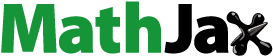
Abstract
The insinuations of the ailments associated with the unrestrained and disorganized proliferation of artificial impervious materials over natural surfaces are prevalent among city dwellers. These impacts can be comprehended by estimating land surface temperature (LST), as it is vital for evaluating urban climate, particularly to explain the intensity of urban heat islands and to define the health and welfare of the planet as well as the living beings. Urbanization-driven landscape changes severely disrupt comfortable living in almost every city, necessitating monitoring and modelling historical, current, and likely future LSTs. This research article proposes two forecasting techniques: Multiple Linear Regression (MLR) and Artificial Neural Network (ANN) models. These models have been widely accepted for the efficient prediction of climatic parameters, including LST, over an urban area. The landscape, elevation, and LST trend served as input to the models for an accurate prediction of LST. The analysis was performed over the Kolkata Metropolitan Area (KMA) with an additional 10 km buffer to understand urban growth and its effect on the LST of the entire region. The two developed models (MLR and ANN) effectively anticipated the LST over the KMA region. A continual increment in the surface temperatures ranging from 1 °C to 4 °C, over existing and likely-predicted urban areas was comprehended. It was anticipated that the regions near the urban areas will also experience severe discomfort and heat waves without proper mitigation measures. This scientific literature provides essential insights for decision-makers, stakeholders, and government officials to articulate new policies and modify the existing ones to create a sustainable and livable urban environment for the inhabitants.
Introduction
The earth at present is experiencing a significant change in urban population that directly affects urban construction rates, significantly altering landscapes, influencing the region’s micro-climate and exacerbating global warming. Cook et al. (Citation2016) identified anthropogenic activities as the primary factor responsible for the earth’s warming at local, regional, and global levels. The past three decades have perceived significant alterations in urban patterns and climate due to large anthropogenic interventions. By 2014, 54% of the global population resided in urban areas (Chen et al. Citation2014), with developing nations expecting further urbanization, adding 2.5 billion residents by 2050 (UN DESA Citation2018, Citation2019). This rapid urbanization is driven by improved living standards, education access, job opportunities, easier commuting, and better healthcare. However, this influx strains resources, leading to housing shortages, water scarcity, insufficient infrastructure, and increased pollution, impacting overall quality of life (Ramachandra et al. Citation2012). Unplanned urban expansion has caused the Earth’s temperature to rise by 0.8–1 °C, leading to serious consequences such as ocean acidification, melting ice, sea-level rise, and intensified natural disasters (NASA Citation2023). In places where urban growth is haphazard, especially in developing nations, these issues are compounded, posing challenges in optimal land allocation, which leads to the expansion of urban areas beyond their boundaries. Moreover, unplanned urban development leads to thermal discomfort, water scarcity, and pollution, further exacerbating rising land surface temperatures.
Land Surface Temperature (LST), the radiative skin temperature of the earth’s surface, serves as one of the most important parameters in the land surface processes at local, regional and global levels (Li et al. Citation2013). One of the biggest problems faced today, is a continual rise in LSTs. The Intergovernmental Panel on Climate Change (IPCC) anticipated a potential increase in global LSTs by 1.4–5.8 °C by 2100, with atmospheric carbon dioxide levels anticipated to double from pre-industrial levels (Singh et al. Citation2017; IPCC Citation2021; Kafy et al. Citation2021). Elevated temperatures affect sectors vital for human life, including energy, agriculture, forest, ecosystem biodiversity, air quality, infrastructure, marine life, healthcare and water resources (Nimish et al. Citation2018). Moreover, alterations in LST severely affect the naturally occurring atmospheric cycles, heat exchanges, and micro, meso, and macro-climatic conditions, modifying rainfall and wind patterns (Bharath et al. Citation2013; Jin et al. Citation2015). Incessant rise in LST and elevated urban temperatures result in Urban Heat Islands (UHIs), disrupting energy flow and stressing urban flora and fauna (Mathew et al. Citation2016; Yang et al. Citation2016). UHIs increase emissions, intensifying heat waves, disrupting work hours, and affecting urban residents’ quality of life, leading to power outages, deteriorated air quality, health issues, increased levels of thermal discomfort, food and water shortages, and rise in extreme events, contributing to increased mortality rates (Founda and Santamouris Citation2017; Nandi and Swain Citation2022). These changes create uncomfortable urban environments, causing socio-environmental issues and impacting global productivity.
Keeping the above facts and scientific studies intact, monitoring and assessing LST plays a vital role in defining the livability and sustainability of an urban area. The forecasted LST can significantly mitigate the urban heat island effect by developing sustainable policies and aiding in new innovative strategies for urban planning (Tran et al. Citation2017). LST, being one of the primary indicators of several natural phenomena, such as climate, energy balance, the greenhouse effect, the health of the region’s ecology, and atmospheric conditions, demands precise forecasting. It is crucial for managing environmental and earth resources (Bisht & Dodamani, Citation2016). In the realm of climate research, understanding and anticipating LST have posed both challenges and intellectual stimulation. Future LST simulation is vital in mitigating the environment’s negative impacts due to increasing urban growth. It is a valuable parameter for understanding and visualizing the areas requiring priority attention. It can assist in developing adequate policies and frameworks to limit or offset the terrible effects of increasing LST on urban residents. The research intends to address two out of the 17 sustainable development goals of the United Nations (UN), which are: (i) making cities and human settlements inclusive, safe, resilient, and sustainable; and (ii) taking immediate actions to combat climate change and its consequences. In addition, the climate action plan 2021–2025, outlined by the World Bank focuses on integrating climate change with upcoming developments, and aims to identify and prioritize mitigation and adaptive initiatives. This necessitates monitoring, visualisation, and prediction of future LST to execute cooling action plans effectively. This research proposes algorithms that can accurately predict the LST of an urban area considering the landscape’s composition and the surface temperature trend. This scientific literature would serve as vital information for decision-makers, stakeholders, and government officials to articulate new policies and modify the existing ones to create a sustainable and livable urban environment for the inhabitants. The communication is divided into the following sections: introduction, review of literature, study area, methodological framework, prediction of landscape, development of models and testing, results, discussion, and conclusive remarks.
Review of literature
Urbanization can be demarcated as the lateral and vertical growth of the urban area due to increased industrialization, demographic pressure and people migrating from rural areas to urban areas in pursuit of better opportunities and improved living standards. It is highly influenced by various socioeconomic, political and cultural factors (Bharath et al. Citation2014). Urbanization of an area can be understood by developing its land use and land cover (LULC), which plays a vital role in defining the terrestrial climate and environment in anthropogenic and natural aspects. Modifications in LULC of an urban area contribute to micro, meso and macro climatic changes and are responsible for several environmental implications (Mahmood et al. Citation2010).
Rehman et al. (Citation2022) comprehended the contribution of LULC changes on LST in the upper Indus basin of Pakistan to identify a 34.26% rise in the LST, which would further increase by 30.19% in future. A similar study performed by Lu et al. (Citation2021) inferred that such research work innovatively advances the understanding of the impact of modifications in the landscape of an urban area on its thermal environment and can efficiently aid in formulating regulatory measures at the planning stage. Imran et al. (Citation2021) observed a considerable increase in thermal discomfort among the residents owing to an increase in built-up and barren areas across Dhaka city in Bangladesh. Tan et al. (Citation2020) investigated modifications in the climate-sensitive and ecologically fragile Dongting lake area in China and found a 3.5 °C rise in LST owing to a reduction in the area associated with the water bodies. Additionally, the authors inferred a negative correlation of LST with spatial indices about water bodies (NDMI) and vegetation (NDVI). Mutiibwa et al. (Citation2014) studied the impact of LULC changes on the regional climate of the United States’ high plains, concluding that they were the primary contributors to regional climate change. Aggarwal et al. (Citation2012) assessed the effects of landscape changes on an urban settlement’s hydrological regime. They discovered that converting natural surfaces to impervious surfaces reduces evapotranspiration rates and increases runoff, resulting in a shortage of surface and sub-surface water tables.
All these studies and other scientific literature necessitate a continual visualization and monitoring of the landscape and LST. However, these kinds of visualizing and monitoring analyses provide information about the present and past, but for development. However, for sustainable policies and aiding new innovative strategies for urban design and planning, it is crucial to understand the probable future scenario, which makes modelling, simulation, prediction and forecasting imperative (Tran et al. Citation2017). This research can assist with environmental and earth resource management (Bisht & Dodamani, Citation2016) while providing residents with a comfortable and sustainable urban environment by implementing various passive cooling measures and adopting region-specific cooling action plans.
An analysis performed by Tian et al. (Citation2023) to predict LST considering future land use change for Nanjing city, comprehended a continual rise in LST, with mean LST being 23.44 °C in 1990 to 25.40 °C in 2020. Further, the study estimated a mean LST of 26.73 °C by 2023, considering future land use changes. Another study performed by Mansourmoghaddam et al. (Citation2023) signified a 67.5% (7.7 °C) rise in mean LST, attributed to a significant land use change, including a 38.5% (8.2 km2) rise in urban areas and 14.7% (3.8 km2) rise in barren lands. Kafy et al. (Citation2021), predicted LULC changes along with LST using a cellular-automata-based ANN algorithm for Dhaka Metropolitan area, anticipating a 13% increase in summer and a 20% increase in winter LST from 2020 to 2030. Khalil et al. (Citation2021) tested several models for predicting future LST and inferred that the convolution neural network outperformed others for their study area (Lahore, Pakistan). In related studies, Jia et al. (Citation2021) employed ANN to simulate future LST maps in Beijing, and Ranjan et al. (Citation2018) utilized ANN to predict LST in the Indian city of Jodhpur.
It could be inferred that NNMs can outperform all the traditionally used methods, including simple regression, time series models (ARIMA, SARIMA), the Box-Jenkins method, and many more, as NNM can capture all the sudden and abnormal changes (Huang et al. Citation2014). Unlike the traditional algorithms used for predicting land covers, NNMs do not assume the data to be linear and stationery (Atkinson and Tatnall Citation1997) and are capable of solving highly complex problems (Foody Citation2006; Mas and Flores Citation2008), thus, providing a higher accuracy in predictions. The type of NNM used for the study is the (ANN) model, which works on the same principle as a human brain (Shabri and Samsudin Citation2014) and is considered to be simple yet one of the most powerful and widely applicable tools for prediction and forecasting studies in numerous disciplines. ANN models can develop a relationship for problems that are difficult to solve via traditional models (Zhang et al. Citation1998). The only requirement for the ANN model is the availability of a large dataset, as it uses self-adaptive methods that learn from the available set of data (Stepchenko Citation2016). The growing demand and extensive movement of people towards the urban areas severely alter the climate, temperature and atmospheric profiles, making it important to understand and predict future growth for sustainable urban development with sufficient resources and a comfortable living environment.
Study area
Kolkata Metropolitan Area (KMA) () is one of the major urban agglomerations in India. KMA was considered for analysis based on infrastructure (planned and unplanned), level of urbanization, socioeconomic factors, population, etc. The study has been carried out for the metropolitan boundary with a 10 km buffer to understand the change in surface temperatures due to urban expansion in the core region and the peri-urban area. illustrates basic geographic, climatic and demographic details about KMA.
Table 1. Geographic, climatic and demographic profile of the Kolkata metropolitan area.
The rising metropolitan population has caused problems such as increased pollution due to land-use change, construction activities, and traffic congestion. Due to the increased anthropogenic activities, the region is experiencing a rise in RSPM (respirable suspended particulate matter) that has crossed the air quality standards provided by India’s national ambient air quality standards, causing many respiratory diseases such as asthma, lung cancer, etc. These changes across the KMA have led to various environmental problems, and the region is facing microclimate change resulting in a changed crop cycle, altered rainfall patterns, and increased surface temperatures.
Methodological framework
The entire analysis was conducted utilizing Landsat imagery, which provides dataset at a spatial resolution of 30 m, which is best available for thermal remote sensing, making it an optimal choice. The data was sourced freely from https://earthexplorer.usgs.gov/, allowing a comprehensive and cost-effective exploration of the study area.
The land use and LST for the study area were estimated for 2004, 2009, 2014, and 2019 using a Gaussian Maximum Likelihood Classifier and the Radiative Transfer Equation, respectively, as shown in Nimish et al. (Citation2020) (method, result, and analysis are in Appendix A). Furthermore, these maps were used to simulate LULC (Land Use and Land Cover) and forecast LST. shows the overall methodological framework and algorithms applied to forecast LST. The overall framework can be divided into four categorical sub-sections. The first subsection details the selection of parameters that served as input to the models. The second subsection provides information about land cover and land use prediction. The third and fourth subsections illustrate the development of the multiple linear regression and ANN models, respectively.
Selection of input parameters
A detailed literature review (Pinty and Verstraete Citation1992; Bannari et al. Citation1995; As-Syakur et al. Citation2012; Sun et al. Citation2017; Acharya et al. Citation2018; Fern et al. Citation2018; Naji Citation2018; Ettehadi et al. Citation2019; Somvanshi and Kumari Citation2020; Gunathilaka Citation2021; Khalid et al. Citation2021; Taloor et al. Citation2021; Tin and Muttitanon Citation2021; Fabijańczyk and Zawadzki Citation2022; Kebede et al. Citation2022; Liu et al. Citation2022) identified various types of land cover indices that have been proven to have the potential for forecasting LST (see Appendix B). Several land cover indices for each of the class, i.e. urban area (7 nos.), vegetation (11 nos.), water bodies (4 nos.), and barren surfaces (3 nos.) were computed for 1994, 1999, 2004, 2009, 2014, and 2019 by using a combination of bands and constants. Further, binary classification was performed for each LC index to extract the associated class (urban, vegetation, water, and others). It was compared with the same class extracted from the land use maps. Among them, the indices (for each class) that exhibited comparable accuracy and were found to be able to define the higher number of pixels for the class, i.e. represented the most accurate landscape conditions, were selected as inputs for forecasting LST. The selected indices were the Urban Index (UI), Atmospherically Resistant Vegetation Index (ARVI), Automated Water Extraction Index (AWEI), and Bare Soil Index (BSI) for urban, vegetation, water bodies, and barren classes, respectively.
Prediction of landscape
Simulation of future land cover
The transition in land cover can play a significant role in understanding the alterations in the landscape over time. Land cover maps are vital in visualizing, monitoring, and predicting studies related to vegetation analysis, urban landscapes, climate change, ecological and environmental changes, forest transitions, biomass estimation, etc. (Cui et al. Citation2020). This study used a Neural Network Model (NNM) to predict the land cover maps by considering the historical land cover maps of the region. The ANN model with its architecture, as shown in , was used. The dataset used for training the model comprised land cover maps for 1994, 1999, 2004, 2009, 2014, and 2019. The dataset was divided into input and output layers for training the model. Land cover maps for 1994, 1999, 2004, 2009, and 2014 were considered input layers, and those for 2019 were considered the output layer. A representative sample area was considered such that it considered and covered approximately 25% of the region and was distributed heterogeneously over the study area.
Figure 3. Neural network model architecture for simulation of future land cover. Note: t: year under consideration. For example: if t = 2019, and the difference is five years, i.e., n = 5, then t – n = 2014, t – 2n = 2009, and so on. The histogram of the selected representative sample was compared with that of the entire region and was aligned with it. The individual land cover maps were then extracted concerning the sample area, and normalization was performed to remove the biases. The model was then trained with alterations to hyperparameters, including the architecture, the number of repetitions (epochs), the learning rate, the activation function, etc. Once satisfactory accuracy was achieved, the weights were fixed. The model’s accuracy was tested using error estimation (mean absolute error, mean square error, root mean square error, and mean percentage error). The trained model was then used to predict land cover maps for 2024 and 2029. Note. The model was generated for individual land covers. Thus, there exist four models with similar hyperparameters exist AWEI and BSI.

Prediction of Land Use using CA-Markov
The CA-Markov model is prevalently used for its ability to consider spatial and temporal alterations and is one of the most effective models for simulating urban landscapes (Bharath et al. Citation2013). The coupled model characterizes the progression in dynamic modelling and prediction by achieving close to real-time landscape (land use) scenarios over time and space (Sang et al. Citation2011; Yang et al. Citation2014; Yirsaw et al. Citation2017). The integration of Cellular Automata-Markov Chain models, i.e. CA-Markov, can simulate or predict any number of land use classes with any transition (Tadese et al. Citation2021). The CA-Markov model considers the probability of state change observed from the Markov chain analysis and the rules of state change from the CA analysis. It also considers the number of iterations and the kernel (contiguity filter) the analyst specifies to predict the future state. Sang et al. (Citation2011) simulated the spatial pattern of land use for various towns and villages in Beijing. Bharath et al. (Citation2018) simulated and analyzed the urban growth in five megacities in India using the CA-Markov model. Hamad et al. (Citation2018) utilized the CA-Markov model to predict land use for 2023, considering two scenarios. Vandansambuu et al. (Citation2020) predicted the land use of the Orkhon province, Mongolia, using the CA-Markov model. Tariq and Shu (Citation2020) predicted land use and urban index (UI) using CA-Markov and further forecasted LST using correlation with UI.
To predict future land use maps and develop the model (), land use for 2009, 2014, and 2019 was used. Using Markov chain analysis, transition matrices (probability and area) were obtained by considering land use in 2009 and 2014. Additionally, a contiguity filter of 5 × 5 was used to capture the effect of the neighboring pixels. Based on the CA-Markov approach, keeping 2014 as the base map and considering the transition from 2009 to 2014, land use in 2019 was simulated with 1, 5, 10, 20, 50, 100, and 150 iterations. It was then compared to the original land use map of 2019, and it was inferred that the accuracies reached near saturation at ten iterations, as shown in . After that, the developed CA-Markov model was used to predict future land use, wherein 2024 was projected by keeping 2019 as the base map and considering the Markov present transition in land use from 2014 to 2019, with a future transition of 5 years. Similarly, the land use was projected for 2029, considering 2019 as the base map and the Markov present transition from 2014 to 2019, with a future transition of 10 years.
Land Surface Temperature forecasting
Multiple linear regression model
Development of the model
Regression analysis is a statistical measure used to derive a relationship between dependent and independent variables of interest. The regression analysis determines how significantly the dependent variable changes with alterations in the independent variables (Needle Citation2020). In most models, the output’s dependency is not limited to a single variable. Instead, multiple inputs are required to define the dependent variable. Multiple linear regression is a solution for such models, as it considers the relative impact of each independent variable on the dependent variable. A generalized multiple linear regression can be expressed as shown in Equationequation 1(1)
(1) .
(1)
(1)
Here,
y → dependent variable
xi → independent variables
β0 → y intercept
βi → slope coefficient for each independent variable
Regression has an extensive range of applications in numerous fields and is one of the most widely used statistical measures for predicting and forecasting climate parameters and urban scenarios (Sreehari and Srivastava Citation2018; Sreehari and Ghantasala Citation2019). The selected land cover maps corresponding to each of the considered land use classes were used to derive a relationship with the LST. As explained earlier, a representative sample area was considered, and maps were then extracted concerning the land use class it represents (for example, the values of the Urban Index were extracted only for the urban class and were made zero for the rest of the region) as shown in . Multiple linear regression was then performed by considering LST as the dependent variable and land cover maps as independent variables. The relationship derived from the regression model was used to estimate LST for the entire region.
The model and algorithm (RTE) estimated LSTs were compared via accuracy assessment. Accuracy assessment was performed by estimation of mean absolute error (MAE), mean squared error (MSE), root mean square error (RMSE) and mean percentage error (MPE) and the formulas are represented by EquationEqs. 2–5.
(2)
(2)
(3)
(3)
(4)
(4)
(5)
(5)
Here.
yi → model predicted value
xi → true value
n → number of data points
Training, testing and sensitivity analysis
The relationship obtained from the initial land covers shows a negative correlation with the ARVI and AWEI, indicating a decrease in LST with an increase in vegetation and water bodies in the region. UI and BSI, however, show a positive correlation, signifying a rise in LST with an increase in concrete structures and barren areas. The model provided satisfactory results; however, it was observed that due to the variation in the ranges of the land cover indices, the results were biased towards the land cover with higher values. The land cover indices were normalized to resolve this problem, and multiple linear regression was performed for the normalized land covers. After developing the relationship and identifying a satisfactory accuracy, the regression equation for the previous time t-5 was used to estimate LST for t, and with the input land covers of t, an accuracy assessment was performed. The relationships obtained from the normalized land cover for 2004, 2009, 2014, and 2019 are shown in EquationEqs. 6–9, and shows the significance and shows the error matrix. It can be observed from the weights assigned to each land cover class in EquationEqs. 6–9 that the change in urban areas contributes to the highest alterations in the LST, followed by the other category (barren area). The vegetated lands moderately affect the region, and water bodies alter the surface temperature minimally.
(6)
(6)
(7)
(7)
(8)
(8)
(9)
(9)
Table 2. Significance level of LST and LC.
Table 3. Error matrix calculated for the analysis.
Accuracy assessment in the form of an error matrix shows that the mean absolute error in the model estimated and RTE (algorithm) estimated LST ranges from 0.84 °C to 1.42 °C for the entire study period, while the root mean square error varies from 1.00 °C to 1.78 °C. When the last time t-5 equation was used to estimate the LST of t and then compared with the LST obtained from the RTE for time t, the mean absolute error ranged from 1.25 °C to 2.26 °C. Sensitivity analysis was carried out by altering the land covers and observing the changes in LST. It was observed that a change of 0.1 in the normalized value of the urban index could alter the LST by 1.02–1.79 °C. A 0.1 change in the normalized value of the BSI representing the barren areas changes the LST by 0.43–1.43 °C. ARVI (representing vegetated areas) and AWEI (corresponding to the water bodies) signified the minor sensitivity as a change of 0.1 in the normalized value of ARVI and AWEI alters the LST by 0.16–0.45 °C and 0.01–0.18 °C, respectively.
Artificial neural network model
As understood from the previous section, the region’s LST can be defined and forecasted by correlating it with the landscape. However, the landscape alone cannot determine the LST. Some other variables need to be considered. It is a well-established fact that climate parameters such as temperature follow specific historical trends, and most predictions are made based on the previous period. Also, altitude plays a vital role in defining the region’s temperature. The altitude is inversely related to the air temperature and follows a lapse rate of −6.5 °C with an increase of 1000 m. However, the surface temperature is affected by altitude in the form of wind turbulence, speed, and gust. Thus, along with the land cover of the region, historical surface temperatures and elevation were considered when developing the model for forecasting LST.
Development of the model, training and testing
The NNM architecture used was 8:12:6:3:1, as shown in . The layers include UI (Urban Index), ARVI (Atmospheric Resistant Vegetation Index), AWEI (Automated Water Extraction Index), BSI (Bare Soil Index), DEM (Digital Elevation Data from ALOS World 3D-30m), and LST for time ‘t’, ‘t-n’, ‘t-2n’, and ‘t-3n’ were first converted into a data frame and then into a matrix. The values were then normalized to remove the input layers’ biases. The data was further divided into input and output layers, wherein input layers comprised UI(t), ARVI(t), AWEI(t), BSI(t), DEM, LST(t-n), LST(t-2n), and LST(t-3n), and the output of the model was considered to be LST(t). The output layer will be a function of all the input variables, as shown in EquationEq. 10(10)
(10) .
(10)
(10)
A representative sample area was considered for training the model, as explained in the section ‘Prediction of Land Use Using CA-Markov’. This sample dataset was used as the test data. A known set of input and output layers were provided to the model architecture, as illustrated in . The hyperparameters considered for the model’s training were its architecture, number of epochs and repetitions, learning rate, and activation function. These parameters were varied, and the ones that provided the minimum error and best accuracy were considered for estimating the weights. The details of the chosen hyperparameters are as follows:
Architecture: 8:12:6:3:1
Epochs:30
Learning rate: 0.01
Activation function: Sigmoid
Mean Absolute Error (MAE) = 0.47 °C
Mean Squared Error (MSE) = 0.38 °C
Results
Predicted land use
The simulated LU maps with the actual and simulated (2024 and 2029) percentage urban areas, are illustrated in , and a continual rise in urban areas was observed. Simulation shows that the urban area has already begun to surpass the metropolitan boundaries and will continue to do so in the future. The metropolitan area is observed to densify in the core for various improved infrastructure developments, like relocating government offices to Howrah. An increased urban density was observed in the near future (2024 and 2029) over the Newtown area (toward the east of the KMA boundary) and in the buffer area, wherein small urban pockets are simulated to increase in terms of urban density slightly. This rise in urban areas is observed over vegetated or open lands, while the water table remains almost unchanged. A significant rise in urban area was observed towards the western part of KMA, adjacent to the highway or road passing via Jala Dhaulagiri, Biparnna Para, Satgharia, and a few other locations.
Multiple linear regression
The relationship obtained between the inputs and outputs of 2019 was used to forecast LST for future years, i.e. 2024 and 2029, as shown in . The inputs for these calculations were the predicted land cover maps, which were extracted to class-wise values based on simulated land use maps. The results indicated a mean LST of 29.59 °C and 29.62 °C. When the LST for 2024 and 2029 was assessed with information from future projected land use maps, it was observed that the LST is expected to rise in locations where urban areas are likely to expand with the replacement of green (vegetation) or open spaces. It was understood from the forecasted LST for 2024 that the core part of Kolkata city, comprising densely populated urban areas and heavily crowded marketplace places, would continue to face the challenging issue of high LSTs (31–33 °C). The rise can be inferred to an increase in concrete congestion (as observed in simulated maps for 2024 and 2029) in the entire neighborhood of the core CBD (Central Business District). Few of the urban pockets with ample open or vegetated spaces have been observed to correspond to moderate LSTs ranging from 30 °C to 31 °C. Another reason for some reduction in LST in these paved regions in the core can be inferred from the presence of small water bodies that enhance the region’s microclimate. The industrial areas and manufacturing units adjacent to the river Hooghly signify higher surface temperatures even in the future as there is an increase in residential and commercial space in the nearby regions. For 2029, the LST shows a slight reduction in the same residential areas. However, the forecasted map shows a persistent rise in LST in the bazaar and highly dense urban areas in the core. Also, most of the region in the buffer shows an increment in the LSTs of approximately 1–2 °C. The metropolitan area’s planned layouts, including New Town and Bidhannagar, showed a slight reduction in the LSTs due to ample open and vegetated spaces and water bodies in the central park and aquaculture.
Figure 9. Forecasted LST using regression modelling for 2024 and 2029. Note. The legend for each year varies in value (range) and color. Note. The legend considered for LST had varying ranges for different years because the study not only focuses on the temporal variation over the years but also understands the variation of LST due to the presence of each class for the same year. LST variations across the study area were easier to comprehend by utilizing the changes in the range of LST. Thus, LST’s maximum and minimum values are used as a legend and color denotations n for all the years instead of using a standard range. (A) Industrial and manufacturing units. It generates waste and emissions in various forms and is surrounded by residential areas. (B) Kolkata city CBD. It consists of densely packed marketplaces and a few residential complexes.

depicts two micro-regions that were considered for better understanding. The ‘A’ region represents the older section of Kolkata, which lies closer to the Hooghly River’s bank (including Khardara and Uttarpara). The surface temperature in a few places in the region was found to be dropping. In contrast, the surface temperature of impervious surfaces was found to be rising sharply, especially in areas where industrial or manufacturing units are located. This increased the overall mean surface temperature of the ‘A’ area. Another zone chosen was the entire metropolitan core business district (CBD), which was designated as ‘B’ and is highly congested owing to the movement of many people. Due to increased built-up areas and traffic congestion/jams, the core and vicinity of this region have been unceasingly experiencing increased LST.
Model using artificial neural network
The generated model was achieved with acceptable accuracy and was fetched with the simulated land cover maps (UI, ARVI, AWEI, BSI) for 2024 and 2029. The projected LC maps for 2024, the DEM and temporal LSTs (2019, 2014 and 2009) were fed to the model for forecasting LST for 2024. Similarly, simulated LC for 2029, DEM and temporal LSTs (2024, 2019 and 2014) were provided as input to the developed model to get the LST predictions for 2029. The forecasted LST maps for 2024 and 2029 are shown in . The overall mean surface temperature of the study area was almost similar. However, the model incorporates some errors; if those are added to the mean, a rise of ∼0.5 °C is observed. As explained in the section ‘Prediction of Land Use using CA-Markov’, the simulated land use maps show a significant rise in urban areas.
Figure 10. Forecasted LST using Artificial Neural Network modelling for 2024 and 2029. Note: The legend for each year varies in value (range) and color. (A) Kolkata city CBD. It consists of densely packed marketplaces and a few residential complexes. (B) Industrial and manufacturing units. It generates waste and emissions in various forms and is surrounded by residential areas. (C) Rajarhat (New Town). It consists of planned residential and commercial layouts with ample open spaces but lacks green spaces.

It was observed that the urban pockets in the core city region towards the western side of Bidhannagar, which is primarily dominated by bazaars (Bara bazaar, Bow bazaar, Hatibagan Market, Raja bazaar) and residential areas, are predicted to have a continual increment in the surface temperatures, owing to the densification of urban areas. However, few of the locations within this region are forecast to have a slight reduction in surface temperature due to a nearby park in the residential locality. When visualized for 2029, it was identified that the regions with LST <28 °C have seen a significant reduction and these regions have transitioned into the temperature range of 28 °C–30 °C. The other regions with a continual rise in surface temperature were the industries along the river Hooghly. Most of the results of the forecasted LST were found to be similar to the regression analysis. However, contrary to the regression results, the newer part of the metropolitan area that comprises the planned layouts (Rajarhat and Bidhannagar) also signified a rise in surface temperature by 1 °C in the case of LST forecasting using ANN, owing to the fact of densification of urban pockets in these areas.
Three micro-regions – ‘A’, ‘B’, and ‘C’ – were chosen for a thorough evaluation of smaller pockets. The LST of impervious surfaces is anticipated to grow attributable to an increase in the concentration of buildings and greater traffic congestion, similar to the results observed in the MLR model. Localities such as Khardara and Uttarpara were included in Region ‘B’. Due to the dominance of manufacturing facilities with a roof that absorbs a large quantity of heat throughout the region, the LST corresponding to that region was estimated to have increased significantly. The area of Rajarhat (New town), currently the hotspot of growth in the Kolkata Metropolitan Area, was represented by Region ‘C’. Due to the construction of numerous commercial buildings and office towers, Rajarhat is one of the fastest-developing areas in the city. Numerous service-related developments, such as hospitals, restaurants, and grocery stores, have sprung up due to the expansion of IT centres and other commercial structures. This has been the most significant contributor to rising LST in the region. The projected land use suggests that the urban area in this region will continue to develop, which might be regarded as one of the reasons for higher LST in the future.
Discussion
The simulated land use maps indicate that the metropolitan area will continue to grow and sprawl in all directions, especially towards the eastern and western sides, as is the usual scenario. These increased alterations in the region’s landscape and the conversion of permeable surfaces to impervious ones are expected to further deteriorate the living environment of the entire region. Forecasted LST using both algorithms suggests an increment in the core area with a rise in the buffer regions. The overall rise in forecasted LST was slightly less in the regression analysis, while the NNM gave a slightly higher LST. As per regression analysis, LST for 2024 had a decent rise, while a very small rise in LST was produced as an output by the model. One of the reasons for that can be the saturation of the land cover values, and another can be the input parameters not considered, i.e. the trend of LST and elevation. On the other hand, ANN fetched better and more realistic results of LST, forecasting it to increase with higher alterations in the landscape, as observed via the historical trends. However, it was understood that the models were unable to capture the outliers, i.e. extreme temperatures (low and high), because, during the training stage, the model tends to increase the accuracy (minimize the errors) by identifying the most significant cloud of data surrounding the mean, thus ignoring the outliers. The training pixels used to train both the models in this study had more than 80% of the data between 25 and 37 °C. Due to this, it can be seen that the forecasted LST value ranges within the same set of values for both models.
It was observed that the regions with extreme surface temperatures in the historical years had shown a decrement in the LST values for 2024 and 2029. However, the models captured the increase in LST over the upcoming landscape changes, which efficiently fulfilled the research scope of this scientific literature. The forecasted results indicate that immediate attention has to be given to the unstoppable urban sprawl of the metropolitan region. The core part of Kolkata city, the Howrah region, and older parts are already densely urbanized with very few water bodies and green spaces. This can be interpreted as the cause of the LST’s continual rise. The outcomes align seamlessly with numerous studies indicating a consistent escalation in Land Surface Temperatures (LSTs) within urban areas. This phenomenon can be attributed to the growing diversification in city landscapes, as observed in the research conducted by Mansourmoghaddam et al. (Citation2023), Tian et al. (Citation2023), Kafy et al. (Citation2021), Mustafa et al. (Citation2020), and Ranjan et al. (Citation2018).
Furthermore, additional energy in the form of heat would be absorbed in the region due to the increasing densification of impermeable surfaces, resulting in enhanced LST not only in the specific locale but also in the surrounding area. Any further increase in these highly dense and jam-packed urban areas might collapse the metropolitan area in terms of comfortable living standards. Another issue associated with the Kolkata metropolitan area is its sprawl, which is predicted towards the western part, wherein small urban pockets might merge into the city. Similarly, due to growing concretization, rising population density, expanded commercial structures, and higher traffic mobilities, the forthcoming hotspot of urban expansion, i.e. the New Town region, was also expected to have an increasing LST trend. These planned developments towards the east might also cross their threshold, resulting in a shortage of living space, a lack of fresh air, a high level of thermal discomfort, improper and inefficient usage of natural resources, food and water shortages, inadequacy in the essential services, and most importantly, insufficiency of healthcare facilities. The metropolitan area needs to decongest with the development of satellite towns where industries and densely populated regions can be relocated. The research is an addition to a group of scientific papers that provide a shred of evidence to support the necessity of identifying and implementing cooling action plans across the globe.
Almost every other city across the globe is currently dealing with increased temperatures and heat waves, making rising cooling demands a major challenge and a unique opportunity. Urban cooling actions are now considered to be directly associated with economic growth and are linked with several SDGs. It is widely acknowledged to play a key role in dwellers’ health, well-being, and productivity. The mitigation strategies for the efficient cooling of cities can be implemented through regulatory instruments (policies and acts) or passive cooling techniques. The regulatory instruments can be further categorized into various thematic areas that includes (i) Cooling at building level: restricting or promoting building construction materials based on their heat retention capacities, mandating efficient building designs as stated in Energy Conservation Building Code (ECBC) for all commercial buildings, making third-party verification and environmental clearance in terms of building cooling obligatory, spreading awareness about the ECBC via various platforms such as social media, print media and conduction of training programme, implementation of star ratings and eco-labelling for cooling equipment, over a long period – legislating efficient and green building designs for both residential and commercial sectors, promoting initiatives to help in the retrofitting of existing buildings for reducing the cooling energy consumption; (ii) Cold chain and refrigeration: encouraging development of environmentally benign (lower global warming potential) refrigerants, mandating the purchase of commercial equipment that uses refrigerants to be purchased under energy efficiency star ratings, incentivizing to encourage the development and use of energy-efficient cold chain infrastructures, providing specialized training for cold chain executives and technicians to encourage the use and operation of updated and energy-efficient technologies, retrofitting outdated cold chain infrastructures with cutting-edge technologies and more efficient refrigerants; (iii) Transportation sector: Endorsing Corporate Average Fuel Economy (CAFE) standards, developing a green labelling system for automobiles, promoting public transportation by improving connectivity and service quality, enforcing the adoption of cleaner fuel sources; (iv) Technician training: evaluating the demand-supply gap and the availability of high-quality training facilities for technicians in cooling industry, introducing new training courses and establishing centres that are constantly improving and upgrading the technology they teach, directly connecting technicians with training providers and consumers via social media platforms, mandating the certificate training courses for the technicians and manufacturing unit personnel; (v) Research and Development (R&D): recognizing urban cooling as one of the top priorities and encouraging cooling-related research, constituting an advisory committee for R&D with members from various ministries, research institutes and academics, promoting collaborative research with international institutes and research centres to nurture their knowledge and expertise, providing financial assistance for interdisciplinary research by developing Public Private Partnerships (PPP). Furthermore, a few of many passive cooling measures implementable at the city level include conversion of the waste/barren spaces into green ones by increased native plantations as they require minimal maintenance and help in recharging the groundwater level; construction of cool pavements by applying a special type of paints/chemicals over the traditional pavements; soft landscaping across the streets with higher foot activity and avenue plantation the collector and arterial roads; addition of water bodies in the form of ponds, lakes, swimming pools, aquaculture, etc. or by daylighting a water body; construction of Sustainable Urban Drainage Systems (SUDS) in the form of rain gardens and swales with plants; proper evaluation of adequate urban geometry, orientation and design during construction. In addition to these, passive cooling measures at the building level can significantly reduce the surface and ambient air temperatures to provide cooling. Some of the few measures that can be implemented are (i) shading the openings, roofs and walls of the building with elements such as chajjas, fins, brise-soleils or louvres, awnings, adjustable sunshade canvas and vegetation landscaping; (ii) insulating the exterior and roof of the building by terrace gardens, installing vertical landscape, hanging gardens and creepers; (iii) improving the ventilation by implementing solar chimneys, air vents, wind catcher/towers and appropriately installing windows and openings; (iv) applying radiative cooling measures in the form of diode roofing and roof ponds; (v) using the efficiency of evaporative cooling measures such as Passive downdraft evaporative cooling (PDEC), Roof surface evaporative cooling (RSEC); (vi) utilizing the potential of geo coupling or earth coupling in the form of earth berming, earth air tunnel and geothermal heat pumps. While these passive cooling techniques are more geared toward the voluntary actions of the citizen, they can also be enforced by the governments and administrative bodies over a period of time to maximize the cooling action plans to promote a thermally comfortable living environment for the residents.
Conclusion
Two models have been developed to anticipate LST in the Kolkata Metropolitan Region for the years 2024 and 2029. These established modelling techniques are widely acknowledged as effective methods for forecasting climate parameters. The study determines the impact of various land cover alterations (UI, ARVI, AWEI, BSI) on the region’s LST. The major goal of the communication was to better understand and identify the locations that rising LST may adversely impact in the near future due to landscape modifications. The research demonstrates a strong link between landscape changes and a region’s LST. However, changes in LST are influenced by various other secondary parameters besides the landscape. The research also demonstrates the capability of the CA-Markov and ANN algorithms to predict the region’s land use and land cover, respectively. The two models, i.e. MLR and ANN, used to forecast LST indicated that there would be a continual increment in the LST over the existing and likely upcoming built-up areas. Additionally, the regions near the developments will face the difficult problem of increased thermal discomfort and heat waves. With the analyses performed, it was understood how alterations and imbalances in the landscape could heavily impact urban health and the environment. It is a known fact that the thermal environment of almost every city, be it small or large, is deteriorating rapidly, and we are facing an issue of micro-climatic alterations and seasonal shifts. The most crucial factor accountable for this is the unplanned growth of urban impervious surfaces, which further leads to several secondary factors, including an increase in anthropogenic heat and a rise in pollutants. The KMA region in India is one of the fastest-growing urban agglomerations, which is now sprawling in almost all directions and has already been saturated in the core. This communication’s finding is coherent with scientific literature, revealing an increase in LST due to landscape modification over an urban area, leading to several negative consequences. The outcomes from the communication will primarily assist in urban heat mitigation efforts and further aid in developing relevant policies for dealing with this biggest developmental challenge. The significant findings from the research will help promote a sustainable and comfortable urban environment for the current and future residents of KMA.
The analysis delved into one of the most intricate and extensively studied domains, unveiling numerous enhanced research avenues. It spotlighted the potential for advancements, including the precise estimation of Land Surface Temperature (LST) by incorporating supplementary atmospheric and surface parameters. Moreover, there’s room for the development of highly accurate, precise, and region-specific forecasting models for LST. These models could harness state-of-the-art technologies, integrating advanced algorithms that take into account a broader spectrum of parameters. Such models could forecast LST based on diverse user-defined or IPCC scenarios, offering a holistic perspective on the future conditions within the city and metropolitan areas.
Disclosure statement
No potential conflict of interest was reported by the author(s).
References
- Acharya TD, Subedi A, Lee DH. 2018. Evaluation of water indices for surface water extraction in a Landsat 8 scene of Nepal. Sensors. 18(8):2580. doi:10.3390/s18082580.
- Aggarwal SP, Garg V, Gupta PK, Nikam BR, Thakur PK. 2012. Climate and Lulc change scenarios to study its impact on hydrological regime. Int Arch Photogramm Remote Sens Spatial Inf Sci. XXXIX-B8:147–152. doi:10.5194/isprsarchives-XXXIX-B8-147-2012.
- As-Syakur AR, Adnyana IWS, Arthana IW, Nuarsa IW. 2012. Enhanced built-up and bareness index (EBBI) for mapping built-up and bare land in an urban area. Remote Sens. 4(10):2957–2970. doi:10.3390/rs4102957.
- Atkinson PM, Tatnall AR. 1997. Introduction neural networks in remote sensing. Int J Remote Sens. 18(4):699–709. doi:10.1080/014311697218700.
- Bannari A, Morin D, Bonn F, Huete A. 1995. A review of vegetation indices. Remote Sens Rev. 13(1-2):95–120. doi:10.1080/02757259509532298.
- Bharath HA, Chandan MC, Vinay S, Ramachandra TV. 2018. Modelling urban dynamics in rapidly urbanising Indian cities. Egypt J Remot Sens Space Sci. 21(3):201–210. doi:10.1016/j.ejrs.2017.08.002.
- Bharath HA, Vinay S, Ramachandra TV. 2013. Prediction of land use dynamics in the rapidly urbanising landscape using land change modeler. Paper presented at International Conference on Advances in Computer Science; Dec; Delhi, India: Elsevier.
- Bharath HA, Vinay S, Ramachandra TV. 2014. Prediction of spatial patterns of urban dynamics in Pune, India. Paper presented at India Conference (INDICON); Dec 11–13; Pune, India. https://ieeexplore.ieee.org/document/7030404/.
- Bisht K, Dodamani SS. 2016. Estimation and modelling of land surface temperature using Landsat 7 ETM + images and fuzzy system techniques. Paper presented in AGU Fall Meeting Abstracts, San Francisco, California; Dec 12–16. http://adsabs.harvard.edu/abs/2016AGUFM.A23A0188B.
- Bisht K, Dodamani SS. 2016. Estimation and modelling of land surface temperature using Landsat 7 ETM + images and fuzzy system techniques. Presented at AGU Fall Meeting Abstracts (Vol. 2016), San Francisco, California, USA; Dec. p. A23A-0188.
- Chen M, Zhang H, Liu W, Zhang W. 2014. The global pattern of urbanization and economic growth: evidence from the last three decades. PLoS One. 9(8):e103799. doi:10.1371/journal.pone.0103799.
- Cook J, Oreskes N, Doran PT, Anderegg WRL, Verheggen B, Maibach EW, Carlton JS, Lewandowsky S, Skuce AG, Green SA, et al. 2016. Consensus on consensus: a synthesis of consensus estimates on human-caused global warming. Environ Res Lett. 11(4):048002. doi:10.1088/1748-9326/8/2/024024.
- Cui C, Zhang W, Hong Z, Meng L. 2020. Forecasting NDVI in multiple complex areas using neural network techniques combined feature engineering. Int J Digital Earth. 13(12):1733–1749. doi:10.1080/17538947.2020.1808718.
- Ettehadi OP, Kaya S, Sertel E, Alganci U. 2019. Separating built-up areas from bare land in mediterranean cities using Sentinel-2A imagery. Remote Sens. 11(3):345. doi:10.3390/rs11030345.
- Fabijańczyk P, Zawadzki J. 2022. Spatial correlations of NDVI and MSAVI2 indices of green and forested areas of urban agglomeration, case study Warsaw, Poland. Remote Sens Appl Soc Environ. 26:100721. doi:10.1016/j.rsase.2022.100721.
- Fern RR, Foxley EA, Bruno A, Morrison ML. 2018. Suitability of NDVI and OSAVI as estimators of green biomass and coverage in a semi-arid rangeland. Ecol Indic. 94:16–21. doi:10.1016/j.ecolind.2018.06.029.
- Foody GM. 2006. Pattern recognition and classification of remotely sensed images by artificial neural networks. In Ecological informatics. Berlin, Heidelberg: Springer. p. 459–477.
- Founda D, Santamouris M. 2017. Synergies between urban heat island and heat waves in Athens (Greece), during an extremely hot summer (2012). Sci Rep. 7(1):10973. doi:10.1038/s41598-017-11407-6.
- Gunathilaka MDKL. 2021. Modelling the behavior of DVI and IPVI vegetation indices using multi-temporal remotely sensed data. Int J Environ Eng Educ. 3(1):9–16. doi:10.55151/ijeedu.v3i1.42.
- Hamad R, Balzter H, Kolo K. 2018. Predicting land use/land cover changes using a CA-Markov model under two different scenarios. Sustainability. 10(10):3421. doi:10.3390/su10103421.
- Huang S, Chang J, Huang Q, Chen Y. 2014. Monthly streamflow prediction using modified EMD-based support vector machine. J Hydrol. 511:764–775. doi:10.1016/j.jhydrol.2014.01.062.
- Imran HM, Hossain A, Islam AKM, Rahman A, Bhuiyan MAE, Paul S, Alam A. 2021. Impact of land cover changes on land surface temperature and human thermal comfort in Dhaka City of Bangladesh. Earth Syst Environ. 5(3):667–693. doi:10.1007/s41748-021-00243-4.
- IPCC. 2021. Sixth assessment report. [accessed 2023 October 23]. https://www.ipcc.ch/report/sixth-assessment-report-cycle/.
- Jia H, Yang D, Deng W, Wei Q, Jiang W. 2021. Predicting land surface temperature with geographically weighed regression and deep learning. Wiley Interdiscip Rev Data Min Knowl Discov. 11(1):e1396.
- Jin M, Li J, Wang C, Shang R. 2015. A practical split-window algorithm for retrieving land surface temperature from Landsat-8 data and a case study of an urban area in China. Remote Sens. 7(4):4371–4390. doi:10.3390/rs70404371.
- Kafy AA , Al Rakib A, Akter KS, Jahir DMA, Sikdar MS, Ashrafi TJ, Mallik S, Rahman MM. 2021. Assessing and predicting land use/land cover, land surface temperature and urban thermal field variance index using Landsat imagery for Dhaka Metropolitan area. Environ Challenges. 4:100192. doi:10.1016/j.envc.2021.100192.
- Kebede TA, Hailu BT, Suryabhagavan KV. 2022. Evaluation of spectral built-up indices for impervious surface extraction using Sentinel-2A MSI imageries: a case of Addis Ababa city, Ethiopia. Environ Challenges. 8:100568. doi:10.1016/j.envc.2022.100568.
- Khalid HW, Khalil RMZ, Qureshi MA. 2021. Evaluating spectral indices for water bodies extraction in western Tibetan Plateau. Egypt J Remote Sens Space Sci. 24(3):619–634. doi:10.1016/j.ejrs.2021.09.003.
- Khalil U, Aslam B, Azam U, Khalid HMD. 2021. Time series analysis of land surface temperature and drivers of urban heat island effect based on remotely sensed data to develop a prediction model. Appl Artif Intel. 35(15):1803–1828. doi:10.1080/08839514.2021.1993633.
- Li Z-L, Tang B-H, Wu H, Ren H, Yan G, Wan Z, Trigo IF, Sobrino JA. 2013. Satellite-derived land surface temperature: current status and perspectives. Remote Sens Environ. 131:14–37. doi:10.1016/j.rse.2012.12.008.
- Liu Y, Meng Q, Zhang L, Wu C. 2022. NDBSI: a normalized difference bare soil index for remote sensing to improve bare soil mapping accuracy in urban and rural areas. Catena. 214:106265. doi:10.1016/j.catena.2022.106265.
- Lu Y, Yue W, Huang Y. 2021. Effects of land use on land surface temperature: a case study of Wuhan, China. Int J Environ Res Public Health. 18(19):9987. doi:10.3390/ijerph18199987.
- Mahmood R, Pielke RA Sr, Hubbard KG, Niyogi D, Bonan G, Lawrence P, McNider R, McAlpine C, Etter A, Gameda S, et al. 2010. Impacts of land use/land cover change on climate and future research priorities. Bull Amer Meteor Soc. 91(1):37–46. doi:10.1175/2009BAMS2769.1.
- Mansourmoghaddam M, Rousta I, Zamani M, Olafsson H. 2023. Investigating and predicting Land Surface Temperature (LST) based on remotely sensed data during 1987–2030 (A case study of Reykjavik city, Iceland). Urban Ecosyst. 26(2):337–359. doi:10.1007/s11252-023-01337-9.
- Mas JF, Flores JJ. 2008. The application of artificial neural networks to the analysis of remotely sensed data. Int J Remote Sens. 29(3):617–663. doi:10.1080/01431160701352154.
- Mathew A, Sreekumar S, Khandelwal S, Kaul N, Kumar R. 2016. Prediction of land-surface temperatures of Jaipur City using linear time series model. IEEE J Sel Top Appl Earth Observations Remote Sensing. 9(8):3546–3552. doi:10.1109/JSTARS.2016.2523552.
- Mustafa EK, Co Y, Liu G, Kaloop MR, Beshr AA, Zarzoura F, Sadek M. 2020. Study for predicting land surface temperature (LST) using landsat data: a comparison of four algorithms. Adv Civ Eng. 2020:1–16. doi:10.1155/2020/7363546.
- Mutiibwa D, Kilic A, Irmak S. 2014. The effect of land cover/land use changes on the regional climate of the USA high plains. Climate. 2(3):153–167. doi:10.3390/cli2030153.
- Naji TA. 2018. Study of vegetation cover distribution using DVI, PVI, WDVI indices with 2D-space plot. J Phys Conf Ser. 1003:012083. doi:10.1088/1742-6596/1003/1/012083.
- Nandi S, Swain S. 2022. Analysis of heatwave characteristics under climate change over three highly populated cities of South India: a CMIP6-based assessment. Environ Sci Pollut Res. 30(44):99013–99025. doi:10.1007/s11356-022-22398-x.
- NASA. 2023. Global climate change: vital signs of the planet. [accessed 2023 Mar 12]. https://climate.nasa.gov/.
- Needle F. 2020. How to use regression analysis to forecast sales: a step-by-step guide. [accessed 2021 Jul 15]. https://blog.hubspot.com/sales/regression-analysis-to-forecast-sales?toc-variant-a=.
- Nimish G, Bharath HA, Lalitha A. 2020. Exploring temperature indices by deriving relationship between land surface temperature and urban landscape. Remote Sens Appl Soc Environ. 18:100299. doi:10.1016/j.rsase.2020.100299.
- Nimish G, Chandan MC, Bharath HA. 2018. Understanding current and future landuse dynamics with land surface temperature alterations: A case study of Chandigarh. ISPRS Ann Photogramm Remote Sens Spatial Inf Sci. IV-5:79–86. doi:10.5194/isprs-annals-IV-5-79-2018.
- Pinty B, Verstraete MM. 1992. GEMI: a non-linear index to monitor global vegetation from satellites. Vegetatio. 101(1):15–20. doi:10.1007/BF00031911.
- Ramachandra TV, Bharath HA, Sanna DD. 2012. Insights to urban dynamics through landscape spatial pattern analysis. Int J Appl Earth Obs Geoinf. 18:329–343. doi:10.1016/j.jag.2012.03.005.
- Ranjan AK, Anand A, Kumar PBS, Verma SK, Murmu L. 2018. Prediction of land surface temperature within Sun City Jodhpur (Rajasthan) in India Using integration of artificial neural network and geoinformatics technology. Asian J Geoinform. 17(3):14–23.
- Rehman A, Qin J, Pervez A, Khan MS, Ullah S, Ahmad K, Rehman NU. 2022. Land-use/land cover changes contribute to land surface temperature: a case study of the Upper Indus Basin of Pakistan. Sustainability. 14(2):934. doi:10.3390/su14020934.
- Sang L, Zhang C, Yang J, Zhu D, Yun W. 2011. Simulation of land use spatial pattern of towns and villages based on CA–Markov model. Math Comput Modell. 54(3-4):938–943. doi:10.1016/j.mcm.2010.11.019.
- Shabri A, Samsudin R. 2014. Daily crude oil price forecasting using hybridizing wavelet and artificial neural network model. Math Prob Eng. 2014:1–10. doi:10.1155/2014/201402.
- Singh P, Kikon N, Verma P. 2017. Impact of land use change and urbanization on urban heat island in Lucknow city, Central India. A remote sensing based estimate. Sustain Cities Soc. 32:100–114. doi:10.1016/j.scs.2017.02.018.
- Somvanshi SS, Kumari M. 2020. Comparative analysis of different vegetation indices with respect to atmospheric particulate pollution using sentinel data. Appl Comput Geosci. 7:100032. doi:10.1016/j.acags.2020.100032.
- Sreehari E, Ghantasala PG. 2019. Climate changes prediction using simple linear regression. J Comput Theor Nanosci. 16(2):655–658. doi:10.1166/jctn.2019.7785.
- Sreehari E, Srivastava S. 2018. Prediction of climate variable using multiple linear regression. In: 2018 4th International Conference on Computing Communication and Automation (ICCCA), Greater Noida, India. IEEE. pp. 1–4. doi:10.1109/CCAA.2018.8777452.
- Stepchenko A. 2016. NDVI index forecasting using a layer recurrent neural network coupled with stepwise regression and the PCA. Paper presented at the 5th Virtual International Conference of Informatics and Management Sciences. Zilina, Slovakia. p. 130–135 doi:10.18638/ictic.2016.5.1.281.
- Sun Z, Wang C, Guo H, Shang R. 2017. A modified normalized difference impervious surface index (MNDISI) for automatic urban mapping from Landsat imagery. Remote Sensing. 9(9):942. doi:10.3390/rs9090942.
- Tadese S, Soromessa T, Bekele T. 2021. Analysis of the current and future prediction of land use/land cover change using remote sensing and the CA-markov model in Majang forest biosphere reserves of Gambella, southwestern Ethiopia. Sci World J. 2021:1–18. doi:10.1155/2021/6685045.
- Taloor, A. K., Manhas, D. S., & Kothyari, G. C. (2021). Retrieval of land surface temperature, normalized difference moisture index, normalized difference water index of the Ravi basin using Landsat data. Applied Computing and Geosciences, 9, 100051. doi:10.1016/j.acags.2020.100051.
- Tan J, Yu D, Li Q, Tan X, Zhou W. 2020. Spatial relationship between land-use/land-cover change and land surface temperature in the Dongting Lake area, China. Sci Rep. 10(1):9245. doi:10.1038/s41598-020-66168-6.
- Tariq A, Shu H. 2020. CA-Markov Chain analysis of seasonal land surface temperature and land use land cover change using optical multi-temporal satellite data of Faisalabad, Pakistan. Remote Sensing. 12(20):3402. doi:10.3390/rs12203402.
- Tian L, Tao Y, Li M, Qian C, Li T, Wu Y, Ren F. 2023. Prediction of land surface temperature considering future land use change effects under climate change scenarios in Nanjing City, China. Remote Sensing. 15(11):2914. doi:10.3390/rs15112914.
- Tin SN, Muttitanon W. 2021. Analysis of Enhanced Built-up and Bare Land Index (EBBI) in the urban area of Yangon, Myanmar. IJG. 17(4):83–94. doi:10.52939/ijg.v17i4.1957.
- Tran DX, Pla F, Latorre-Carmona P, Myint SW, Caetano M, Kieu HV. 2017. Characterizing the relationship between land use land cover change and land surface temperature. ISPRS J Photogramm Remote Sens. 124:119–132. doi:10.1016/j.isprsjprs.2017.01.001.
- UN DESA. 2018. 2018 Revision of world urbanization prospects. [accessed 2018 Sept 27]. https://www.un.org/development/desa/publications/2018-revision-of-world-urbanization-prospects.html.
- UN DESA. 2019. 2018 Revision of world urbanization prospects. [accessed 2021 Dec 5]. https://population.un.org/wup/Publications/Files/WUP2018-Report.pdf.
- Vandansambuu B, Davaa T, Gantumur B, Purevtseren M, Lkhagva O, Wu F. 2020. Spatiotemporal monitoring and prediction of land use/land cover changes using CA-Markov chain model: a case study in Orkhon Province, Mongolia. Remote Sens Technol Appl Urban Environ. 11535:115350E. doi:10.1117/12.2574032.
- Yang L, Qian F, Song D, Zheng K. 2016. Research on urban heat-island effect. Paper presented at 4th International Conference on Countermeasures to Urban Heat Island, Singapore. doi:10.11648/j.ijema.20150302.15.
- Yang X, Zheng XQ, Chen R. 2014. A land use change model: integrating landscape pattern indexes and Markov-CA. Ecol Modell. 283:1–7. doi:10.1016/j.ecolmodel.2014.03.011.
- Yirsaw E, Wu W, Shi X, Temesgen H, Bekele B. 2017. Land use/land cover change modeling and the prediction of subsequent changes in ecosystem service values in a coastal area of China, the Su-Xi-Chang Region. Sustainability. 9(7):1204. doi:10.3390/su9071204.
- Zhang G, Patuwo BE, Hu MY. 1998. Forecasting with artificial neural networks: the state of the art. Int J Forecast. 14(1):35–62. doi:10.1016/S0169-2070(97)00044-7.
Appendix A
Land use for study area
Land surface temperature for study area
Table A1. Statistical analysis of LST for study area.
Appendix B
Table