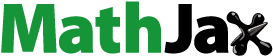
Abstract
Timely and objective assessment of the optimal season for the construction of remote sensing ecological index (RSEI) is of great significance for accurate and effective assessment of ecological environment quality. We manipulated RSEI in to monitor seasonal variations in ecological environment quality (EEQ) in the Beijing-Tianjin-Hebei (JJJ) region from 2001 to 2020. First, we evaluated image quality across all four seasons and filled in missing observations through liner interpolation. Second, Seasonal RSEI was constructed using MODIS and compared across different years. Third, temporal and spatial variations within the same seasons in EEQ. Additionally, Moran’s I was utilized to evaluate spatial autocorrelation of EEQ, and the stability of the correlation between RSEI and four indicators seasonal indicators was compared. The results showed that: 1) the PC1 component concentrates most of the characteristics of the four indicators, especially in summer (over 71%); 2) the Moran’ I in the summer of 2001, 2006, 2011, 2016 and 2020 are 0.909, 0.898, 0.917, 0.921 and 0.892, respectively, which indicated that the EEQ has a strong positive spatial correlation. 3) the correlation between the four indicators and summer RSEI showed high correlation in different years, and the standard deviation of the correlation between the four indicators and RSEI fluctuated most slightly in summer, which the std of NDVI, WET, LST and, NDBSI were 0.005, 0.052, 0.026 and 0.017, respectively. This study theoretically demonstrates that summer is the optimal season for constructing RSEI, filling the research gap in previous studies regarding the rationale for selecting images from periods of vigorous vegetation growth for RSEI construction, which can provide a reference for selecting the optimum season for the ecological quality monitoring of urban in the future.
1. Introduction
The status of ecological environment is inextricably linked to human survival (Yue et al. Citation2019). Since reforming and opening in 1978, urbanization has accelerated dramatically and Land use and Land cover (LULC) also changed significantly in China (Ji et al. Citation2020a), according to the National Bureau of Statistics, China’s urbanization rate increased from 17.92% to 63.89% between 1978 and 2020 (Ji et al. Citation2022), with China’s urbanization rate expected to reach 70% by 2030 (Tian et al. Citation2020). Urbanization has promote the economic, social and cultural development (Zhou et al. Citation2018), but it also has aggravated the pressure on ecological environment quality (EEQ) and led to a series of environmental issues (Airiken et al. Citation2022; Huang et al. Citation2021), such as biodiversity loss (McDonald et al. Citation2013), desertification (Zhang et al. Citation2018), grassland degradation (Wen et al. Citation2013), soil erosion (Jeong and Dorn Citation2019), hydrological fluxes and biogeochemical cycles alteration (Kalantari et al. Citation2017; Schneider et al. Citation2015) and so forth. In areas of increasing urbanization, the ecological environment has become extremely vulnerable, with a slew of ecological function degradation and eco-environmental issues (Tian et al. Citation2020; Zang et al. Citation2011). It is urgent and realistic to conduct timely, accurate, quick monitoring and quantitative assessment of the spatiotemporal changes in the eco-environment of the Beijing-Tianjin-Hebei (JJJ) region over a long time series period.
With the continuous advancement of remote sensing technology, numerous satellites with varying temporal and spatial resolutions have been launched and due to the benefits of regular, extensive and repetitive observations of the earth, remote sensing technology has grown in importance for eco-environmental monitoring (Huang et al. Citation2021; Levin et al. Citation2020; Turner et al. Citation2003). With the help of remote sensing technology, the establishment of comprehensive index model of regional ecological environment quality monitoring and evaluation has become a hotspot and focus in this field (Xu et al. Citation2023). At present, there are a plenty indicators and models for EEQ assessment based on remote sensing, including remote sensing ecological index (RSEI) (Xu Citation2013), Landscape ecological condition index(LEEI) (Chi et al. Citation2019), Soil salinization ecological index(SSEI) (Yang et al. Citation2020), Environmental vulnerability index(EVI) (Barnett et al. Citation2008), Pressure-state-response model(PSR) (Das et al. Citation2020), and Comprehensive index of land use degree(CILUD) (Li et al. Citation2019). Because RSEI couples the greenness, humidity, heat and dryness indexes in the natural environment through principal component analysis, it avoids the problem that a single index is difficult to objectively evaluate the ecological environment, and the data collection efficiency is high, no human weight is required, and the EEQ is objectively reflected, the RSEI has been widely used in the assessment of EEQ in various scenarios, such as in cities (Ji et al. Citation2020a; Maity et al. Citation2022; Zhang et al. Citation2021b), islands (Han et al. Citation2022; Liu et al. Citation2021), basins (Wu et al. Citation2020; Xiong et al. Citation2021; Zhang et al. Citation2022; Zhou and Liu Citation2022), oases(Gao et al. Citation2020), plateaus (Cao et al. Citation2022; Sun et al. Citation2020) and others (Hui et al. Citation2021; Zhu et al. Citation2020).
MODIS and Landsat data are the most used remote sensing data sources in RSEI application scenarios. However, previous research using RSEI to monitor and evaluate EEQ based on Landsat was limited to the small region level due to cloud/cloud shadow/snow contamination and the long 16-day revisit period, making it difficult to obtain all cloud-free images covering a large region at a short time (Ji et al. Citation2020a). At the regional level, Hang et al. (2020) assessed the impact of urbanization in Nanjing, Jiangsu Province using RSEI based on Landsat-5 and Landsat-8. Xiong et al. (2021) employed RSEI based on Landsat-5 and Landsat-8 to evaluate the EEQ of Erhai lake basin in Yunnan Province. Gou and Zhao (2020) monitored the EEQ of Beijing, China by combining RSEI with Random Forest (RF) based on Landsat-8. Liu et al. (2021) utilized RSEI based on Landsat-TM/OLI/TIRS to evaluate the spatiotemporal change in EEQ of Xiamen and Kinmen islands, China.
On the other hand, MODIS data provides a cost-effective approach for monitoring EEQ at a large scale and regular intervals (Xu et al. Citation2019). Some researchers applied time series RSEI based on MODIS data to evaluate EEQ and analyze the driving factors in the JJJ urban agglomeration and China (Ji et al. Citation2022; Ji et al. Citation2020a, Citation2020b, Citation2021). Xu et al. (Citation2019) applied RSEI and change vector analysis (CVA) method to detect the eco-environment changes in Fujian province using MODIS data from 2002–2017. Yang et al. (2021) calculate the RSEI based on MODIS to reveal the changes in EEQ in the Yangtze River basin from 2001–2019. Liao and Jiang (Citation2020) evaluated the spatiotemporal changes in EEQ using RSEI based on MODIS from 2000 to 2017 in China. Additionally, Xia et al. (Citation2022) utilized MODIS data to construct RSEI and investigate the ecological changes in Central Asia. In addition, RSEI is often calculated from a single image (Gou and Zhao Citation2020; Hang et al. Citation2020; Liu et al. Citation2020; Xiong et al. Citation2021; Xu et al. Citation2018; Yuan et al. Citation2021), monthly images (Airiken et al. Citation2022; Xu et al. Citation2019; Yang et al. Citation2021) or synthesize images of vegetation growing seasonal to replace annual RSEI in the previous studies (Huang et al. Citation2021; Ji et al. Citation2022; Ji et al. Citation2020a).
However, previous published research used RSEI to monitor and evaluate EEQ with the limitation of using a single image and composing monthly or vegetation growing images to calculate RSEI to replace the annual value, which have not explained why images from the growing season or the specific period were chosen. These studies, on the other hand, focus on EEQ inter-annual variation in a specific period while ignoring the seasonal RSEI difference. Furthermore, RSEI is composed of four indicators: greenness, humidity, dryness and heat, all of which are influenced by seasonal factors such as rainfall, temperature and vegetation growth state. Unfortunately, previous studies have not thoroughly examined the changes in seasonal RSEI across different seasons over a long time series, not have their correlation between RSEI and the four indicators.
Given the issues raised above, the JJJ region was chosen as the study area to monitor and evaluate the spatiotemporal changes in EEQ in different seasons using MODIS data and the GEE platform. The goal of this study is to analysis the stability of the correlation between the four indicators and seasonal RSEI and to examine the performance of various seasonal RSEI to the JJJ ecological quality assessments to determine the optimal season for assessing the ecological quality of urban metropolitan regions. From the aspects of PC1 cumulative contribution rate of RSEI in different seasons, the correlation between temperature, humidity, heat and dryness factors and RSEI in different seasons, and the stability of correlation changes in time series, this study explored the selection of the optimal time phase suitable for RSEI construction in JJJ regions, to objectively evaluate the ecological environment quality.
2. Materials and methods
A workflow was established for monitoring and evaluating the changes of seasonal EEQ as well as comparing the difference of seasonal RSEI (). First, after removing the clouds/cloud shadow and snow/ice pixels from the images, the linear interpolation method was used to interpolate the bad observations, this approach allowed us to generate complete and reliable datasets for further analysis; Second, seasonal and annual images were synthesized to calculate the RSEI for 2001, 2006, 2011, 2016 and 2020, respectively. By examining RSEI values at different seasonals, we aimed to capture temporal patterns and changes in EEQ; third, the spatiotemporal changes of EEQ were analyzed by Local indicator of spatial association (LISA) and Moran’s index (Moran’s I). LISA provided insights into the local spatial clustering of EEQ values, highlighting areas with similar environmental equity characteristics. On the other hand, Moran’s I allowed us to assess the overall spatial autocorrelation of EEQ, indicating the presence of spatial patterns on a broader scale; Finally, we explored the correlation between different seasonal RSEI and the four indicators of RSEI. This analysis helped us explore the differences in correlation between specific indicators and RSEI across seasons and their stability over time series changes.
2.1. Study area
JJJ region is in northern China (36°05′∼42°40′N, 113°27′∼119°50′E) and covers approximately 2.18 × 104 km2 (Ji et al. Citation2020a) (). The region contains a variety of landforms. Mountains, plateaus and basins dominate the western and northern regions, while plains dominate the eastern and southern regions (Zhang et al. Citation2021a). The elevation of the region is higher in the northwest than in the southeast and the predominate land types are construction land, forest and grassland (Liang et al. Citation2022). In addition, the JJJ region was consist of the municipality directly under the central government of Beijing, Tianjin and 11 cities in Hebei Province (Zhou et al. Citation2018). The coordinated development of the JJJ region is one of the three national strategies (Li et al. Citation2022). The JJJ region has a temperate semi-humid and semi-arid continental monsoon climate with four distinct seasons and significant annual rainfall variations (Deng et al. Citation2021).
2.2. Data and preprocessing
In this study, we employed MOD09A1 and MOD11A2 to calculate the four indicators that synthesize the RSEI. The data were pre-processed on GEE, including corrections for atmospheric conditions such as Rayleigh scattering (Vermote et al. Citation2011). The MOD09A1 product provides an estimate of the surface spectral reflectance of Terra MODIS bands 1–7 at 500 m resolution (Vermote et al. Citation2011). The MOD11A2 product provides an average 8 days land surface temperature (LST) at 1000 m resolution (Wan et al. Citation2015) (). The MOD11A2 data is resampled to 500 m to unify the spatial resolution of the MOD11A2 and MOD09A1(Yang et al. Citation2022). The images of 2001, 2006, 2011, 2016 and 2020 were synthesized based on GEE according to season (Spring: 1 Mar to 31 May; Summer: 1 Jun to 31 Aug; Autumn: 1 Sep to 30 Nov; Winter: 1 Dec to 28 Feb of the following year) and the annual from Jan to Dec.
Table 1. Datasets catalog introduction.
2.3 Assessment of image quality and interpolation
The quality of all MOD09A1 pixels was evaluated. According to the F-mask, the bad observations of clouds/cloud shadow and snow/ice were classified as NODATA (Wang et al. Citation2017; Zhu and Woodcock Citation2012). Since the RSEI was constructed on the GEE platform by applying a median function synthesis to seasonal images, it was necessary to ensure that each composite image had at least one good observation pixel. The proportion of bad observations was counted in 13 cities in different seasons of 2001, 2006, 2011, 2016 and 2020, respectively (). The analysis revealed that worst observations were concentrated in winter, particularly in Chengde and Zhangjiakou. In 2016 and 2020, the number of pixels that had good observations was zero accounts for more than 16% and 20% in the winter season in Zhangjiakou respectively. To fill the bad observations, a linear interpolation method was employed for each time series based on GEE (Stöckli et al. Citation2005; Wang et al. Citation2010). The rationale behind this approach is that, in a reasonable short time frame, the values of surface reflectance tend to change linearly. This property of local linearity enables one to derive the missing surface reflectance values in an image by linearly interpolating the values of another one or two images on other dates (referred to as reference images), where the surface reflectance of the same region are not missing. If there are more than one reference images available temporarily close to the missing image within a short time frame, one can fit a linear regression model to obtain a more robust estimation of the missing value in the missing image (Luo et al. Citation2018).
2.4. Construction of remote sensing ecological indices
2.4.1. Four indicators of RSEI
RSEI was proposed by Xu and it can quickly monitor and evaluate ecological conditions solely based on remotely sensed data (Xu Citation2013; Xu et al. Citation2018; Xu et al. Citation2019). The four most important indicators for the human intuitive perception of the excellent or poor quality of ecological conditions were integrated using Principal Component Analysis (PCA) (Xu Citation2013). Greenness, moisture, heat and dryness are the four indicators, which represent vegetation, soil moisture, temperature and built lands/bare areas, respectively (Hu and Xu Citation2018; Xu et al. Citation2019).
Greenness denoted with NDVI can be expressed as (Rousel et al. Citation1973);Wet as a component derived from Tasseled Cap Transformation (TCT) represents the moisture component of RSEI. The wet component based on MOD09A1 can be calculated as (Lobser and Cohen Citation2007); NDBSI consists of the index-based built-up index (IBI) and the soil index (SI), with the NDBSI representing built-up lands and bare areas according to Hu and Xu (2018). Heat is represented by the LST of MOD11A2 in this study. LST is an important indicator used to investigate ecological processes and climate change (Liao et al. Citation2022; Xu et al. Citation2019). RSEI and the four indicators can be expressed according to .
Table 2. Expressions for RSEI and the four indicators.
2.4.2. Integration of the four indicators
PCA was selected to integrate the four indicators because it is a multi-dimensional data compression technique that chooses a few important variables through an orthogonal linear transformation of multiple variables. The advantage of PCA is that the weight of each indicators is determined automatically and objectively based on the character of the data and the contribution rate of each index to each principal component (Xu Citation2013). The contribution of each indicator to RSEI is weighted by its loading to PC1 (Xu et al. Citation2018). RSEI can be expressed as:
(1)
(1)
On the one hand, indicators need to be normalized to [0, 1] before PCA due to the dimensions of the four indicators is different. On the other hand, the RSEI calculated from EquationEquation (1)(1)
(1) has low values for representing excellent ecological conditions and high values for negative ones (Xu et al. Citation2019). If the high RSEI values to represent ecologically poor and low values represent good ones, the RSEI would be subtract from one to let higher values represent better ecological status as usually expected (Xu et al. Citation2019).
(2)
(2)
To facilitate the measurement and comparison of indicators, also needs to be normalized to [0, 1] (Xu Citation2013):
(3)
(3)
The RSEI calculated by EquationEquation (3)(3)
(3) is the final RSEI in this study and the lower the RSEI value is, the poor the ecological condition is, while the higher the value represent the better (Xu Citation2013). The RSEI is divided into 5 grades at equal intervals, representing poor, fair and moderate, good and excellent ecological environment, respectively (Wang et al. Citation2016; Xu et al. Citation2019).
2.4.3. Detection of ecological condition changes in the same season of different years
To reveal the dynamic change of EEQ in the same season of different years, the difference in RSEI levels was calculated in 5 periods (2001 to 2006, 2006 to 2011, 2011 to 2016, 2016 to 2021 and 2001 to 2020). For each pixel in the study area, we defined the score from 1 to 5 corresponding to the five ecological condition levels from poor to excellent, respectively. The score interval in EEQ between periods ranged from −5 to +5. When the score is positive (1, 2, 3, 4, 5), it indicates that the EEQ has improved; when the score is 0 represented there has no change, and while the score is negative (-1, −2, −3, −4, −5) represented the EEQ has degraded. In addition, a lower score indicates a more serious ecological degradation, whereas a higher score indicates a better ecological environment.
2.4.4. Spatial autocorrelation analysis
Moran’s I and LISA are often used to analyze the spatial autocorrelation of EEQ (Jing et al. Citation2020; Xiong et al. Citation2021). Moran’s I reflect the correlation between the neighboring units (the pixels of 500 m × 500m) of geospatial space, and a value closer to 1, the stronger the correlation between units. Therefore, we utilized Moran’s I to verify the correlation between the RSEI units in this study, which can be expressed as (Gong et al. Citation2014):
(4)
(4)
(5)
(5)
where n is the grids in this study area;
=1, 2, 3 …, n;
= 1, 2, 3 …, m.
is the RSEI value of the location of
represent the average RSEI values of all units in this study area;
is the spatial units variance;
is the weight matrix which can represent the relationship of spatial units. Moran’s I values range from −1 to 1, with a value close to 1 indicating that the RSEI of spatially adjacent units is positively correlated and a value close to −1 indicating that it is negatively correlated. LISA index as an important index to analyze local spatial autocorrelation due to it can calculate the value of Moran’s I at each spatial unit. Therefore it is to Anselin (Citation1995). In this study, LISA was used to analyze the correlation of EEQ in each unit (pixels unit), the equation as follows (Gong et al. Citation2014; Xiong et al. Citation2021):
(6)
(6)
Where positive I indicates that adjacent space units have similar values (both high or both low), whereas negative I indicates that adjacent units have large value differences.
2.4.5. Pearson’s correlation analysis
For the terrestrial ecosystem, its EEQ may be determined by the four indicators of RSEI (Yuan et al. Citation2021), but the effects of these indicators on seasonal RSEI are unclear. To analyze which indicator influences EEQ in different seasons in this study area, the Pearson correlation analysis method was conducted for seasonal RSEI and the four indicators. The function can be calculated as (Ahlgren et al. Citation2003):
(7)
(7)
Where denotes Pearson’s correlation coefficient. When
is close to 0, the two variables are not correlated; when
is close to −1 or 1, a strong correlation between the two variables.; N is the number of spatial units;
and
denote the values of variables and RSEI of its units respectively;
denotes the mean of the variables;
represents the mean value of RSEI.
3. Results
3.1. Spatiotemporal changes of seasonal EE Q
RSEIs of JJJ of the study years were computed by scoring the PC1 of the four indicators based on their contributions. is the PCA analysis results. It shows that PC1 has the largest eigenvalue among the four PCs in the study years, with a proportion ranging from 71% to 80%, indicating that PC1 gathers most variability information of the four metrics. It is found that the four metrics in PC1 are grouped into two categories based on their signs, NDVI and Wet in one category, LST and IBI in the other. The opposite signs of the two categories suggest that their contributions to ecological status are in opposite ways (Xu et al. Citation2019). Most the four indicators’ characteristic information is concentrated on PC1. However, the contribution rate varies by season, with summer having a significantly higher eigenvalues contribution rate than the other three seasons. This may be explained by Xu (2013), who states that vegetation greenness is a key factor for RSEI (Xu and Deng Citation2022), therefore the eigenvalues contribution rate of PC1 of vegetation growing season is higher.
Table 3. PC1 Of four indicators in different seasons.
The variation of the seasonal RSEI mean values was depicted in . The graph showed that RSEI showed different trends in different seasons under the long time series. Therefore, different seasons to construct RSEI will lead to significant differences in the assessment of ecological quality. The RSEI in summer are 0.423, 0.480, 0.505, 0.481 and 0.509, respectively, showing a trend of rising, declining and rising. With the implementation of tree planting and afforestation project in northern China and the South-North Water Diversion project (Qi et al. Citation2018), the vegetation coverage in the Beijing-Tianjin-Hebei region has been increasing, and the ecological environment has continued to improve (Xu et al. Citation2021).
In addition, the spatial distribution of RSEI based on seasonal images also varies greatly (). Except for the southern part of the study area, the ecological quality was poor in other regions in spring () and the ecological quality was better in northeast, but worse in northwest and south in summer (). In autumn, the northwest of JJJ region showed poor ecological quality compared to other regions (). During winter, there was significant inter-annual variation. In the first three periods (), the south of the JJJ region had better ecological conditionals, while in the following two periods, it worsened (, x). However, the spatial distribution of RSEI throughout the year is consistent with that in summer ().
3.2. Dynamic change analysis of EEQ
The spatiotemporal change of EEQ in the same season of different years and scores are shown in and listed in . In fact, the scores are mainly concentrated on −1, 0 and 1, and the trend shows an inverted V-shape. In addition, compared with the pixels with the changes, the pixels with no change of EEQ accounted for the largest proportion in spring, summer and autumn. Except in winter, the proportion of pixels with scores of −4, −3, +3 or +4 respectively does not exceed 8%. It is proved that the variation of EEQ in the same season of different years is not very drastic. However, within the same time range (5-year interval), the degree of improvement or degradation of RSEI varies significantly among different seasons. For example, in the spring of 2001–2006, +1 and −1 pixels accounted for 19.69% and 10.11% respectively, with a difference of 9.58%. In winter, there was a 32.34% difference in RSEI scores between +1 and −1 grades. For the whole study period (2001–2020), the scores of 1 and −1 mean that the pixels changed slightly in five seasons: spring: 17.62%, 17.58%, summer: 32.25%, 12.34%, autumn: 24.32%, 20.77%, winter: 13.26%, 11.78% and the whole year: 22.30%,21.10, the differences were 0.04%, 19.91%, 3.55%, 1.44%, 1.2% (). Therefore, it is very important to define the optimal season to construct RSEI for the objective evaluation of ecological quality.
Table 4. RSEI levels change in the same seasons from 2001 to 2020.
From the perspective of spatiotemporal changes of EEQ, in the south of the study area was first degraded from 2001 to 2006, then improved from 2006 to 2011, and began to decline from 2011 to 2016, then increase again from 2016 to 2020 which spring, summer and annual show such a changing trend (). Zhang et al. (Citation2021b) also demonstrated that only summer RSEI showed a similar trend throughout the year. However, in the winter of 2011–2006 () and 2011–2020 (), pixels with higher scores appeared in the north, which may due to the interpolation.
According to the findings in , notable enhancements were observed in the northwestern sector, particularly in Zhangjiakou city. This noteworthy progress can be attributed to the series of government policies that were implemented, including initiatives such as the 'Returning Farmland to Forest (Grass) Project,' the 'Three-North Shelter Forest Program,' and the 'Beijing-Hebei Ecological Water Resources Protection Forest Project,' among others. The degraded area was mainly distributed in the eastern Hebei plain, where there had intensive anthropogenic activities (Ji et al. Citation2020a).
3.3. Spatial autocorrelation analysis of EEQ
To explore whether the spatial adjacent of RSEI has a certain correlation, we plotted the Moran’ I scatter plots for summer in 2001, 2006, 2011, 2016 and 2020 shown in . The first and third quadrants are where the scatter points are most concentrated, and the Moran’ I values are 0.909, 0.898, 0.917, 0.921 and 0.892, indicating that the spatial correlation of EEQ is strong positive. In other words, the spatial distribution of EEQ showed a characteristic of clustering.
The spatial clustering is shown in in which the H-H clustering area in the northeast like Chengde, while L-L clustering is in the northwest in these years. From 2001 to 2016, the L-L clustering continuously increased in the south of this study area like Shijiazhuang, Xingtai and Handan. Besides, the L-L clustering area gradually expanded in Cangzhou and Langfang from 2001 to 2020. The distribution of Not insignificant regions was scattered and the L-H and H-L regions were almost absent.
4. Discussion
4.1. Correlation between RSEI and four indicators
We synthesized seasonal images to construct RSEI to evaluate EEQ in the JJJ region in this study, which not only considered the interannual variation of EEQ in the same season, but also explored the stability of EEQ in the same season under long-term time series.
The results revealed that the characteristics of the four indicators were primarily concentrated on the PC1, particularly in the summer. Besides, the greenness (NDVI) and wetness (WET) indicators of PC1 had positive effects on EEQ, while heat (LST) and dryness (NDBSI) indicators were negative, respectively (). The proved results were similar to the previous researches (Huang et al. Citation2021; Ji et al. Citation2022; Yuan et al. Citation2021).
However, the Pearson correlation coefficient between seasonal RSEI and the four indicators showed a great difference in different periods (). Seasonal RSEI was positively correlated with NDVI and WET, but negatively with NDBSI. Meanwhile, the correlation between LST and RSEI was sometimes positive or negative. Furthermore, previous studies on RSEI time series have typically used a specific period to assess the changing characteristics of RSEI. Instance, some researchers chose the image of the growing season (July to September, 1 June to 31 October and May to October) to construct RSEI (Cao et al. Citation2022; Ji et al. Citation2020b; Jian et al. Citation2022). But there is no explanation for chose the images of the above period. Fortunately, the correlation signs between the four indicators and summer RSEI showed consistency in different years (NDVI, WET are positive and LST, NDBSI are negative). All the correlation coefficients of four indicators in summer showed strong correlation (abs: 0.6–0.8) to high correlation (abs: 0.8–1), while the LST of the other three seasons and the whole year showed moderate correlation (abs:0.4–0.6) to weak correlation (abs: <0.4) in some years (). This can be explained by that vegetation greenness is a key factor for RSEI (Xu and Deng Citation2022) and vegetation grows the most flourish and greenest in summer, while the leaves turn yellow in autumn and fall off in spring winter. In addition, to explore the correlation between the four indicators and RSEI in different seasons, we plotted the standard deviation and the mean of the correlation coefficients in different seasons from 2001 to 2020 (). The results showed that the standard deviation of the correlation between the four indicators and RSEI fluctuated slightly in summer, which the standerror of mean of NDVI, WET, LST and, NDBSI were 0.947 ± 0.005, 0.669 ± 0.052, −and, ± 0.026 and −0.944 ± 0.017, respectively. However, the mean and standard deviation of the correlation between LST and RSEI in the other three seasons, including the whole year, fluctuated greatly, as follows: (spring: −0.100 ± 0.194; autumn: −0.140 ± 0.139; winter: 0.206 ± 0.827; annual: −er: 0 ± 0.182).
Figure 9. The mean and standard deviation of correlation coefficients between RSEI and the four indicators in different seasons.

Table 5. Pearson correlation analysis of seasonal RSEI with four indicators of RSEI.
4.2. Optimizing seasonal RSEI construction for sustainable urban ecological assessment
This study marks a significant departure from traditional approaches in ecological environment quality (EEQ) assessment by emphasizing the rationale behind the seasonal selection for Remote Sensing Ecological Index (RSEI) construction. The primary focus has been to address a critical knowledge gap prevalent in previous research: the lack of a clear understanding of why specific seasons, particularly the vegetation growth season, are optimal for RSEI construction. Our approach, grounded in Principal Component Analysis (PCA) and the analysis of temporal correlations between RSEI and its constituent indicators - NDVI, NDBSI, WET and LST, offers a methodical resolution to this ambiguity.
The novel application of PCA in our study highlights that the highest contribution rate to the RSEI is consistently observed during the summer season. This is a crucial finding, considering that most existing studies, such as those by Xu (Citation2013) and others focusing on RSEI applications, have primarily adopted a generic approach by selecting imagery from the vegetation growing season without a thorough understanding of the underlying reasons. Our analysis demonstrates that the summer season not only exhibits the highest PCA contribution but also maintains the most stable and significant correlation with RSEI over the studied time series. This consistency aligns with the observations made by researchers like Hu and Xu (Citation2018), who noted the sensitivity of RSEI indicators to seasonal variations.
Further, the examination of the temporal stability in the correlation between RSEI and its indicators adds a layer of robustness to our findings. In the realm of urban ecological studies, such as those conducted by Huang et al. (Citation2021), the focus has been predominantly on the spatial aspects of ecological quality. Our study extends this by integrating a temporal dimension, revealing that the summer season not only provides the most representative data for ecological assessment but also ensures consistency and reliability over time. This aspect is particularly crucial considering the rapid urbanization and ecological changes occurring in regions like the JJJ. Our findings have significant implications for urban ecological monitoring and sustainable development. By identifying the optimal season for RSEI construction, we enable more accurate and reliable assessments of EEQ, which are essential for informed decision-making in urban planning and environmental policy.
4.3. Uncertainty analysis
Although this study analyzed the stability of seasonal RSEI in a long time series and reveals the spatial-temporal variation of seasonal EEQ of the JJJ region and proved that the summer is the most suitable time to conduct RSEI, it has several limitations. Firstly, our study employed the traditional RSEI method using NDVI, WET, LST and NDBSI indicators. However, researchers have expanded this approach by incorporating additional factors such as GDP, population and aerosol optical depth to conduct the MRSEI (Nong et al. Citation2021; Zhang et al. Citation2023). Secondly, while MODIS data was chosen for RSEI due to the large-scale study area and long time series, the coarse-resolution of 500 m may not capture fine temporospatial changes in EEQ. Acquiring high-precision, multi-temporal data for EEQ analysis is time-consuming and labor-intensive (Huang et al. Citation2021). Thirdly, this study focused on the temporospatial evolution of seasonal RSEI. Despite the synthesis of 3-month images in winter, there were still worst observations in northern Zhangjiakou and Chengde City (). Although a linear interpolation method was employed to compensate these observations, abnormal results persisted due to the lack of good observations for extended periods (). Lastly, the choice of synthesis method for seasonal image synthesis in constructing RSEI can have an impact on the results. While this study used the median function, Chen et al. (Citation2021) demonstrated significant differences in images synthesized using different methods. The influence of using mean, max or min functions for seasonal image synthesis on RSEI needs further investigation. In summary, future research should address these limitations by considering alternative indicators, utilizing higher-resolution data, improving missing data handling techniques and exploring the impact of different synthesis methods on RSEI outcomes.
5. Conclusions
Since the RSEI is completely based on remote sensing, the weights of each index are objectively determined from the load values generated by the principal component transformation, and there is no artificial subjective weighting, it is proved that the model has strong robustness. The results showed that the eigenvalues contribution rate of PC1 was more than 71% in the summer, while the other seasons ranged from 40.157% to 60.707%. In addition, the fluctuation of RSEI in the summer from 2001 to 2020 was 0.428, 0.480, 0.505, 0.481 and 0.590, respectively, which indicated that the EEQ of the JJJ has improved in this period. The EEQ in the northeast of the study area was much better than that in other places in the summer. There were significant disparities in the changes of EEQ in the JJJ region across different seasons. Furthermore, the change intensities were relatively low, focusing primarily on the scores −1, 0 and 1. LULC and climate change may explain the variation of EEQ in the JJJ region. RSEI was significantly correlated with the other four indicators and was relatively stable in summer. The findings of this study suggest that the summer images should be employed as much as possible when evaluate the EEQ of JJJ regions to ensure the veracity of the calculation results of RSEI and the objectivity of ecological quality assessment.
Data availability statement
Data will be made available on request.
Disclosure statement
The authors declare that they have no known competing financial interests or personal relationships that could have appeared to influence the work reported in this article.
Additional information
Funding
References
- Ahlgren P, Jarneving B, Rousseau R. 2003. Requirements for a cocitation similarity measure, with special reference to Pearson’s correlation coefficient. J Am Soc Inf Sci. 54(6):550–560. doi: 10.1002/asi.10242.
- Airiken M, Zhang F, Chan NW, Kung HT. 2022. Assessment of spatial and temporal ecological environment quality under land use change of urban agglomeration in the North Slope of Tianshan, China. Environ Sci Pollut Res Int. 29(8):12282–12299. doi: 10.1007/s11356-021-16579-3.
- Anselin L. 1995. Local indicators of spatial association—LISA. Geog Anal. 27(2):93–115. doi: 10.1111/j.1538-4632.1995.tb00338.x.
- Barnett J, Lambert S, Fry I. 2008. The hazards of indicators: insights from the environmental vulnerability index. Annal Assoc American Geograph. 98(1):102–119. doi: 10.1080/00045600701734315.
- Cao J, Wu E, Wu S, Fan R, Xu L, Ning K, Li Y, Lu R, Xu X, Zhang J, et al. 2022. Spatiotemporal dynamics of ecological condition in Qinghai-Tibet Plateau based on remotely sensed ecological index. Remote Sens. 14(17):4234. doi: 10.3390/rs14174234.
- Chen B, Yun T, An F, Kou W, Li H, Luo H, Yang C, Wang Q, Sun R, Wu Z. 2021. Asessment of tornado disaster in rubber plantation in western Hainan using Landsat and Sentinel-2 time series images. Nat Remote Sens Bull. 25(3):816–829. (In Chinese) doi: 10.11834/jrs.20210184.
- Chi Y, Zhang Z, Gao J, Xie Z, Zhao M, Wang E. 2019. Evaluating landscape ecological sensitivity of an estuarine island based on landscape pattern across temporal and spatial scales. Ecological Indicators. 101:221–237. doi: 10.1016/j.ecolind.2019.01.012.
- Das S, Pradhan B, Shit PK, Alamri AM. 2020. Assessment of wetland ecosystem health using the pressure–state–response (PSR) model: a case study of mursidabad district of West Bengal (India). Sustainability. 12(15):5932. doi: 10.3390/su12155932.
- Deng Y, Ling Z, Sun N, Lv J. 2021. Daily estimation of soil moisture over Beijing-Tianjin-Hebei region based on general regression neural network model. J Geo-Info Sci. 23:749–761. (In Chinese)
- Gao P, Kasimu A, Zhao Y, Lin B, Chai J, Ruzi T, Zhao H. 2020. Evaluation of the temporal and spatial changes of ecological quality in the Hami Oasis based on RSEI. Sustainability. 12(18):7716. doi: 10.3390/su12187716.
- Gong J, Xie Y, Zhao C, Gao Y. 2014. Landscape ecological risk assessment and spatial and temporal differentiation of Bailong River Basin in Gansu Province. China Environ. Sci. 34:2153–2160.
- Gou R, Zhao J. 2020. Eco-environmental quality monitoring in Beijing, China, using an RSEI-based approach combined with random forest algorithms. IEEE Access. 8:196657–196666. doi: 10.1109/ACCESS.2020.3034376.
- Han N, Hu K, Yu M, Jia P, Zhang Y. 2022. Incorporating ecological constraints into the simulations of tropical urban growth boundaries: a case study of Sanya City on Hainan Island, China. Applied Sciences. 12(13):6409. doi: 10.3390/app12136409.
- Hang X, Luo X, Cao Y, Li Y. 2020. Ecological quality assessment and the impact of urbanization based on RSEI model for Nanjing, Jiangsu Province, China. Ying Yong Sheng tai xue bao = Ying Yong Sheng Tai Xue Bao. 31(1):219–229. doi: 10.13287/j.1001-9332.202001.030.
- Hu X, Xu H. 2018. A new remote sensing index for assessing the spatial heterogeneity in urban ecological quality: a case from Fuzhou City, China. Ecol Indic. 89:11–21. doi: 10.1016/j.ecolind.2018.02.006.
- Huang H, Chen W, Zhang Y, Qiao L, Du Y. 2021. Analysis of ecological quality in Lhasa Metropolitan Area during 1990–2017 based on remote sensing and Google Earth Engine platform. J Geogr Sci. 31(2):265–280. doi: 10.1007/s11442-021-1846-8.
- Hui J, Bai Z, Ye B. 2021. Eco-environment evaluation of grassland based on remote sensing ecological index: a case in Hulunbuir Area, China. JCC. 9(6):203–213. doi: 10.4236/jcc.2021.96012.
- Jeong A, Dorn RI. 2019. Soil erosion from urbanization processes in the Sonoran Desert, Arizona, USA. Land Degrad Dev. 30(2):226–238. doi: 10.1002/ldr.3207.
- Ji J, Tang Z, Zhang W, Liu W, Jin B, Xi X, Wang F, Zhang R, Guo B, Xu Z, et al. 2022. Spatiotemporal and multiscale analysis of the coupling coordination degree between economic development equality and eco-environmental quality in China from 2001 to 2020. Remote Sensing. 14(3):737. doi: 10.3390/rs14030737.
- Ji J, Wang S, Zhou Y, Liu W, Wang L. 2020a. Spatiotemporal change and landscape pattern variation of eco-environmental quality in Jing-Jin-Ji urban agglomeration from 2001 to 2015. IEEE Access. 8:125534–125548. doi: 10.1109/ACCESS.2020.3007786.
- Ji J, Wang S, Zhou Y, Liu W, Wang L. 2020b. Studying the eco-environmental quality variations of Jing-Jin-Ji urban agglomeration and its driving factors in different ecosystem service regions from 2001 to 2015. IEEE Access. 8:154940–154952. doi: 10.1109/ACCESS.2020.3018730.
- Ji J, Wang S, Zhou Y, Liu W, Wang L. 2021. Studying the coupling coordination degree between socio-economic and eco-environment of Jing-Jin-Ji urban agglomeration during 2001–2015. IOP Conf Ser: earth Environ Sci. 675:12043.
- Jian K, Wang S, Wu X, Zhang Q. 2022. Analysis of the eco-environmental quality index in the tropical rainforest National Park in China during 1990–2020.
- Jing Y, Zhang F, He Y, Kung H-t, Johnson VC, Arikena M. 2020. Assessment of spatial and temporal variation of ecological environment quality in Ebinur Lake Wetland National Nature Reserve, Xinjiang, China. Ecol Indic. 110:105874. doi: 10.1016/j.ecolind.2019.105874.
- Kalantari Z, Ferreira CSS, Walsh RPD, Ferreira AJD, Destouni G. 2017. Urbanization development under climate change: hydrological responses in a peri‐urban Mediterranean catchment. Land Degrad Dev. 28(7):2207–2221. (doi: 10.1002/ldr.2747.
- Levin N, Kyba CCM, Zhang Q, Sánchez de Miguel A, Román MO, Li X, Portnov BA, Molthan AL, Jechow A, Miller SD, et al. 2020. Remote sensing of night lights: a review and an outlook for the future. Remote Sens Environ. 237:111443. doi: 10.1016/j.rse.2019.111443.
- Li X, Wang N, Tian S. 2019. Eco-environment quality evaluation of the Yongxing Island and its surronding sea area from1989 to 2014. Marine Science Bulletin. 38:470–480. (In Chinese)
- Li Y, Chen M, Fu Y. 2022. Analysis of the changes in the Beijing-Tianjin-Hebei urban agglomeration’s spatial structure using NPP-VIIRS data. Bulletin of Surveying and Mapping. 2:50–55. (In Chinese). doi: 10.13474/j.cnki.11-2246.2022.0042.
- Liang Y, Zou B, Feng H, Liu N. 2022. Seasonal deviation correction enhanced BGIM downscaling algorithm for remote sensing AOD products. National Remote Sensing Bulletin. 26(8):1602–1613. (In Chinese) doi: 10.11834/jrs.20219351.
- Liao W, Jiang W. 2020. Evaluation of the spatiotemporal variations in the eco-environmental quality in China based on the remote sensing ecological index. In, Remote Sensing. 12(15):2462. doi: 10.3390/rs12152462.
- Liao W, Jiang W, Huang Z. 2022. Spatiotemporal variations of eco-environment in the Guangxi Beibu Gulf Economic Zone based on remote sensing ecological index and granular computing. J Geogr Sci. 32(9):1813–1830. doi: 10.1007/s11442-022-2024-3.
- Liu C, Yang M, Hou Y, Zhao Y, Xue X. 2021. Spatiotemporal evolution of island ecological quality under different urban densities: a comparative analysis of Xiamen and Kinmen Islands, southeast China. Ecol Indic. 124:107438. doi: 10.1016/j.ecolind.2021.107438.
- Liu XY, Zhang XX, He YR, Luan HJ. 2020. Monitoring and assessment of ecological change in coastal cities based on Rsei. Int Arch Photogramm Remote Sens Spatial Inf Sci. XLII-3/W10:461–470. doi: 10.5194/isprs-archives-XLII-3-W10-461-2020.
- Lobser S, Cohen W. 2007. MODIS tasselled cap: land cover characteristics expressed through transformed MODIS data. Int J Remote Sens. 28(22):5079–5101. doi: 10.1080/01431160701253303.
- Luo Y, Guan K, Peng J. 2018. STAIR: a generic and fully-automated method to fuse multiple sources of optical satellite data to generate a high-resolution, daily and cloud-/gap-free surface reflectance product. Remote Sens Environ. 214:87–99. doi: 10.1016/j.rse.2018.04.042.
- Maity S, Das S, Pattanayak JM, Bera B, Shit PK. 2022. Assessment of ecological environment quality in Kolkata urban agglomeration, India. Urban Ecosystems. 25(4): 1137–1154.
- McDonald RI, Marcotullio PJ, Güneralp B. 2013. Urbanization and global trends in biodiversity and ecosystem services. Urbanization, biodiversity and ecosystem services: challenges and opportunities (p. 31–52). Dordrecht: Springer.
- Nong L, Wang J, Yu Y. 2021. Research on ecological environment quality in Central Yunnan based on MRSEI model. J Ecol Rural Environ. 37:972–982. (in Chinese)
- Qi X, Fu Y, Wang RY, Ng CN, Dang H, He Y. 2018. Improving the sustainability of agricultural land use: an integrated framework for the conflict between food security and environmental deterioration. Appl Geogr. 90:214–223. doi: 10.1016/j.apgeog.2017.12.009.
- Rousel J, Haas R, Schell J, Deering D. 1973. Monitoring vegetation systems in the great plains with ERTS. In, Proceedings of the Third Earth Resources Technology Satellite—1 Symposium; NASA SP-351 (p. 309–317).
- Schneider A, Mertes CM, Tatem AJ, Tan B, Sulla-Menashe D, Graves SJ, Patel NN, Horton JA, Gaughan AE, Rollo JT, et al. 2015. A new urban landscape in East–Southeast Asia, 2000–2010. Environ Res Lett. 10(3):34002. doi: 10.1088/1748-9326/10/3/034002.
- Stöckli R, Vermote E, Saleous N, Simmon R, Herring D. 2005. The Blue Marble Next Generation-A true color earth dataset including seasonal dynamics from MODIS. Published by the NASA Earth Observatory.
- Sun C, Li X, Zhang W, Li X. 2020. Evolution of ecological security in the tableland region of the Chinese loess plateau using a remote-sensing-based index. Sustainability. 12(8):3489. doi: 10.3390/su12083489.
- Tian Y, Zhou D, Jiang G. 2020. Conflict or coordination? Multiscale assessment of the spatio-temporal coupling relationship between urbanization and ecosystem services: the case of the Jingjinji Region, China. Ecol Indic. 117:106543. doi: 10.1016/j.ecolind.2020.106543.
- Turner W, Spector S, Gardiner N, Fladeland M, Sterling E, Steininger M. 2003. Remote sensing for biodiversity science and conservation. Trends Ecol Evol. 18(6):306–314. doi: 10.1016/S0169-5347(03)00070-3.
- Vermote E, Kotchenova S, Ray J. 2011. MODIS surface reflectance user’s guide. MODIS Land Surface Reflectance Science Computing Facility, Version. 1, 1–40.
- Wan Z, Hook S, Hulley G. 2015. MOD11A2 MODIS/Terra land surface temperature/emissivity8-day L3 global 1 km SIN grid V006; distributed by NASA EOSDIS LP DAAC. USGS, Sioux Falls. 10(10.5067).
- Wang H, Jia G, Fu C, Feng J, Zhao T, Ma Z. 2010. Deriving maximal light use efficiency from coordinated flux measurements and satellite data for regional gross primary production modeling. Remote Sens Environ. 114(10):2248–2258. doi: 10.1016/j.rse.2010.05.001.
- Wang J, Xiao X, Qin Y, Dong J, Geissler G, Zhang G, Cejda N, Alikhani B, Doughty RB. 2017. Mapping the dynamics of eastern redcedar encroachment into grasslands during 1984–2010 through PALSAR and time series Landsat images. Remote Sens Environ. 190:233–246. doi: 10.1016/j.rse.2016.12.025.
- Wang S, Zhang X, Zhu T, Yang W, Zhao J. 2016. Assessment of ecological environment quality in the Changbai Mountain Nature Reserve based on remote sensing technology. Progress Geograph. 35:1269.
- Wen L, Dong S, Li Y, Li X, Shi J, Wang Y, Liu D, Ma Y. 2013. Effect of degradation intensity on grassland ecosystem services in the alpine region of Qinghai-Tibetan Plateau, China. PLoS One. 8(3):e58432. doi: 10.1371/journal.pone.0058432.
- Wu T, Sang S, Wang S, Yang Y, Li M. 2020. Remote sensing assessment and spatiotemporal variations analysis of ecological carrying capacity in the Aral Sea Basin. Sci Total Environ. 735:139562. doi: 10.1016/j.scitotenv.2020.139562.
- Xia Q, Chen Y, Zhang X, Ding J. 2022. Spatiotemporal changes in ecological quality and its associated driving factors in Central Asia. Remote Sens. 14(14):3500. doi: 10.3390/rs14143500.
- Xiong Y, Xu W, Lu N, Huang S, Wu C, Wang L, Dai F, Kou W. 2021. Assessment of spatial–temporal changes of ecological environment quality based on RSEI and GEE: A case study in Erhai Lake Basin, Yunnan province, China. Ecol Indic. 125:107518. doi: 10.1016/j.ecolind.2021.107518.
- Xu H. 2013. A remote sensing index for assessment of regional ecological changes. China Environ Sci. 33:889–897.
- Xu H, Deng W. 2022. Rationality analysis of MRSEI and its difference with RSEI. Remote Sens Technol Appl. 37:1–7.
- Xu H, Wang M, Shi T, Guan H, Fang C, Lin Z. 2018. Prediction of ecological effects of potential population and impervious surface increases using a remote sensing based ecological index (RSEI). Ecol Indic. 93:730–740. doi: 10.1016/j.ecolind.2018.05.055.
- Xu H, Wang Y, Guan H, Shi T, Hu X. 2019. Detecting ecological changes with a remote sensing based ecological index (RSEI) produced time series and change vector analysis. Remote Sensing. 11(20):2345. doi: 10.3390/rs11202345.
- Xu K, Chi Y, Wang J, Ge R, Wang X. 2021. Analysis of the spatial characteristics and driving forces determining ecosystem quality of the Beijing–Tianjin–Hebei region. Environ Sci Pollut Res Int. 28(10):12555–12565. doi: 10.1007/s11356-020-11146-8.
- Xu W, Xiong Y, Huang S, Wang L. 2023. Review and researh progress of the application of remote sensing in ecologial environment quaility monitoring and evaluation. Journal of Southwest Forestry University(Natural Sciences). 43:195–204. (in Chinese)
- Yang J, Zhao J, Zhu G, Wang Y, Ma X, Wang J, Guo H, Zhang Y. 2020. Soil salinization in the oasis areas of downstream inland rivers—case study: minqin oasis. Quaternary International. 537:69–78. doi: 10.1016/j.quaint.2020.01.001.
- Yang X, Meng F, Fu P, Wang Y, Liu Y. 2022. Time-frequency optimization of RSEI: A case study of Yangtze River Basin. Ecol Indic. 141:109080. doi: 10.1016/j.ecolind.2022.109080.
- Yang X, Meng F, Fu P, Zhang Y, Liu Y. 2021. Spatiotemporal change and driving factors of the Eco-Environment quality in the Yangtze River Basin from 2001 to 2019. Ecol Indic. 131:108214. doi: 10.1016/j.ecolind.2021.108214.
- Yuan B, Fu L, Zou Y, Zhang S, Chen X, Li F, Deng Z, Xie Y. 2021. Spatiotemporal change detection of ecological quality and the associated affecting factors in Dongting Lake Basin, based on RSEI. J Cleaner Prod. 302:126995. doi: 10.1016/j.jclepro.2021.126995.
- Yue H, Liu Y, Li Y, Lu Y. 2019. Eco-environmental quality assessment in China’s 35 major cities based on remote sensing ecological index. IEEE Access. 7:51295–51311. doi: 10.1109/ACCESS.2019.2911627.
- Zang S, Wu C, Liu H, Na X. 2011. Impact of urbanization on natural ecosystem service values: a comparative study. Environ Monit Assess. 179(1–4):575–588. doi: 10.1007/s10661-010-1764-1.
- Zhang C-L, Li Q, Shen Y-P, Zhou N, Wang X-S, Li J, Jia W-R. 2018. Monitoring of Aeolian desertification on the Qinghai-Tibet Plateau from the 1970s to 2015 using Landsat images. Sci Total Environ. 619–620:1648–1659. doi: 10.1016/j.scitotenv.2017.10.137.
- Zhang J, Yang L, Gong E, Yu W, Ren J, Liu M. 2023. Dynamic monitoring of eco-environment quality in Xi’ an based on GEE and adjusted RSEI. Acta Ecologica Sinica. 43(5):2114–2127. (in Chinese)
- Zhang P, Liu S, Zhao Z, Liu C, Xu L, Gao X. 2021a. Supply and demand measurement and spatio-temporal evolution of ecosystem services in Beijing-Tianjin-Hebie Region. Acta Ecologica Sinica. 41:3354–3367. (in Chinese)
- Zhang Q, Sun C, Chen Y, Chen W, Xiang Y, Li J, Liu Y. 2022. Recent oasis dynamics and ecological security in the Tarim River Basin, Central Asia. Sustainability. 14(6):3372. doi: 10.3390/su14063372.
- Zhang T, Yang R, Yang Y, Li L, Chen L. 2021b. Assessing the urban eco-environmental quality by the remote-sensing ecological index: application to Tianjin, north China. IJGI. 10(7):475. doi: 10.3390/ijgi10070475.
- Zhou D, Tian Y, Jiang G. 2018. Spatio-temporal investigation of the interactive relationship between urbanization and ecosystem services: case study of the Jingjinji urban agglomeration, China. Ecol Indic. 95:152–164. doi: 10.1016/j.ecolind.2018.07.007.
- Zhou J, Liu W. 2022. Monitoring and evaluation of eco-environment quality based on remote sensing-based ecological index (RSEI) in Taihu Lake Basin, China. Sustainability. 14(9):5642. doi: 10.3390/su14095642.
- Zhu D, Chen T, Zhen N, Niu R. 2020. Monitoring the effects of open-pit mining on the eco-environment using a moving window-based remote sensing ecological index. Environ Sci Pollut Res Int. 27(13):15716–15728. doi: 10.1007/s11356-020-08054-2.
- Zhu Z, Woodcock CE. 2012. Object-based cloud and cloud shadow detection in Landsat imagery. Remote Sens Environ. 118:83–94. doi: 10.1016/j.rse.2011.10.028.