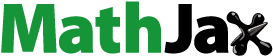
Abstract
The rapid urban growth and anthropogenic activities have posed a threat to the local environment and ecosystem around the world. This situation has become a hindrance to planners and policy makers for sustainable urban development. Therefore, this study mainly focuses on the assessment of urban growth patterns in relation to urban sprawl in Jalpaiguri urban agglomeration. Multi-temporal Landsat data have been used for land use change detection and urban sprawl quantification. The maximum likelihood classifier technique has been performed to create land use land cover maps for each study year (2001, 2011 and 2021). Urban expansion intensity index has been applied to determine the magnitude of urban expansion. Landscape metrics and Shannon’s entropy have been employed to assess the urban sprawl to a spatial extent. Spatiotemporal land use changes reveal that the non-urban class (vegetation, agriculture, water bodies, and fallow) have been decreasing consistently with an increase in built-up areas over time. Built-up area has increased by almost seven times in the span of the last 20 years (2001–2021). In the first decade, the growth rate of urban areas was 145.42% with a medium speed of expansion and in the next decade, it was 180.83% with a very high speed. Landscape metrics show that the fragmentation of the entire urban landscape into small patches happened from 2001 to 2011 in a higher magnitude indicating the occurrence of sprawling characteristics. But in recent times, the entire landscape is aggregating into large single urban patches which indicate a clumpy situation and would affect the local ecological environment. Shannon’s entropy model also verifies the compact urban sprawl in different directions and distances from the city centre. The understanding of urban growth dynamics and land use changes is essential for addressing the rapid urbanization within this urban region. There is an immediate need for an appropriate strategy for effective utilization of land use and monitoring of uncontrolled and haphazard urban growth. This research study would help the urban planner to take a specific scope of action for future urban growth and development.
1. Introduction
Over the recent decades, the developing countries have been experiencing rapid urbanization and development that results in an unexpected increase of urban population (Sun et al. Citation2020; Pande et al. Citation2023). India, as one of the world’s fastest growing urbanized countries, has seen the same trend in dynamic growth of urban population (Tumbe Citation2016). According to a recent United Nations report, there is now 55% of the world’s population living in urban areas, and a proportion which is set to reach 68% by 2050 if the current trend continues. The 2018 Revision of World Urbanization Prospects stated that India, China and Nigeria will collectively account for a growth rate of 35% of the world’s projected urban population during the 2018–2050 time periods. In India, the number of urban dwellers is predicted to rise to 416 million by 2050 (Ritchie and Roser Citation2018). Rapid growth of population and migration are inadvertently leading to urbanization in India (Varkey and Manasi Citation2019; Roy et al. Citation2022). In urbanization, people concentrate into discrete areas, and transformation of a rural area into an urban one occurs (Brown and Schafft Citation2011). The urban growth that results from urbanization involves intensive land use (Patra et al. Citation2018). In a more precious way, urban growth means the process of growth rate of population as well as area of urban land (Bhatta Citation2010). It usually changes the morphology of a town or city (Zhou and Chen Citation2018). Moreover, the ever increasing population leads to the rise in unplanned urban land growth in the suburbs of the city, which is usually termed as urban sprawl (Bugliarello Citation2003). It usually consists of some type of development which is characterized as farmland loss, deforestation, haphazard urban expansion, growth along major routes, traffic congestion, and loss of local water bodies and wetlands, open space, ecologically sensitive habitats etc. (Sudhira et al. Citation2003; Aithal et al. Citation2018; Al-Kofahi et al. Citation2018; Rahaman et al. Citation2019; Chettry and Surawar Citation2020). In simpler words, the boundary of an urban region or city expands as its population increases to accommodate the growth; this expansion is known as sprawl (Sudhira et al. Citation2003). Urban sprawl is related to unplanned and unmanaged urban land development. Hence, urban sprawl is a dynamic phenomenon (Batty et al. Citation1999; Torrens and Alberti Citation2000; Sudhira et al. Citation2004). It is defined as a dispersion of spatial expansion of the city or town towards its peripheral and sub-urban areas (Galster et al. Citation2001; Tewolde and Cabral Citation2011). Experts have different opinions about the causes of sprawl, as its patterns differ by characteristics (Habibi and Asadi Citation2011). Typically, urban sprawls occur at the edges of urban areas, along the highways, or at the urban fringe; and the distinct patterns of sprawl development are ribbon on strip, leapfrog (isolated), and low density (radial) sprawl (Barnes et al. Citation2001; Sudhira et al. Citation2003). Infill growth and intensive land use nearer to urban centres are encouraged by compact urban growth, while dispersed urban growth encourages uncontrolled urban growth outside the existing built-up areas (Qing et al. Citation2020; Yılmaz and Terzi Citation2020; Sapena and Ruiz Citation2021). Expanded urbanized areas and associated changes in existing land use land cover (LULC) have a negative impact on the natural resources and environment (Alphan Citation2003; Mosammam et al. Citation2017; Sumari et al. Citation2020). These phenomena are remarkably dynamic and visible in Asian countries (Zhao et al. Citation2020). Likewise, the ecosystem services related to urban sprawl had certain peculiarities, both spatially and functionally in Central and Southeast European states (Sýkora and Ourednek Citation2007; Grigorescuet al. Citation2012; MITRIC et al. Citation2014). African cities have also been experiencing rapid urban growth and sprawl and its impact on the urban environment for the last few decades (Sumari et al. Citation2020; Shao et al. Citation2021). However, African cities are less compact by urban land than the medium and large sized cities in Asia, Europe and North America (Xu et al. Citation2019). Urban sprawl phenomenon has been discussed worldwide and became relevant to contemporary urban planners and researchers (Johnson Citation2001; Colsaet et al. Citation2018; Saini and Tiwari Citation2020). Researchers have been introduced several new approaches and techniques in recent decades to analyse and monitor urbanization and its emerging issues in terms of sustainable urban growth and structure over spatial context (Ismael Citation2021). Remote sensing (RS) and geographic information system (GIS) techniques have been frequently used to critically investigate different aspects of urban growth and patterns of sprawl (Sudhira et al. Citation2003; Rahman et al. Citation2011; Rahaman et al. Citation2019). However, it has been analysed effectively using Shannon’s entropy and landscape metrics by integrating with RS and GIS techniques, and statistics in many studies. Based on these two approaches, monitoring and modelling of urban growth dynamics were used by several researchers over different cities worldwide (Sudhira et al. Citation2004; Aguilera et al. Citation2011; Ramachandra et al. Citation2014; Tewolde and Cabral Citation2011). Shannon’s entropy is used to quantify the degree of compactness or dispersion of a certain geophysical variable like built-up area in a spatial unit (Lata et al. Citation2001; Jat et al. Citation2008; Bhatta Citation2012). While, landscape metrics help to understand and quantify the urban patterns in terms of spatial configuration and heterogeneity of landscape (McGarigal and Marks Citation1995; Herold et al. Citation2002; Hassan Citation2017). Empirical studies illustrated that as the consequences of rapid urbanization and development, Indian megacities such as Mumbai, Delhi, Kolkata, and Chennai have rapidly expanded and sprawl occurred among them (Ghosh and Das Citation2017). This sprawling encourages the surrounding rural people to move into the urban areas or to get the urban amenities and services nearer to the urban body. It gradually encroaches and merges the adjacent rural areas and smaller urban bodies with the main urban unit (Shaw and Satish Citation2007; Bhatta Citation2010). Besides, many researchers have shown their interest in urban expansion and studied effectively the significant LULC change and urban growth in relation to sprawling characteristics in the Indian mid-sized and smaller cities like municipalities, municipal corporations, urban agglomeration, special planning areas etc.
Lata et al. (Citation2001) carried out work on the spatial processes in land development in Hyderabad city, India, and its environment by integrating with RS and GIS. Shannon’s entropy approach was also adopted to quantify urban sprawl patterns at various land use zones. Jat et al. (Citation2008) in their research examined the dynamics of spatial urban form or impervious area of Ajmer City in India using satellite images. Landscape metrics and Shannon’s entropy model were used for the spatial distribution of urban sprawl. Bhatta (Citation2012) identified and analysed the pattern of urban growth of Kolkata in India. Shannon’s entropy combined with several statistical methods was applied to understand the growth pattern which shows dispersed development of built-up land. Rahman et al. (Citation2011) investigated significant urban sprawl in Hyderabad-Secunderabad twin city and its surrounding area. They used Shannon’s entropy model to assess the city growth and its direct impact on existing LULC. Urban sprawl resulted in remarkable loss of agricultural lands, green spaces and surface water bodies. Shetty et al. (Citation2012) in their research used landscape metrics to depict the changing extent of land cover in Bangalore city, India. The research also explores the rapidly changing scenario of land cover and its adverse impact on the landscape elements in geographic extent. Ramachandra and Aithal (Citation2013) focused on urban growth dynamics as a consequence of land use changes of Raichur City in India. They adopted Shannon’s entropy to assess the sprawl in and around the city. Landscape metrics also indicated a convoluted and a single patch urban growth at the centre and a fragmented outgrowth at the periphery of the city. Das Chatterjee et al. (Citation2016) also carried out a research on application of landscape metrics and Shannon’s entropy in assessment of urban sprawl patterns in Greater Bhubaneswar city, India. They provided a quantitative measurement for rapidly growing urban regions in quantifying the dynamics of urban sprawl in spatial form. Dutta and Das (Citation2019) in their study measured the extent and magnitude of urban sprawl by employing the Shannon’s entropy index and landscape metrics analysis in remote sensing and GIS environments. They also predicted the future urban growth areas based on the sprawling magnitude in different directions. Thakur et al. (Citation2020) have explained urban dynamics using Shannon’s entropy index and landscape metrics in order to measure the urban growth using built-up area as a spatial unit. They also introduced statistical techniques to explore the association of dynamic urban growth with its contributory factors. Das and Angadi (Citation2021) have attempted a study on spatial modelling in the quantification and monitoring of LULC changes in Barrackpore sub-divisional region, India. Shannon’s entropy and landscape metrics revealed and verified urban sprawl patterns and significance changes in LULC. Shikary and Rudra (Citation2021) used Shannon’s entropy to measure urban land use change and sprawl in Purulia Municipality of West Bengal. They examined compact sprawling and the built-up class is rapidly decreasing from the core area to town outskirts due to increasing distance.
The cities in India are sprawling very rapidly (Das and Angadi Citation2021). Due to the exceeding carrying capacities of the larger Indian cities, future demand for urban growth is likely to happen in smaller or mid-sized cities (Mitra and Mehta Citation2011). For the management of predicted future urban growth and its development in a sustainable manner, it is essential to assess and understand the current status of land use. Therefore, the monitoring of spatiotemporal LULC changes can help the authorities and planners to understand the city growth and structure and can make a sustainable city plan for overall urban development (Patra et al. Citation2018; Sumari et al. Citation2019; Mallick et al. Citation2021). Therefore, the objective of this study is to analyse the urban growth patterns in relation to urban sprawl using temporal remotely sensed data from 2001 to 2021 in GIS environment by integrating with Shannon’s entropy and landscape metrics. Jalpaiguri urban agglomeration is one of the important urban region in eastern Himalayan foothills. The location and accessibility make this region as corridor to country’s north-eastern parts and neighbouring countries (Bhutan and Bangladesh). This urban region is a Class-I urban agglomeration in India and has experienced huge land use dynamics and urban growth in various land use sites in the last two decades (2001–2021). Factors like growing population and settlement pressure on land resources; expansion of highways and development of overall accessibly (by both roadways and railways); growing of industries (tea, cement industry); allocation of infrastructure and public service centres (super speciality hospital, medical college, circuit bench, schools, shopping malls, automobile); economic activities etc. have made a huge transition of vegetation, agricultural land, fallow and water bodies into built-up items in this urban region. This situation has created sprawling characteristics of this urban body and made attention on adverse impact of urban growth on ecosystem services. Therefore, it is important to identify how urban spatial growth has been occurred during the period of urban expansion at different directions and distances from city centre. Literature survey established that the growth dynamics in urban land development and urban sprawl in this urban region have not been investigated earlier. The function and role of India’s smaller or middle sized cities are different from that of large cities. This study may help to encourage future research interest in geospatial domains on characteristics of urban growth in small urban regions like Jalpaiguri urban agglomeration. To understand the urban growth dynamics and characteristics, this study used two different mythologies, i.e. Landscape metrics and Shannon’s entropy model, which enhanced the results of the investigation. Therefore, this research work attempts an advanced examining of urban expansion to the spatial extent and may provide a visualization and quantification of expanding urban footprint to local authorities for future planning.
2. Study area
Jalpaiguri urban agglomeration is the only urban agglomeration (UA) in the district of Jalpaiguri, a northern district of the state West Bengal (). This UA is one of the important urban local bodies, political and cultural centre in Jalpaiguri district as well as North Bengal covering an area of 44.44 sq.km which lies at 26°28′09′′ to 26°34′19′′ North latitude and 88°39′41′′ to 88°45′05′′ East longitude with an average altitude of 89 meters. This urban region is located on the river Teesta’s western bank. Geomorphologically, the region is characterised as an alluvial plain with a very low lying slope. Kerala is the main river of this town, which runs through its middle part from north-west to south-east direction and finally it meets river Teesta about 5.5 km. from the city centre. The annual rainfall of this region is 3500 mm; and the maximum and minimum temperature are 38 °C in June and 9 °C in January, respectively.
This urban region got its recognition of UA with a status of Class-I UA in India in the latest census in 2011. It consists of Jalpaiguri Municipality and Kharia Census Town. Jalpaiguri district is the sub-divisional headquarter of districts in North Bengal. The oldest municipality in the district is Jalpaiguri Municipality which was established in 1885. The district headquarter is also located in Jalpaiguri town. The population of the municipality was 107,341 persons in 2011, which fall in the Class I category as per the Census classification of town in India. Jalpaiguri district experienced rapid urbanization during the decade between 2001 and 2011 census and consequently, the number of Census Towns (CT) also jumped to 35 in 2011 from only 13 in 2001. Kharia, the peripheral mouza of Jalpaiguri Municipality also got its recognition as a CT in 2011. Now it is a fast growing urbanizing mouza in the district. It had a population of 61,661 persons with a status of Class-II urban centre in India in 2011. Besides, different socio-economic factors also played an important role in the emergence of Jalpaiguri UA in 2011. As per the report of the 2011 census, the sex ratio (no. of females per thousand males) and literacy rate were 978 and 84.87%, respectively, in this UA. The work participation rate of the UA was 37.51% and among them, 89.38% of workers were engaged in the household industry and other workers (excluding cultivators and agricultural labourers) (censusindia.gov.in). As the recent census (after 2011) report has not yet been published by Indian government, it is difficult to relate different socio-economic aspects regarding urban growth with recent time scenarios. Presently, it has a projected population size of approximately 231,000 persons which was 169,002 in 2011 and 150,458 in 2001. Major land use of this UA area is characterised by extensive agricultural fields, vegetation with smaller patches of tea gardens, open fallows, and built-up areas. The UA is well connected by both highways and railways. The National Highway (NH) 31 connects the town via State Highway (SH) 12 A, which creates the township as a corridor to India’s north-eastern part, neighbouring country Bhutan and network to other NHs. The Haldibari SH passes through the township in a north to south direction and connects the UA with the international (Indo-Bangladesh) border approximately 19 km. from the township. Similarly, two railway stations, i.e. Jalpaiguri Town Station (inside the municipality) and Jalpaiguri Road Station (outside the municipality) provide railway communication services to the people in this township. The railways connect this urban area with the State Capital, National Capital and the country’s North-Eastern states. Strategically, the Jalpaiguri-Haldibari railway connection is another transport communication between these two towns and the Indo-Bangladesh commercial channel via Haldibari.
3. Data and methodology
Jalpaiguri urban region has been experiencing rapid urban growth for the last two decades. The growth of population and its pressure has accelerated the development of new built-up items over existing buildable land. As a result, unplanned and haphazard urban growth (sprawling characteristics) has occurred throughout the township. The core area of this urban region has become more compact by urban land due to higher built-up density and lack of buildable land. This situation has created clumpy urban growth inside the main town and encouraged urban expansion at the fringe area. Huge alteration of natural land uses was also affected by this expansion of urban land to a spatial extent. This growing unplanned urban growth creates a limit to sustainable urban development in accordance with socio-economic and environmental aspects.
Information on the formation of Jalpaiguri UA has been collected from the Population Census (Citation2011) conducted by the Census Organization of India (censusindia.gov.in). Maps of Khariamouza and Jalpaiguri Municipality were collected from the Office of the District Magistrate, Jalpaiguri; and the Office of the Jalpaiguri Municipality, Jalpaiguri, respectively. Since the area of interest (AOI) is not readily available, it has been digitized in the GIS environment based on the municipality and mouza map. Temporal population data have been collected from the District Census Handbook of Jalpaiguri district (censusindia.gov.in). Three Landsat satellite images, i.e. Landsat 5 for the years 2001 and 2011 and Landsat 8 for 2021 were obtained from the official website (https://earthexplorer.usgs.gov/) of the United States Geological Survey (USGS) for quantifying the land use dynamics and pattern of urban growth and its expansion characteristics in spatial context. The entire study area falls under a single scene with Landsat Path/Row of 139/042. The table below contains the details of the Landsat imageries (). The methodology along with the entire study has been completed in a systematic manner which is shown in a flowchart below (). Microsoft Excel 2007, ArcGIS 10.3, and FRAGSTATS 4.2.1 have been utilized for analysis and representation of data and findings.
Table 1. Descriptions of selected satellite images.
3.1. Landsat image processing and classification
Landsat images of 2001, 2011 and 2021 were utilized to quantify the land use dynamics and pattern of urban expansion. These three images were taken in different dates as well as different times of a date with a minimal alteration in the radiometric and geometric accuracy. Further rectification of the images is needed in this regard.
3.1.1. Pre-processing of images
The main objective of pre-processing the Landsat images is to get more accuracy in the outputs. The images were pre-processed with a sequence of adjustments and corrections. The acquired images (Landsat 5) used in this work were corrected radiometrically and geometrically. The obtained images are pre-geo-referenced and images were projected to the UTM zone 45 north using the reference datum of WGS 1984.
3.1.2. Processing of images and classification
Layer stacking process was performed to prepare a multi-bands image from the corrected spectral bands (optical). The processed images were clipped by AOI, the boundary area of Jalpaiguri UA. Then the subset composite images were used as the inputs in supervised classification. This classification method is a technique based on decision rules. Samples are taken with known features in the field and compared with spectral signatures of pixels in the input images. These image classifications were performed using a widely used maximum likelihood classifier technique (Settle and Briggs Citation1987; Liang et al. Citation2022). For multi-temporal classification of images, this classifier is a reliable and probability based algorithm for classification (Strahler Citation1980). The main objective of this classification is to understand the spatiotemporal changes in LULC with five major categories, i.e. agricultural land, built-up land, fallow land, vegetation area and water bodies. The signatures for the particular class were tabulated and performed the classification based on the classification system of Anderson et al. (Citation1976). Thus, LULC with five major classes (built-up land, agricultural land, fallow land, vegetation area and water bodies) was prepared for each study year (2001, 2011 and 2021). The statistics of each LULC item has been quantified for changes in LULC over time. Land areas with residential; commercial services; industrial; transportation, communications, and utilities; industrial and commercial complexes; mixed built-up land; and other urban or built-up land have been considered under urban or built-up land class (Anderson et al. Citation1976). Areas other than these urban or built-up items have been considered as non-urban or non-built-up land (agricultural land, fallow land, vegetation area, and water bodies). The urban or built-up land areas were extracted from each LULC classification for different study years. The extracted urban or built-up land features in 2001, 2011 and 2021 and their respective statistics have been used for further discussion.
3.1.3. Accuracy assessment
Accuracy assessment is a vital part that compares classified images with ground truth scenarios to validation. It was applied to estimate how accurately the pixels are performed for individual feature classes (Khatami et al. Citation2017; Shukla and Jain Citation2019). This accuracy assessment of the classifications can be addressed by the global positioning system (GPS) technique and ‘historical view’ of Google earth. For 2021, 130 ground control points were taken from the field using GPS. Based on reference points for each classification year, four parameters were established for accuracy assessment, i.e. product’s accuracy (error of omission), user’s accuracy (error of commission), overall accuracy, and Kappa coefficient (Story and Congalton Citation1986; Foody Citation2002). Thus, validation of classification was performed with an acceptable accuracy rate.
3.2. Inter-cardinal directions and concentric buffer zones creation
Kadamtala is the central business district of Jalpaiguri urban region, where Kadamtala More is considered as the city centre. City centre of an urban body is the first settled (often historical) place wherethe greater concentration of city’s economy, socio-cultural and political power are exhibited. Therefore, it is important to examine the expansion of urban development at different directions and distance from the city centre (first settled place) over time. For this investigation, the entire study area was divided into four inter-cardinal directions and seven concentric buffer zones from the city centre. For creating divisions, the entire agglomeration area was divided into four inter-cardinal directions, i.e. north-west (NW), north-east (NE), south-west (SW), and south-east (SE) to determine the significant growth direction. Furthermore, considering the farthest boundary distance of the study area from the central point (Kadamtala more), seven buffer zones with a radius of 1 km for zone 1, and then a 1 kilometre common interval for the rest zones (zone 2 to zone 7) were created within the study area boundary limit. Both inter-cardinal direction and buffer zone creation processes were performed using ArcGIS 10.3. Extracted built-up feature classes from each classification (2001, 2011, and 2021) were used for deeper investigation of spatial urban growth at different directions and distances from the city centre.
3.3. Urban expansion intensity index (UEII)
In the urbanization process, the expansion of urban growth is not uniform in each direction as well as each zone due to different urban drivers and their spatial impacts. UEII is a quantitative approach to assess and analyse the spatial expansion differences in urban growth. It helps to recognize the urban growth preference in a specific period (Ren et al. Citation2013). The index reflects the potential of expansion of urban areas and its probable future directions. It also compares the intensity or speed of urban spatial growth on a temporal basis (Al-Sharif et al. Citation2014). The UEII standard has five divisions of values and the value of the index ranges between 0 and >1.92. The division standards are as follows: 0–0.28 indicates slow development, 0.28–0.59 indicates low speed, 0.59–1.05 indicates medium speed, 1.05–1.92 indicates high speed, and >1.92 indicates very high speed development. Expansion intensity can be calculated using EquationEquation 1(1)
(1) .
(1)
(1)
where UEIIit represents the annual average UEII of the ith spatial zone in time period t; ULAi,a and ULAi,b represent the built-up area quantities at time period a and b in the ith spatial zone, respectively; and TLAi represents the total area of ith spatial zone.
3.4. Landscape metrics
The landscape is a geographical area that has a spatial heterogeneity of variables of interest (Wu Citation2013). In order to fully understand landscape, landscape fragmentation (breaking-up of landscape into smaller patches), landscape pattern (composition and configuration of elements), landscape structure (composition and arrangement of elements including patches, corridors in spatial context, and matrix), landscape dynamics (structural and functional changes on temporal basis) are the prime aspects of a landscape which are driven by both natural and man-made processes.
Landscape metrics are based on information theory and fractal geometry concepts (Frazier and Kedron Citation2017). The main purpose of using landscape metrics is that these metrics are the quantitative indices that describe landscape structures and patterns (McGarigal and Marks Citation1995; Herold et al. Citation2002). In an attempt to fully describe urban processes and models, the use of RS alone is a bit incomplete. Therefore, the addition of landscape metrics enhances its results (Berila and Isufi Citation2021). These metrics are used to have a clear and unambiguous understanding of the urban landscape and its characteristics in order to sustainably manage urban environments (Aguilera et al. Citation2011). Some most frequently used metrics at class-level and landscape-level were computed for all the classified images to quantify urban landscape dynamics. Thus, three areas and edges (Class area, Percentage of land, and Edge density), one shape (Area weighted mean patch fractal dimension), two aggregations (Patch density, and Landscape shape index), and one diversity landscape metric (Simpson’s diversity index) were selected to understand landscape dynamics and were computed using the FRAGSTATS 4.2.1 program of spatial pattern analysis (McGarigal and Marks Citation1995). The details of these seven landscape metrics are listed in , and their results are shown in and .
Figure 3. Different landscape metrics in 2001, 2011 and 2021: (a) CA, (b) PLAND, (c) ED, (d) FRAC_AM, (e) PD, (f) LSI, (g) SIDI.

Table 2. Description of selected landscape metrics.
Table 3. Landscape metrics of built-up class in Jalpaiguri UA and its different directions.
3.5. Shannon’s entropy model
Simply entropy represents the degree of randomness or uncertainty in the system. Shannon’s entropy or information entropy concept was proposed by Shannon as a concept based on which a measure of uncertainty about the occurrence of a certain event can be calculated (Shannon Citation1948). Shannon’s entropy model is a common and widely used technique of measuring the magnitude of growth of an urban body in a region using RS and GIS (Lata et al. Citation2001; Tewolde and Cabral Citation2011; Mosammam et al. Citation2017). It measures the degree of concentration or dispersion of any geographical variable in any spatial unit (Sudhira et al. Citation2004; Bhatta Citation2012). The model’s structure can be calculated by applying EquationEquation (2)(2)
(2) below.
(2)
(2)
(3)
(3)
where Hn is the Shannon’s entropy, Pi is the proportion of the variable (built-up item) in ith zone, xi is the observed value of the variable and n is the total number of zones in the study region.
This model is used to get the results of relative entropy which has been applied to understand the change in expansion of built-up land whether it is compact or dispersed. The relative entropy value varies from 0 to 1. The value nearer to zero (0) denotes the compact distribution of built-up land, and the value nearer to one (1) denotes the dispersed distribution. Urban sprawl is indicated by dispersion (unevenly dispersed distribution), i.e. the higher values of entropy (Bhatta Citation2012). The halfway value of relative entropy is measured as a threshold. Hence, if the value is higher than that, the urban body is experiencing sprawl or huge expansion with unevenly dispersed spatial distribution of built-up land (Das Chatterjee et al. Citation2016; Das and Angadi Citation2021). The relative entropy for n number of zones can be calculated using EquationEquation 4(4)
(4) (Thomas Citation1981).
(4)
(4)
where H1 is the relative entropy, Hn is the absolute entropy and n is the total number of zones. Entropy value 0.5 is considered as threshold value, and the value higher the threshold is considered as urban sprawl with dispersed physical expansion of built-up land (Das Chatterjee et al. Citation2016).
To measure the change rate of sprawl or magnitude of change in sprawling, dispersion between two time periods is calculated using EquationEquation 5(5)
(5) .
(5)
(5)
where ΔH1 is the disparity of relative entropy value between two time periods, H1(t1) is the relative entropy at time t1 (initial), and H1(t2) is the relative entropy at time t2 (final) (Sun et al. Citation2007).
4. Results
4.1. The spatiotemporal LULC changes
Land use classifications of the UA from 2001 to 2011 and 2011 to 2021 are shown in both the table and figure format (; ). The results of the classification indicated that vegetation, water bodies, fallow or open land and agricultural or cropland areas have declined from 2001 to 2021 and on the other hand, built-up land has increased during the decades. The overall accuracy results of classified output images were 87.28% (2001), 90.0% (2011) and 91.55% (2021). Kappa coefficients of the classified images of 2001, 2011 and 2021 are 0.84, 0.88, and 0.89, respectively, which denotes a very good agreement between the classified images and ground truth. The land use classification outputs of the agglomeration have been carried out into five sections (UA and its directional zones, i.e. NE, SE, SW, and NW).
Table 4. LULC changes of classes (area in sq.km) in Jalpaiguri UA and its different directions.
4.1.1. Jalpaiguri UA
The temporal LULC changes show that built-up has increased tremendously in the agglomeration area. The area was mainly covered by agricultural fields and vegetation cover in 2001. Vegetation cover and surface water bodies were converted into built-up land, agricultural land and open fallow in some places of the agglomeration area in 2011. But eventually, in 2021, the built-up land had got a massive increase in areas over other land use forms due to impact of growing urbanization on land use. Kharia, the peripheral rural mouza of Jalpaiguri municipality, had got the recognition of urban body as new CT in 2011, and the UA was developed at the same time. Thereafter, the entire urban region has faced crucial changing aspects of LULC dramatically. Built-up land was only 3.06 sq.km (6.26%) in 2001 and then rapidly increased to 7.51 sq.km (15.36%) in 2011 and 21.09 sq.km (43.15%) in 2021 (; ). Agricultural land and fallow land were also increased in areas of 20.60 (42.14%) to 21.59 sq.km (44.17%) and 7.08 (14.48%) to 7.13 sq.km (14.58%), respectively, from 2001 to 2011 and then decreased to 13.63 sq.km (27.88%) and 4.49 sq.km (9.18%), respectively, in 2021. The area of vegetation cover was declined by 16.81 (34.40%) to 11.77 sq.km (24.07%) from 2001 to 2016 and then again sharply decreased by 8.88 sq.km (18.17%) in 2021. Similarly, the proportion of water body to total area was also gradually reduced by 1.33 (2.72%) to 0.88 sq.km (1.80%) from 2001 to 2011 and then again declined by 0.79 sq.km (1.62%) in 2021 across the agglomeration area. So, the overall status of LULC classification depicts that during the subsequent years, 2001 to 2021, a substantial increase in built-up land have recorded and contrarily, the area of other classified land use items have declined at the same time period.
4.1.2. NE Zone
NE Zone is the north-east directional zone from the city centre. This zone has experienced significant changes in fallow land and vegetation into built-up land (; ). In 2001, the proportion of fallow and vegetation to total land area was 45.91% (4.44 sq km) and 31.13% (3.01 sq km), respectively. The fallow land was reduced by 36.72% from 2001 to 2011 and 27.70% of the land from 2011 to 2021; and similarly, vegetation cover was also sharply decreased by 16.23% from 2001 to 2011 and 14% of the land from 2011 to 2021.On the other hand, the area of agricultural land and water body were increased from 2001 to 2011; and then, reduced again in 2021. Temporary local water bodies were created due to the construction of road connectivity and other residential activities during 2001–2011. As a result, the area of surface water bodies was increased from 2.14% in 2001 to 3.65% in 2011; and thereafter, again reduced by 3.03% coverage of the land in 2021. Similarly, vegetation cover and fallow land were transformed into agricultural land in some places during 2001–2011; and then, converted into built-up items from 2011 to 2021. The area covered by agricultural land was 13.47% in 2001, 23.78% in 2011 and 8.53% in 2021. The built-up land was increased by 19.63% in 2011 from 7.35% in 2001; and 46.73% in 2021 from 2011 due to rising demand on land use by urban growth process.
4.1.3. SE Zone
SE Zone is covered in the south-eastern part of the agglomeration area from the city center. This area has also experienced a huge transition of vegetation cover and agricultural land into built-up land (; ). Agricultural land was occupied by 36.36% of the land in 2001, 30.02% in 2011, and finally, 15.88% in 2021. Similarly, the area of land covered by vegetation was 33.43% in 2001, 13.04% in 2011, and finally, 18.35% in 2021. Open or fallow land was increased from 11.05% to 29.24% during 2001–2011 due to loss of vegetation cover along with agricultural fields for residential/settlement planning to accommodate the population of this zone. Local water bodies also disappeared during 2001–2011 and the respective area reduced by 3.41% in 2011 from 5.95% in 2001. Among other land use types, the changing status of built-up land is in a positive and consistent way and the area covered by built-up was 13.21% in 2001, 24.29% in 2011, and 49.81% in 2021.
4.1.4. SW Zone
SW Zone is the south-western zone from the city center of the study area. This zone is mainly covered by crop fields or agricultural land followed by vegetation (; ). This area is dominated by primary economic activities rather than secondary and tertiary. Therefore, comparatively less amount of built-up area has developed across the zone. Only 1.63% of the land was covered by built-up in 2001 and then, it was 6.33% in 2011. During the last decade (2011–2021), significant transformation of agricultural land into built-up has occurred in the inner part of the zone, and built-up area was increased by 30.21% in 2021. However, built-up land has occupied 6.41 sq.km areas with a built-up density of 0.30 sq.km/sq.km area of the zone in 2021. The share of agricultural land to total land was 62.32, 62.19 and 45.52% in 2001, 2011 and 2021, respectively. Changing rate of vegetation cover is also negative and consistently lower than other zones. Vegetation area was 32.46% in 2001, 27.27% in 2011 and 23.16% in 2021. During 2001–2011, area covered by fallow was increased by 3.72% from 2.19% and after that, it declined at 0.65% in 2021. Area of the water body was 1.40, 0.49 and 0.46% in 2001, 2011 and 2021 respectively. It is noticeable that due to concretization of built-up land, the local ponds and other water bodies disappeared in many places in this zone over the times.
4.1.5. NW Zone
NW Zone covers the north-western part of the agglomeration area from the city center. This zone has experienced a significant reduction in vegetation and agricultural fields from 2001 to 2021 (; ). The vegetation area was 41.24% in 2001 which decreased to 30.91% in 2011 and 12.37% in 2021. However, during 2001–2011, the area of agricultural land was increased by 36.04% from 32.26%, and then again reduced by 18.38% in 2021. A negative and consistent change in areas of fallow and water body also happened over the decades (2001–2021). In case of fallow, it was 12.66, 7.85 and 7.47% of the land in 2001, 2011 and 2021 respectively. The area occupied by the water bodies was 3.85% in 2001, 1.78% in 2011 and 1.57% in 2021. Many water bodies and fallow lands were converted into built-up due to highway expansion, new road network connectivity; and residential and commercial purposes over the time. Presently, this zone is highly dense (more than 60% of total area is built-up) by built-up land as compared to other zones. The built-up was 9.98% of the land in 2001 which increased to 23.42% in 2011 and 60.21% in 2021. It is remarkable that a rapid rate of impervious growth has occurred in this zone.
4.2. Spatio-temporal assessment of urban growth and its intensity
Built-up area was extracted from the classified LULC of each study year. For the deeper analysis of urban growth and its intensity in every spatial corner of the UA over time, both direction and concentric zone wise discussion have been made in this present context of study. LULC change analysis reveals that Jalpaiguri UA has experienced a significant growth and development of urban areas during 2001–2021 (). Therefore, a positive and consistent change in built-up land has occurred. Only 6.26% of the land out of total agglomeration area was covered by built-up in 2001 which was increased to 15.36% in 2011 and 43.15% in 2021 (; ). The decadal growth rate of urban areas was 145.42% during 2001–2011 and 180.83% during 2011–2021 time periods. Similarly, the expansion intensity results show that the value of UEII was 0.91 that indicates moderate urban expansion speed throughout the entire agglomeration during 2001–2011. But the value of UEII has increased dramatically to 2.78 which is nothing but the very high speed of expansion during 2011–2021. This intensity of expansion gives an indication of urban sprawl occurrence. In addition, it is observed in the years 2001–2011 that the SW Zone had recorded a low urban expansion speed, whereas it was high speed for the other three directional zones. On the other hand, the expansion intensity of urban areas was very high in all the zones from 2011 to 2021.
As urban growth is not uniform in each direction, it is necessary to measure urban growth in different directions for better understanding the growth patterns ( and ). In inter-cardinal zone NE, urban area’s growth rate was 167.06% (from 0.71 to 1.90 sq.km) during 2001–2011 and after that, it was increased by 238.11% (from area 1.90 to 4.52 sq.km.) for the next decade of 2011–2021(). The UEII had always the larger value in NE Zone in 2001–2011 (1.23) and 2011–2021 (2.71), indicating high and very high urban expansion speed respectively. Similarly, this growth rate was 83.84% (from 0.85 to 1.56 sq.km.) with high speed of urban expansion (1.11) during year 2001–2011; and 105.07% (from are 1.56 to 3.21 sq.km) with very high speed (2.55) during year 2011–2021 in SE Zone (). For SW Zone, decadal growth rate of urban area was 288.50% (from 0.35 to 1.34 sq.km) from year 2001 to 2011 and 377.29% (from are 1.34 to 6.41 sq.km) during 2011–2021 time period (). UEII had the lowest value (0.47) in the first decade, indicating low speed of urban expansion, and then it was very high speed (2.39) in the last decade. The maximum growth rate of urban area was recorded in NW Zone in both the decades. It was 134.64% (from area 1.15 to 2.70 sq.km.) with high speed of urban expansion (1.34) during year 2001–2011; and then increased to 157.07% (from area 2.70 to 6.95 sq.km) with very high speed (3.68) during year 2011–2021 ().
Figure 6. Concentration of built-up area (in sq.km) at different directions and distance from city center: (a) direction wise in 2001, 2011, 2021; (b) distance wise in 2001, (c) distance wise in 2011, (d) distance wise in 2021; (e) built-up density from core to town outskirts in 2001, 2011, 2021.

Additionally, the statistical evaluation shows that the built-up density is consistently low from the city centre to the outer part of the town ( and ). Ever increasing commercial demand on land has influenced the process of concretization of natural land to manmade land (built-up) in the core area of the town as this central area is characterized mainly by commercial activities rather than residential. So, Zone 1 is the most compact zone which shared 61.44% in 2001, 79.85% in 2011, and 94.68% of built-up area out of total area of the zone (). For Zone 2, it was 21.55, 40.20 and 75.34% in 2001, 2011 and 2021, respectively (). In Zone 3, only 12.06% of the land was covered by built-up land in 2001, after that, it was increased by 26.50% in 2011 and finally by 58.84% in 2021 (). During 2001–2011, built-up cover was increased from 8.64 to 20.12% which further increased to 49.89% in 2021 across Zone 4 (). In Zone 5, it was 7.11% of the land in 2001, 17.25% in 2011, and 46.15% in 2021 (). For Zone 6, it was only 6.39% of the land in 2001, and after that, it increased to 15.66 and 43.84% of the land in 2011 and 2021, respectively (). The ultimate outer limit of Zone 7 is actually the study area itself and in this zone, the area of built-up land was 6.26% in 2001 which increased by 15.36% in 2011 and 43.15% in 2021 (; ). It is noticeable that built-up density has increased consistently in every spatial zone since 2001. Core of the town has become most compact by built-up land, and the degree of compactness is gradually low from the core to periphery of the town ( and ).
4.3. The spatial pattern of urban sprawl by landscape metrics
The selected landscape metrics were computed using the classified LULC map for the year 2001, 2011 and 2021 that gives the information on changing landscape pattern and its configuration based on edge, shape, fragmentation and contagion. The patterns of spatial urban structure were examined depending on types, density, diversity and evenness of the patches (; ).
4.3.1. Class area (CA)
CA is the total patch area of a particular land class (McGarigal and Marks Citation1995). The area of built-up land patches in Jalpaiguri UA was calculated by this metric (; ). It had added 444.99 hectares built-up area during 2001–2011 and then dramatically gained 1358.03 hectares between 2011 and 2021, i.e. it increased almost seven times in a span of the last 20 years. In addition, among different directions, the maximum growth rate of urban patch area was developed in SW Zone during both time periods of 2001–2011, and 2011–2021. On the opposite, it was lowest in SE Zone in both the decades due to more minimal fragmentation in built-up patches and higher built-up density. This spatiotemporal growth of the built-up class indicates the influence of sprawling characteristics of urban growth.
4.3.2. Per centage of land (PLAND)
PLAND is the proportion of a particular class in a whole landscape (McGarigal and Marks Citation1995). Outputs of this metric indicate the higher growth rate of built-up land over the study period (; ). The proportion of built-up class to the entire landscape (all the classes of patch) area was 6.26% in 2001, and then increased to 15.36% in 2011 and 43.15% in 2021. During 2001–2011, the growth rate of the percentage of land (built-up patch) was 167.06% in NE, 83.84% in SE, 288.50% in SW, and 134.64% in NW Zone. For the next decade (2011–2021), it was 138.11, 105.07, 377.29 and 157.07% in the direction of NE, SE, SW, and NW Zone respectively. Therefore, the growth rate of built-up patch area was exceptionally high over time, and it was higher in the last decade than the previous one.
4.3.3. Edge density (ED)
ED is used to quantify the landscape’s fragmentation level and spatial heterogeneity (Wei and Zhang Citation2012). ED is related to the number of edges in a particular landscape. Here, ED is the total number of built-up edges in respect to the entire landscape area. Study region has experienced an increase in ED since 2001, i.e. it was 27.71 in 2001, then increased to 70.64 in 2011, and finally to 141.71 meters per hectare in 2021 (; ). Similar increase in ED was also found in all the directions from the city center, which means the spatial heterogeneity and level of fragmentation in urban landscape was more, and it indicates a sprawling effect on physical expansion of the town region. However, the magnitude of the growth rate of ED was lower throughout the region in the last decade than in the previous one. Direction-wise results of ED also followed the same pattern. Only the SE Zone has recorded a slightly higher growth rate of ED during 2011–2021 as compared to the 2001–2011 time periods.
4.3.4. Area weighted mean patch fractal dimension (FRAC_AM)
FRAC_AM is the area-weighted mean value of the fractal dimension values of all patches of a particular class (Akın et al. Citation2013). The weightings of the area of individual patches are put into each patch in this indicator that measures the patch complexity based on patch size in a landscape. The study region along with each direction has recorded the values closer to one (1) that indicate the built-up lands were composed of patches in the time periods of urban expansion with simple geographical shape of built-up patches (; ). In addition, NW Zone had met a reduction in FRAC_AM from 1.26 in 2011 to 1.25 in 2021 indicating the complexity in the shape of built-up class patches.
4.3.5. Patch density (PD)
PD is an indicator to measure landscape fragmentation (Ramachandra et al. Citation2014). PD is associated with the number of patches (NP) as with an increase in NP results in an increase in PD in general. PD of built-up class has steadily increased since 2001, rising from 10.33 in 2001 to 21.50 in 2011 and after that to 26.80 per sq.km in 2021 across the entire landscape (; ). A similar rising pattern in PD is noticed in SE and SW Zone due to more segmentation in built-up patches in the landscape. But in 2021, reducing PD in NE, and NW Zone indicates less NP due to the combination of two or more smaller patches and the formation of single large patches. Nevertheless, the core, as well as the peripheral area with a completely occupied built-up class with lower PD in 2021, denotes the compact urban growth at the core and town outskirts.
4.3.6. Landscape shape index (LSI)
LSI can be used to measure the complexity (class aggregation or clumpy) of patches in a landscape (Ramachandra and Aithal Citation2013). The lower value represents the compactness of built-up patch. A positive trend in LSI has been observed since 2001. However, the values of this index between 2001 and 2021 indicate that built-up land was less dispersed across the entire area and its each direction (; ). The value of LSI in recent times also depicts built-up area in SE, and NW Zone are less fragmented, i.e. LSI of only 9.73 in 2021 and 10.61 in 2021, respectively. Therefore, the study area has experienced a lower value of LSI over the study period that indicates the clumpy urban growth in the core area of the town and the development of new built-up patches in peripheral regions or outskirt of the main town.
4.3.7. Simpson’s diversity index (SIDI)
Depending on the evenness and richness of the patch type, the heterogeneity of a landscape can be measured using this diversity indicator. The value of SIDI is zero (0) when a landscape consists of only one (1) patch which means no diversity (McGarigal and Marks Citation1995). There has been a slight increase in the value of this indicator from 2001 to 2021. Each inter-cardinal direction has followed the same trend in SIDI for each corresponding year (; ). It indicates patch richness and however, the values are towards no diversity indication. This suggests that the study region has been emerging as a compact built-up land expansion since 2001. SIDI is somewhat less sensitive to rare patch types than Shannon’s diversity index (McGarigal and Marks Citation1995), which has been discussed later (in Shannon’s entropy model) and that also indicates the compact distribution of built-up area.
4.4. Shannon’s entropy for urban sprawl analysis
The built-up area in different buffer zones from the city centre has been extracted and calculated from classified land use maps in each study year (). For both urban expansion and growth, Shannon’s entropy indicator is widely used and a meaningful technique to critically assess them. The results of the model are presented in both tabular and figure format (; ). The steadily increasing trend in the area of built-up has been observed in Jalpaiguri UA and each inter-cardinal direction, i.e. NE, SE, SW, and NW Zone over time (; and ). So, the analysis and elaboration have been discussed in five parts (UA and its four directions) below.
Figure 8. Results of Shannon’s entropy model in Jalpaiguri UA and its different directions in 2001, 2011 and 2021 based on spatial distance from the city center: (a) Jalpaiguri UA, (b) NE Zone, (c) SE Zone, (d) SW Zone, (e) NW Zone.

Table 5. Results of shannon’s entropy model in Jalpaiguri UA and its different spatial directions.
The results of the models reveal that this region has experienced huge urban growth in the last two decades (2001–2021). In relative entropy, the log(n) values were 0.84 for the entire UA, 0.78 for NE Zone, 0.70 for SE Zone, 0.84 for SW Zone and 0.78 for NW Zone, respectively (). The magnitude of change in sprawl or dispersion (relative entropy) was from 2001 to 2011 by 0.14, and from 2011 to 2021 by 0.09 over the agglomeration area (); from 2001 to 2011 by 0.16, and from 2011 to 2021 by 0.05 in the NE Zone (); from 2001 to 2011 by 0.11, and from 2011 to 2021 by 0.01 in SE Zone (); from 2001 to 2011 by 0.13, and from 2011 to 2021 by 0.22 in SW Zone (); and from 2001 to 2011 by 0.10, and from 2011 to 2021 by 0.04 in NW Zone (). However, entropy values were closer to zero (0) in all spatial cases over the study period. Similarly, relative entropy values were also below the threshold (0.5) that indicates technically compact distribution of built-up land over the region (). In 2021, relative entropy values are nearer to the threshold (towards 0.5 from 0) and comparatively higher (except NW Zone) than that of 2001 and 2011, which denotes compact sprawling. Notably, NW Zone has evolved into a compact zone, as it resulted in lower relative entropy in 2021 than that of 2011. Significant class aggregation of built-up class patches has occurred across the entire urban landscape in recent time, which is also verified by landscape metrics previously (). Therefore, the growth of built-up area in past two decades resulted in the occurrence of sprawling characteristics with the compact distribution of built-up land in the core of the urban region ().
5. Discussion
Urban growth is a dynamic process which has been discussed by researchers around the world. This research study examined the dynamics of the land use change phenomenon, the spatial dimension of urban growth pattern and its intensity from the core of the town to the periphery in the spatiotemporal context in Jalpaiguri UA. Among the land use items, the area of agricultural land, vegetation, fallow, and water bodies have been reduced significantly due to the influence of urbanization between 2001 and 2021. This situation may have a negative impact on the natural environment (Alphan Citation2003; Mosammam et al. Citation2017; Sumari et al. Citation2020). Simultaneously, urban or built-up land has increased rapidly over time. It has shown consistent growth in areas and eventually, it increased almost seven times (from 3.06 sq.km in 2001 to 21.09 sq.km in 2021) in a time span of the last 20 years (2001–2021 periods). Growth of built-up was higher in the last decade than the previous one due to the emergence of peripheral new CT around the municipality by the encouragement of urbanization in 2011. UEII also shows that the expansion intensity of urban areas was moderate in the first period and then it was very high speed in the next decade. Growth rate and expansion intensity are not the same in each spatial direction from the city centre due to their heterogeneous land use activities (Ren et al. Citation2013). Among the inter-cardinal directions, the SW zone recorded the highest urban growth rate in both decades, but the maximum intensity of spatial expansion of the urban body occurred in the NW zone in the same time periods. It is depicted that areas nearer to the city centre are more compact by urban land than the areas at the urban fringe. It indicates lower compactness and fragmentation at the town outskirts. This type of urban land development is commonly notable in small and medium sized cities in Africa and Asia (Xu et al. Citation2019; Zhao et al. Citation2020).
Landscape metrics have verified urban growth patterns in relation to sprawl in various directions from the centre of the town. The temporal results of CA represent the continuously growing status of built-up land across the entire urban region as well as its different directional zones. PLAND also shows a similar trend within the urban landscape. The PD analysis demonstrates the reasonable landscape fragmentation and sprawling with time. A decrease in the number of patches or patch density in the NW, and NE Zone during 2011–2021 indicates the compact built-up growth in the core area along with the main roadsides. The temporal results of ED illustrate the urban growth characteristics over time. The LSI analysis demonstrates the same trend of measurement with significant shape complexity with clumpy urban growth. The temporal FRAC_AM analysis indicates that the landscape is composed mainly of urban built-up patches with simple geographic shapes. Considering FRAC_AM, NW direction shows the same urban growth pattern similar to NW, and NE zones in the case of PD analysis. Finally, outputs of SIDI depict no diversity in the landscape which means compact expansion of built-up class nearer to the core area during the urban expansion period. Therefore, in most cases, these landscape metrics have confirmed the occurrence of sprawling characteristics in urban growth in the last two study decades. As a result, the core area of the urban region had become compact due to intensive development and activities and exceeded demand for land which created limits to dispersed random sprawling patterns. The core area has experienced infilling and intensive land use during the period of urban land development (Qing et al. Citation2020; Yılmaz and Terzi Citation2020; Sapena and Ruiz Citation2021). The smaller urban patches in the peripheral area of the main town connected together and created new large patches or the small patches combined with existing single large patches at the town’s outskirts (Das and Angadi Citation2021). Shannon’s entropy has quantified urban sprawl with time at different spatial directions and distances from the city centre. The analysis shows the compact urban growth across the region over the study period. This type of growth pattern is visible in small and medium sized cities in Asia, Europe and North America (Xu et al. Citation2019). Among the directional zones, the NW zone has become the most compact built-up land in recent times. Consequently, it has an adverse impact on other land use items in this zone as well as its surrounding areas. This clumpy growth of urban or built-up land at different geographical extents would affect the local environment and ecosystem services. However, the SW zone is in favour of the predicted future expansion of urban physical growth while the rest zones have exhibited comparatively compact built-up land.
The growth of population and urban land are the main aspects of urban growth monitoring. There is always a strong relationship between them. In order to understand how changes in urban land with respect to population growth are occurring, the study used 25 municipal wards and 1 CT as observations. The increments of urban population and urban land in each zone from 2001 to 2021 are highly correlated (). Kharia CT is located around the municipality, where urban land expansion and population growth are most during the 2001–2021 periods. The increment of urban land in the municipal area is very low. The municipal wards nearer to the city centre have gained less amount of urban land from 2001–2021 due to higher built-up density and limited land space for urban land expansion.
The urban growth and its related issues are alarming in developing countries like India. The medium and small sized cities in India are sprawling rapidly (Das and Angadi Citation2021). Hence, it is very important to identify the urban growth pattern for future prediction and planning (Yılmaz and Terzi Citation2021). The present study focuses on the major land use transformation and urban expansion to a spatial extent over time. However, in order to understand the urban growth scenario, existing built-up items fully not provide the understanding of urban growth. Several socio-economic factors are also responsible for urban growth in a certain region (Camagni et al. Citation2002; Correia Filho et al. Citation2022). The main limitation of this research work is the lack of some important data and information like current population size, migration, household and economic activities, vehicle data etc. Deficiency of literature is another limitation of this research study.
6. Conclusion
The small and medium sized urban bodies in India have a different scenario of urban growth and its related issues from large cities. This research work emphasized temporal urban physical growth and landscape changes in a small urban region like Jalpaiguri UA. This UA has seen huge urban growth during the 2001–2021 period. The results of this research show that urban or built-up land has increased expressively with a significant loss of non-built-up items. Urban land has increased by 21.09 sq.km in 2021 from 3.06 sq.km in 2001, which created sprawling characterises of urban growth throughout the region. The inner part of the town has become compacted by built-up items through infilling and intensive land use processes, while uncontrolled and unplanned urban land development was encouraged by dispersed urban growth at the outskirts of the municipality. Key findings of Landscape metrics and Shannon’s entropy established that this urban region has experienced compact urban expansion during the period of urban growth. This clumpy situation has been created due to the aggregation of urban patches, while the dispersed urban growth at the fringe happened due to the fragmentation of the entire landscape into small patches. Direction and distance (from the city centre) wise analysis of urban sprawl demonstrates that the south-western direction of this township has a probability to expand in the future while the other directions are mostly compact by built-up items. Urban growth in Jalpaiguri UA is not a planned one because of its uncontrolled and haphazard distribution of urban land over the region. Urban growth means not only the growth of built-up land, rather it also associated with a growing population and its activities in urban localities. However, this research work faced a deficiency of data and information like current population size, economic status, infrastructure etc. The lack of literature is another limitation of this study as not even a single research study has been done on urban growth in this urban region. Hence, this study may help to explore the future research interest in urban growth and dynamics related study in this urban region. It can also be helpful to urban planners and researchers in understanding the urban growth dynamics in smaller urban bodies like Jalpaiguri UA. This research study suggests that there is an immediate need for an appropriate strategy for the effective utilization of land use resources and allocation of infrastructure by managing improper enforcement of land use in accordance with environment and ecosystem services in this urban region. The findings of the study also provide a visualization and quantification of dense and expanding urban footprint which would help the planners and local authorities to take proper planning approach for sustainable urban development.
Authors contributions
SB, DR and BCS: Methodology, SB, DR and BCS: Software, SB, DR and BCS: Formal analysis and investigation, SB, DR, BCS, HGA, and HA: Writing—original draft preparation, SB, DR, BCS, HGA and HA: Writing—review and editing, SB, HGA and HA: Supervision. All authors have read and agreed to the published version of the manuscript.
Ethical approval
This article does not contain any studies with human participants or animals performed by any of the authors.
Acknowledgements
The authors are thankful to the editors and potential reviewers
Disclosure statement
No potential conflict of interest was reported by the author(s).
Availability of data
The data that support the findings of this study are available on request from the corresponding author.
References
- Aguilera F, Valenzuela LM, Botequilha-Leitão A. 2011. Landscape metrics in the analysis of urban land use patterns: a case study in a Spanish metropolitan area. Landsc Urban Plan. 99(3-4):226–238. doi: 10.1016/j.landurbplan.2010.10.004.
- Aithal BH, Vinay S, Ramachandra TV. 2018. Simulating urban growth by two state modelling and connected network. Model Earth Syst Environ. 4(4):1297–1308. doi: 10.1007/s40808-018-0506-1.
- Akın A, Erdoğan MA, Berberoğlu S. 2013. The spatiotemporal land use/cover change of Adana city. Int Arch Photogramm Remote Sens Spatial Inf Sci. XL-7/W2(ISPRS2013-SSG):1–6. doi: 10.5194/isprsarchives-XL-7-W2-1-2013.
- Al-Kofahi SD, Hammouri N, Sawalhah MN, Al-Hammouri AA, Aukour FJ. 2018. Assessment of the urban sprawl on agriculture lands of two major municipalities in Jordan using supervised classification techniques. Arab J Geosci. 11(3):1–12. doi: 10.1007/s12517-018-3398-5.
- Alphan H. 2003. Land‐use change and urbanization of Adana, Turkey. Land Degrad Dev. 14(6):575–586. doi: 10.1002/ldr.581.
- Al-Sharif AA, Pradhan B, Shafri HZ, Mansor S. 2014. Quantitative analysis of urban sprawl in Tripoli using Pearson’s Chi-Square statistics and urban expansion intensity index. In: Shariff ARM, editor. IGRSM 2014. 7th IGRSM International Remote Sensing & GIS Conference and Exhibition; Apr 22–23; Kuala Lumpur, Malaysia: IOP Publishing. p. 012006. doi: 10.1088/1755-1315/20/1/012006.
- Anderson JR, Hardy EE, Roach JT, Witmer RE. 1976. A land use and land cover classification system for use with remote sensor data. Geological Survey Professional Paper 964, United States Government Printing Office, Washington. doi: 10.3133/pp964.
- Barnes KB, Morgan JM, IIIRoberge MC, Lowe S. 2001. Sprawl development: its patterns, consequences, and measurement [dissertation]. Towson: Towson University.
- Batty M, Xie Y, Sun Z. 1999. The dynamics of urban sprawl. Working Paper Series, Paper 15. Center for Advanced Spatial Analysis, University College London; [accessed 2022 Nov 3]. https://www.ucl.ac.uk/bartlett/casa/bartlett-center-advanced-spatial-analysis.
- Berila A, Isufi F. 2021. Two decades (2000–2020) measuring urban sprawl using GIS, RS and landscape metrics: a case study of municipality of Prishtina (Kosovo). J Ecol Eng. 22(6):114–125. doi: 10.12911/22998993/137078.
- Bhatta B. 2010. Analysis of urban growth and sprawl from remote sensing data. Heidelberg: Springer Berlin. Chapter 2, Causes and consequences of urban growth and sprawl. p. 17–36. 10.1007/978-3-642-05299-6_2.
- Bhatta B. 2012. Urban growth analysis and remote sensing: a case study of Kolkata, India 1980–2010. Netherlands: Springer; [accessed 2022 May 07]. 10.1007/978-94-007-4698-5.
- Brown DL, Schafft KA. 2011. Rural people and communities in the 21st century: resilience and transformation. Cambridge: Polity.
- Bugliarello G. 2003. Large urban concentrations: a new phenomenon. In: Heiken G, Fakundiny R, Sutter J, editors. Earth science in the city: a reader. New York: Wiley; p. 7–19. doi: 10.1029/SP056p0007.
- Camagni R, Gibelli MC, Rigamonti P. 2002. Urban mobility and urban form: the social and environmental costs of different patterns of urban expansion. Ecol Econ. 40(2):199–216. doi: 10.1016/S0921-8009(01)00254-3.
- Chettry V, Surawar M. 2020. Urban sprawl assessment in Raipur and Bhubaneswar urban agglomerations from 1991 to 2018 using geoinformatics. Arab J Geosci. 13(14):667. doi: 10.1007/s12517-020-05693-0.
- Colsaet A, Laurans Y, Levrel H. 2018. What drives land take and urban land expansion?: a systematic review. Land Use Policy. 79:339–349. doi: 10.1016/j.landusepol.2018.08.017.
- Correia Filho WLF, de Oliveira-Júnior JF, dos Santos CTB, Batista BA, de Barros Santiago D, da Silva Junior CA, Teodoro PE, da Costa CES, da Silva EB, Freire FM. 2022. The influence of urban expansion in the socio-economic, demographic, and environmental indicators in the City of Arapiraca-Alagoas, Brazil. Remote Sens Appl: Soc Environ. 25:100662. doi: 10.1016/j.rsase.2021.100662.
- Das Chatterjee N, Chatterjee S, Khan A. 2016. Spatial modeling of urban sprawl around Greater Bhubaneswar city, India. Model Earth Syst Environ. 2(1):14. doi: 10.1007/s40808-015-0065-7.
- Das S, Angadi DP. 2021. Assessment of urban sprawl using landscape metrics and Shannon’s entropy model approach in town level of Barrackpore sub-divisional region, India. Model Earth Syst Environ. 7(2):1071–1095. doi: 10.1007/s40808-020-00990-9.
- Dutta I, Das A. 2019. Exploring the dynamics of urban sprawl using geo-spatial indices: a study of English Bazar urban agglomeration, West Bengal. Appl Geomat. 11(3):259–276. doi: 10.1007/s12518-019-00257-8.
- Foody GM. 2002. Status of land cover classification accuracy assessment. Remote Sens Environ. 80(1):185–201. doi: 10.1016/S0034-4257(01)00295-4.
- Frazier AE, Kedron P. 2017. Landscape metrics: past progress and future directions. Curr Landscape Ecol Rep. 2(3):63–72. doi: 10.1007/s40823-017-0026-0.
- Galster G, Hanson R, Ratcliffe MR, Wolman H, Coleman S, Freihage J. 2001. Wrestling sprawl to the ground: defining and measuring an elusive concept. Hous Policy Debate. 12(4):681–717. doi: 10.1080/10511482.2001.9521426.
- Ghosh S, Das A. 2017. Exploring the lateral expansion dynamics of four metropolitan cities of India using DMSP/OLS night time image. Spat Inf Res. 25(6):779–789. doi: 10.1007/s41324-017-0141-3.
- Grigorescu I, Mitrică B, Kucsicsa G, Popovici E-A, Dumitraşcu M, Cuculici R. 2012. Post-communist land use changes related to urban sprawl in the Romanian metropolitan areas. Human Geographies. 6(1):35–46. doi: 10.5719/hgeo.2012.61.35.
- Habibi S, Asadi N. 2011. Causes, results and methods of controlling urban sprawl. Procedia Eng. 21:133–141. doi: 10.1016/j.proeng.2011.11.1996.
- Hassan MM. 2017. Monitoring land use/land cover change, urban growth dynamics and landscape pattern analysis in five fastest urbanized cities in Bangladesh. Remote Sens Appl Soc Environ. 7:69–83. doi: 10.1016/j.rsase.2017.07.001.
- Herold M, Scepan J, Clarke KC. 2002. The use of remote sensing and landscape metrics to describe structures and changes in urban land uses. Environ Plan A. 34(8):1443–1458. doi: 10.1068/a3496.
- Ismael HM. 2021. Urban form study: the sprawling city- review of methods of studying urban sprawl. GeoJournal. 86(4):1785–1796. doi: 10.1007/s10708-020-10157-9.
- Jat MK, Garg PK, Khare D. 2008. Modelling of urban growth using spatial analysis techniques: a case study of Ajmer city (India). Int J Remote Sens. 29(2):543–567. doi: 10.1080/01431160701280983.
- Johnson MP. 2001. Environmental impacts of urban sprawl: a survey of the literature and proposed research agenda. Environ Plan A. 33(4):717–735. doi: 10.1068/a3327.
- Khatami R, Mountrakis G, Stehman SV. 2017. Mapping per-pixel predicted accuracy of classified remote sensing images. Remote Sens Environ. 191:156–167. doi: 10.1016/j.rse.2017.01.025.
- Lata KM, Rao CS, Prasad VK, Badarianth KVS, Rahgavasamy V. 2001. Measuring urban sprawl: a case study of Hyderabad. GIS Dev. 5(12):26–29.
- Liang F, Zhang X, Li H, Yu H, Lin Q, Jiang M, Zhang J. 2022. Land use classification based on maximum likelihood method. In: Pan JS, Balas VE, Chen CM, editors. Advances in intelligent data analysis and applications. Singapore: Springer; p. 133–139. doi: 10.1007/978-981-16-5036-9_15.
- Mallick SK, Das P, Maity B, Rudra S, Pramanik M, Pradhan B, Sahana M. 2021. Understanding future urban growth, urban resilience and sustainable development of small cities using prediction-adaptation-resilience (PAR) approach. Sustain Cities Soc. 74:103196. doi: 10.1016/j.scs.2021.103196.
- McGarigal K, Marks BJ. 1995. FRAGSTATS: Spatial pattern analysis program for quantifying landscape structure. Gen Tech Rep PNW-GTR-351, Portland. US Department of Agriculture, Forest Service, Pacific Northwest Research Station; pp. 1–122. doi: 10.2737/PNW-GTR-351.
- Mitra A, Mehta B. 2011. Cities as the engine of growth: evidence from India. J Urban Plann Dev. 137(2):171–183. doi: 10.1061/(ASCE)UP.1943-5444.0000056.
- Mitric B, Geat RS, Grigorescu I. 2014. The Romanian urban system – an overview of the post-communist period. Forum geographic.. XIII(2):230–241. doi: 10.5775/fg.2067-4635.2014.179.d.
- Mosammam HM, Nia JT, Khani H, Teymouri A, Kazemi M. 2017. Monitoring land use change and measuring urban sprawl based on its spatial forms: the case of Qom city. Egypt J Remote Sens Space Sci. 20(1):103–116. doi: 10.1016/j.ejrs.2016.08.002.
- Pande CB, Moharir KN, Varade AM, Abdo HG, Mulla S, Yaseen ZM. 2023. Intertwined impacts of urbanization and land cover change on urban climate and agriculture in Aurangabad city (MS), India using google earth engine platform. J Clean Prod. 422:138541. doi: 10.1016/j.jclepro.2023.138541.
- Patra S, Sahoo S, Mishra P, Mahapatra SC. 2018. Impacts of urbanization on land use/cover changes and its probable implications on local climate and groundwater level. J Urban Manag. 7(2):70–84. doi: 10.1016/j.jum.2018.04.006.
- Population Census. 2011. Office of the Registrar General & Census Commissioner, India. https://censusindia.gov.in/census.website/
- Qing L, Petrosian HA, Fatholahi SN, Chapman MA, Li J. 2020. Quantifying urban expansion using Landsat images and landscape metrics: a case study of the Halton Region, Ontario. Geomatica. 74(4):220–237. doi: 10.1139/geomat-2020-0017.
- Rahaman M, Dutta S, Sahana M, Das DN. 2019. Analysing urban sprawl and spatial expansion of Kolkata urban agglomeration using geospatial approach. In: Kumar P, Rani M, Chandra Pandey P, Sajjad H, Chaudhary BS, editors. Applications and challenges of geospatial technology. Switzerland: Springer; p. 205–221. doi: 10.1007/978-3-319-99882-4_12.
- Rahman A, Aggarwal SP, Netzband M, Fazal S. 2011. Monitoring urban sprawl using remote sensing and GIS techniques of a fast growing urban center, India. IEEE J Sel Top Appl Earth Observations Remote Sensing. 4(1):56–64. doi: 10.1109/JSTARS.2010.2084072.
- Ramachandra TV, Aithal BH. 2013. Urbanisation and sprawl in the Tier II City: metrics, dynamics and modelling using spatio-temporal data. Int J Remote Sens Appl. 3(2):65–74.
- Ramachandra TV, Aithal BH, Sowmyashree MV. 2014. Urban structure in Kolkata: metrics and modelling through geo-informatics. Appl Geomat. 6(4):229–244. doi: 10.1007/s12518-014-0135-y.
- Ren P, Gan S, Yuan X, Zong H, Xie X. 2013. Spatial expansion and sprawl quantitative analysis of mountain city built-up area. In: Bian F, Xie Y, Cui X, Zeng Y, editors. Geo-informatics in resource management and sustainable ecosystem. Heidelberg: Springer; p. 166–176. doi: 10.1007/978-3-642-45025-9_19.
- Ritchie H, Roser M. 2018. Urbanization. Our World in Data; [accessed 2022 May 07] https://ourworldindata.org/urbanization.
- Roy S, Bose A, Majumder S, Roy Chowdhury I, Abdo HG, Almohamad H, Abdullah Al Dughairi A. 2022. Evaluating urban environment quality (UEQ) for Class-I Indian city: an integrated RS-GIS based exploratory spatial analysis. Geocarto Int. 38(1):2153932. doi: 10.1080/10106049.2022.2153932.
- Saini V, Tiwari RK. 2020. A systematic review of urban sprawl studies in India: a geospatial data perspective. Arab J Geosci. 13(17):840. doi: 10.1007/s12517-020-05843-4.
- Sapena M, Ruiz LA. 2021. Identifying urban growth patterns through land-use/land-cover spatio-temporal metrics: simulation and analysis. Int J GeogrInf Sci. 35(2):375–396. doi: 10.1080/13658816.2020.1817463.
- Settle JJ, Briggs SA. 1987. Fast maximum likelihood classification of remotely-sensed imagery. Int J Remote Sens. 8(5):723–734. doi: 10.1080/01431168708948683.
- Shannon CE. 1948. A mathematical theory of communication. Bell Syst Tech J. 27(3):379–423. doi: 10.1002/j.1538-7305.1948.tb01338.x.
- Shao Z, Sumari NS, Portnov A, Ujoh F, Musakwa W, Mandela JP. 2021. Urban sprawl and its impact on sustainable urban development: a combination of remote sensing and social media data. Geo Spat Inf Sci. 24(2):241–255. doi: 10.1080/10095020.2020.1787800.
- Shaw A, Satish MK. 2007. Metropolitan restructuring in post-liberalized India: separating the global and the local. Cities. 24(2):148–163. doi: 10.1016/j.cities.2006.02.001.
- Shetty PJ, Gowda S, Gururaja KV, Sudhira HS. 2012. Effect of landscape metrics on varied spatial extents of Bangalore, India. Asian J Geoinformatics. 12(1):1–11.
- Shikary C, Rudra S. 2021. Measuring urban land use change and sprawl using geospatial techniques: a study on Purulia municipality, West Bengal, India. J Indian Soc Remote Sens. 49(2):433–448. doi: 10.1007/s12524-020-01212-6.
- Shukla A, Jain K. 2019. Modeling urban growth trajectories and spatiotemporal pattern: a case study of Lucknow city, India. J Indian Soc Remote Sens. 47(1):139–152. doi: 10.1007/s12524-018-0880-1.
- Story M, Congalton RG. 1986. Accuracy assessment: a user’s perspective. Photogramm Eng Remote Sens. 52(3):397–399.
- Strahler AH. 1980. The use of prior probabilities in maximum likelihood classification of remotely sensed data. Remote Sens Environ. 10(2):135–163. doi: 10.1016/0034-4257(80)90011-5.
- Sudhira HS, Ramachandra TV, Jagadish KS. 2003. Urban sprawl pattern recognition and modeling using GIS. Proc Map India. 2003:28–31.
- Sudhira HS, Ramachandra TV, Jagadish KS. 2004. Urban sprawl: metrics, dynamics and modelling using GIS. Int J Appl Earth Obs Geoinf. 5(1):29–39. doi: 10.1016/j.jag.2003.08.002.
- Sumari NS, Cobbinah PB, Ujoh F, Xu G. 2020. On the absurdity of rapid urbanization: spatio-temporal analysis of land-use changes in Morogoro, Tanzania. Cities. 107:102876. doi: 10.1016/j.cities.2020.102876.
- Sumari NS, Xu G, Ujoh F, Korah PI, Ebohon OJ, Lyimo NN. 2019. A geospatial approach to sustainable urban planning: lessons for Morogoro Municipal Council, Tanzania. Sustainability. 11(22):6508. doi: 10.3390/su11226508.
- Sun L, Chen J, Li Q, Huang D. 2020. Dramatic uneven urbanization of large cities throughout the world in recent decades. Nat Commun. 11(1):5366. doi: 10.1038/s41467-020-19158-1.
- Sun H, Forsythe W, Waters N. 2007. Modeling urban land use change and urban sprawl: Calgary, Alberta, Canada. Netw Spat Econ. 7(4):353–376. doi: 10.1007/s11067-007-9030-y.
- Sýkora L, Ourednek M. 2007. Sprawling post-communist metropolis: commercial and residential suburbanization in Prague and Brno, the Czech Republic. In: Razin E, Dijst M, VÁZquez C, editors. Employment deconcentration in European metropolitan areas. Dordrecht: Springer; vol 91, p. 209–233. doi: 10.1007/978-1-4020-5762-5_8.
- Tewolde MG, Cabral P. 2011. Urban sprawl analysis and modeling in Asmara, Eritrea. Remote Sens. 3(10):2148–2165. doi: 10.3390/rs3102148.
- Thakur PK, Kumar M, Gosavi VE. 2020. Monitoring and modelling of urban sprawl using geospatial techniques – a case study of Shimla city, India. In: Sahdev S, Singh R, Kumar M, editors. Geoecology of landscape dynamics. Singapore: Springer; p. 263–294. doi: 10.1007/978-981-15-2097-6_17.
- Thomas RW. 1981. Information statistics in geography. Norwich: Geo Abstracts.
- Torrens P, Alberti M. 2000. Measuring sprawl. Working Paper Series, Paper 27. CenterFor Advanced Spatial Analysis, University College London; [accessed 2022 Nov 3]. https://www.ucl.ac.uk/bartlett/casa/bartlett-center-advanced-spatial-analysis.
- Tumbe C. 2016. Urbanization, demographic transition and the growth of cities in India, 1870–2020. IGC Working Paper C-35205-INC-1; [accessed 2022 Oct 12]. https://www.theigc.org/publications.
- Varkey AM, Manasi S. 2019. A review of peri-urban definitions, land use changes and challenges to development. Urban India. 39(1):96–111.
- Wei Y, Zhang Z. 2012. Assessing the fragmentation of construction land in urban areas: an index method and case study in Shunde, China. Land Use Policy. 29(2):417–428. doi: 10.1016/j.landusepol.2011.08.006.
- Wu J. 2013. Landscape ecology. In: Leemans R, editor. Ecological systems. New York: Springer; p. 179–200. doi: 10.1007/978-1-4614-5755-8_11.
- Xu G, Dong T, Cobbinah PB, Jiao L, Sumari NS, Chai B, Liu Y. 2019. Urban expansion and form changes across African cities with a global outlook: spatiotemporal analysis of urban land densities. J Clean Prod. 224:802–810. doi: 10.1016/j.jclepro.2019.03.276.
- Yılmaz M, Terzi F. 2020. Characteristics of spatio-temporal urban growth patterns due to the driving forces of urbanization: the coastal city of Antalya, Turkey. IRSPSD International. 8(3):16–33. doi: 10.14246/irspsd.8.3_16.
- Yılmaz M, Terzi F. 2021. Measuring the patterns of urban spatial growth of coastal cities in developing countries by geospatial metrics. Land Use Policy. 107:105487. doi: 10.1016/j.landusepol.2021.105487.
- Zhao M, Zhou Y, Li X, Cheng W, Zhou C, Ma T, Li M, Huang K. 2020. Mapping urban dynamics (1992–2018) in Southeast Asia using consistent nighttime light data from DMSP and VIIRS. Remote Sens Environ. 248:111980. doi: 10.1016/j.rse.2020.111980.
- Zhou X, Chen H. 2018. Impact of urbanization-related land use land cover changes and urban morphology changes on the urban heat island phenomenon. Sci Total Environ. 635:1467–1476. doi: 10.1016/j.scitotenv.2018.04.091.