration using the AMS-3000 DOM/DEM, indicating that higher accuracy products can effectively improve the calibration result. While our bundled images group shows an advantage in concentrating residual errors near piecewise curves over the AMS-3000 DOM/DEM, this advantage is less pronounced compared to the public DOM/DEM, as depicted in ). Indeed, the RMSE of GCPs after calibration in our bundled images group is 0.47 m, outperforming the 0.55 m RMSE of the AMS-3000 products group and the 1.02 m RMSE of the public DOM/DEM group. The impact of the 2 m vertical error in AMS-3000 DEM is not as significant as expected, likely due to the relatively flat terrain of dataset A, where elevations range from 160.31 m to 243.23 m, with a mean elevation of 197.68 m. However, the absence of readily available suitable DOM and DEM in the database for camera calibration may necessitate new production tasks, thereby hindering efficiency and increasing costs. Given that each project saves the bundle adjustment results, utilizing bundled images for geo-referencing stands out for ensuring the highest calibration accuracy while also improving efficiency.
5.4. Georeferencing accuracy validation
To further assess the stability and accuracy of the calibration result, experiments were conducted with and without the use of RTK GCPs, as shown in , on datasets B and C. When all the RTK GCPs were utilized as checkpoints, the reprojection errors were computed. In cases where several RTK GCPs were utilized as control points while the others were used as checkpoints, bundle adjustment was performed and the reprojection errors of the checkpoints were subsequently calculated based on the refined EOP. Both experiments were based on the calibration result obtained from dataset A. The RMSEs and the standard deviations of the checkpoints after calibration are listed in . For ease of comparison, the results of the bundle adjustment without calibration are also listed in .
Table 4. The georeferencing accuracy for bundle adjustment of AHS-1000 VNIR subsystem before and after calibration.
As shown in , the use of sparse RTK GCPs for bundled adjustment in groups 2 and 4 resulted in higher accuracy compared to groups 1 and 3 without RTK GCPs, as expected. It is worth noting that the RMSE along the line direction in group 1 was significantly larger (over 20 pixels) than those in group 3 (less than 10 pixels), which can be attributed to potentially unfavorable flight conditions during data acquisition. Besides, the RMSE along the sample direction in groups 2 and 4 exhibited more significant improvement compared to the improvement along the line direction, respectively. This improvement difference can be attributed to the adjustment of the EOP, which effectively compensated for errors in the line direction. visually demonstrates the successful compensation of relative errors along the line direction through our proposed calibration method. Moreover, there was an improvement in standard deviation after calibration, indicating enhanced convergence during bundle adjustment.
Analyzing the bundle adjustment results with sparse RTK GCPs, the experiment in group 2 exhibited a notable improvement in RMSE along the sample direction from 1.52 pixels to 0.66 pixels after calibration, while group 4 showed improvement from 1.20 pixels to 0.68 pixels along the sample direction. These results provide evidence that our proposed method effectively calibrated the equivalent distortion terms, leading to improved accuracy.
6. Conclusions
In this paper, an equivalent geometric calibration method based on the master focal plane for the airborne LMS was proposed. We first projected the sensor array of slave cameras to the master focal plane. Then, original sub-images were rectified into Level 1 sub-images for correcting geometric deformation, which were used to match with existing bundled images to obtain corresponding points that cover the whole FOV of LMS. The ground coordinates of these points were calculated with the accurate orientation parameters of existing bundled images and used as control points in strip bundle adjustment. Finally, the equivalent distortions on the master focal plane were calibrated using a post-compensation method to fit the reprojection errors of GCPs. In the experiment, the VNIR sub-system of AHS-1000, which consisted of three independent cameras, was calibrated using existing bundled images of another airborne stereo mapping camera AMS-3000. The results demonstrate that our equivalent modeling method surpasses the classic method by 2.83 pixels in interior accuracy and 0.86 pixels in relative accuracy, and outperforms another similar equivalent modeling method by 1.22 pixels in interior accuracy and 0.18 pixels in relative accuracy. Besides, the correspondence offset along the line direction in narrow overlapping areas of the proposed method was reduced to the sub-pixel level, which was better than the comparison methods with visible offset. The comparison experiment between the georeferencing strategy using existing bundled images and DOM/DEM from public data sources or aerial TLS indicated that dense digital GCPs from existing bundled images can provide more accurate calibration result. The georeferencing accuracy validation experiment further revealed that the proposed calibration method can improve georeferencing accuracy along the sample direction from 1.52 pixels to 0.66 pixels.
Disclosure statement
No potential conflict of interest was reported by the author(s).
Data availability statement
The experimental dataset is constrained by a confidentiality agreement, preventing the public disclosure of the dataset at this stage.
Additional information
Funding
Notes on contributors
Hao Zhang
Hao Zhang received the B.E. degree from Wuhan University, Wuhan, China, in 2018. He is currently pursuing the PhD degree with the Wuhan University, China. His research interests include simultaneous localization and mapping, photogrammetry and machine learning.
Yansong Duan
Yansong Duan received the PhD degree from Wuhan University, Wuhan, China, in 2016. He is currently an associate professor with the School of Remote Sensing and Information Engineering, Wuhan University. He works in the fields of digital photogrammetry, multi-view images 3D reconstruction and machine learning.
Qi Zhou
Qi Zhou received the B.E. degree from Wuhan University, Wuhan, China, in 2021. She is currently a master at Wuhan University, China. Her research interests include image matching, remote sensing and photogrammetry.
Qi Chen
Qi Chen received the PhD degrees from Wuhan University, Wuhan, China, in 2015. He is currently an associate professor. His research interests include space and aerial photogrammetry and pattern recognition from remote sensing imagery with deep-learning techniques.
Bowen Cai
Bowen Cai received the B.E. degree in remote sensing science and technology from Wuhan University in 2018. He is currently pursuing his PhD degree with the School of Remote Sensing and Information Engineering, Wuhan University. His research interests include urban remote sensing, social ecology and remote sensing applications.
Pengjie Tao
Pengjie Tao is currently an associate professor. His research interests include satellite photogrammetry, registration of optical images and LiDAR points, and multi-view images 3D reconstruction.
Zuxun Zhang
Zuxun Zhang is a professor and major in digital photogrammetry and remote sensing. He was selected as a member of International Academy of Sciences for Europe and Asia in 1995 and a member of Chinese Academy of Engineering in 2003. He has led a number of national and ministerial major research projects and has achieved substantial outcomes in aviation image mapping automation.
References
- Aguilar, M. A., M. D. M. Saldaña, and F. J. Aguilar. 2013. “Assessing Geometric Accuracy of the Orthorectification Process from GeoEye-1 and WorldView-2 Panchromatic Images.” International Journal of Applied Earth Observation and Geoinformation 21 (April): 427–435. https://doi.org/10.1016/j.jag.2012.06.004.
- Breton, E., A. Bouillon, R. Gachet, and F. Delussy. 2002. “Pre-Flight and In-Flight Geometric Calibration of SPOT5 HRG and HRS Images.” The International Archives of Photogrammetry, Remote Sensing & Spatial Information Sciences 34 (1): 20–25. https://doi.org/10.1117/12.462637.
- Cao, J., H. Shang, N. Zhou, and S. Xu. 2022. “In-Orbit Geometric Calibration of Multi-Linear Array Optical Remote Sensing Satellites with Tie Constraints.” Optics Express 30 (15): 28091. https://doi.org/10.1364/OE.464112.
- Cao, J., F. Wang, Y. Zhou, and Z. Ye. 2021. “In-Orbit Geometric Calibration of HaiYang-1C Coastal Zone Imager with Multiple Fields.” Optics Express 29 (12): 18950. https://doi.org/10.1364/OE.427023.
- Cao, J., Z. Zhang, S. Jin, and X. Chang. 2020. “Geometric Stitching of a HaiYang-1C Ultra Violet Imager with a Distorted Virtual Camera.” Optics Express 28 (9): 14109. https://doi.org/10.1364/OE.391418.
- Cheng, Y., M. Wang, S. Jin, L. He, and Y. Tian. 2018. “New On-Orbit Geometric Interior Parameters Self-Calibration Approach Based on Three-View Stereoscopic Images from High-Resolution Multi-TDI-CCD Optical Satellites.” Optics Express 26 (6): 7475. https://doi.org/10.1364/OE.26.007475.
- Chen, Y., Z. Xie, Z. Qiu, Q. Zhang, and Z. Hu. 2015. “Calibration and Validation of ZY-3 Optical Sensors.” IEEE Transactions on Geoscience and Remote Sensing 53 (8): 4616–4626. https://doi.org/10.1109/TGRS.2015.2403957.
- Goudarzi, M. A., and R. Landry Jr. 2017. “Assessing Horizontal Positional Accuracy of Google Earth Imagery in the City of Montreal, Canada.” Geodesy and Cartography 43 (2): 56–65. https://doi.org/10.3846/20296991.2017.1330767.
- Gruen, A., and L. Zhang. 2002. “Sensor Modeling for Aerial Mobile Mapping with Three-Line-Scanner (TLS) Imagery.” The International Archives of Photogrammetry, Remote Sensing and Spatial Information Sciences 34 (2): 139–146.
- Guo, M., Y. Zhou, J. Zhao, T. Zhou, B. Yan, and X. Huang. 2021. “Urban Geospatial Information Acquisition Mobile Mapping System Based on Close-Range Photogrammetry and IGS Site Calibration.” Geo-Spatial Information Science 24 (4): 558–579. https://doi.org/10.1080/10095020.2021.1924084.
- Hartley, R. I. 1997. “In Defense of the Eight-Point Algorithm.” IEEE Transactions on Pattern Analysis and Machine Intelligence 19 (6): 580–593. https://doi.org/10.1109/34.601246.
- Hinsken, L., S. Miller, U. Tempelmann, R. Uebbing, and A. S. Walker. 2002. “Triangulation of LH Systems ADS40 Imagery Using Orima GPS/IMU.” The International Archives of Photogrammetry, Remote Sensing and Spatial Information Sciences 34 (3/A): 156–162.
- Hu, P., Q. Lu, R. Shu, and J. Wang. 2005. “An Airborne Pushbroom Hyperspectral Imager with Wide Field of View.” Chinese Optics Letters 3 (12): 689–691.
- Jacobsen, K. 1999. “Geometric and Information Potential of IRS-1C PAN-Images.” Paper presented at the IEEE 1999 International Geoscience and Remote Sensing Symposium 1:428–430, Hamburg, Germany, June 28 - July 2.
- Jiang, Y., K. Xu, R. Zhao, G. Zhang, K. Cheng, and P. Zhou. 2017. “Stitching Images of Dual-Cameras Onboard Satellite.” Journal of Photogrammetry and Remote Sensing 128 (June): 274–286. https://doi.org/10.1016/j.isprsjprs.2017.03.018.
- Jiang, Y., G. Zhang, X. Tang, D. Li, W. Huang, and H. Pan. 2014. “Geometric Calibration and Accuracy Assessment of ZiYuan-3 Multispectral Images.” IEEE Transactions on Geoscience and Remote Sensing 52 (7): 4161–4172. https://doi.org/10.1109/TGRS.2013.2280134.
- Jia, J., Y. Wang, J. Chen, R. Guo, R. Shu, and J. Wang. 2020. “Status and Application of Advanced Airborne Hyperspectral Imaging Technology: A Review.” Infrared Physics and Technology 104 (January): 103115. https://doi.org/10.1016/j.infrared.2019.103115.
- Kocaman, S., and A. Gruen. 2008. “Orientation and Self‐Calibration of ALOS PRISM Imagery.” The Photogrammetric Record 23 (123): 323–340. https://doi.org/10.1111/j.1477-9730.2008.00493.x.
- Lenz, A., H. Schilling, D. Perpeet, S. Wuttke, W. Gross, and W. Middelmann. 2014. “Automatic In-Flight Boresight Calibration Considering Topography for Hyperspectral Pushbroom Sensors.” Paper presented at the 2014 IEEE Geoscience and Remote Sensing Symposium, 2981–2984, Quebec City, July 13-18.
- Leprince, S., P. Muse, and J.-P. Avouac. 2008. “In-Flight CCD Distortion Calibration for Pushbroom Satellites Based on Subpixel Correlation.” IEEE Transactions on Geoscience and Remote Sensing 46 (9): 2675–2683. https://doi.org/10.1109/TGRS.2008.918649.
- Lichti, D. D., D. Jarron, W. Tredoux, M. Shahbazi, and R. Radovanovic. 2020. “Geometric Modelling and Calibration of a Spherical Camera Imaging System.” The Photogrammetric Record 35 (170): 123–142. https://doi.org/10.1111/phor.12315.
- Li, S., T. Liu, and H. Wang. 2010. “Image Mosaic for TDICCD Push-Broom Camera Image Based on Image Matching.” Remote Sensing Technology and Application 24 (3): 374–378. https://doi.org/10.11873/j.issn.1004-0323.2009.3.374.
- Li, J., L. Ma, Y. Fan, N. Wang, K. Duan, Q. Han, X. Zhang, G. Su, C. Li, and L. Tang. 2021. “An Image Stitching Method for Airborne Wide-Swath HyperSpectral Imaging System Equipped with Multiple Imagers.” Remote Sensing 13 (5): 1001. https://doi.org/10.3390/rs13051001.
- Meng, W., S. Zhu, B. Zhu, and S. Bian. 2013. “The Research of TDI-CCDs Imagery Stitching Using Information Mending Algorithm.” In Proceedings Volume 8908, International Symposium on Photoelectronic Detection and Imaging 2013: Imaging Sensors and Applications, edited by J. Ohta, N. Wu, and B. Li, 342–351. Washington: SPIE.
- Nister, D. 2004. “An Efficient Solution to the Five-Point Relative Pose Problem.” IEEE Transactions on Pattern Analysis and Machine Intelligence 26 (6): 756–770. https://doi.org/10.1109/TPAMI.2004.17.
- Okolie, C. J., J. P. Mills, A. K. Adeleke, J. L. Smit, M. V. Peppa, A. O. Altunel, and I. D. Arungwa. 2024. “Assessment of the Global Copernicus, NASADEM, ASTER and AW3D Digital Elevation Models in Central and Southern Africa.” Geo-Spatial Information Science February: 1–29. https://doi.org/10.1080/10095020.2023.2296010.
- Pi, Y., X. Li, and B. Yang. 2020. “Global Iterative Geometric Calibration of a Linear Optical Satellite Based on Sparse GCPs.” IEEE Transactions on Geoscience and Remote Sensing 58 (1): 436–446. https://doi.org/10.1109/TGRS.2019.2936891.
- Pi, Y., B. Yang, X. Li, and M. Wang. 2019. “Study of Full-Link On-Orbit Geometric Calibration Using Multi-Attitude Imaging with Linear Agile Optical Satellite.” Optics Express 27 (2): 980. https://doi.org/10.1364/OE.27.000980.
- Pulighe, G., V. Baiocchi, and F. Lupia. 2016. “Horizontal Accuracy Assessment of Very High Resolution Google Earth Images in the City of Rome, Italy.” International Journal of Digital Earth 9 (4): 342–362. https://doi.org/10.1080/17538947.2015.1031716.
- Rameau, F., J. Park, O. Bailo, and I. S. Kweon. 2022. “MC-Calib: A Generic and Robust Calibration Toolbox for Multi-Camera Systems.” Computer Vision and Image Understanding 217 (March): 103353. https://doi.org/10.1016/j.cviu.2021.103353.
- Ruzgienė, B., X. Q. Xiang, and S. Gečytė. 2011. “Rectification of Satellite Imagery from Google Earth: Application for Large Scale City Mapping.” Proceedings of the The 8th International Conference on Environmental Engineering, 3:1451–1454. Vilnius, Lithuania: Vilnius Gediminas Technical University.
- Shan, J., Z. Li, D. Lercel, K. Tissue, J. Hupy, and J. Carpenter. 2023. “Democratizing Photogrammetry: An Accuracy Perspective.” Geo-Spatial Information Science 26 (2): 175–188. https://doi.org/10.1080/10095020.2023.2178336.
- Tachikawa, T., M. Hato, M. Kaku, and A. Iwasaki. 2011. “Characteristics of ASTER GDEM Version 2.” Paper presented at the 2011 IEEE International Geoscience and Remote Sensing Symposium. Vancouver, July 24-29.
- Tao, P., L. Lu, Y. Zhang, B. Xu, and S. Zou. 2014. “On-Orbit Geometric Calibration of the Panchromatic/Multispectral Camera of the ZY-1 02C Satellite Based on Public Geographic Data.” Photogrammetric Engineering and Remote Sensing 80 (6): 505–517. https://doi.org/10.14358/PERS.80.6.505-517.
- Wang, M., Y. Cheng, X. Chang, S. Jin, and Y. Zhu. 2017. “On-Orbit Geometric Calibration and Geometric Quality Assessment for the High-Resolution Geostationary Optical Satellite GaoFen4.” ISPRS Journal of Photogrammetry and Remote Sensing 125: 63–77. https://doi.org/10.1016/j.isprsjprs.2017.01.004. March.
- Wang, J., Y. Ge, G. B. M. Heuvelink, C. Zhou, and D. Brus. 2012. “Effect of the Sampling Design of Ground Control Points on the Geometric Correction of Remotely Sensed Imagery.” International Journal of Applied Earth Observation and Geoinformation 18 (August): 91–100. https://doi.org/10.1016/j.jag.2012.01.001.
- Wang, Y., L. Wei, L. Yuan, C. Li, G. Lv, F. Xie, G. Han, R. Shu, and J. Wang. 2016. “New Generation VNIR/SWIR/TIR Airborne Imaging Spectrometer.” Proceedings Volume 10156, Hyperspectral Remote Sensing Applications and Environmental Monitoring and Safety Testing Technology, edited by Y. Wang, L. Wei, L. Yuan, C. Li, G. Lv, F. Xie, G. Han, R. Shu, and J. Wang. Washington: SPIE.
- Xi, K., and Y. Duan. 2020. “AMS-3000 Large Field View Aerial Mapping System: Basic Principles and the Workflow.” International Archives of the Photogrammetry, Remote Sensing and Spatial Information Sciences XLIII-B1-2020 (August): 79–84. https://doi.org/10.5194/isprs-archives-XLIII-B1-2020-79-2020.
- Yang, B., Y. Pi, X. Li, and Y. Yang. 2020. “Integrated Geometric Self-Calibration of Stereo Cameras Onboard the ZiYuan-3 Satellite.” Journal of Photogrammetry and Remote Sensing 162 (April): 173–183. https://doi.org/10.1016/j.isprsjprs.2020.02.015.
- Zhang, Z., J. He, S. Huang, and Y. Duan. 2016. “Dense Image Matching with Two Steps of Expansion.” ISPRS - International Archives of the Photogrammetry Remote Sensing and Spatial Information Sciences XLI-B3 (June): 143–149. https://doi.org/10.5194/isprsarchives-XLI-B3-143-2016.
- Zhang, Z., L. Lu, P. Tao, Y. Zhang, and Y. Zhang. 2011. “Registration of CBERS-02B Satellite Imagery in Quick GIS Updating.” In MIPPR 2011: Remote Sensing Image Processing, Geographic Information Systems, and Other Applications, edited by J. Liu, J. Tian, H. Sang, and J. Ma, 83–90. Guilin, China: SPIE. https://doi.org/10.1117/12.901652.
- Zhang, Z., and P. Tao. 2017. “An Overview on ‘Cloud Control’ Photogrammetry in Big Data Era.” Acta Geodaetica et Cartographica Sinica 46 (10): 1238. https://doi.org/10.11947/j.AGCS.2017.20170337.
- Zhang, D., L. Yuan, S. Wang, H. Yu, C. Zhang, D. He, G. Han, J. Wang, and Y. Wang. 2019. “Wide Swath and High Resolution Airborne HyperSpectral Imaging System and Flight Validation.” Sensors 19 (7): 1667. https://doi.org/10.3390/s19071667.
- Zhang, Y., M. Zheng, J. Xiong, Y. Lu, and X. Xiong. 2014. “On-Orbit Geometric Calibration of ZY-3 Three-Line Array Imagery with Multistrip Data Sets.” IEEE Transactions on Geoscience and Remote Sensing 52 (1): 224–234. https://doi.org/10.1109/TGRS.2013.2237781.
- Zheng, M., Y. Zhang, J. Zhu, and X. Xiong. 2015. “Self-Calibration Adjustment of CBERS-02B Long-Strip Imagery.” IEEE Transactions on Geoscience and Remote Sensing 53 (7): 3847–3854. https://doi.org/10.1109/TGRS.2014.2385706.
- Zhu, J., X. Hu, Z. Zhang, and X. Xiong. 2015. “Hierarchical Outlier Detection for Point Cloud Data Using a Density Analysis Method.” Acta Geodaetica et Cartographica Sinica 44 (3): 282. https://doi.org/10.11947/J.AGCS.2015.20130423.