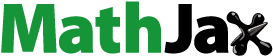
Abstract
Choosing appropriate locations for built-up growth while maintaining natural balance has become necessary for sustainable urban planning. To this end, two multi-criteria decision-making (MCDM) approaches—the multi-influencing factor (MIF) and the analytical hierarchy process (AHP)—have been applied in this work. The MIF model results show that 23.546% of the study region was very highly suitable for newly built-up areas, and 18.786% was restricted for urban settlement, whereas, in the AHP model, 28.907% of the area was highly suitable, 17.619% was restricted for urban settlement. Finally, the dependability and efficiency of the two models used for the study area were assessed through the receiver operating curve (ROC) and area under curve (AUC) analysis. The comparative analysis demonstrates that the MIF approach (AUC 0.817) is more suitable and reliable than the AHP (AUC 0.783). The present work provides new insights to integrate GIS tools with MCDM for practical urban land suitability assessment.
1. Introduction
Identifying the ideal location for future residential urban growth is essential for sustainable regional and urban land-use planning (Asadzadeh et al. Citation2014; Musakwa et al. Citation2017; Nikuze et al. Citation2022). Government and planning organizations face significant challenges in providing appropriate housing for the expanding population due to the rapid and unplanned urban expansion in recent decades, particularly in emerging nations like India (Nikolakaki Citation2004; Kilicoglu et al. Citation2020; Qiu et al. Citation2023). The land demand for future urban residential construction is growing faster because of urban population expansion. Indian urban planners are most concerned with effective town planning due to the rapid increase in the urban population (Dadhich and Hanaoka Citation2011; Chadchan and Shankar Citation2012; Pawe and Saikia Citation2018). In addition, since the Industrial Revolution, rural-to-urban migration has become more prevalent worldwide (Li et al. Citation2023). This has led to unplanned urbanization in cities with more infrastructure development (Srivastava and Chinnasamy Citation2023). Without proper planning, rapid urbanization may put significant stress on ecosystems. Unplanned urban expansion has a severe impact on the natural resources and environment and can lead to air pollution, which is a challenging issue for many emerging cities throughout the world (Ifatimehin et al. Citation2009; Dadhich and Hanaoka Citation2011; Miguez et al. Citation2015; Bose and Chowdhury Citation2020). Unplanned or inappropriate urbanization could have several detrimental effects, including the growth of industrial activities, threats to infrastructure (Chaturvedi et al. Citation2020), an increase in unmanaged waste, high non-renewable resource consumption (Kasinja and Tilley Citation2018), an increase in resource demands (such as for energy, water, and nutrients), and worsening of economic and social conditions in terms of living costs and unemployment rate (El Asmar et al. Citation2012; Nengroo et al. Citation2017).
Considering the environmental issues, sustainable urban growth is crucial for developing all emerging nations. A balance between quality of life, environmental effects, and social planning is required for effective urban planning (Hassan and Nazem Citation2016; Bose and Chowdhury Citation2020). Tracing places that adhere to particular standards or limits is aided by suitability analysis. Demarcating new locations for urban expansion or renovation has become more challenging for urban planning organizations (Singh et al. Citation2021). City geospatial transformation is a continuous and complicated cycle characterized by excessive and usually unregulated industry development, loss of natural habitat, quick and haphazard urban growth, escalating air pollution, and depletion of water and other natural resources (Moore et al. Citation2003). Urban land use drastically alters the environment, and human development activities impact the ecosystem (Peptenatu et al. Citation2011; Li and Gong Citation2016). For sustainable planning, land use for settlements must be appropriately governed. Determining the most acceptable geospatial patterns for future planning and development requires formulating management priorities and applying decision-support technologies (Martellozzo et al. Citation2018; Huang et al. Citation2023).
Site selection is an essential component of sustainable urban development and directly affects the design of a project. It is included in one of the core building planning ideas (Zucca et al. Citation2008; Mallick et al. Citation2022). Critical design decisions need to be made by engineers and architects depending on how a site interacts with its surrounding environment. The traditional site selection approach entails a team of specialists from various professions choosing the best option based on data availability and spatial characteristics (Bandira et al. Citation2021). The site choice largely determines whether a project is successful or unsuccessful. An inappropriate building site is frequently to blame for project failure, especially in developing nations. One of the crucial elements of planning is selecting a construction site (Chen et al. Citation2024). In a land suitability study, the potential of the land is divided into components according to how well it can be used for that purpose. A highly appropriate land parcel indicates a good chance of being used for a particular purpose. On the other hand, low suitability denotes that the land has a comparatively low potentiality to fulfill a specific function. The result of a land suitability study is a map showing potential future land use patterns (Zhao et al. Citation2023).
Economic and ecological considerations and various physical elements significantly impact the choice of a construction location (Kim et al. Citation2015). Conducting a land suitability study is the best and most efficient way to identify locations ideal for future urban expansion and urban heat islands direct effect for urban area (Yin et al. Citation2023; Shang et al. Citation2023). This approach uses a variety of metrics or variables that affect one’s decision to settle somewhere and are given a particular weighting (Shaikh et al. Citation2020). Models combined with remote sensing (RS), geographical information systems (GIS), and model-based spatial analysis are crucial for creating geographical decision support for urban development (Guan et al. Citation2023). The decision-maker can more effectively choose the best locations for urban development by developing a geospatial evaluation model (Kılıc et al. Citation2022).
GIS technology and multicriteria decision-making (MCDM) are often used to evaluate land appropriateness. Numerous multi-attribute (or multicriteria) evaluation processes, particularly for assessing land suitability, have been used in the RS-GIS context, including the analytical hierarchy method (AHP) and weighted linear combination with its variations (Shang and Luo Citation2021; Zhu et al. Citation2022; Shang et al. Citation2023). Using an RS-GIS-based land suitability model, the studies evaluated a systematic approach to ideal decision-making for site selection and optimal land allocation for future urban growth (Gelan Citation2021; Al Fanatseh, Citation2022; Mishra Citation2024; Shelar et al. Citation2022). AHP is regarded as the most reliable method for reducing challenges in an MCDM process by weighing the parameters with the expertise of experts (Saha and Roy Citation2021; Abdo et al. Citation2023; Wen et al. Citation2024). Urban planners are increasingly interested in using RS-GIS-based MCDM tools to address spatial planning issues (Stojčić et al. Citation2019; Rane and Jayaraj Citation2021). Additionally, studies have included GIS-based multicriteria evaluation (MCE), which compares each aspect based on its preference or relevance and creates weights of each component for choosing the optimal locations for situating facilities (Greene et al. Citation2011). Fuzzy logic and fuzzy sets are applicable across various environmental challenges. For instance, they have been utilized in tasks such as mineral exploration and predicting landslide hazards in spatial contexts (Bui et al. Citation2012). Additionally, they have been instrumental in modeling the spatial dispersion and species density of vegetation (Ibrahim et al. Citation2015; Xie et al. Citation2021), evaluating the spatial and temporal variations in salinity (Wang et al. Citation2020), time-series modeling of atmospheric pollution (Dincer and Akkuş Citation2018), forecasting soil erosion and its patterns (Saha et al. Citation2019), as well as determining land suitability for agricultural cultivation (Singha and Swain Citation2016; Zhu et al. Citation2022; Haile and Abebe Citation2022).
RS and GIS techniques are used to provide data that will be utilized for planning and research. Several studies on Indian cities have been undertaken in recent years to assess the feasibility of the land for urban development utilizing RS and GIS-based multi-criteria method with an AHP that produces more complex evaluations (Kaushik et al. Citation2020; Saha and Roy Citation2021; Mallick, Rudra, et al. Citation2024; Mallick et al. Citation2022). Singh et al. (Citation2021) recently implemented the MIF model and discovered that it is an effective and affordable way to evaluate the locations of urban settlements. By considering a particular set of elements and their assessing weights, land suitability evaluation helps determine whether a given site fits a specific application (Chandio et al. Citation2011; Hu et al. Citation2022). The study environment, the planner’s convenience, and the data availability are the foundations for identifying the criteria for spatial planning decisions through appropriateness evaluation (Karimi et al. Citation2019; Bandira et al. Citation2021). Recent studies deal with the complex interaction of physical and socioeconomic factors that demarcated acceptable city expansion sites (Saha and Roy Citation2021; Hoque and Rohatgi Citation2022; Mallick, Rudra, et al. Citation2024). These factors might be quantitative or qualitative for sustainable development (Pande Citation2020).
By reducing the statistical complexities of making decisions based on structured expert judgment, the literature shows that MIF and AHP models have attracted attention as effective models for potential site selection for cost-effective, precise, and rapid land suitability evaluation. AHP is an MCDM model that uses GIS to compare geographical attributes pairwise and give weights based on the specialist review (Saha and Roy Citation2021; Moharir et al. Citation2023). According to ratings awarded based on major and minor essential factors impacting the appropriateness of the land, the MIF is an MCDM approach that determines spatial correlations among a dependent and independent variable (Magesh et al. Citation2012; Raju et al. Citation2019; Rane et al. Citation2023). It is based on how the dependent and independent variables are related spatially. These two strategies have grown in popularity due to their relative simplicity and usefulness for real-world applications (Singh et al. Citation2021; Pande et al. Citation2023).
The current study aims to utilize and compare two established MCDM methodologies, AHP and MIF, for predicting site suitability for future urban development. This work integrates an RS-GIS-based MIF and AHP into the decision-making process to better comprehend site suitability for future urban planning and development based on suitability maps. In particular, the research attempted to investigate how several interrelated elements impact the study area’s potential for urban development. The resultant maps differ from a general site selection strategy because they articulate social, economic, and natural objectives. Policymakers, economists, and government planners will be significantly aided by the results of this study in developing effective urban settlement planning strategies. The efficacy of MIF and AHP models was assessed through a receiver operating characteristic (ROC) with the area under the curve (AUC), which considers all potential decision thresholds from observed data. Sensitivity analysis was also performed to see how each theme layer would impact the delineated urban settlement.
2. Methodology
2.1. Study area
The Ambarnath Tehsil (Study area), situated east of Greater Mumbai within the Thane District’s Municipal Council, forms an integral part of the Mumbai Metropolitan Region. Ambarnath is located in the Thane district of Maharashtra, a state in India. On the Mumbai to Pune railway route, the study area is located between Latitude 19°14′53.78″N and 19° 7′25.79″ N and Longitude 73°11′20.48″E and 73°23′4.11″ E. The research area covers about 336 sq. km. area. shows the location map of the study region with vegetation density. The research area’s highest temperature is between 26 and 32 °C, while the lowest is between 16 and 20 °C. The research region receives annual rainfall from the South West and North West monsoons that range from 2500 to 3000 mm (AMC Citation2021). Like many Mumbai suburbs, Ambarnath is separated into East Ambarnath and West Ambarnath by the railway. Hundreds of small and big chemical industries can be found in Ambarnath West, an industrial area. Ambernath is a significant outlying community of Mumbai. Due to the rapid increase in land prices or house costs combined with Mumbai’s affordability, the cities around began to acquire popularity because of their reasonable rates (Patil et al. Citation2022). As a result, the city of Ambernath is proliferating. In the research region, the population increase rate is rising continuously (Census District Census Handbook Thane Directorate of Census Operations Maharashtra Census of India Citation2011). The middle and lower classes began moving to the Ambernath. However, the abrupt population and commercial activity expansion and Ambernath’s haphazard, unplanned, and quick growth have put significant pressure on the city’s current infrastructural development (AMC Citation2021). As a result, Ambernath’s environment and natural resources are under threat. The issues of the Ambernath council are exacerbated by the pressure of industrial growth on the one hand and settlement development on the other. This issue demonstrates the necessity of identifying and defining the study area’s suitable sites for future urban development.
2.2. Determination of criteria, data gathering, and preparation
In MCDM analysis, selecting an objective or goal is the first priority as it lays the foundation stone to achieve that goal, and based on this, different criteria are selected. The selection and number of criteria lie on the researcher. They are predefined as this work’s objective is to find environmentally sustainable places for new urban development, which falls on the multi-attribute decision-making type of MCDM analysis (Türk and Çelik Citation2013). Physical, environmental, and socioeconomic considerations govern the ideal location for urban growth (Singh et al. Citation2021; Mallick, Rudra, et al. Citation2024). The factors that affect the development of the urban settlement were chosen based on the availability of field observation and geospatial data, as well as the consequences of the factors considered. In this study, a total of ten factors have been chosen, which include physical factors such as elevation and land use; socio-economic factors including the location of health services, location of industries, population density, location of railway stations, and proximity to roads; and environmental factors like the location of water bodies, groundwater recharge area, and vegetation density. shows the flowchart of the site suitability mapping for new built-up areas.
The land use map was prepared using supervised field observation in the ERDAS Imagine 2018 program. The vegetation density is prepared using the Normalized Difference Vegetation Index (NDVI) from the Landsat 8 OLI sensor data. The ArcGIS spatial analyst tool created elevation layers from the 30 m Shuttle Radar Topography Mission (SRTM) DEM data. The distances of the road network, railway, waterbodies, and industry in the study region were extracted using the toposheets obtained from the Survey of India (SOI), and their geographical distances were verified using Landsat-8 OLI imagery. The data on population density was acquired from the Census of India. In addition to the aforementioned geographical data, groundwater recharge priority data are collected from the Groundwater Survey and Development Agency (GSDA). After selecting criteria and collecting the dataset, ten criteria maps have been prepared in the GIS platform to delineate optimal sites for future urban development. Most of the maps are in vector, so these maps are transformed into a raster for the weighted overlaying operation.
2.3. Analytic hierarchy process (AHP)
Saaty (Citation1977) proposed the AHP for determining the weightage of an influencing criterion in MCDM evaluation by pairwise matrix. It is an effective method that helps decision-makers prepare priorities criteria, sub-criteria, and alternatives in decision-making and helps them find the best decision (Saaty Citation2004; Onüt and Soner Citation2008; Saha and Roy Citation2021). It is built on a hierarchical structure, allowing for more significant and visible attention to every criterion (Türk and Çelik Citation2013; Mishra et al. Citation2023; Rajesh et al. Citation2021). The following steps are employed to evaluate the weights and ranks of the influencing factors,
2.3.1. Step 1: Pairwise comparison of criteria for achieving the objectives
As stated earlier, AHP works with a pairwise matrix table; hence, Saaty proposed a numeric scale from 1 to 9 to calculate weightage. This pairwise comparison is based on the opinions of experts depending on specific questions on the factors that are more significant for decision-making, and the relative significance among two factors is quantified by the 9-point scale (Jozaghi et al. Citation2018). An example of pairwise matrix A (m*m matrix) is given below to understand the overall process and numeric value of AHP.
(1)
(1)
Where, ajk denotes how significant the jth criteria compared to the kth criteria. As long as ajk < 1, the jth criteria are less significant than the kth criteria. In contrast, if ajk > 1, the jth criteria precede the kth criteria. When two criteria are equally important, the entry for ajk will be 1, which indicates equal weight. shows the pairwise comparison matrix for weight calculation by AHP.
Table 1. Pairwise comparison matrix table for weight calculation by AHP.
2.3.2. Step 2: Normalized pairwise comparison of the criteria and weights
Normalizing each value of the pairwise matrix table and getting weightage are the next steps of AHP. Each value in the pair-wise matrix table was divided by the corresponding total column value to get the normalized value. Then, the arithmetic mean approach was applied row-wise to determine the weight of each factor. To get the weightage of the sub-class of each criterion, weighted rating and total weight have been calculated (Shaban et al. Citation2006). Weighted rating is the multiplicative value of weight calculated from a normalized pairwise table and respective ranks of each sub-class of each criterion, and the total weight of each sub-class is the percentage of the total weighted rating of all criteria. shows the normalized pairwise comparison matrix for weight calculation by AHP.
Table 2. Normalized pairwise comparison matrix table for weight calculation by AHP.
2.3.3. Step 3: Priority vector calculations
A vector of global values is calculated by multiplying
and
(2)
(2)
Where is the pairwise comparison of the options matrix and
is the criteria weight vector.
2.3.4. Step 4: Consistency ratio (CR) calculations
The CR must be computed once weights are determined to determine the degree of consistency of any expert’s judgments (Weldu and Deribew Citation2016). Saaty (Citation1977) has given an index to identify whether the comparison matrix is consistent or inconsistent. The CR values are stated as,
(3)
(3)
Where, is the consistency ratio,
is consistency index ratio, and
is the random inconsistency index of a randomly generated pairwise comparison matrix of order 1–10 computed by approximating random indices through a sample of 500 (Saaty Citation2000). In
equation,
is calculated as:
(4)
(4)
Where, is consistency vector average, n is the total number of factors. shows the random inconsistency values. The consistency ratio is set up so that if the value is less than 10%, the matrix is considered adequately consistent and acceptable; however, if it is greater than 10%, it is a symptom of inconsistent judgment.
Table 3. Random inconsistency values.
2.4. Multi influencing factor (MIF)
MIF is a practical and straightforward model that determines the weightage of different factors in MCDM analysis. There are two main parts to achieve weightage. The first part is to find out the proposed relative weight of each criterion by establishing interrelations between them; the second part is to get the final proposed weight.
2.4.1. Step 1: Identifying interrelation and calculating proposed relative weight
This stage involves creating interrelations between various criteria and weight calculation depending on the impact of the relationship among each factor and its major and minor interrelations (Singh et al. Citation2021). A weight of 1.0 and 0.5 is assigned to each major and minor effect, respectively (Nasir et al. Citation2018; Ghosh et al. Citation2020). shows the factor’s relation in the MIF model.
2.4.2. Step 2: Calculation of the final proposed weight
In this step, each factor’s cumulative weight of major and minor effects was employed to establish the relative weights of each influencing factor (Kumari et al. Citation2022; Rane and Jayaraj Citation2022). The final proposed weight has been determined by dividing the addition of each criterion’s major and minor effects by the total major and minor effects of all criteria and multiplying by 100. shows the weight calculation by the MIF model. The equation used to get this final weight is as follows,
(5)
(5)
Table 4. Weight calculation by MIF.
Where, WMajor is the major and WMinor is the minor influencing factor.
The weightage of sub-classes of each criterion has remained the same as done in AHP.
2.5. Sensitivity analysis and validation
A sensitivity analysis is conducted to comprehend the influence of each class’s assigned ranks and weights, the thematic layer, and the influential thematic layers of the acquired suitability zones. The map removal approach was used to conduct the sensitivity analysis for the MIF and AHP models (Gontara et al. Citation2016; Tomer et al. Citation2019). Using this approach, each input parameter/layer was systematically removed from the suitability map, and the variation index was used to calculate its influence on the remaining layers. The efficacy of probabilistic deterministic detection and prediction systems can be understood mathematically through the Receiver Operating Characteristic curve (ROC) curve (Manap et al. Citation2014; Tien Bui et al. Citation2019). The ROC curve is utilized to evaluate the model’s accuracy or how effectively the models can predict presence or absence (McNeil and Hanley Citation1984). The false-positive and true-positive rates were computed using the technique used by Pontius and Schneider (Citation2001), and ROC was drawn through this. The maps have been validated using 200 observed points, of which 100 points are observed for the present point and 100 for the absent category. The cumulative percentage of suitability maps called specificity and the cumulative percentage of building occurrences called sensitivity is plotted on the ‘x’ and ‘y’ axes, respectively. If that area falls under the curve (AUC) of 1, then it denotes high accuracy, and if the area under the curve is less than 0.5, then it shows low accuracy, and all the layer falls in the 0.05 significance level (Singh et al. Citation2021).
3. Results and discussion
In the last two decades, large, medium, and small cities of developing and underdeveloped countries are expanding their area rapidly. In most cases, these urban centers needed to follow the scientific way to establish themselves sustainably, making these urban centers complex and haphazard. These lead to identifying suitable places for residential plot demarcation in a sustainable way, which aims to minimize the effect on natural productive landscapes. Ten factors from different sectors were considered for this novel work. Combinations of these factors are capable of identifying suitable sites for residential development.
3.1. Details of criteria
Ten criteria that have been considered in this work are from four backgrounds: physical, environmental, socio-economical, and accessibility. The physical factor is the location of water bodies, elevation, and groundwater recharge area. Vegetation density and land use fall in the environmental category, location of health services, location of industries, and population density lie in the socio-economical, and lastly, the essential criteria, proximity to roads and location of railway stations are in the accessibility factor. shows the thematic maps prepared for each factor with their classes. shows the factors, including their classes and areas occupied.
Figure 4. Spatial variability of (a) health services, (b) land use, (c) population density, (d) water bodies, (e) railways, (f) roads, (g) industry, (h) groundwater recharge priority, (i) elevation in the study area.
Table 5. Factor their classes and areas occupied.
An urban center should always be built at a preferable distance from the flowing rivers if that area is prone to annual floods. However, many settlements tend to settle their dwellings near the water bodies if there is an acute water shortage. Ambernath taluka in Thane district has a maximum area under 57 meters of elevation and lies on the windward side of Western Ghat Mountain. It receives heavy rainfall and gets flooded most of the time during the monsoon season. Ulhas River is the main one, flowing from the middle of the study area. Some small lakes and parts of Barvi Lake fall in the study area, which are also considered. The study region was classified into five elevated zones with the highest height of 782 meters in the southwestern part of the study area. People tend to live in lower elevations for easy access. Hence, the 0–57 meters category has been assigned as rank 5, which means highest priority, and the 419–782 meters category as 1, which means lowest priority. Groundwater availability is another essential criterion that attracts people to live near it. Near water bodies, groundwater availability will be higher, and because of low-lying areas, these are the most preferable areas for groundwater recharge. So, the higher potential recharge area ranks 5, and the lowest is 1.
Development and destruction co-occur, but sustainable development always finds a proper way by which natural resources can be preserved as much as possible. In this work, the Normalized Vegetation Difference Index (NDVI), classified into five categories as an indicator of vegetation density, has been taken. The lowest density, with a range from −0.15 to 0.03, has been assigned with rank 5, which denotes the highest priority area for new developmental purposes, whereas the highest density area, which lies mainly in the higher elevated area, should preserved for the betterment of the environment and this highest density zone has been given rank 1 with least priority. In the land-use map, already built-up areas and water bodies have been ranked as 3 and 1, respectively, which denotes moderate and most minor priority zones for further development. Bare land has been chosen as the fifth highest priority, as it generally has no productive value.
Some social institutions play an essential role in choosing a land for new built-up development. Schools, colleges, and health institutions positively impact settlement in new places. Here, the location of health institutions was considered, and the least distance category was ranked at 5. In contrast, the highest distances from health centers are classified as least important, with a rank of 1. The location of industries affects human health, and for this, the farthest distance from these industries is ranked as 5 with the highest priority area, and nearer places as 1 means the most minor priority area. This area is a part of the greater Mumbai region, which carries extra population pressure. It has been seen that a lower population density area is preferable to a higher density area. For this, the lowest density area has been ranked by five and the highest by 1 ().
Table 6. Factors relative weights with normalized and total weights for each feature.
Smooth and efficient movement of the entire city is the first criterion for people who buy a new plot to reside in. Commercial buildings usually need to set up their establishments just beside the major roads, and residential establishments want to settle along minor or sub-arterial roads. But here, just beside the major road category, it has been ranked 5 with the highest importance for new buildings, and away from roads gets the decreasing value of importance. Ambernath falls in the greater Mumbai region, so people tend to move to Thane and Mumbai regularly for different purposes. This area is well connected with a suburban railway, and people want to reside near the railway stations for daily purposes. So, the location of railway stations is a determinant factor in the development of newly built-up areas. But a preferable distance from railway stations is most suitable because they are slightly calm and can be movable within ten minutes of the journey. Hence, the second category of railway station distance map has been assigned five ranks with the highest priority and the farthest distance as least priority with one rank.
3.2. AHP output
In this study, ten criteria have been utilized for the analysis. Adhering to the AHP methodology, the pairwise matrix table was constructed based on the formula (n(n−1)/2), resulting in a sum of 45 queries directed at experts. These experts were requested to provide ratings ranging from 1 to 9 by Saaty’s established scale. The best advice has been taken for further calculation. Out of 100 boxes, 45 have been filled up, according to the expert’s opinion. As usual, the diagonal value will always be one because the influences of one criterion with itself will be the same. Reciprocal values have filled up another 45 boxes.
In the normalized pairwise matrix table, all the values of the pairwise matrix table have been normalized by dividing each value by their respective column value. After that, the arithmetic mean method was applied to get the final weight. It has been found that () proximity to road has the highest rank and location of industries has the minor importance in choosing a new place to reside. According to their weight and rank, other essential criteria are the availability of groundwater, health services facility, and population density, which impact someone choosing a land for a new residential building. Priority vector has been calculated for each criterion, and λ value has been calculated, which is 11.2133. To get the consistency of the entire matrix, the consistency ratio (CR) has been calculated, which is 9.05%, and it is less than 10%, which denotes the judgment is consistent for analysis.
3.3. MIF output
Interrelations have been made graphically and numerically among these ten criteria to determine their importance. It should be mentioned that all criteria do not interrelate with each other. Some criteria have a major impact on another, and some have a minor effect. Major impact is assigned with one, whereas minor impact is given 0.5. Based on these values, the final weightage has been calculated. shows the given values of major and minor effects of different criteria.
Land use, population density, proximity to roads, and groundwater recharge priority areas get the highest value of major impact, which denotes the higher importance of these criteria for the new residential development. Land use and population density have the highest relative weight, followed by proximity to road and groundwater recharge priority. The total relative weight is 54.5, and on this total relative weight, the final proposed weight has been calculated by dividing each relative weight by the total relative weight. Land use and population density get the first rank as their proposed weight is higher, followed by proximity to the road and groundwater recharge priority. In AHP, proximity to the road gets the first rank, and groundwater recharge priority gets second, followed by the location of health services and population density. But in MIF, population density and land use get the first rank, followed by proximity to roads, groundwater recharge priority, and location of health services. Overall, the discussed criteria are decisive in new constructions.
3.4. Analysing final suitability maps
Suitable land identification for new building development is crucial for developers and policymakers. Till now, it has been demarcated by traditional ways without following norms, and these are mainly found in small and medium-class cities. Low cost and better accessibility are the prime essential factors for traditional decision-making criteria to identify lands without making holistic and systematic analysis. Ambernath Taluka falls in the greater Mumbai region, and for this, a systematic and scientific method should be followed while working on some developing activity. Ten criteria were used for the analysis, and AHP and MIF models were used to integrate GIS to determine the most suitable places. Two models are then compared at ground level for the best places.
After calculating weights by AHP and MIF, criteria maps were prepared on the GIS platform. Maximum maps were in vector format, so the overlay operation needed to be converted to raster format. After converting, these maps are integrated by weighted overlay analysis, and final maps are produced. Suitability maps in each model have been classified into five categories, from very high suitability zone to very low suitability ( and ). In the AHP model, the percentage of the very high suitability zone is 28.907, whereas in the MIF model, it shows 23.546. Very high and high category zones can be considered the best places for future development, and these areas are found just beside the already built-up area, along the extended Neral-Badlapur highway, on both sides of Ulhas River. In both models, very high and high zones are found in the northwestern part of the study area in Chatrapati Shivaji Nagar, Ajad Nagar, Mahendra Nagar, Mahatma Jyotiba Phule Nagra, Kamlakar Nagar. The main reasons behind this are that these areas are comparatively near the district headquarters in Thane, and the minimum land in this area falls in the barren land category. Besides these, the major road of this area, the Kalyan-Badlapur highway, is not too far, and also because of the nearness of Ambernath railway station. New patches of built-up area can be set up after the area of Badlapur, which will grow along the Neral-Badlapur highway and the Ulhas River. In both models, this has shown clearly that this will be the next growing and environmentally suitable area because most parts of Goregaon, Kudsavare, Dhawalpeda, Ekvira nagar, Dhavale, Vangani are still vacant land as barren, nearness of Vangani railway station and also for the flat and sandwiched land between northeastern and southern hilly part. This area initially showed linear development along the Kalyan-Badlapur highway and the railway tracks. Future development can also be seen in the extended part of this already built-up area.
Table 7. Optimum site suitability statistics through the MIF model.
Table 8. Optimum site suitability statistics through the AHP model.
From and , it is visible that the output of both the models is nearly the same for moderate suitability zones and found in the same portions of the study area in both maps. The percentages of moderate suitability zones are 20.690 and 17.117 in MIF and AHP, respectively, and these areas are demarcated in the Chargaon, Mohagar, Malegaon, Chamtoli, and Khuntavali. These selections are because small hillocks regionally dissect these areas and are far away from the main highway. These areas are slightly elevated, and road connectivity needs to be improved. Besides these, railway connectivity is absent here, and the problem of groundwater recharge facilities needs to be higher to moderate. A health service facility is a prime factor in settling on a new place, but this facility severely needs to improve these areas. Meanwhile, in the Vangani area, the condition of the health facilities is moderate, which will influence people to settle in new buildings.
3.5. Sensitivity analysis
and show the sensitivity variation index in the AHP and MIF models after each layer has been eliminated from the evaluation. After removing the vegetation density and industrial distance, the analysis revealed that the maximum variance of index mean values was 230.41 and 2.421 for the MIF and AHP models, respectively. Vegetation density, industrial distance, and elevation are the main factors influencing land suitability mapping, according to the variation index of MIF and AHP models. This demonstrates how crucial these elements are in defining urban settlement. Population density, groundwater recharge priority, and road proximity exhibited the lowest sensitivity in the MIF analysis, with variation indices of 218.09, 216.88, and 212.44, respectively. The least sensitive components in the AHP model were the importance of groundwater recharge priority, the distance to health services, and the vicinity to roads, with scores of 2.201, 2.083, and 1.658, respectively. In general, the variation index values of all the factors utilized are substantially greater, indicating the significance of all the elements for an appropriate delineation of urban suitability.
Table 9. Sensitivity variation index of the MIF model.
Table 10. Sensitivity variation index of the AHP model.
3.6. Validation through ROC
A crucial phase of every scientific investigation is validation. In this work, both models were validated using the ROC curve. False-positive and true-positive rates have been plotted along the ‘x’ and ‘y’ axes. The prediction rate is indicated by the blue line in and , and the forecasting percentage or accuracy is evaluated using an AUC scale that ranges between 0.5 and 1.0. This scale is further divided into five categories according to performance, and these are 0.5–0.6, 0.6–0.7, 0.7–0.8, 0.8–0.9, and 0.9–1.0 which are termed as poor, average, good, very good and excellent respectively (Arabameri et al. Citation2018). The results of the ROC evaluation ( and ) show that the AUC is 81.7 and 78.3% for MIF and AHP, respectively. So, it can be said that MIF delivers a very good accuracy. In contrast, AHP also showed promising results for delineating newly built-up suitable zones, which are quite accurate and acceptable methods in the study area.
4. Conclusions
GIS-based MCDM analysis is relatively easy and efficient in identifying locations for any purpose. AHP and MIF are the techniques by which one can quickly determine the weightage of different criteria. This study aims to identify suitable and sustainable locations for newly built-up areas in the Ambernath region using AHP and MIF methods. Previous works have used AHP mostly in identifying suitable built-up areas, but in this work, we have applied AHP and MIF in the same study to verify the results and efficacy of these models. For this, a total of ten criteria from different geo-environmental backgrounds were taken, and after preparing criteria maps and assigning MIF and AHP weightage, final suitability maps were produced using the GIS platform. The ranking of criteria according to their weightage are almost the same in both models. The first five criteria are proximity to the road, location of health centers, groundwater recharge priority area, population density, and land use pattern, which are the prime criteria among the ten criteria. Final suitability maps indicate that most very high and high suitability zones are near the already built-up area of the Badlapur and Ambernath region and the research area’s southeastern region along the Neral-Badlapur highway. In the validation part, it has been seen that MIF presents more accuracy than the AHP model as the percentage of AUC is 81.7 and 78.3, respectively, though there are not much higher differences between them. Overall, we can say that this study area still has comparatively abundant spaces for new development activity. Both models’ percentages of high and high suitability zones are 23.546 and 28.927 in MIF and 28.907 and 26.307 in AHP, respectively. Sensitivity analysis results show that vegetation density, industry location, and elevation are the dominant factors affecting the sustainable site selection for urban settlement in the study region. The sustainability of the environment has been taken into consideration in this work, and due to this, most of the higher suitability areas will come from barren land and some portions from agricultural land without harming the vegetation land.
Besides these, we have found some difficulties in doing this work, and these very high-resolution images of the satellite are necessary for this urban-related work. Still, we have concluded it with a medium-resolution image. AHP involves (n(n-1)/2) criteria for a pairwise matrix, and it cannot take more than ten criteria, so we have to be bound to take ten criteria for this study. However, this problem can be minimized by classifying criteria into different sub-criteria, which may further complicate the result. Besides these problems, this work will help policymakers and also local enthusiastic people to identify suitable lands quickly for their future purposes. The findings can be utilized to identify future development pillars and considerably eliminate the shortcomings of existing land suitability study methods. The key benefit of this strategy is that it can be accomplished quickly by employing data processing and GIS technology’s capabilities for land suitability analysis in making urban development decisions. As a result, the findings of this study are beneficial for the construction of future development plans that employ GIS-based land suitability analysis modeling. By demonstrating the RS-GIS-based methodology of the scientific policy-making process and designing a multicriteria system for evaluating suitability maps, this work provides new insights into integrating GIS tools and the MCDM into built-up area suitability mapping. Decision-makers can assess their influencing factor and select a policy direction for sustainable urban planning by contrasting various scenarios. As a result, the MIF offers more choice scenarios and more accurate outcomes, which help develop practical options.
Ethical approval
This article does not contain any studies with human participants or animals performed by any of the authors.
Author contributions
Nitin Liladhar Rane: Conceptualization, Idea of a topic, Formal Analysis, Data Interpretations, Methodology, Software, results and analysis, Creation and Interactive visualization of figures and methodology chart, writing – original draft, Writing – review & editing; Saurabh Purushottam Choudhary: Methodology; writing – original draft, Writing – review & editing; Arjun Saha: Investigation; Formal Analysis; Writing – review & editing; Aman Srivastava: Formal Analysis, Methodology, Creation and Interactive Visualization of Figures, Writing – review & editing; Chaitanya Baliram Pande: Conceptualization, Formal Analysis, writing – original draft, Writing – review & editing; Fahad Alshehri: Conceptualization, Formal Analysis, Validation; Okan Mert Katipoğlu and Ranjan Roy: Conceptualization, Formal Analysis and Validation; Hazem Ghassan Abdo: writing – original draft, Writing – review & editing
Acknowledgments
The authors extend their appreciation to Abdullah Alrushaid, Chair for Earth Science Remote Sensing Research, for funding. The authors are thankful to the editors and potential reviewers.
Disclosure statement
No potential conflict of interest was reported by the author(s).
Data availability statement
The data that support the findings of this study are available on request from the corresponding author.
References
- Abdo HG, Aljohani THD, Almohamad H, Al-Dughairi AA, Al-Mutiry M. 2023. Sanitary municipal landfill site selection by integration of GIS and multi-criteria techniques for environmental sustainability in Safita area, Tartous governorate, Syria. Environ Sci Pollut Res Int. 30(11):30834–30854. doi: 10.1007/s11356-022-24287-9.
- Al. Fanatseh A. 2022. Land suitability analysis of urban development in the Aqaba area, Jordan, using a GIS-based analytic hierarchy process. GeoJournal. 87(5):4143–4159.
- AMC. 2021. Ambernath city clean air action plan, city air action plan for control of air pollution in Ambernath city: one of the non-attainment city of Maharashtra. [assessed 2022 Jun 5]. https://ambarnathcouncil.net/EIPPROD/NCAP.pdf.
- Arabameri A, Pradhan B, Pourghasemi HR, Rezaei K. 2018. Identification of erosion-prone areas using different multi-criteria decision-making techniques and GIS. Geomatics Nat Hazards Risk. 9(1):1129–1155. doi: 10.1080/19475705.2018.1513084.
- Asadzadeh A, Sikder SK, Mahmoudi F, Kötter T. 2014. Assessing site selection of new towns using topsis method under entropy logic: a case study: new towns of Tehran metropolitan region (TMR). EMSD. 3(1):123. doi: 10.5296/emsd.v3i1.4874.
- Bandira PNA, Mahamud MA, Samat N, Tan ML, Chan NW. 2021. GIS-based multi-criteria evaluation for potential inland aquaculture site selection in the George Town Conurbation, Malaysia. Land. 10(11):1174. doi: 10.3390/land10111174.
- Bose A, Chowdhury IR. 2020. Monitoring and modeling of spatio-temporal urban expansion and land-use/land-cover change using Markov chain model: a case study in Siliguri Metropolitan area, West Bengal, India. Model Earth Syst Environ. 6(4):2235–2249. doi: 10.1007/s40808-020-00842-6.
- Bui DT, Pradhan B, Lofman O, Revhaug I, Dick OB. 2012. Spatial prediction of landslide hazards in Hoa Binh province (Vietnam): a comparative assessment of the efficacy of evidential belief functions and fuzzy logic models. Catena. 96:28–40. doi: 10.1016/j.catena.2012.04.001.
- Census: District Census Handbook Thane, Directorate of Census Operations Maharashtra, Census of India. 2011. [assessed 2022 Jul 3]. https://thane.nic.in/document/census-2011.
- Chadchan J, Shankar R. 2012. An analysis of urban growth trends in the post-economic reforms period in India. Int J Sustainable Built Environ. 1(1):36–49. doi: 10.1016/j.ijsbe.2012.05.001.
- Chandio IA, Matori AN, Lawal DU, Sabri S. 2011. GIS-based land suitability analysis using AHP for public parks planning in Larkana City. MAS. 5(4):177. https://. doi: 10.5539/mas.v5n4p177.
- Chaturvedi V, Kuffer M, Kohli D. 2020. Analysing urban development patterns in a conflict zone: a case study of Kabul. Remote Sensing. 12(21):3662. doi: 10.3390/rs12213662.
- Chen Z, Chen L, Zhou X, Huang L, Sandanayake M, Yap P. 2024. Recent technological advancements in BIM and LCA integration for sustainable construction: a review. Sustainability. 16(3):1340. doi: 10.3390/su16031340.
- Dadhich PN, Hanaoka S. 2011. Spatio-temporal urban growth modeling of Jaipur, India. Journal of Urban Technology. 18(3):45–65. doi: 10.1080/10630732.2011.615567.
- Dincer NG, Akkuş Ö. 2018. A new fuzzy time series model based on robust clustering for forecasting of air pollution. Ecol Inf. 43:157–164. doi: 10.1016/j.ecoinf.2017.12.001.
- El Asmar JP, Ebohon JO, Taki A. 2012. Bottom-up approach to sustainable urban development in Lebanon: the case of Zouk Mosbeh. Sustainable Cities and Society. 2(1):37–44. doi: 10.1016/j.scs.2011.10.002.
- Gelan E. 2021. GIS-based multi‐criteria analysis for sustainable urban green spaces planning in emerging towns of Ethiopia: the case of Sululta town. Environ Syst Res. 10(1):1–14. doi: 10.1186/s40068-021-00220-w.
- Ghosh D, Mandal M, Karmakar M, Banerjee M, Mandal D. 2020. Application of geospatial technology for delineating groundwater potential zones in the Gandheswari watershed, West Bengal. Sustain Water Resour Manag. 6(1):1–14. doi: 10.1007/s40899-020-00372-0.
- Gontara M, Allouche N, Jmal I, Bouri S. 2016. Sensitivity analysis for the GALDIT method based on the assessment of vulnerability to pollution in the northern Sfax coastal aquifer, Tunisia. Arab J Geosci. 9(5):1–15. doi: 10.1007/s12517-016-2437-3.
- Greene R, Devillers R, Luther JE, Eddy BG. 2011. GIS‐based multiple‐criteria decision analysis. Geography Compass. 5(6):412–432. doi: 10.1111/j.1749-8198.2011.00431.x.
- Guan H, Huang J, Li L, Li X, Miao S, Su W, Ma Y, Niu Q, Huang H. 2023. Improved Gaussian mixture model to map the flooded crops of VV and VH polarization data. Remote Sens Environ. 295:113714. doi: 10.1016/j.rse.2023.113714.
- Haile MM, Abebe AK. 2022. GIS and fuzzy logic integration in land suitability assessment for surface irrigation: the case of Guder watershed, Upper Blue Nile Basin, Ethiopia. Appl Water Sci. 12(10):240. doi: 10.1007/s13201-022-01761-w.
- Hassan MM, Nazem MNI. 2016. Examination of land use/land cover changes, urban growth dynamics, and environmental sustainability in Chittagong city, Bangladesh. Environ Dev Sustain. 18(3):697–716. doi: 10.1007/s10668-015-9672-8.
- Hoque I, Rohatgi S. 2022. Identification of Potential Urban Residential Area by Integrating AHP and WCL in RS and GIS Environment: a Case Study of Siliguri Municipal Corporation and Its Buffer of 7 km, West Bengal. J Indian Soc Remote Sens. 50(8):1437–1456. doi: 10.1007/s12524-022-01534-7.
- Hu Q, Zhao Y, Hu X, Qi J, Suo L, Pan Y, Song B, Chen X. 2022. Effect of saline land reclamation by constructing the “Raised Field-Shallow Trench” pattern on agroecosystems in Yellow River Delta. Agric Water Manag. 261:107345. doi: 10.1016/j.agwat.2021.107345.
- Huang H, Huang J, Wu Y, Zhuo W, Song J, Li X, Li L, Su W, Ma H, Liang S. 2023. The improved winter wheat yield estimation by assimilating GLASS LAI into a crop growth model with the proposed Bayesian posterior-based ensemble Kalman filter. IEEE Trans Geosci Remote Sens. 61:1–18. doi: 10.1109/TGRS.2023.3259742.
- Ibrahim L, Vogiatzakis IN, Incerti G, Feoli E. 2015. The use of fuzzy plant species density to indicate the effects of land-cover changes on biodiversity. Ecol Indic. 57:149–158. doi: 10.1016/j.ecolind.2015.04.036.
- Ifatimehin OO, Musa SD, Adeyemi JO. 2009. An analysis of the changing land use and its impact on the environment of Anyigba town, Nigeria. J Sustain Develop Africa. 10(4):357–364.
- Jozaghi A, Alizadeh B, Hatami M, Flood I, Khorrami M, Khodaei N, Ghasemi Tousi E. 2018. A comparative study of the AHP and TOPSIS techniques for dam site selection using GIS: a case study of Sistan and Baluchestan Province, Iran. Geosciences. 8(12):494. doi: 10.3390/geosciences8120494.
- Karimi H, Amiri S, Huang J, Karimi A. 2019. Integrating GIS and multi-criteria decision analysis for landfill site selection, case study: Javanrood County in Iran. Int J Environ Sci Technol. 16(11):7305–7318. doi: 10.1007/s13762-018-2151-7.
- Kasinja C, Tilley E. 2018. Formalization of informal waste pickers’ cooperatives in Blantyre, Malawi: a feasibility assessment. Sustainability. 10(4):1149. doi: 10.3390/su10041149.
- Kaushik PS, Patel J, Patel CR, Tailor RM. 2020. Assessing land suitability for managing urban growth: an application of GIS and RS. In IGARSS 2020. IEEE International Geoscience and Remote Sensing Symposium, Waikoloa, Hawaii. p. 4243–4246.
- Kılıc D, Yagci C, Iscan F. 2022. A GIS-based multi-criteria decision analysis approach using AHP for rural settlement site selection and eco-village design in Erzincan, Turkey. Socio-Econ Plan Sci. 86:101478. doi: 10.1016/j.seps.2022.101478.
- Kilicoglu C, Cetin M, Aricak B, Sevik H. 2020. Site selection by using the multi-criteria technique—a case study of Bafra, Turkey. Environ Monit Assess. 192(9):608. doi: 10.1007/s10661-020-08562-1.
- Kim JI, Senaratna DM, Ruza J, Kam C, Ng S. 2015. Feasibility study on an evidence-based decision-support system for hospital site selection for an aging population. Sustainability. 7(3):2730–2744. doi: 10.3390/su7032730.
- Kumari S, Poddar A, Kumar N, Shankar V. 2022. Delineation of groundwater recharge potential zones using the modeling based on remote sensing, GIS and MIF techniques: a study of Hamirpur District, Himachal Pradesh, India. Model Earth Syst Environ. 8(2):1759–1770. doi: 10.1007/s40808-021-01181-w.
- Li X, Gong P. 2016. Urban growth models: progress and perspective. Science Bullet. 61(21):1637–1650. doi: 10.1007/s11434-016-1111-1.
- Li R, Zhu G, Lu S, Sang L, Meng G, Chen L, Jiao Y, Wang Q. 2023. Effects of urbanization on the water cycle in the Shiyang River basin: based on a stable isotope method. Hydrol Earth Syst Sci. 27(24):4437–4452. doi: 10.5194/hess-27-4437-2023.
- Magesh NS, Chandrasekar N, Soundranayagam JP. 2012. Delineation of groundwater potential zones in Theni district, Tamil Nadu, using remote sensing, GIS and MIF techniques. Geosci Front. 3(2):189–196. doi: 10.1016/j.gsf.2011.10.007.
- Mallick J, Ibnatiq AA, Kahla NB, Alqadhi S, Singh VP, Hoa PV, Hang HT, Hong NV, Le HA. 2022. GIS-based decision support system for safe and sustainable building construction site in a mountainous region. Sustainability. 14(2):888. doi: 10.3390/su14020888.
- Mallick SK, Rudra S, Maity B. 2024. Land suitability assessment for urban built-up development of a city in the Eastern Himalayan foothills: a study towards urban sustainability. Environ Develop Sustain. 26:3767–3792. doi: 10.1007/s10668-022-02857-8.
- Manap MA, Nampak H, Pradhan B, Lee S, Sulaiman WNA, Ramli MF. 2014. Application of probabilistic-based frequency ratio model in groundwater potential mapping using remote sensing data and GIS. Arab J Geosci. 7(2):711–724. doi: 10.1007/s12517-012-0795-z.
- Martellozzo F, Amato F, Murgante B, Clarke KC. 2018. Modelling the impact of urban growth on agriculture and natural land in Italy to 2030. Appl Geogr. 91:156–167. doi: 10.1016/j.apgeog.2017.12.004.
- McNeil BJ, Hanley JA. 1984. Statistical approaches to the analysis of receiver operating characteristic (ROC) curves. Med Decis Making. 4(2):137–150. doi: 10.1177/0272989X8400400203.
- Miguez MG, Veról AP, De Sousa MM, Rezende OM. 2015. Urban floods in lowlands—levee systems, unplanned urban growth and river restoration alternative: a case study in Brazil. Sustainability. 7(8):11068–11097. doi: 10.3390/su70811068.
- Mishra AP, Khali H, Singh S, Pande CB, Singh R, Chaurasia SK. 2023. An assessment of in-situ water quality parameters and its variation with Landsat 8 level 1 surface reflectance datasets. Int J Environ Anal Chem. 103(18):1–23.
- Mishra. 2024. Assessment of water quality index using analytic hierarchy process (AHP) and GIS: a case study of a struggling Asan River. Int J Environ Anal Chem. 104(5):1–13.
- Moharir KN, Pande CB, Gautam VK, Singh SK, Rane NL. 2023. Integration of hydrogeological data, GIS and AHP techniques applied to delineate groundwater potential zones in sandstone, limestone and shales rocks of the Damoh district,(MP) central India. Environ Res. 228:115832. doi: 10.1016/j.envres.2023.115832.
- Moore M, Gould P, Keary BS. 2003. Global urbanization and impact on health. Int J Hyg Environ Health. 206(4–5):269–278. doi: 10.1078/1438-4639-00223.
- Musakwa W, Tshesane RM, Kangethe M. 2017. The strategically located land index support system for human settlements land reform in South Africa. Cities. 60:91–101. doi: 10.1016/j.cities.2016.08.007.
- Nasir MJ, Khan S, Zahid H, Khan A. 2018. Delineation of groundwater potential zones using GIS and multi influence factor (MIF) techniques: a study of district Swat, Khyber Pakhtunkhwa, Pakistan. Environ Earth Sci. 77(10):1–11. doi: 10.1007/s12665-018-7522-3.
- Nengroo ZA, Bhat MS, Kuchay NA. 2017. Measuring urban sprawl of Srinagar city, Jammu and Kashmir, India. Journal of Urban Management. 6(2):45–55. doi: 10.1016/j.jum.2017.08.001.
- Nikolakaki P. 2004. A GIS site-selection process for habitat creation: estimating connectivity of habitat patches. Landscape Urban Plann. 68(1):77–94. (03)00167-1 doi: 10.1016/S0169-2046.
- Nikuze A, Flacke J, Sliuzas R, van Maarseveen M. 2022. Urban induced-displacement of informal settlement dwellers: a comparison of affected households’ and planning officials’ preferences for resettlement site attributes in Kigali, Rwanda. Habitat Int. 119:102489. doi: 10.1016/j.habitatint.2021.102489.
- Onüt S, Soner S. 2008. Transshipment site selection using the AHP and TOPSIS approaches under fuzzy environment. Waste Manag. 28(9):1552–1559. doi: 10.1016/j.wasman.2007.05.019.
- Pande CB. 2020. Sustainable watershed development planning. In: Sustainable watershed development. SpringerBriefs in water science and technology. Cham: Springer. doi: 10.1007/978-3-030-47244-3_4.
- Pande CB, Moharir KN, Varade AM, Abdo HG, Mulla S, Yaseen ZM. 2023. Intertwined impacts of urbanization and land cover change on urban climate and agriculture in Aurangabad city (MS), India using google earth engine platform. J Clean Prod. 422:138541. doi: 10.1016/j.jclepro.2023.138541.
- Patil M, Kumar S, Pandey A, Pentayya P, Kazi F. 2022. Real-time detailed dynamic model of the Mumbai system in HYPERSIM. In 4th International Conference on Energy, Power and Environment (ICEPE), Shillong, India. p. 1–6.
- Pawe CK, Saikia A. 2018. Unplanned urban growth: land use/land cover change in the Guwahati Metropolitan Area, India. Geografisk Tidsskrift-Danish J Geogr. 118(1):88–100. doi: 10.1080/00167223.2017.1405357.
- Peptenatu D, Pintilii RD, Draghici C. 2011. Environmental risk management of urban growth poles regarding national importance. Int J Environ Sci Technol. 8(4):737–746. doi: 10.1007/BF03326258.
- Pontius RG, Schneider LC. 2001. Land-cover change model validation by an ROC method for the Ipswich watershed, Massachusetts, USA. Agric Ecosyst Environ. 85(1–3):239–248. doi: 10.1016/S0167-8809.
- Qiu S, Yang H, Zhang S, Huang S, Zhao S, Xu X, He P, Zhou W, Zhao Y, Yan N, et al. 2023. Carbon storage in an arable soil combining field measurements, aggregate turnover modeling and climate scenarios. CATENA. 220:106708. doi: 10.1016/j.catena.2022.106708.
- Rajesh J, Pande CB, Kadam SA, Gorantiwar SD, Shinde MG. 2021. Exploration of groundwater potential zones using analytical hierarchical process (AHP) approach in the Godavari river basin of Maharashtra in India. Appl Water Sci. 11(12):182. doi: 10.1007/s13201-021-01518-x.
- Raju RS, Raju GS, Rajasekhar M. 2019. Identification of groundwater potential zones in Mandavi River basin, Andhra Pradesh, India using remote sensing, GIS and MIF techniques. HydroResearch. 2:1–11. doi: 10.1016/j.hydres.2019.09.001.
- Rane N, Jayaraj GK. 2021. Stratigraphic modeling and hydraulic characterization of a typical basaltic aquifer system in the Kadva river basin, Nashik, India. Model Earth Syst Environ. 7(1):293–306. doi: 10.1007/s40808-020-01008-0.
- Rane NL, Jayaraj GK. 2022. Comparison of multi-influence factor, weight of evidence and frequency ratio techniques to evaluate groundwater potential zones of basaltic aquifer systems. Environ Dev Sustain. 24(2):2315–2344. doi: 10.1007/s10668-021-01535-5.
- Rane NL, Achari A, Choudhary SP, Mallick SK, Pande CB, Srivastava A, Moharir KN. 2023. A decision framework for potential dam site selection using GIS, MIF and TOPSIS in Ulhas river basin, India. J Cleaner Prod. 423:138890. doi: 10.1016/j.jclepro.2023.138890.
- Saaty TL. 1977. A scaling method for priorities in hierarchical structures. J Mathemat Psychol. 15(3):234–281. (77)90033-5 doi: 10.1016/0022-2496.
- Saaty TL. 2000. Fundamentals of decision making and priority theory. Pittsburgh: RWS Publications.
- Saaty TL. 2004. Decision making—the analytic hierarchy and network processes (AHP/ANP). J Syst Sci Syst Eng. 13(1):1–35. doi: 10.1007/s11518-006-0151-5.
- Saha A, Roy R. 2021. An integrated approach to identify suitable areas for built-up development using GIS-based multi-criteria analysis and AHP in Siliguri planning area, India. SN Appl Sci. 3(4):1–17. doi: 10.1007/s42452-021-04354-5.
- Saha S, Gayen A, Pourghasemi HR, Tiefenbacher JP. 2019. Identification of soil erosion-susceptible areas using fuzzy logic and analytical hierarchy process modeling in an agricultural watershed of Burdwan district, India. Environ Earth Sci. 78(23):1–18. doi: 10.1007/s12665-019-8658-5.
- Shaban A, Khawlie M, Abdallah C. 2006. Use of remote sensing and GIS to determine recharge potential zones: the case of Occidental Lebanon. Hydrogeol J. 14(4):433–443. doi: 10.1007/s10040-005-0437-6.
- Shaikh SA, Memon MA, Prokop M, Kim KS. 2020. An AHP/TOPSIS-based approach for an optimal site selection of a commercial opening utilizing geospatial data. In IEEE International Conference on Big Data and Smart Computing (BigComp), Busan, Korea. p. 295–302.
- Shang M, Luo J. 2021. The Tapio decoupling principle and key strategies for changing factors of Chinese urban carbon footprint based on cloud computing. Int J Environ Res Public Health. 18(4):2101. doi: 10.3390/ijerph18042101.
- Shang Y, Song K, Lai F, Lyu L, Liu G, Fang C, Hou J, Qiang S, Yu X, Wen Z. 2023. Remote sensing of fluorescent humification levels and its potential environmental linkages in lakes across China. Water Res. 230:119540. doi: 10.1016/j.watres.2022.119540.
- Shelar RS, Shinde SP, Pande CB, Moharir KN, Orimoloye IR, Mishra AP, Varade AM. 2022. Sub-watershed prioritization of Koyna river basin in India using multi criteria analytical hierarchical process, remote sensing and GIS techniques. Phys Chem Earth. 128:103219. doi: 10.1016/j.pce.2022.103219.
- Singh L, Saravanan S, Jennifer JJ, Abijith D. 2021. Application of multi-influence factor (MIF) technique for the identification of suitable sites for urban settlement in Tiruchirappalli City, Tamil Nadu, India. Asia-Pac J Reg Sci. 5(3):797–823. doi: 10.1007/s41685-021-00194-8.
- Singha C, Swain KC. 2016. Land suitability evaluation criteria for agricultural crop selection: a review. AR. 37(2):125–132. doi: 10.18805/ar.v37i2.10737.
- Srivastava A, Chinnasamy P. 2023. Watershed development interventions for rural water safety, security, and sustainability in semi-arid region of Western-India. Environ Dev Sustain. :1–35. doi: 10.1007/s10668-023-03387-7.
- Stojčić M, Zavadskas EK, Pamučar D, Stević Ž, Mardani A. 2019. Application of MCDM methods in sustainability engineering: a literature review 2008–2018. Symmetry. 11(3):350. doi: 10.3390/sym11030350.
- Tien Bui D, Shirzadi A, Chapi K, Shahabi H, Pradhan B, Pham B, Singh V, Chen W, Khosravi K, Bin Ahmad B, et al. 2019. A hybrid computational intelligence approach to groundwater spring potential mapping. Water. 11(10):2013. doi: 10.3390/w11102013.
- Tomer T, Katyal D, Joshi V. 2019. Sensitivity analysis of groundwater vulnerability using DRASTIC method: a case study of National Capital Territory, Delhi, India. Groundwater Sustainable Dev. 9:100271. doi: 10.1016/j.gsd.2019.100271.
- Türk E, Çelik HM. 2013. Impacts of planners’ different viewpoints on optimum land-use allocation. European Planning Studies. 21(12):1937–1957. doi: 10.1080/09654313.2012.722967.
- Wang Z, Zhang F, Zhang X, Chan NW, Kung HT, Zhou X, Wang Y. 2020. Quantitative evaluation of spatial and temporal variation of soil salinization risk using GIS-based geostatistical method. Remote Sensing. 12(15):2405. doi: 10.3390/rs12152405.
- Weldu WG, Deribew IA. 2016. Identification of potential sites for housing development using GIS-based multi-criteria evaluation in Dire Dawa city, Ethiopia. Int J Sci Basic Appl Res. 28(3):34–49.
- Wen Z, Wang Q, Ma Y, Jacinthe PA, Liu G, Li S, Shang Y, Tao H, Fang C, Lyu L, et al. 2024. Remote estimates of suspended particulate matter in global lakes using machine learning models. Int Soil Water Conserv Res. 12(1):200–216. doi: 10.1016/j.iswcr.2023.07.002.
- Xie X, Xie B, Cheng J, Chu Q, Dooling T. 2021. A simple Monte Carlo method for estimating the chance of a cyclone impact. Nat Hazards. 107(3):2573–2582. doi: 10.1007/s11069-021-04505-2.
- Yin Z, Liu Z, Liu X, Zheng W, Yin L. 2023. Urban heat islands and their effects on thermal comfort in the US: new York and New Jersey. Ecol Indic. 154:110765. doi: 10.1016/j.ecolind.2023.110765.
- Zhao R, Huang X, Xue J, Guan X. 2023. A practical simulation of carbon sink calculation for urban buildings: a case study of Zhengzhou in China. Sustain Cities Soc. 99:104980. doi: 10.1016/j.scs.2023.104980.
- Zhu G, Yong L, Zhao X, Liu Y, Zhang Z, Xu Y, Sun Z, Sang L, Wang L. 2022. Evaporation, infiltration and storage of soil water in different vegetation zones in the Qilian Mountains: a stable isotope perspective. Hydrol Earth Syst Sci. 26(14):3771–3784. doi: 10.5194/hess-26-3771-2022.
- Zucca A, Sharifi AM, Fabbri AG. 2008. Application of spatial multi-criteria analysis to site selection for a local park: a case study in the Bergamo Province, Italy. J Environ Manage. 88(4):752–769. doi: 10.1016/j.jenvman.2007.04.026.