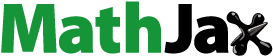
Abstract
Methane (CH4) is the second most abundant anthropogenic greenhouse gas after carbon dioxide (CO2), accounting for about 20% of global emissions. CH4 anthropogenic emission sources include landfills, oil and natural gas systems, agricultural activities, coal mining, stationary and mobile combustion, wastewater treatment and certain industrial processes. In this work, we examine the spatio-temporal dynamics of CH4 and its relationship to climatic and vegetation parameters in the Northern Cape province in South Africa. Various datasets from the TROPOspheric Monitoring Instrument, Moderate Resolution Imaging Spectroradiometer, Atmospheric Infrared Sounder and the Global Precipitation Climatology Project were used. The results show an increasing trend of CH4 concentration throughout the entire province. The greatest increase in CH4 concentration is observed in the western parts of the province during June–July–August (JJA) season. CH4 concentration shows negligible correlation with most climatic parameters, i.e. Temperature (Temp), Precipitation (Precip) and NDVI for both seasons. The Temp–NDVI relationship shows high correlation values of = –0.71 and
= 0.82 for the DJF and JJA seasons, respectively. Seasonality plays a critical role in the relationships of the CH4 to climatic and vegetation parameters. This study shows that we are in a crisis, and robust mitigation strategies are needed to combat this.
1. Introduction
Methane (CH4) is a powerful greenhouse gas and is the second-largest contributor to climate warming after carbon dioxide (CO2) (Subak and Craighill Citation1999; Howarth Citation2014). It is responsible for approximately 20% of the direct radiative forcing since 1750 (Forster et al. Citation2021). Globally, CH4 emissions and atmospheric concentrations are continuing to rise (Carrol et al. Citation2020; Jackson et al. Citation2020), with no reversal of this trend on the horizo (Höglund-Isaksson et al. Citation2020). Therefore, there is an urgency of mitigating CH4 emissions.
A molecule of CH4 traps more heat than a molecule of CO2, but CH4 has a relatively short lifespan of 7 to 12 years in the atmosphere (Prather et al. Citation2012), while CO2 can persist for hundreds of years or more (Solomon et al. Citation2009). CH4 has various sources from both natural- and man-made activities (Miller et al. Citation2013; Carrol et al. Citation2020). Natural sources include wetlands, termites, oceans, hydrates, geological sources, wild animals and wildfires (Van Amstel Citation2012). However, some of the largest sources of CH4 are in agriculture, fossil fuels and decomposition of landfill waste activities (Khalil and Shearer Citation1993; Heilig Citation1994; Moss et al. Citation2000; Van Amstel Citation2012; Jackson et al. Citation2020). There are a few sink/removal processes of CH4 from the atmosphere which include: (1) atmospheric oxidation: 88% of CH4 is oxidized in the troposphere via the hydroxy radical (OH) and 7% is oxidized in the stratosphere (Boucher et al. Citation2009) and (2) absorption of CH4 by soil, about 5% (Boucher et al. Citation2009).
As aforementioned, agricultural activities are a predominant source of CH4. Livestock emissions from manure and gastroenteric releases account for approximately 32% of man-made CH4 emissions (UN Environmental programme Citation2021). There are various livestock production systems which span from industrial factory farming to extensive grazing on open rangelands (Scoones Citation2023). Extensive livestock producers make use of rangelands that cover over half the world’s land surface (Scoones Citation2023). Of concern is the ability of ruminant livestock to produce 250 to 500 L of CH4 per day (Johnson and Johnson Citation1995). Factors that influence CH4 emissions from cattle include level of feed intake, type of carbohydrate in the diet, feed processing, addition of lipids or ionophores to the diet and alterations in the ruminal microflora (Johnson and Johnson Citation1995). However, the manipulation of these factors can reduce CH4 emissions from ruminants. Some of the techniques used for mitigation of CH4 emission from ruminants include improving nutritional management, manipulation of ruminal fermentation by changing feed composition and defaunation (Shibata and Terada Citation2010; Gerber et al. Citation2013). In general, developing countries have greater CH4 emission rates driven by poor feed digestibility, low slaughter weights, greater age at slaughter and poor animal husbandry (Herrero et al. Citation2013; Thompson and Rowntree Citation2020). Conversely, developed countries have lower CH4 emission rates due to improved grazing management leading to greater diet digestibility, more intensive feeding practices and temperate conditions (Herrero et al. Citation2013; Thompson and Rowntree Citation2020).
Wetlands are possibly the largest natural sources of CH4 to the atmosphere and contribute to about 20% of the current global annual emission (Wang et al. Citation1996; IPCC Citation2013). On the contrary, wetlands are important carbon sinks since anoxic conditions favor carbon accumulation by limiting the microbial decomposition of plant residues (Koffi et al. Citation2020). It has also been observed that constructed wetlands (CWs) applied for wastewater treatment also release a significant amount of CH4 (Yin et al. Citation2023). CWs are artificial systems that use natural processes to treat wastewater containing organic pollutants (Yu et al. Citation2022). In their review, Xu et al. (Citation2019) showed that CH4 concentration are only slightly higher in vegetated CWs than those in unvegetated CWs while obtaining a better pollutant removal efficiency.
The fossil fuel industry, including oil/gas production and coal mining contribute significantly of the total anthropogenic methane emission. Coal mining is responsible for about 12% of total anthropogenic methane emissions with 90% coming from underground mines (Janssens-Maenhout et al. Citation2019; Kholod et al. Citation2020). The amount of CH4 released depends on the productivity of the coal mine, the gassiness of the coal seam and any underlying and overlying formations, operational variables and geological conditions (Karacan et al. Citation2011).
Measuring, monitoring and management of CH4 emissions is important in mitigation measures. Satellite observation is able to provide global measurements of CH4 at a high observation rate. Satellites retrieve atmospheric CH4 column concentrations with near-unit sensitivity down to the surface by measuring spectrally resolved backscattered solar radiation in the shortwave infrared (Jacob et al. Citation2022). The atmospheric infrared sounder (AIRS) on board National Aeronautics and Space Administration (NASA) Aqua satellite has been measuring CH4 since 2020 to 2022. AIRS has a swath of 1650 km, a spatial resolution of 13.5 km at horizontal at nadir and measure’s the Earth’s outgoing radiation at 0.4 to 1.0 µm and 3.7 to 15.4 µm. More recently, the TROPOspheric Monitoring Instrument (TROPOMI) onboard Sentinel-5 Precursor was launched and has a better spatial resolution compared to AIRS. TROPOMI has an improved spatial resolution of 7 × 5.5 km and a swath of 2600 km. This makes it an ideal instrument to use for regional and local measurements.
Shikwambana et al. (Citation2022a) showed in their study the spatial distribution of CH4 over South Africa. The Northern Cape province (29.0467°S, 21.8569°E) showed the highest concentration of CH4 compared to the other provinces. Various sources were attributed to this, however, in this work we examine the extent at which livestock contributes to the CH4 concentration in the province. We also examine the effect of meteorological parameters on the concentration of CH4.
2. Materials and methods section
2.1. Study site
The Northern Cape is the largest province situated on the west coast of South Africa (see ). It borders the Atlantic Ocean in the west, Namibia in the north–west and Botswana in the north. It is a hot and dry region with a climate similar to that of desert or semi-desert areas. The west of the province experiences winter rainfall while the rest of the province experience rain predominately in the summer months. Summer day time temperatures range between 34 °C and 50 °C while winter day time temperatures range from 18 °C to 27 °C. Winter night time temperatures often drop below freezing.
Figure 1. (a) A map of the location of Northern Cape province in South Africa and the spatial distribution of mines and wetlands. (b) Rangeland types over the Northern Cape province.

The two main economic activities in the province are the agriculture and mining sectors. Agricultural activities in the province include cultivated subsistence farming, cultivated orchards, commercial pivots, vines and commercial fields (NC Citation2014). The dominant land use activity in the province is commercial agriculture production. Livestock farming of sheep, goats, cattle and game is a prominent feature of the agricultural landscape (NC Citation2014).
Mines and wetlands are also known sources of CH4 (Shikwambana et al. Citation2022a). shows the distribution of mines and wetlands over the Northern Cape province. Mines and Quarries data was derived from the South African National Land Cover 2018, which was generated using Sentinel-2 Multi-Spectral Instrument (MSI) satellite imagery of 1 January − 31 December 2018. The dataset has an overall accuracy of 90.14% (confidence interval: 89.65 − 90.62%, kappa: 89.91) and a mean class accuracy of 89.63%. The data was produced by GeoTerra Image (GTI) Pty Ltd (Mark Thompson, www.geoterraimage.com) for the South African Department of Environmental Affairs (DEA, https://egis.environment.gov.za/sa_national_land_cover_datasets). (The kappa coefficient is a popular comprehensive classification accuracy measure or indicator of the overall performance of a particular data or classification algorithm, where values closer to 1 mean a higher accuracy). The wetlands data was retrieved from the National Freshwater Ecosystem Priority Areas dataset of the South African National Biodiversity Institute (SANBI)’s Biodiversity GIS platform (https://bgis.sanbi.org/), which provides Wetland Freshwater Priority Areas (FEPAs), wetland ecosystem types and wetland conditions on a national scale.
shows the rangeland types over the Northern Cape. The rangeland types dataset was obtained from the AFRCS Rangeland map (https://africultures-platform.eu/en/). It is a high-resolution prototype product that characterizes grazing and browsing resources in Africa described in Kganyago et al. (Citation2022) and Alexandridis et al. (Citation2021). The product is provided at 30 m resolution, and it is based on Random Forest classifier and time series data from Sentinel-2 Multi-Spectral imager (MSI) and Landsat series within Google Earth Engine. The Northern Cape province was mapped at 82.24% (Kganyago et al. Citation2022). The rangeland data is important because it shows where the grazing and browsing livestock are situated. Grazing animals consume mainly the leaves and stems of grass, e.g. cattle and sheep, while browsing animals usually prefer feeding on leaves and stems of trees, shrubs or herbs, e.g. goats and giraffes. Sheep and cattle are grazing animals while goats are browsing animals.
2.2. Data
2.2.1. TROPOMI
The TROPOspheric Monitoring Instrument (TROPOMI) is an instrument on board the Sentinel-5P satellite. It was launched on 13 October 2017. The instrument operates in a push-broom configuration (non-scanning), with a swath width of approximately 2600 km on Earth’s surface. The typical pixel size (near nadir) is 7 × 3.5 km2 for all spectral bands, with the exception of the ultraviolet 1 (UV1) band (7 × 28 km2) and shortwave infrared (SWIR) bands (7 × 7 km2). The science objectives of TROPOMI are (1) to better constrain the strength, evolution and spatio-temporal variability of the sources of trace gases and aerosols impacting air quality and climate, (2) improve upon the attribution of climate forcing by a better understanding of the processes controlling the lifetime and distribution of CH4 – Methane, tropospheric O3 – Ozone and aerosols, (3) better estimate long-term trends in the troposphere related to air quality and climate from the regional to the global scale and (4) develop and improve air quality model processes and data assimilation in support of operational services including air quality forecasting and protocol monitoring (Veefkind et al. Citation2012). More specific details on sentinel-5P can be found in Theys et al. (Citation2017) and Tilstra et al. (Citation2020). In this work the CH4 product is used.
2.2.2. MODIS
The Moderate Resolution Imaging Spectroradiometer (MODIS) is an instrument aboard the Terra and Aqua satellites. The Terra and Aqua satellites have a viewing swath width of 2330 km and are able to view the entire Earth’s surface every one to two days. Its detectors measure 36 spectral bands between 0.405 and 14.385 µm covering wavelengths from visible (VIS) to long-wave infrared (LWIR). It acquires data at three spatial resolutions − 250, 500 and 1000 m. MODIS generates more than 30 science products that are openly distributed worldwide to various users (Xiong and Butler Citation2020). More details on the specifications and products of MODIS can be found in Justice et al. (Citation2002) and Xiong and Butler (Citation2020). In this study the normalized difference vegetation index (NDVI) product is used. NDVI is a measure of the amount and vigor of vegetation on the land surface. NDVI values range from −1.0 to 1.0, with negative values indicating clouds and water. Positive values near zero indicating bare soil, and higher positive values of NDVI ranging from sparse vegetation (0.1 − 0.5) to dense green vegetation (0.6 and above).
2.2.3. AIRS
The Atmospheric Infrared Sounder (AIRS) on NASA's Aqua satellite, gathers infrared energy emitted from Earth’s surface and atmosphere globally, every day. Some of the data it provides includes 3D measurements of temperature and water vapor through the atmospheric column. AIRS has 2378 infrared channels in the spectral range of 3.7 to 15.4 microns, with a spatial resolution of 13.5 km and 4 Vis/NIR channels from 0.4 to 0.8 microns with a spatial resolution of 2.3 km. More details of the instrument are discussed by Hartmut et al. (Citation1995), Chahine et al. (Citation2006), Pagano et al. (Citation2003) and Menzel et al. (Citation2018). In this study, we use the air temperature product.
2.2.4. GPCP
The Global Precipitation Climatology Project (GPCP) aim is to produce a global, monthly precipitation dataset. GPCP combines microwave and infrared measurements, and outgoing longwave radiation retrievals from satellites and, over land, also incorporates rain gauge observations. Data from rain gauge stations, satellites and sounding observations estimate monthly rainfall on a 2.5 deg × 2.5 deg latitude-longitude global grids from 1979 to the present. The key limitation of this dataset is the indirect and complex nature of translating sparse satellite precipitation measurements into gridded precipitation estimates. Also, the lack of rain gauges for ground validation over ocean introduces unknown systematic errors into this and all ocean precipitation estimates from satellites. More details on the specifications of GPCP can be found in Huffman et al. (Citation1997), Adler et al. (Citation2018) and Huffman et al. (Citation2023).
2.2.5. DALRRD
The Department of Agriculture, Land Reform and Rural Development (DALRRD) is one of the departments of the South African government. DALRRD to accelerate land reform, catalyze rural development and improve agricultural production to stimulate economic development and food security DALRRD keeps a record of the livestock data across South Africa under its directorate of statistics and economic analysis. The data presented in this report can be accessed on this link (http://www.old.dalrrd.gov.za/Home/Crop-Estimates/Statistical-Information/Livestock).
2.2.6. Statistical analysis
Pearson correlation (r) analysis was executed to establish the relationship between the climatic and environmental conditions. MATLAB was the software used to determine and plot the relationship between the parameters. The specific parameters tested for their relationship are normalized difference vegetation index (NDVI), precipitation (Precip), air temperature (Temp), relative humidity (RH) and CH4.
(1)
(1)
where
is the correlation coefficient, variables
and
represent values for each respective variable
and
respectively, and
and
are mean values of the
and
variables, respectively. Further information on the Pearson correlation test can be found in Benesty et al. (Citation2009).
A summary of the parameters used in this study is shown in .
Table 1. Data used to study emissions from wildfires.
3. Results
3.1. CH4 spatial distribution
The summer season is made up by the months December–January–February (DJF) and the winter season is made up by the months June–July–August (JJA). The seasonal spatial distribution of CH4 concentration in the Northern Cape Province is shown in . The northern parts of the province (27oS, 19oE) has high concentration (1785.5–1825.5 ppbV) of CH4 seasonally. The contributors to this are the wetlands (see ) and livestock in that area. Cloete and Olivier (Citation2010) showed that this area has a higher grazing capacity, as seen in , indicating that there is a large number of livestock in that region which emits large amounts of CH4 into the atmosphere.
Comparison of the seasons show that there is higher CH4 concentration distribution in the winter season (see ) than in the summer season (see ). The results also show an increasing trend of CH4 concentration throughout the entire province. The greatest increase in CH4 concentration is observed in the western parts of the province during the JJA season (see , red block). The increase in the CH4 concentration could be due to the accumulation of CH4 over time since CH4 has a long-life time in the atmosphere. However, the main sources of the CH4 emission is from the mining and livestock.
3.2. Transport and dispersion
Generally, wind speed is a function of the atmospheric pressure gradient, with the principal driver being the sun. Varied heating of the earth results in global circulation patterns with Coriolis forces essentially driving large-scale motion of the air (Wright and Grab Citation2017). Wind speed is also strongly influenced by local temperature gradients as well as large-scale climate oscillations such as the El Nino Southern Oscillation (ENSO) and Madden-Julian Oscillation (MJO) (Fant et al. Citation2016). Wind variability at a regional scale is strongly influenced by microscale, mesoscale and synoptic-scale factors such as topography, water bodies (e.g. oceans, lakes) and land use (Wright and Grab Citation2017). In general, the higher the wind speed, the more pollutants are dispersed and the lower their concentration. shows the wind speed distribution and wind direction over the Northern Cape Province during the DJF and JJA seasons (see , respectively). Higher wind speeds of up to 7.8 m.s−1 are observed in the JJA season compared to the DJF season with wind speeds up to 6.1 m.s−1. However, high wind speeds of 10 m.s−1 are observed over the Atlantic Ocean during the DJF season. Kruger et al. (Citation2010) showed that the high wind speeds are caused by the ridging of the Atlantic high-pressure system, and is strongest during the DJF months. The high wind speeds over the Atlantic Ocean, during the DJF season, travels in a northerly and easterly directions. These winds tend to push the pollutants produced on the coastline in land, thus, increasing the concentration of these pollutants. On the contrary, during the JJA season some wind travel toward the Atlantic Ocean while others travel in a southerly direction toward the Western Cape and Eastern Cape provinces, thus, increasing the concentrations. The stable atmosphere dominates during the in the JJA season which is characterized by high wind speed, caused by the damped vertical motions of air (Sucevic and Djurisic Citation2012). Winds are responsible for the dispersion of pollutants.
3.3. Trends and correlation
3.3.1. Trends
Seasonal adjustment is the process of removing a nuisance periodic component. The result of a seasonal adjustment is a de-seasonalized time series. De-seasonalized data is useful for exploring the trend and any remaining irregular component. The de-seasonalization was performed using MATLAB. MATLAB calculates the de-seasonalized series by subtracting (or dividing by) the estimated seasonal component, depending on the assumed decomposition.
shows the timeseries and the de-seasonalized trends for CH4, temperature, precipitation, NDVI and livestock number for the Northern Cape province. shows the timeseries and trend of CH4 concentration for the period 2013 to 2023. An increasing trend is observed between 2013 and 2023. Global studies by several researcher have also shown increasing trends of CH4 concentrations overtime (He et al. Citation2020; Turner et al. Citation2019; Van Dingenen et al. Citation2018). This is in agreement with the results of this study. Although the sources of emissions are known, it is not clear at this point what the main drivers of this increase are.
shows a fluctuating pattern of temperature for the period 2013 to 2023. High temperatures of approximately 52 °C are observed during the DJF season while low temperatures of approximately 26 °C are observed during the JJA season at day time. shows a rather complicated trend of the temperature. A slight increase in temperature is observed between the year 2015 and 2016. This is the period were a strong El Niño was observed (Shikwambana et al. Citation2022b). El Niño is associated with warmer and drier conditions. Between 2016 and 2022 a decrease in temperature is observed. Overall, the period between 2016 and 2022 was characterized by the La Niña phase.
shows a fluctuating pattern of precipitation for the period 2013 to 2023. High precipitation rates are observed during the JJA season on the western side of the province and the eastern side of the province experiences precipitation during the DJF season. The main stimulus for JJA precipitation in this region is the combination of the cold Benguela current and the northward displacement of high-pressure systems (du Plessis and Schloms Citation2017). shows the trend of precipitation. A decreasing trend is observed between 2014 and 2016, this partly due to the El Niño phase. An increasing trend is observed from 2016 to 2022. shows a fluctuating pattern of NDVI. Low values of NDVI are observed during the JJA season and high values are observed during the DJF seasons. shows a decrease in trend from 2019 to 2022. This decline could imply a loss in a healthy a vegetation which is likely to affect the grazing and browsing areas for livestock. Interestingly, the livestock number shown in is also decreasing, from 2016 to 2022. The trend in shows that the livestock number started decreasing from 2013.
3.3.2. Correlation
Correlation is a statistical measure that expresses the extent to which two variables are linearly related. The results of the statistical relationships between different parameters during the DJF and JJA season are presented in . For simplicity of interpretations, the coefficients are grouped into negligible (±0.0 to ±0.3), weak (±0.31 to ±0.5), moderate (±0.51 to ±0.7), high (±0.71 to ±0.9) and very high (±0.91 to ±1.0) negative or positive correlations (Shikwambana et al. Citation2022b).
Figure 5. The relationship between CH4, temperature (Temp), precipitation (Precip) and NDVI during the DJF and JJA seasons using the Pearson’s correlation for the period 2013–2023 over the Northern Cape province.

The DJF season (see ) constitute mostly of positive correlation whereas the JJA season (see ) has mostly negative correlation. CH4 concentration shows negligible correlation with most of the climatic parameters, i.e. Temp, Precip and NDVI for both seasons. The Temp–NDVI relationship shows high correlation values of −0.71 and 0.82 for the DJF and JJA seasons, respectively. This means that during the DJF season, high temperatures result in lower NDVI values, and vice versa. This implies that drier vegetation is as a result from higher temperatures and less precipitation during this period. The Temp–Precip relationship (0.60) during the DJF season supports this observation. Increasing temperatures contribute to increasing precipitation. The Precip–NDVI relationship (0.47) shows a weak and positive correlation. This correlation implies that the increase in precipitation contributes to the increase in greener vegetation. During the JJA season the Temp–NDVI relationship shows a high and positive correlation. This implies that high temperatures contribute to the greener vegetation. Surprisingly, the Precip–NDVI relationship (–0.49) shows a weak and negative correlation in the JJA season. This result implies that low temperatures contribute to high precipitation. This is expected as some parts of the Northern Cape province experience precipitation in the JJA season, and this drives the temperature down. Overall, the results show that seasonality place a huge role in the relationships of the parameters. Furthermore, the relationships of the parameters differ immensely.
3.3.3. Climatic parameters and CH4 atmospheric emission in livestock
The role of climatic and vegetation parameters on the livestock emissions is shown in . NDVI–Temp–Precip relationship have a moderate to high correlation (see ). High precipitation and temperature leads to greener healthier vegetation (high NDVI values). Livestock would graze on the healthy vegetation and then the rumination process would follow. CH4 emission would then follow from the ruminant livestock production systems (Tedeschi et al. Citation2022). The higher the livestock numbers the more the CH4 emissions and concentration. Although the flow diagram presented is simplistic, it does give a good idea of how livestock CH4 emissions are related to climatic parameters.
4. Discussion and conclusions
Globally, atmospheric CH4 concentration is on the rise (Skeie et al. Citation2023). Various sources such as wetlands, landfills, oil and natural gas systems, agricultural activities and coal mining contribute to the increase. An increase in natural CH4 production is driven by globally warmer temperatures and heavier rainfall. South Africa contributes to the global CH4 concentration. Specifically, the Northern Cape province in South Africa is the main contributor of CH4. This study sort to understand the contribution and role of climatic parameters on the CH4 concentration. The study found that wind speed and direction is responsible for the atmospheric dispersion and transport of CH4 seasonally. Horizontal dispersion showed that CH4 is spread more during the JJA season compared to the DJF season. The correlation analysis shows that CH4 is not affected by climatic and vegetation parameters such as temperature, precipitation and NDVI. Gedney et al. (Citation2004) showed seasonal variations of CH4 emissions in most southern Africa regions are not strongly related to changes in surface temperature, which is in agreement without findings. Akimoto et al. (Citation2015) in the study of CH4 concentration changes in East Asia observed seasonal variations in CH4 in their study area, which peaked in August. This is agreement with the observations of this study. An interesting results was observed by Chandra et al. (Citation2017). In their study conducted in the eastern region of India an increase in CH4 is observed in the DJF season. They claim this is due to increased microbial activity in these areas. This observation is not in agreement with our observation. Although the geographical sites are different, more work has to be done to understand these discrepancies.
Overall, all study shows an increase in CH4 concentration, therefore, the implementation of CH4 reduction is very important and needs to be executed soon to keep the 1.5 °C Paris Agreement target within reach. This study clearly shows that we are in a crisis and robust mitigation strategies are needed to combat this.
Disclosure statement
No potential conflict of interest was reported by the authors.
References
- Adler RF, Sapiano MRP, Huffman GJ, Wang J-J, Gu G, Bolvin D, Chiu L, Schneider U, Becker A, Nelkin E, et al. 2018. The Global Precipitation Climatology Project (GPCP) monthly analysis (New Version 2.3) and a Review of 2017 Global Precipitation. Atmosphere (Basel). 9(4):138. doi: 10.3390/atmos9040138.
- Akimoto H, Kurokawa JI, Sudo K, Nagashima T, Takemura T, Klimont Z, Amann M, Suzuki K. 2015. SLCP co-control approach in East Asia: tropospheric ozone reduction strategy by simultaneous reduction of NOx/NMVOC and methane. Atmos Environ. 122:588–595. doi: 10.1016/j.atmosenv.2015.10.003.
- Alexandridis TK, Ovakoglou G, Cherif I, Gómez Giménez M, Laneve G, Kasampalis D, Moshou D, Kartsios S, Karypidou MC, Katragkou E, et al. 2021. Designing AfriCultuReS services to support food security in Africa. Trans GIS. 25(2):692–720. doi: 10.1111/tgis.12684.
- Benesty J, Chen J, Huang Y, Cohen I. 2009. Pearson correlation coefficient. In: noise reduction in speech processing. Springer topics in signal processing, Vol 2. Berlin, Heidelberg: Springer. doi: 10.1007/978-3-642-00296-0_5.
- Boucher O, Friedlingstein P, Collins B, Shine KP. 2009. The indirect global warming potential and global temperature change potential due to methane oxidation. Environ Res Lett. 4(4):044007. doi: 10.1088/1748-9326/4/4/044007.
- Carrol M, Castaldi S, Chandra N, Crevoisier C, Crill PM, Covey K, Curry CL, Etiope G, Frankenberg C, Gedney N, et al. 2020. The Global Methane Budget 2000–2017. Earth Syst Sci Data. 12(3):1561–1623. doi: 10.5194/essd-12-1561-2020.
- Chahine MT, Pagano T, Aumann H, Atlas R, Barnet C, Blaisdell J, Chen L, Divakarla M, Fetzer E, Goldberg M, et al. 2006. AIRS: improving weather forecasting and providing new data on greenhouse gases. Bull Amer Meteor Soc. 87(7):911–926. doi: 10.1175/BAMS-87-7-911.
- Chandra N, Hayashida S, Saeki T, Patra PK. 2017. What controls the seasonal cycle of columnar methane observed by GOSAT over different regions in India? Atmos Chem Phys. 17(20):12633–12643. doi: 10.5194/acp-17-12633-2017.
- Cloete S, Olivier JJ. 2010. South African sheep and wool industry. In: The International Sheep and Wool Handbook; p. 95–112. United Kingdom: Nottingham University Press.
- Du Plessis JA, Schloms B. 2017. An investigation into the evidence of seasonal rainfall pattern shifts in the Western Cape, South Africa. J S Afr Inst Civ Eng. 59(4):47–55. doi: 10.17159/2309-8775/2017/v59n4a5.
- Fant C, Schlosser CA, Strzepek K. 2016. The impact of climate change on wind and solar resources in southern Africa. Appl Energy. 161:556–564. doi: 10.1016/j.apenergy.2015.03.042.
- Forster P, Storelvmo T, Armour K, Collins W, Dufresne J-L, Frame D, Lunt DJ, Mauritsen T, Palmer MD, Watanabe M, et al. 2021. The Earth’s energy budget, climate feedbacks, and climate sensitivity Climate Change 2021: The Physical Science Basis. Contribution of Working Group I to the Sixth Assessment Report of the Intergovernmental Panel on Climate Change. Cambridge University Press.
- Gedney N, Cox PM, Huntingford C. 2004. Climate feedback from wetland methane emissions. Geophys Res Lett. 31(20):L20503. doi: 10.1029/2004GL020919.
- Gerber PJ, Hristov AN, Henderson B, Makkar H, Oh J, Lee C, Meinen R, Montes F, Ott T, Firkins J, et al. 2013. Technical options for the mitigation of direct methane and nitrous oxide emissions from livestock: a review. Animal. 7(Suppl.2):220–234. doi: 10.1017/S1751731113000876.
- Hartmut H, Aumann H, Miller CR. 1995. Atmospheric infrared sounder (AIRS) on the earth observing system. Proceedings of the SPIE 2583, Advanced and Next-Generation Satellites, Paris, France; p. 332–343.
- He J, Naik V, Horowitz LW, Dlugokencky E, Thoning K. 2020. Investigation of The Global Methane Budget over 1980–2017 using GFDL-AM4.1. Atmos Chem Phys. 20(2):805–827. doi: 10.5194/acp-20-805-2020.
- Heilig GK. 1994. The greenhouse gas methane (CH4) 1994. Sources and sinks, the impact of population growth, possible interventions. Popul Environ. 16(2):109–137. doi: 10.1007/BF02208779.
- Herrero M, Havlík P, Valin H, Notenbaert A, Rufino MC, Thornton PK, Blümmel M, Weiss F, Grace D, Obersteiner M. 2013. Biomass use, production, feed efficiencies, and greenhouse gas emissions from global livestock systems. Proc Natl Acad Sci USA. 110(52):20888–20893. doi: 10.1073/pnas.1308149110.
- Höglund-Isaksson L, Gómez-Sanabria A, Klimont Z, Rafaj P, Schöpp W. 2020. Technical potentials and costs for reducing global anthropogenic methane emissions in the 2050 timeframe –results from the GAINS model. Environ Res Commun. 2(2):025004. doi: 10.1088/2515-7620/ab7457.
- Howarth RW. 2014. A bridge to nowhere: methane emissions and the greenhouse gas footprint of natural gas. Energy Sci Eng. 2(2):47–60. doi: 10.1002/ese3.35.
- Huffman GJ, Adler RF, Arkin P, Chang A, Ferraro R, Gruber A, Janowiak J, McNab A, Rudolf B, Schneider U. 1997. The Global Precipitation Climatology Project (GPCP) Combined Precipitation Dataset. Bull Amer Meteor Soc. 78(1):5–20.
- Huffman GJ, Adler RF, Behrangi A, Bolvin DT, Nelkin EJ, Gu G, Ehsani MR. 2023. The New Version 3.2 Global Precipitation Climatology Project (GPCP) Monthly and Daily Precipitation Products. J Climate. 36(21):7635–7655. doi: 10.1175/JCLI-D-23-0123.1.
- IPCC, Stocker T, Qin D, Plattner G-K, Tignor M, Allen SK, Boschung J. 2013. The Physical Science Basis. Contribution of Working Group I to the Fifth Assessment Report of the Intergovernmental Panel on Climate Change. In: ZauelsXia A, Bex V, Midgley PM, editors. Chapter 6, Carbon and other biogeochemical cycles. Cambridge University Press; p. 466–570.
- Jackson RB, Saunois M, Bousquet P, Canadell JG, Poulter B, Stavert AR, Bergamaschi P, Niwa Y, Segers A, Tsuruta A. 2020. Increasing anthropogenic methane emissions arise equally from agricultural and fossil fuel sources. Environ Res Lett. 15(7):071002. doi: 10.1088/1748-9326/ab9ed2.
- Jacob DJ, Varon DJ, Cusworth DH, Dennison PE, Frankenberg C, Gautam R, Guanter L, Kelley J, McKeever J, Ott LE, et al. 2022. Quantifying methane emissions from the global scale down to point sources using satellite observations of atmospheric methane. Atmos Chem Phys. 22(14):9617–9646. doi: 10.5194/acp-22-9617-2022.
- Janssens-Maenhout G, Crippa M, Guizzardi D, Muntean M, Schaaf E, Dentener F, Bergamaschi P, Pagliari V, Olivier JGJ, Peters HW, et al. 2019. EDGAR v4.3.2 Global Atlas of the three major greenhouse gas emissions for the period 1970–2012. Earth Syst Sci Data. 11(3):959–1002. doi: 10.5194/essd-11-959-2019.
- Johnson KA, Johnson DE. 1995. Methane emissions from cattle. J Anim Sci. 73(8):2483–2492. doi: 10.2527/1995.7382483x.
- Justice C, O; Townshend JRG, Vermote EF, Masuoka E, Wolfe RE, Saleous N, Roy DP, Morisette JT. 2002. An overview of MODIS Land data processing and product status. Remote Sens Environ. 83(1–2):3–15. doi: 10.1016/S0034-4257(02)00084-6.
- Karacan C, Ruiz FA, Cotè M, Phipps S. 2011. Coal mine methane 2011. A review of capture and utilization practices with benefits to mining safety and to greenhouse gas reduction. Int J Coal Geol. 86(2–3):121–156. doi: 10.1016/j.coal.2011.02.009.
- Kganyago M, Ramoelo A, Zoungrana E, Mashiyi N, Garba I. 2022. Characterizing the Spatial Distribution of Grazing and Browsing Resources in Africa Using Random Forest Classifier and Multi-Sensor Data. In the Proceedings of IEEE International Geoscience and Remote Sensing Symposium Kuala Lumpur; July 17–22; Malaysia. doi: 10.1109/IGARSS46834.2022.9883536.
- Khalil MAK, Shearer MJ. 1993. Sources of methane: an overview. In: Khalil MAK, editor. Atmospheric Methane: sources, Sinks, and Role in Global Change. Vol. 13. Berlin, Heidelberg: NATO ASI Series, Springer. doi: 10.1007/978-3-642-84605-2_10.
- Kholod N, Evans M, Pilcher RC, Roshchanka V, Ruiz F, Coté M, Collings R. 2020. Global methane emissions from coal mining to continue growing even with declining coal production. J Clean Prod. 256:120489. doi: 10.1016/j.jclepro.2020.120489.
- Koffi EN, Bergamaschi P, Alkama R, Cescatti A. 2020. An observation-constrained assessment of the climate sensitivity and future trajectories of wetland methane emissions. Sci Adv. 6(15):eaay4444. doi: 10.1126/sciadv.aay444.
- Kruger AC, Goliger AM, Retief JV, Sekele S. 2010. Strong wind climatic zones in South Africa. Wind Struct. 13(1):37–55. doi: 10.12989/was.2010.13.1.037.
- Menzel WP, Schmit TJ, Zhang P, Li J. 2018. Satellite-based atmospheric infrared sounder development and applications. Bull Am Meteorol Soc. 99(3):583–603. doi: 10.1175/BAMS-D-16-0293.1.
- Miller SM, Wofsy SC, Michalak AM, Kort EA, Andrews AE, Biraud SC, Dlugokencky EJ, Eluszkiewicz J, Fischer ML, Janssens-Maenhout G, et al. 2013. Anthropogenic emissions of methane in the United States. Proc Natl Acad Sci USA. 110(50):20018–20022. doi: 10.1073/pnas.1314392110.
- Moss A, Jouany J-P, Newbold J. 2000. Methane production by ruminants: its contribution to global warming. Ann Zootech. 49(3):231–253. doi: 10.1051/animres:2000119.
- NC. 2014. [accessed 2023 Sept. 9] https://www.namakwa-dm.gov.za/wp-content/uploads/2023/04/Northern-Cape-Climate-Change-Response-Plan-Namakwa-2023.pdf.
- Pagano TS, Aumann HH, Gaiser SL, Gregorich DT. 2003. Early Calibration Results from the Atmospheric Infrared Sounder (AIRS) on Aqua. Proceedings of the SPIE 4891, Optical Remote Sensing of the Atmosphere and Clouds III, Hangzhou, China; p. 76–83. doi: 10.1117/12.465869.
- Prather MJ, Holmes CD, Hsu J. 2012. Reactive greenhouse gas scenarios: systematic exploration of uncertainties and the role of atmospheric chemistry. Geophys Res Lett. 39(9):L09803. doi: 10.1029/2012GL051440.
- Scoones I. 2023. Livestock, methane, and climate change: the politics of global assessments. Wiley Interdiscip Rev Clim Change. 14(1):e790. doi: 10.1002/wcc.790.
- Shibata M, Terada F. 2010. Factors affecting methane production and mitigation in ruminants. Anim Sci J. 81(1):2–10. doi: 10.1111/j.1740-0929.2009.00687.x.
- Shikwambana L, Kganyago M, Xulu S. 2022a. Analysis of wildfires and associated emissions during the recent strong ENSO phases in Southern Africa using multi-source remotely-derived products. Geocarto Int. 37(27):16654–16670. doi: 10.1080/10106049.2022.2113449.
- Shikwambana L, Mokgoja B, Mhangara P. 2022b. A Qualitative Assessment of the Trends, Distribution and Sources of Methane in South Africa. Sustainability. 14(6):3528. doi: 10.3390/su14063528.
- Skeie RB, Hodnebrog Ø, Myhre G. 2023. Trends in atmospheric methane concentrations since 1990 were driven and modified by anthropogenic emissions. Commun Earth Environ. 4(1):317. doi: 10.1038/s43247-023-00969-1.
- Solomon S, Plattner GK, Knutti R, Friedlingstein P. 2009. Irreversible climate change due to carbon dioxide emissions. Proc Natl Acad Sci USA. 106(6):1704–1709. doi: 10.1073/pnas.0812721106.
- Subak S, Craighill A. 1999. The contribution of the paper cycle to global warming. Mitig Adapt Strateg Glob Change. 4(2):113–136. doi: 10.1023/A:1009683311366.
- Sucevic N, Djurisic Z. 2012. Influence of Atmospheric Stability Variation on Uncertainties of Wind Farm Production Estimation. In Proceedings of European Wind Energy Conference & Exhibition; April 16–19; Copenhagen, Denmark,
- Tedeschi LO, Abdalla AL, Álvarez C, Anuga SW, Arango J, Beauchemin KA, Becquet P, Berndt A, Burns R, De Camillis C, et al. 2022. Quantification of methane emitted by ruminants: a review of methods. J Anim Sci. 100(7):1–22. doi: 10.1093/jas/skac197.
- Tilstra LG, de Graaf M, Wang P, Stammes P. 2020. In-orbit Earth reflectance validation of TROPOMI on board the Sentinel-5 Precursor satellite. Atmos Meas Tech. 13(8):4479–4497. doi: 10.5194/amt-13-4479-2020.
- Theys N, De Smedt I, Yu H, Danckaert T, Van Gent J, Hörmann C, Wagner T, Hedelt P, Bauer H, Romahn F, et al. 2017. Sulfur dioxide retrievals from TROPOMI onboard Sentinel-5 Precursor: algorithm theoretical basis. Atmos Meas Tech. 10(1):119–153. doi: 10.5194/amt-10-119-2017.
- Thompson LR, Rowntree JE. 2020. Invited Review: methane sources, quantification, and mitigation in grazing beef systems. Appl Anim Sci. 36(4):556–573. doi: 10.15232/aas.2019-01951.
- Turner AJ, Frankenberg C, Kort EA. 2019. Interpreting contemporary trends in atmospheric methane. Proc Natl Acad Sci USA. 116(8):2805–2813. doi: 10.1073/pnas.1814297116.
- UN Environmental Programme. 2021. [accessed 2023 Aug 25] https://www.unep.org/news-and-stories/story/methane-emissions-are-driving-climate-change-heres-how-reduce-them.
- Van Amstel A. 2012. Methane. A review. J Integr Environ Sci. 9(Suppl. 1):5–30. doi: 10.1080/1943815X.2012.694892.
- Van Dingenen R, Crippa M, Maenhout G, Guizzardi D, Dentener F. 2018. Global trends of methane emissions and their impacts on ozone concentrations. Luxembourg: Publications Office of the European Union. doi: 10.2760/820175.
- Veefkind JP, Aben I, McMullan K, Förster H, de Vries J, Otter G, Claas J, Eskes HJ, de Haan JF, Kleipool Q, et al. 2012. TROPOMI on the ESA Sentinel-5 Precursor: a GMES mission for global observations of the atmospheric composition for climate, air quality and ozone layer applications. Remote Sens Environ. 120:70–83. doi: 10.1016/j.rse.2011.09.027.
- Wang Z, Zeng D, Patrick WH. 1996. Methane emissions from natural wetlands. Environ Monit Assess. 42(1–2):143–161. doi: 10.1007/BF00394047.
- Wright MA, Grab SW. 2017. Wind speed characteristics and implications for wind power generation: cape regions, South Africa. S Afr J Sci. 113(7/8):8. doi: 10.17159/sajs.2017/20160270.
- Xiong X, Butler JJ. 2020. MODIS and VIIRS calibration history and future outlook. Remote Sens. 12(16):2523. doi: 10.3390/rs12162523.
- Xu G, Li Y, Wang S, Kong F, Yu Z. 2019. An overview of methane emissions in constructed wetlands: how do plants influence methane flux during the wastewater treatment? J Freshw Ecol. 34(1):333–350. doi: 10.1080/02705060.2019.1588176.
- Yin X, Jiang C, Xu S, Yu X, Yin X, Wang J, Maihaiti M, Wang C, Zheng X, Zhuang X. 2023. Greenhouse gases emissions of constructed wetlands: mechanisms and affecting factors. Water. 15(16):2871. doi: 10.3390/w15162871.
- Yu G, Wang G, Chi T, Du C, Wang J, Li P, Zhang Y, Wang S, Yang K, Long Y, et al. 2022. Enhanced removal of heavy metals and metalloids by constructed wetlands: a review of approaches and mechanisms. Sci Total Environ. 821:153516. doi: 10.1016/j.scitotenv.2022.153516.