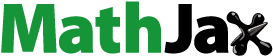
Abstract
In this article, a multi-source data-based method for urban-rural fringe extraction is proposed to solve the problem of insufficiently accurate division of urban-rural fringe, which can conveniently and accurately realise the extraction of the area of urban-rural fringe. The method uses multi-source data such as night light remote sensing images, POI data and high-resolution remote sensing images. We constructed the Combined Urban-Rural Fringe Index (CUFI) model based on the characteristics of NDVI and NTL, POI kernel density value points that change from the city centre to the surrounding area. The calculation of CUFI involves three aspects. The initial step involves processing data, which includes reducing noise in nighttime light images and removing inaccurate information from POI data. The subsequent step involves calculating combined features of NTL and POI kernel density, which includes analyzing the kernel density of POI data, standardizing the results of POI kernel density and nighttime light data, computing NTL & POI indexes using the equal weight combined feature calculation method and reclassifying NTL & POI indexes .The third part involves rejecting misinformation using NDVI. This includes calculating the NDVI index using high-resolution satellite data, rejecting bare land information in the NDVI index using nighttime light data, and reclassifying the processed results. The reclassified results are then multiplied with the reclassification results of the NTL&POI index to obtain the CUFI calculation results. In order to verify the effectiveness and reliability of the method, Tangshan City, Hebei Province was selected as the experimental area, and the method was used to compare and analyse the accuracy with the traditional urban built-up area boundary extraction method. The results show that compared with the traditional method, the extraction accuracy of the CUFI method is higher, reaching 89.51%, which is able to effectively identify urban villages and lakes in the urban area, as well as improving the resolution of extracting Urban-Rural Fringe using NTL& POI methods, and also the urban-rural fringe which has weak night light and fewer POI points, but actually has urban-rural duality attributes Extraction.
1. Introduction
The earliest concept of Urban-Rural Fringe was the ‘Stadtrand Zonen’ proposed by the German geographer Lewis Herbert (Louis Citation1936). The definition of Urban-Rural Fringe was gradually refined by some subsequent scholars (Pryor Citation1968, Chen Citation1995). With the continuous development of society and the economy, the built-up area of cities is increasing year by year, and the boundaries between urban and rural areas are becoming more and more blurred, resulting in the emergence of zones between urban and rural areas with both urban and rural dualities, namely the urban-rural fringe. The urban-rural fringe is a transition zone between urban and rural areas, including the transition from high-density to low-density population, from urban to agricultural land, and from high-density to low-density services (Chen et al. Citation2021).
The urban-rural fringe is in a state of dynamic change, with the urban fringe gradually moving towards the cities, and the rural fringe continuing to expand and move into the countryside. In the process of urban-rural integration, the urban-rural fringe plays an important role. Therefore, identifying the urban-rural fringe is of great significance for urban construction planning, rural revitalisation, urban-rural integration and urban economic transformation (Basu Citation2000).
Due to their spatial and temporal continuity, night light data are widely used for urban system analysis (Han et al. Citation2018, Che and Gamba Citation2019, Shi et al. Citation2023), urban built-up area extraction (Wang et al. Citation2018, Zhai et al. Citation2020, Li et al. Citation2020, Xiong et al. Citation2020, Bu et al. Citation2022), urban centre identification (Lu et al. Citation2024), urban sprawl analysis (Liu et al. Citation2012, Xiao et al. Citation2014, Xin et al. Citation2017, Xu et al. Citation2023), urbanisation dynamics study (Yu et al. Citation2018, Zheng et al. Citation2019, Li et al. Citation2022, Wang et al. Citation2022) and urban-rural fringe delineation (Yu et al. Citation2023). High-resolution remote sensing data can be accurately extracted for land use data, surface coverage data (Pozzi and Small Citation2005) and urban built-up area range (Fen Citation2012, Zhang et al. Citation2014, Bai et al. Citation2022). Point of Information (POI) data contains precise location information and rich attribute information. It has a fast update speed, wide coverage, and urban-rural distribution differences. POI data is widely used in urban functional zone delineation (Zhang et al. Citation2017, Wang et al. Citation2021), community life circle research (Li et al. Citation2019), land use analysis (Yao et al. Citation2016)and urban-rural integration area delineation (Yu et al. Citation2023) and other research areas.
Early methods of extracting data for the urban-rural fringe primarily focused on the distance from the core area (Bryant and Russwurm Citation1979), population (Gu Citation1993, Zhang and Xu Citation1997), and commuting distance (Sharp and Clark Citation2008).
Lin et al. used the threshold value method and spatial superposition method to identify Urban-Rural Fringe territories based on non-agricultural construction density and land tenure characteristics (Lin et al. Citation2007). Zhang et al. constructed a combined value model for lighting luminance and lighting luminance undulation features for the first time. The combined features of lighting luminance and lighting luminance undulation were analysed and classified using the breakpoint method for the spatial identification of the Urban-Rural Fringe. Some scholars have also used impermeable surface analysis for the Urban-Rural Fringe (Zhang et al. Citation2016). Yu et al. combined features were constructed based on the luminance value of the lighting and the change value of the kernel density of points of interest to identify the Urban-Rural Fringe in Wuhan (Yu et al. Citation2023).
Previous studies have analysed cities using nighttime light data and other economic, demographic, and GPS data. However, there are fewer extraction theories for the Urban-Rural Fringe, and most of them only consider the urban structure of the urban-rural dichotomous structure, without taking into account the urban-rural ternary structure. Currently, extraction methods for the Urban-Rural Fringe primarily rely on nighttime light data and POI data. However, early nighttime light data suffers from ‘saturation’ and ‘overflow’ effects, leading to blurred boundaries between urban and rural areas. As a result, urban villages and fringes are often mistakenly extracted. The accuracy of extracting the Urban-Rural Fringe at the edge of the city is insufficient. Recent nighttime light data, such as NPP-VIIRS, has reduced the effects of ‘saturation’ and ‘spillover’. Many scholars have proposed desaturation methods in response to the saturation spillover effect of early nighttime light data (Zhang et al. Citation2013, Zheng et al. Citation2019). However, the limited resolution of nighttime light data results in low-resolution and inaccurate data extracted from urban and rural fringe zones. Although scholars have introduced POI data, the improvement in accuracy is limited. High-resolution remote sensing images can be more effective in addressing the problem of poor extraction accuracy of urban-rural Fringe aggregates.
2. Overview of the study area
Tangshan City is situated in the northeastern part of the North China Plain and the eastern part of Hebei Province, adjacent to Beijing and Tianjin. It is administratively divided into 7 municipal districts (Lunan, Lubei, Guye, Kaiping, Fengnan, Fengrun, and Caofeidian), 4 counties (Luannan, Leting, Qiansi, and Yutian), and 3 county-level cities (Zunhua, Qian’an, and Luanzhou). Tangshan is a provincial vice-centre city in Hebei province and is also one of the central cities identified by the State Council’s approval. It is an important part of the Beijing-Tianjin-Tangshan industrial zone, with a GDP of RMB 89.07 billion in 2022, ranking 27th in China.
Previous studies have mainly focused on single-centre megacities and mega-cities, with less attention given to multi-centre cities. Monocentric cities typically have a single core urban area that radiates to the surrounding region. In contrast, polycentric cities have two or more non-contiguous core urban areas. The spatial distribution of the urban-rural Fringe differs significantly between these two types of cities.
Tangshan City is a typical polycentric city with a development goal of one port and two cities. Tangshan’s industrial characteristics and development strategy have resulted in a multi-centre distribution of the city, with significant differences in urbanization levels and the proportion of Urban-Rural Fringe in each county and district. The central urban area, comprising Lubei District, Lunan District, Fenrun District, and Caofeidian District, has a high level of urbanization, while the peripheral urban areas have lower rates. The selection of Tangshan City as the study area holds great significance for the analysis of urban structure and multi-centre cities. The location of Tangshan City is shown in .
3. Research methodology
Nighttime light images can be useful for identifying population and economic distribution. Some scholars introduced points of interest (POI) to improve the identification accuracy of urban-rural Fringe. However, relying solely on NTL and POI kernel density is insufficient for some Urban-Rural Fringe areas that have both urban and rural attributes, but have weak nighttime light and fewer POI points.
Furthermore, the changes in POI kernel density and NTL undulation in urban areas and the urban-rural fringe are not significant, which may lead to misclassification. Additionally, the accuracy of NTL and POI for the urban-rural fringe is limited due to the resolution of the POI and nighttime light data (500 m).
It has been noted that there is a strong negative correlation between the degree of vegetation cover and impervious surface (Sutton et al. Citation2001). To desaturate the nighttime light index, NDVI was used. This method involves using high-resolution remote sensing satellite data to calculate NDVI. The previous method has been improved by taking into account the changing characteristics of NDVI values in urban, urban-rural fringe, and rural areas. The specific characteristic control values are shown in .
Table 1. Comparative table of characteristics of various types of districts in Tangshan city.
During the process of urbanisation, ‘villages in the city’ refer to two types of settlements. The first type includes villages that have lost or mostly lost their arable land due to urban development but still maintain their autonomy and collective ownership of rural areas. The second type includes settlements that have emerged after the expropriation of most or all of the arable land, where farmers have become residents but still reside in their original villages. As both urban villages and parks are situated within the urban built-up area, we consider urban villages as part of the city in our study of Urban-Rural Fringe extraction.
Using the Tangshan Research Institute of Beijing Jiaotong University as the reference point, a profile line was drawn in an easterly direction on the NPP-VIIRS nighttime light remote sensing image of Tangshan City. The profile analysis included NDVI, NPP-VIIRS nighttime light remote sensing image normalized value, and point-of-interest (POI) kernel density normalized value. The results are presented in .
We propose a method for extracting Urban-Rural Fringe based on nighttime light images, POI kernel density and high-resolution remote sensing satellite images. The method is based on the correlation characteristics of NDVI with NTL and POI kernel density values, which change from the city centre to the surrounding area. The calculation of CUFI involves three aspects.
The initial step involves processing data, which includes reducing noise in nighttime light images and removing inaccurate information from POI data. The subsequent step involves calculating combined features of NTL and POI kernel density, which includes analyzing the kernel density of POI data, standardizing the results of POI kernel density and nighttime light data, computing NTL & POI indexes using the equal weight combined feature calculation method and reclassifying NTL & POI indexes. The third part involves rejecting misinformation using NDVI. This includes calculating the NDVI index using high-resolution satellite data, rejecting bare land information in the NDVI index using nighttime light data, and reclassifying the processed results. The reclassified results are then multiplied with the reclassification results of the NTL&POI index to obtain the CUFI calculation results.
NPP-VIIRS nighttime light data and Landsat 8 Collection 2 Level 2 high-resolution satellite remote sensing data were chosen to validate the CUFI. Other nighttime light data, such as DMSP and Luojia I, as well as other high-resolution remote sensing satellite data, can also be used for computation. The detailed operation of each process will be introduced in the following sections.
3.1. Date
In order to visualise the source and resolution of the data, we have created a table to display this information, which is shown in . Please contact the first author of this article if you need this data.
Table 2. Data sources and resolution.
3.2 Pre-processing
3.2.1. Noise reduction of nighttime light data
Preprocessing was necessary to filter out lighting noise from the NPP-VIIRS nighttime light averaged cloud-free synthetic annual images, as they did not contain outliers caused by extremely bright pixels or other factors resulting from transient lighting over a short period of time. The maximum lighting threshold (Dlmax) for Tangshan Research Institute of Beijing Jiaotong University was selected based on the highest lighting data value in the surrounding commercial area of Tangshan City, which has the highest NTL value in the city. This threshold is used to filter out extremely bright pixel values. Tangshan City District has two large lakes: Steep River Reservoir and South Lake. South Lake is a park close to the city, which results in higher lighting values in and around the park. To avoid this issue, we chose Steep River Reservoir for sampling. We recorded the light pixel values at this location and calculated the average pixel value as the Minimum lighting threshold (Dlmin). Following the principle of 0 for large inland waters, areas smaller than the minimum light threshold were assigned a value of 0.
3.2.2. Pre-processing of POI data
To prepare the data for kernel density analysis in ArcMap 10.8, we used Excel to remove duplicates and errors.
3.3. NTL & POI (NP) combination calculation methods
3.3.1. Kernel density analysis method
The method of kernel density analysis is frequently used to indicate the level of aggregation of geographic elements in spatial distribution. When conducting kernel density analysis for POIs, the experimental results are greatly influenced by the bandwidth value. After comparative analysis, a bandwidth of 50 m was selected for the analysis (Zheng et al. Citation2019). The study area’s points of interest (POIs) were analysed for their spatial distribution characteristics using Arcmap 10.8’s kernel density analysis tool. A higher kernel density value indicates a more aggregated spatial distribution of POIs, while a lower kernel density value indicates a more sparse spatial distribution of POIs. EquationEquation (1)(1)
(1) shows the specific formula.
(1)
(1)
where
epresents the calculated value of kernel density at each i point in space, R denotes the search radius of the kernel density function, n refers to the total number of j points belonging to the study object within the bandwidth R, and
is the weight of each j point, where R is greater than
the Euclidean distance between the i point and j point.
3.3.2. Normalized processing
Normalization of the data values within the range of 0–1 mitigates the influence of the nighttime light imagery and the kernel density values of the points of interest due to varying values. The formula for this normalization is exhibited in EquationEqs. (2)(2)
(2) and Equation(3)
(3)
(3) .
(2)
(2)
(3)
(3)
where
and
are the normalised values of Tangshan NPP-VIIRS nighttime lighting image brightness and point-of-interest kernel density, respectively.
are the minimum and maximum values of Tangshan NPP-VIIRS nighttime lighting image brightness value,
and POI kernel density value,
respectively.
3.3.3. Calculation method for combined features
With reference to the coordination formula (Zhang et al. Citation2016), we have improved the method of calculating the mean value (EquationEq. (4)(4)
(4) ) and proposed the NTL&POI (NP) combination calculation method (Yu et al. Citation2023). This method uses equal weights of NTL and POI for the combined feature calculation of NTL and POI kernel density. Compared to the previous method, it retains more information on Urban-Rural Fringe and rural areas, which is convenient for later multiplication calculation with NV results. illustrates the specific differences.
(4)
(4)
(5)
(5)
Using Tangshan Research Institute of Beijing Jiaotong University as the focal point, we conducted a profile analysis of NPP & NDVI along the east and south directions, yielding the results depicted in .
The NTL&POI values exhibit three stages of change as the distance from the centre increases: a rapid decrease in the urban area, a rise followed by a decrease in the Urban-Rural Fringe, and a slow decay in the countryside.
The two turning points of the three phases were selected as the thresholds for reclassification of the NP index. The results shown in were obtained after multivariate processing. The reclassified results were then processed to a raster size of 30 m using the resampling tool to facilitate subsequent multiplication.
Table 3. Comparison table of NTL&POI index features.
3.4 Error message rejection using NDVI
3.4.1. NDVI & NL (NV) index calculation
The normalised vegetation index index (NDVI) is widely used for vegetation extraction. Due to the existence of a large number of built-up areas interspersed with bare land in Urban-Rural Fringe zones, the spectral features of the two types of features in Landsat 8 Collection 2 Level 2 images are similar and easy to form mixed pixels, which is difficult to distinguish on multispectral images .NPP-VIIR nighttime light remote sensing images were introduced to exclude the bare land and low-density vegetation cover information from NDVI. The NDVI is shown in EquationEq. (6)(6)
(6) .
(6)
(6)
where NIR is the near-infrared band and R is the visible red band.
The NDVI was processed using the NPP-VIIRS results after noise reduction. The difference in grey values of nighttime light remote sensing imagery was used to distinguish between built-up urban areas, Urban-Rural Fringe, and low-density vegetation cover areas. The nighttime light remote sensing images and NDVI were separately binarised, with urban land and Urban-Rural Fringe assigned a value of 1 and non-urban land assigned a value of 0. The NDVI&NL index was obtained by multiplying the binarised NTL and NDVI results. The resulting values of 0 and 1 represent non-urban and urban land, respectively. To facilitate subsequent multiplication with the NP index, the binarisation results need to be reclassified according to the feature comparison table. To achieve the purpose of excluding bare land and areas with low-density vegetation cover, it is appropriate to lower the threshold for binarisation of the NL index when introducing NPP-VIIRS. The NDVI&NL index can be obtained by multiplying the two shows a comparison between the indices and the features.
Table 4. NDVI&NTL index feature comparison table.
3.4.2 CUFI index calculation
The Urban-Rural Fringe Index was calculated by multiplying the reclassification results of the combined NTL&POI(NP) with the NDVI&NTL(NV) results; see for a detailed feature comparison.
(7)
(7)
Table 5. CUFI comparison table.
The CUFI was reclassified based on the actual feature results, and the findings are presented in .
4. Extraction results
4.1. Identification results of urban-rural fringe
The analysis indicates that Tangshan City has a total urban area of 335 square kilometers, equating to 2.49% of the overall city area. The urban-rural fringe area is 1,810 square kilometers, which is 5.24% of the total city area. The remaining area consists of countryside, which encompasses approximately 124,300 square kilometers or 92.27% of the city’s total area.
To examine the constraints on the direction of urban expansion, the study compared the DEM data and lake data of Tangshan City with the extracted results of the urban-rural fringe districts. The analysis indicated that topography and lakes significantly influence the direction of urban expansion in Tangshan City. Lakes including South Lake, Steep River Reservoir, Qiuzhuang Reservoir (Hyeongxianghe River), Panjiakou Reservoir, Dahaiting (Luanhe River), and others exert a greater influence on the direction of urban expansion, whereas topography plays a more restrictive role in shaping the direction of urban expansion in counties and districts such as Qian’an City, Zunhua City, and Qianxi County.
4.2. Verification of the extraction accuracy of urban-rural fringe
Overall Accuracy and Kappa Coefficient are commonly used to verify the accuracy of classification results. Overall Accuracy is used to express the degree of correct classification of all image elements, while Kappa Coefficient is mainly used to express the accuracy of the whole image classification results. The larger the Kappa Coefficient, the higher the degree of consistency between the classification results and the actual features. Conversely, the smaller the Kappa Coefficient, the lower the degree of consistency between the classification results and the actual features.
We chose two types of counties: one is a more economically developed city district (Fengrun District), and the other is an economically underdeveloped county (Yutian County). We made this selection based on the results of visual interpretation of Google Maps and fieldwork. We manually created the correct classification image of the three methods to verify accuracy and classification comparisons. The specific rubbing of yellow is shown in and .
Compared to the NPP undulation combination method and the NPP&POI combination method, the CUFI combination method demonstrates higher accuracy. It effectively excludes urban villages and lakes within the urban area, supplements information on the urban-rural fringe, which has weak nighttime light and fewer POI points but exhibits urban-rural duality attributes, and attenuates errors caused by the resolution phenomenon of nighttime light remote sensing images and POI kernel density values.
It is important to note that urban villages are also considered urban areas in the classification results. The urban area in CUFI comprises both urban villages and urban built-up areas, with built-up area of 264 km2 and Urban village and park areas of 71 km2. For details, please see .
Table 6. Classifies the results of the three methods.
Table 7. Comparison table of the accuracy of the classification results of the three methods %.
5. Discussion
5.1. Innovative points
The Combined urban-rural junction index, constructed using multi-source data, effectively distinguishes between the city, urban-rural fringe, and countryside.
The method combines features of nighttime light data and POI data, and uses the reference coordination degree method to improve upon the method excluded by Yu et al.(Zhang et al. Citation2016, Yu et al. Citation2023). These results in the retention of more information about the Urban-Rural Fringe compared to the method of Yu et al. According to the theory of negative correlation between plant cover and density of urban built-up areas and the correlation between NDVI and NTL& POI kernel density value points change from the city centre to the surrounding areas, NDVI data are introduced to supplement the Urban-Rural Fringe, which has urban-rural duality attributes but weaker nighttime light and fewer POI points. This supplementation is also necessary for the urban-rural fringe that is excluded due to nighttime light data and POI resolution problems. The resolution of the CUFI extraction results has been further improved with the introduction of high-resolution remote sensing images.
5.2. Shortcomings and prospects
The effectiveness of CUFI in extracting Urban-Rural Fringe in typical multi-centre cities in China is demonstrated. This method shows improved accuracy compared to the original method that relies solely on POI data and the traditional method that relies on nighttime light and POI data.
However, it is important to note that this method still has limitations. The degree of change in vegetation density from urban centres to the countryside is used as the key to extract the Urban-Rural Fringe. While this approach can improve accuracy, it may also introduce errors. For instance, in certain developed nations, urban areas have more greenery, resulting in a higher degree of vegetation cover in the city centre and a corresponding increase in NDVI values. Therefore, the method of relying solely on NDVI to extract the urban-urban interface has not been verified in such cities. Secondly, CUFI relies on the coupling of point of interest (POI) data and nighttime light data. While recent POI data is easily obtainable, obtaining older data can be challenging, which poses difficulties for long-term research using this method.
6. Conclusion
The current method of combining NTL and POI data has a significant error in identifying the Urban-Rural Fringe and Urban Village due to data resolution issues. The NTL and NTL undulation combination method only relies on the undulation of nighttime light remote sensing images to distinguish between the Urban-Rural Fringe and Urban-Rural Fringe, with relatively weak accuracy.
To address this issue, we propose a method based on multi-source data, such as NPP-VIIRS nighttime light remote sensing images, POI data, and Landsat 8 Collection 2 Level remote sensing images. A combined Urban-Rural Fringe index model is constructed using the correlation characteristics of NDVI and nighttime light remote sensing images and POI kernel density value points changing from the city centre to the surrounding area.
Compared to the traditional method, the CUFI method has a higher extraction accuracy. It effectively eliminates information on urban villages and lakes within the urban area and solves the accuracy problem caused by the resolution of nighttime light remote sensing images and POI kernel density values. This allows for the effective identification of urban-rural fringes with weak nighttime light and fewer POI points, but with actual urban-rural duality attributes.
Disclosure statement
No potential conflict of interest was reported by the authors.
Additional information
Funding
References
- Bai M, Zhang S, Wang X, Feng Y, Wang J, Peng P. 2022. Deep semantic segmentation for rapid extraction and spatial-temporal expansion variation analysis of China’s urban built-up areas. Front Earth Sci. 10:883779. doi: 10.3389/feart.2022.883779.
- Basu B. 2000. Rural–urban migration, urban unemployment and the structural transformation of a dual economy. J Int Trade Econ Develop. 9(2):137–149. doi: 10.1080/09638190050028144.
- Bryant C, Russwurm L. 1979. The impact of non-farm development on agriculture: a synthesis. PlanCanada. 1979(2):122–139.
- Bu L, Dai D, Tu L, Zhang Z, Deng M, Xie X. 2022. An STP-HSI index method for urban built-up area extraction based on multi-source remote sensing data. R Soc Open Sci. 9(11):220597–220597. doi: 10.1098/rsos.220597.
- Che M, Gamba P. 2019. Intra-urban change analysis using sentinel-1 and nighttime light data. IEEE J Sel Top Appl Earth Observ Remote Sens. 12(4):1134–1142. doi: 10.1109/JSTARS.2019.2899881.
- Chen M, Zhou Y, Huang X, Ye C. 2021. The integration of new-type urbanization and rural revitalization strategies in China: origin, reality and future trends. Land. 10(2):207–207. doi: 10.3390/land10020207.
- Chen Y. 1995. Urban-rural staggered zone nomenclature debate. Geograph Geo-Info Sci. (1):47.
- Fen Z. 2012. RS and GIS-based analysis on dynamic changes of landscape pattern in urban-rural fringe. J Anhui Agri Sci. 40(9):5382–5385+5656.
- Gu CL. 1993. The study of the urban fringes in Chinese megalopolises. Acta Geographica Sinica. 48(4):317–328.
- Han X, Tana G, Qin K, Letu H. 2018. Estimating industrial structure changes in china using DMSP—OLS night-time light data during 1999–2012. Int Arch Photogramm Remote Sens Spatial Inf Sci. XLII–3/W5: 9–15. doi: 10.5194/isprs-archives-XLII-3-W5-9-2018.
- Li F, Yan Q, Bian Z, Liu B, Wu Z. 2020. A POI and LST adjusted NTL urban index for urban built-up area extraction. Sensors. 20(10):2918. doi: 10.3390/s20102918.
- Li T, Guo Z, Ma C. 2022. Dynamic characteristics of urbanization based on nighttime light data in China’s “Plain–mountain transition zone. Int J Environ Res Public Health. 19(15):9230–9230. doi: 10.3390/ijerph19159230.
- Li Z, Zheng J, Zhang Y. 2019. Study on the layout of 15-minute community-life circle in third-tier cities based on POI: Baoding city of Hebei Province. ENG. 11(9):592–603. doi: 10.4236/eng.2019.119041.
- Lin J, et al. 2007. A study on territorial identification and land use of urban-rural fringe—a case study of Beijing central city area. Urban Planning. 31(8):9.
- Liu Z, He C, Zhang Q, Huang Q, Yang Y. 2012. Extracting the dynamics of urban expansion in China using DMSP-OLS nighttime light data from 1992 to 2008. Landscape and Urban Planning. 106(1):62–72. doi: 10.1016/j.landurbplan.2012.02.013.
- Louis H. 1936. Die geographische Gliederung von Gross-Berlin. Englehorn: Stuttgart.
- Lu Y, Gong M, Lu L, Wang Y, Wang Y. 2024. Urban polycentrism and total-factor energy efficiency: an analysis based on the night light data. Technol Forecasting Social Change. 198:122984. doi: 10.1016/j.techfore.2023.122984.
- Pozzi F, Small C. 2005. Analysis of urban land cover and population density in the United States. Photogramm Eng Remote Sens. 71(6):719–726. doi: 10.14358/PERS.71.6.719.
- Pryor RJ. 1968. Defining the rural-urban fringe. Social Forc. 47(2):202. doi: 10.2307/2575150.
- Sharp JS, Clark JK. 2008. Between the country and the concrete: rediscovering the rural-urban fringe. City Commun. 7(1):61–79. doi: 10.1111/j.1540-6040.2007.00241.x.
- Shi K, Wu Y, Liu S, Chen Z, Huang C, Cui Y. 2023. Mapping and evaluating global urban entities (2000–2020): a novel perspective to delineate urban entities based on consistent nighttime light data. GIScience & Remote Sens. 60(1):2161199. doi: 10.1080/15481603.2022.2161199.
- Sutton P, Roberts D, Elvidge C, Baugh K. 2001. Census from heaven: an estimate of the global human population using night-time satellite imagery. Int J Remote Sens. 22(16):3061–3076. doi: 10.1080/01431160010007015.
- Wang J, Qiu S, Du J, Meng S, Wang C, Teng F, Liu Y. 2022. Spatial and temporal changes of urban built-up area in the yellow river basin from nighttime light data. Land. 11(7):1067–1067. doi: 10.3390/land11071067.
- Wang L, Zhu J, Xu Y, Wang Z. 2018. Urban built-up area boundary extraction and spatial-temporal characteristics based on land surface temperature retrieval. Remote Sens. 10(3):473–473. doi: 10.3390/rs10030473.
- Wang Z, Ma D, Sun D, Zhang J. 2021. Identification and analysis of urban functional area in Hangzhou based on OSM and POI data. PLoS ONE. 16(5):e0251988–e0251988. doi: 10.1371/journal.pone.0251988.
- Xiao P, Wang X, Feng X, Zhang X, Yang Y. 2014. Detecting China’s urban expansion over the past three decades using nighttime light data. IEEE J Sel Top Appl Earth Observ Remote Sens. 7(10):4095–4106. doi: 10.1109/JSTARS.2014.2302855.
- Xin X, Liu B, Di K, Zhu Z, Zhao Z, Liu J, Yue Z, Zhang G. 2017. Monitoring urban expansion using time series of night-time light data: a case study in Wuhan, China. Int J Remote Sens. 38(21):6110–6128. doi: 10.1080/01431161.2017.1312623.
- Xiong H, et al. 2020. Using wavelet transforms to fuse nighttime light data and POI Big data to extract urban built-up areas. Remote Sens. 12(23):3887–3887.
- Xu Y, Hao S, Cui Y, Li P, Sheng L, Liao C. 2023. Analysis of the spatiotemporal expansion and pattern evolution of urban areas in Anhui Province, China, based on nighttime light data. Ecol Indicators. 157:111283. doi: 10.1016/j.ecolind.2023.111283.
- Yao Y, Li X, Liu X, Liu P, Liang Z, Zhang J, Mai K. 2016. Sensing spatial distribution of urban land use by integrating points-of-interest and google word2vec model. Int J Geograph Info Sci. 31(4):825–848. doi: 10.1080/13658816.2016.1244608.
- Yu B, Lian T, Huang Y, Yao S, Ye X, Chen Z, Yang C, Wu J. 2018. Integration of nighttime light remote sensing images and taxi GPS tracking data for population surface enhancement. Int J Geograph Info Sci. 33(4):687–706. doi: 10.1080/13658816.2018.1555642.
- Yu J, Meng Y, Zhou S, Zeng H, Li M, Chen Z, Nie Y. 2023. Research on spatial delineation method of urban-rural fringe combining poi and nighttime light data—taking Wuhan city as an example. Int J Environ Res Public Health. 20(5):4395–4395. doi: 10.3390/ijerph20054395.
- Zhai W, Han B, Cheng C. 2020. Evaluation of Luojia 1-01 nighttime light imagery for built-up urban area extraction: a case study of 16 cities in China. IEEE Geosci Remote Sensing Lett. 17(10):1802–1806. doi: 10.1109/LGRS.2019.2955496.
- Zhang J, Li P, Wang J. 2014. Urban built-up area extraction from Landsat TM/ETM + images using spectral information and multivariate texture. Remote Sens. 6(8):7339–7359. doi: 10.3390/rs6087339.
- Zhang J, Xu X. 1997. Review and perspective of the studies on rural-urban fringe. Human Geography. 12(3):9–12.
- Zhang Q, Schaaf C, Seto KC. 2013. The vegetation adjusted NTL urban index: A new approach to reduce saturation and increase variation in nighttime luminosity. Remote Sens Environ. 129(2):32–41. doi: 10.1016/j.rse.2012.10.022.
- Zhang X, Du S, Wang Q. 2017. Hierarchical semantic cognition for urban functional zones with VHR satellite images and POI data. ISPRS J Photogram Remote Sens. 132:170–184. doi: 10.1016/j.isprsjprs.2017.09.007.
- Zhang Z, Zhang A,Guo H. 2016. Research on spatial identification of Urban-Rural Fringe based on dmsp/ols nighttime light data—a case study of Chongqing main city. Geograph Geographic Info Sci. 32(6):6.
- Zheng H, Gui Z, Li F, Wu H, Wu X, Zhang J, Han W. 2019. A built-up area extraction method combining nighttime light data and point-of-interest data. Geograph Geographic Info Sci. 35(2):25–32.
- Zheng Z, Chen Y, Wu Z, Ye X, Guo G, Qian Q. 2019. The desaturation method of DMSP/OLS nighttime light data based on vector data: taking the rapidly urbanized China as an example. Int J Geograph Info Sci. 33(3):431–453. doi: 10.1080/13658816.2018.1538516.