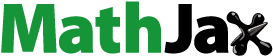
Abstract
The periodic storage and release of water in reservoirs have the potential to accelerate deformation or trigger ancient landslides. Interferometric synthetic aperture radar (InSAR) technology, renowned for its wide coverage and high precision, has emerged as an effective technique for the identification and monitoring of landslides. This study utilized spaceborne InSAR technology to identify and interpret landslides in the Liujiangxia reservoir area in China, leading to the detection of a total of 20 landslides. Moreover, among these 20 landslides, the landslide with substantial deformation (LJX02) was chosen, and the wavelet quantitative analysis technique was employed to investigate the correlation and time lag between various elevation positions of this landslide, the reservoir water level, and rainfall. The study successfully demonstrated that the deformation of the landslide lagged behind the reservoir water level by 152.10-182.50 days (from low to high elevation) and was delayed by 45.60-68.44 days compared to rainfall (from high to low elevation).
1. Introduction
The periodic fluctuations in reservoir water level, causing variations in thrust, pore water pressure, and groundwater level, modify the geological environment on both sides of the reservoir. Consequently, these fluctuations have the potential to reactivate dormant landslides or initiate the occurrence of new ones (Matsuura et al. Citation2008). Hydraulic engineering projects, both domestically and internationally, have led to numerous large-scale landslide disasters, posing significant threats to human lives and property. An exceptional incident took place at the southern slope of the Vajont Dam in Italy, resulting in a massive landslide that claimed the lives of nearly three thousand people and caused immeasurable property damage (Dykes and Bromhead Citation2018). Additionally, other cases such as the Xingguang landslide in the Xiluodu Reservoir area of China, the Qianjiangping landslide in the Three Gorges Reservoir area, and the Chana landslide in the Longyangxia Reservoir area were all triggered by the periodic fluctuation of reservoir water levels, leading to instability. These cases have drawn increased attention to the study of landslides in reservoir areas. As early as 1989, Fu et al. (Citation1989) conducted a thorough investigation of the geological structure of the left bank slope of the Liujiaxia Hydropower Station. They conducted systematic tests on rock masses and weak interlayers, providing valuable firsthand data for studying slope stability in the Liujiaxia Reservoir area. In the early twenty first century, Li et al. (Citation2007) analyzed the causes of landslides in the Longyangxia-Liujiaxia river section from the perspective of the historical evolution of the Yellow River, suggesting that the occurrence of landslides was related to intensified erosion by the Yellow River and the redistribution of water resources. However, research on slope stability in the Liujiaxia Reservoir area remained stagnant for the subsequent 15 years. It was not until 2023 that Gao et al. (Citation2023) started to assess the susceptibility of landslides in the Liujiaxia Reservoir area using InSAR technology and a Convolutional Neural Network (CNN) model. Reflecting on the Liujiaxia Hydropower Station since its establishment in 1980, the research on slope stability in the Liujiaxia Reservoir area has been inadequate. While traditional measurements and field investigations offer valuable insights into slope geological structures, the challenging terrain constrains human activities, and previous studies have not adequately addressed the significant triggering factor of periodic fluctuation of reservoir water levels in relation to landslide occurrences on both sides of the reservoir. Therefore, it is imperative to undertake research employing new technologies and methodologies to identify and monitor landslides in the Liujiaxia Reservoir area, comprehending the impacts of periodic reservoir water level fluctuations. This endeavor plays a crucial role in enabling proactive prevention and management of landslides by relevant authorities.
Water reservoir-type landslides indeed tend to occur in remote, steep mountainous canyons alongside the reservoir, making it challenging to implement traditional monitoring methods due to limited human access (Dun et al. Citation2021). However, Interferometric Synthetic Aperture Radar (InSAR) technology has emerged as a valuable tool for surface dynamic monitoring, offering several advantages such as unmanned operation, wide coverage, all-weather capability, high precision, and good temporal resolution (Gabriel et al. Citation1989). InSAR technology has proven to be particularly effective in monitoring and providing early warning for landslides over large areas and extended time periods (Dong et al. Citation2019, Kang et al. Citation2017, Sun et al. Citation2015). It not only identifies potential sliding surfaces but also aids in qualitative analysis of temporal displacement (Dai et al. Citation2020). Consequently, in the case of reservoir-area landslides, the utilization of InSAR technology for identification, monitoring, and early warning, combined with the qualitative analysis of temporal displacement along with influencing factors like rainfall and reservoir water level, has made significant progress (Dun et al. Citation2021, Liu et al. Citation2013, Zhao et al. Citation2018).
Reservoir water level and rainfall are two significant triggering factors for landslides in reservoir areas, and related research has made certain progress. Some scholars have studied the mechanisms of landslide deformation caused by reservoir water level using methods such as field investigations, physical models, and numerical simulations (Tang et al. Citation2019). For example, Jiang et al. (Citation2011) constructed a physical geological model of the Qiaotou landslide and conducted numerical simulations using a combination of field investigations, FLAC3D, and numerical modeling. They concluded that a rapid decrease in reservoir water level generates strong seepage forces, leading to significant landslide deformation. Zhou et al. (Citation2022) conducted in-depth research on the relationship between the Tangjiao landslide and Tanjiahe landslide with reservoir water level fluctuations through numerical simulations and field monitoring, proposing classification criteria for seepage-driven landslides and buoyancy-driven landslides in the Three Gorges Reservoir area. Some researchers have employed a combination of various machine learning algorithms to analyze and predict the impact of rainfall and reservoir water level on landslides in reservoir areas. For instance, Zhou et al. (Citation2016) used a coupled model of particle swarm optimization and support vector machine (PSO-SVM model) to predict the response relationship between the water level of the Three Gorges Reservoir, rainfall, and the Bazimen landslide. Wu et al. (Citation2021) combined bidirectional variance analysis and K-means clustering algorithm to analyze the relationship between reservoir water level, rainfall during different periods, and the Gapa landslide in a certain dam. Some scholars have integrated multiple technical methods, such as InSAR technology, unmanned aerial vehicle (UAV) technology, and wavelet analysis, with increasing frequency of InSAR technology applications. For example, Zhou et al. (Citation2018) combined wavelet transform and machine learning algorithms to construct a new model, finding that the impact of rapid changes in reservoir water level during the rainy season had the greatest effect on landslides on both sides of the reservoir. Yi et al. (Citation2022) employed a combination of field surveys, UAV aerial photography, and InSAR technology in a sky-ground integration approach to investigate the relationship between the water level of the Baihetan Reservoir and landslides. Tomás et al. (Citation2016) used InSAR technology and wavelet transform to demonstrate the strong correlation between loess slope landslides and reservoir water level and rainfall. All the aforementioned studies have confirmed that reservoir water level and rainfall are two important triggering factors for landslides in reservoir areas. These factors should be given due consideration in the analysis of the causes of landslides in reservoir areas.
Therefore, this study is centered on the Liujiaxia Reservoir area and employs SBAS-InSAR technology to identify landslides within the designated region. Subsequently, the obtained results are integrated with optical imagery acquired from Google Earth to facilitate the visual interpretation of landslides occurring in the reservoir area. Furthermore, the LJX02 landslide, which exhibits one of the highest deformation rates recorded between 2017 and 2021, is selected as the primary focus of investigation in this study. The quantitative analysis of the correlation and time lag between rainfall, reservoir water level, and various elevation zones of the LJX02 landslide is conducted using cross-wavelet transform and wavelet coherence analysis techniques. Additionally, this study aims to investigate the specific causal factors that contribute to these phenomena, providing reliable evidence to assist relevant departments in effectively mitigating landslides associated with fluctuations in reservoir water level and rainfall.
2. Scope of the study area
The Liujiaxia Reservoir, situated in the southwest part of central Gansu Province, China, occupies a geographical position approximately at a longitude of 103.25° E and a latitude of 35.86° N (). The reservoir is situated on the upper reaches of the Yellow River, with higher terrain found to the southwest and lower terrain to the northeast. Stretching in a southwest-northeast direction, it begins from the Liujiaxia Dam in the east and extends to the Bingling Temple Gorge in the west. Dongxiang Autonomous County and Linxia County border it to the south, while Yongjing County borders it to the north. With a storage capacity of approximately 5.7 billion cubic meters and a normal water level of 1735 meters above sea level, the Liujiaxia Reservoir plays a crucial role in the region. The highest elevation in the area is 2595 meters (). The region is characterized by a rugged landscape, featuring numerous gullies, narrow river valleys, and steep slopes, predominantly exhibiting V-shaped landforms (Gao et al. Citation2023). The exposed strata in the area comprise Tertiary claystone and sandstone, Quaternary loess, and alluvial deposits, including river terrace deposits, alongside Pre-Cambrian metamorphic rocks. The slopes primarily consist of muscovite quartzite and schist with garnet (Fu et al. Citation1989). Regarding the climate, the region experiences a temperate continental climate, with an average annual rainfall ranging from 350 to 500 millimeters. The majority of the rainfall is concentrated from May to August. Vegetation along the reservoir area is sparse, and the slopes of the reservoir are susceptible to landslides and collapses. Soil erosion is a significant concern, posing challenges to the stability of the area.
3. Materials and methods
3.1. Materials
This article selects C-band Sentinel-1A radar satellite images from the European Space Agency (ESA). These images are widely used for surface deformation monitoring due to their short revisit period (12 days) and strong temporal consistency. The image dataset includes 135 ascending orbit images from March 27, 2017, to December 31, 2021, and 130 descending orbit images from February 24, 2017, to December 12, 2021. shows the coverage area of the SAR data for both ascending and descending orbits. The imaging mode is Interferometric Wide (IW), and the polarization mode is Vertical-Vertical (VV). Detailed information about the data is provided in . Given that the study area is surrounded by high mountains, SAR images, which are acquired from a side-looking perspective, can be affected by shadows, layover, and foreshortening. To mitigate these limitations, images from different orbits are used to complement each other. Additionally, ASTER GDEM 30 m resolution DEM data downloaded from the Geospatial Data Cloud (https://www.gscloud.cn/) are employed to remove the topographic phase. Considering the impact of orbit information errors, this study utilizes the Precise Orbit Determination (POD) data provided by ESA to perform orbit information correction on the SAR data, effectively mitigating systematic errors caused by orbit information inaccuracies.
Table 1. Main parameters of SAR datasets.
To investigate the correlation between landslide deformation in the Liujiaxia Reservoir area and reservoir water level and rainfall, this study downloaded Liujiaxia Reservoir water level data from the “Yellow River Conservancy Commission” (www.yrcc.gov.cn) for the period from March 27, 2017, to December 31, 2021, and from February 24, 2017, to December 12, 2021. Furthermore, monthly rainfall data with a 1 km resolution for China (1901-2022) was obtained from the “National Tibetan Plateau Data Center” (http://data.tpdc.ac.cn) (Ding and Peng, Citation2020 Peng, Citation2020 Peng et al. Citation2019, Peng et al. Citation2017, Peng et al. Citation2018).
3.2. Methods
The purpose of this study is to monitor and analyze landslides in the Liujiaxia Reservoir area using Small Baseline Subset-Interferometric Synthetic Aperture Radar (SBAS-InSAR) technology. The process is illustrated in. It employs two methods, namely, Cross Wavelet Transform and Wavelet Coherence, to quantitatively analyze the correlation and time lag relationship between different elevation zones of the LJX02 landslide, reservoir water level, and rainfall. The process is illustrated in .
Figure 2. The technical process of SBAS-InSAR and wavelet transform. (a) Qualitative analysis of liujiaxia reservoir SBAS-InSAR. (b) Wavelet-based quantitative analysis of a landslide in liujiaxia reservoir area.

3.2.1. Small baseline subset InSAR (SBAS-InSAR)
The Small Baseline Subset InSAR (SBAS-InSAR) method was initially proposed by Berardino et al. This method enables effective monitoring of surface deformation and addresses specific limitations of traditional InSAR methods. SBAS-InSAR involves the utilization of multiple SAR images of the same study area (typically more than 6 scenes) while setting a small threshold for the temporal baseline. The method selects one or multiple master images and designates the remaining images as slave images. It identifies interferometric pairs exhibiting high coherence and removes the flat-earth phase by utilizing orbit parameters. It further eliminates the topographic phase by utilizing high-precision DEM and enhances coherence through the implementation of filtering and multilooking techniques. The wrapped interferometric phase is unwrapped, and the relationship between the phase of each coherent pixel and its corresponding observation time is determined. Deformation parameters are estimated through the utilization of singular value decomposition and least squares estimation. Atmospheric phase is estimated based on the residuals. These steps collectively yield the final deformation results (Shi et al. Citation2019). illustrates the time-position plots generated during the SBAS-InSAR interferometric processing utilizing the datasets from both the ascending and descending orbits. depicts the time-position plot of the ascending orbit dataset, whereas portrays the time-position plot of the descending orbit dataset.
3.2.2. Wavelet transform
The Wavelet Transform (WT), derived from the Fourier Transform and the Short-Time Fourier Transform (STFT), is an analysis method. It overcomes the limitation of a fixed window size in STFT by offering a variable window size that can adapt to frequency changes. As a result, the WT demonstrates multi-scale characteristics and localization capability, rendering it suitable for analyzing the periodicity and underlying information of signals at various time scales. In this study, the primary utilization revolves around the Cross Wavelet Transform and Wavelet Coherence.
3.2.2.1. Continuous wavelet transform (CWT)
The principle of Continuous Wavelet Transform (CWT) involves convolving a signal with wavelets of various scales in a sliding window fashion, which produces a matrix of wavelet coefficients. CWT is especially valuable for analyzing the information contained in a single time series because it transforms the time series into a wavelet frequency image. In this context, wavelet frequency is defined as a function of both time and the period of the time series (Tomás et al. Citation2020). The wavelet frequencies calculated through CWT can unveil the time and corresponding periodicity of high-frequency components, allowing for a more intuitive visual identification of these crucial pieces of information.
Assuming a discrete time series =0,1,…,N-1
sampled with a fixed sampling interval Δd, the Continuous Wavelet Transform (CWT) can be defined as follows (Bales Citation2022):
(1)
(1)
Where, * represents complex conjugate; represents the wavelet basis function. In practical applications, different wavelet bases should be selected based on the specific application domain and the applicable range of the wavelet basis. The Morlet wavelet is the most widely used and relatively effective wavelet transform in continuous wavelet transforms (Sloukia et al. Citation2017, Ngui et al. Citation2013);
represents the wavelet scale.
3.2.2.2. Cross-wavelet transform (XWT)
The principle of XWT involves calculating the complex two-dimensional representation by multiplying the CWT of one time series by the complex conjugate of the CWT of another time series (Tomás et al. Citation2016). The XWT not only analyzes the degree of interrelationship between signals but also provides information about the phase relationship of signals in the time-frequency domain. These phase relationships can indicate the correlation (synchronization, leading, or lagging) between two different time series (Issartel et al. Citation2014, Rateb and Kuo Citation2019). Therefore, XWT is particularly suitable for revealing the correlation and time lag between landslides and external factors such as reservoir water level and rainfall in time series.
Assume two discrete time series, and
The Cross-Wavelet Transform between them can be defined as follows:
(2)
(2)
Where, represents the CWT of the time series
in the frequency range
represents the complex conjugate of the CWT of the time series
The cross wavelet power spectrum is denoted as
(Grinsted et al. Citation2004, Wang et al. Citation2023).
3.2.2.3. Wavelet coherence (WTC)
Considering that when performing XWT, if one of the time series has strong peaks in its CWT, the absolute values of the results obtained from the two time series will also be high. This can lead to a misinterpretation of strong correlation between two time series that are not actually strongly correlated. To address this issue, we can normalize and scale the magnitudes of the time series and
By scaling the spectra of these two time series to the range of [0, 1], we ensure the accuracy of coherence between the two time series. This method is referred to as Wavelet Coherence (WTC)(Grinsted et al. Citation2004). The Wavelet Coherence can be defined as follows:
(3)
(3)
Where, represents the corresponding XWT,
denotes the smoothing factor, and
represents the Wavelet Coherence between the time series
and
This approach resolves the issue of coherence misjudgment in XWT, enabling more accurate identification of correlation and periodicity.
3.2.2.4. Wavelet phase analysis
The phase plot can unveil the correlation (lead or lag) between two time series. In the phase plot, a horizontal arrow pointing to the right signifies a phase difference of 0. A counterclockwise rotation of 90° corresponds to a phase difference of π/2, whereas a 180° counterclockwise rotation corresponds to a phase difference of π. Conversely, in the clockwise direction, the phase differences are opposite: a clockwise rotation of 90° corresponds to a phase difference of -π/2, whereas a 180° clockwise rotation corresponds to a phase difference of -π. Specifically, a phase difference of 0 indicates that the time series Kn and Mn are positively correlated in the same direction. A phase difference of π indicates that they are negatively correlated in the opposite direction. Phase differences between (0, π/2) and (-π, -π/2) indicate that time series leads
while phase differences between (π/2, π) and (-π/2, 0) indicate that time series Kn lags behind
(Su et al. Citation2017). These relationships can be represented using a Cartesian coordinate system, as depicted in .
The analysis of the phase plot obtained from wavelet transformation not only allows for an effective determination of the correlation (lead or lag) between two signals but also enables the estimation of the approximate time difference between them. The lead or lag time (Δt) between two signals can be expressed as follows (Torrence and Compo Citation1998):
(4)
(4)
Where, Δφ represents the phase difference between the two time series, which can be obtained from the phase spectrum plot. T represents the wave period of strong correlation determined through a 95% confidence level test (indicated by the highlighted section in the phase plot, represented by a thick black solid line circle).
4. Result
4.1. Landslide identification in the reservoir area
Based on the SBAS-InSAR technique, as discussed in Section 2, we were able to obtain surface deformation velocity maps of the Liujiaxia Reservoir area, which are depicted in . Indeed, it is crucial to acknowledge that errors can arise between the radar line of sight displacement and the actual landslide displacement. Various factors, including radar incidence angle, slope gradient, and orientation, can contribute to these errors. These factors can significantly impact the accuracy of obtaining precise three-dimensional surface deformation using radar side-looking imaging.(Dai et al. Citation2022). In this study, we made use of data from both ascending and descending orbits to complement each other and mitigate the limitations mentioned earlier in landslide monitoring. By combining the deformation velocity maps obtained from both orbits, we were able to observe significant subsidence areas on both the left and right banks of the Liujiaxia Reservoir. Notably, areas showing blue and purple colors indicate significant motion away from the satellite, while green color represents stable regions. This comprehensive approach allowed us to gain a more comprehensive understanding of the surface deformation patterns in the study area.
Figure 5. LOS deformation rate images of Liujiaxia Reservoir area. (a) Ascending displacement rate map of the Liujiaxia Reservoir area; (b) descending displacement rate map of the Liujiaxia Reservoir area.

However, it is not accurate to solely rely on InSAR results to identify landslides. Other non-landslide features such as construction sites, cultivated land, and collapses can also be misinterpreted as landslides. To mitigate the influence of non-landslide hazards arising from collapses and construction activities in the InSAR results, we adopted a comprehensive approach. We combined the findings from both ascending and descending orbits with optical imagery sourced from Google Earth for a more accurate interpretation of landslides. By integrating multiple data sources, we aimed to minimize the risk of misidentifying non-landslide features and enhance the reliability of our landslide assessments. This approach allowed us to gain a more holistic understanding of the study area and make more informed interpretations regarding landslide occurrences. As a result, we identified twenty landslides (named LJX01-20) as shown in . displays the InSAR deformation results for these twenty landslides. illustrates the imagery features of the aforementioned twenty unstable slopes on Google Earth. Analysis reveals that the average deformation rate range of the aforementioned 20 landslides is −55 to 23 mm/year. Both the left and right banks of the Liujiagou Reservoir area exhibit subsidence regions. The larger subsidence area on the left bank is located in Xianyuan Town, Dongfeng Village, and Majiashan (LJX03-05), with the highest annual average deformation rate reaching −55 mm/year (). On the right bank, the prominent subsidence areas are found in Bashige Village, Dayuan Village, and Kaole Township (LJX01-02, LJX18-20), with the highest annual average deformation rate reaching −42 mm/year ().
4.2. Time series analysis
The LJX02 landslide is located on the right side of Bashigenyuan Village, with a large area of villages and cultivated land on the upper part of the slope. As indicated in Section 2.1, the InSAR results for the LJX02 landslide are favorable (-LJX02), which is beneficial for temporal analysis. From the imagery in July 2018 (), it can be observed that there is a clear circle of cracks and depressions in the upper part of the slope, indicating an overall downward movement of the slope. In July 2019 (), a small-scale slippage occurred in the lower part of the slope, and cracks on the landslide bent downwards along the extension direction. As shown in , the optical imagery from February 2021 reveals a significant collapse of the slope, with an increased number of cracks observed on the slope surface.
Figure 9. Overlay of the ascending deformation of LJX02 and optical images. (a) ascending deformation rate of LJX02 and distribution of three characteristic points; (b) optical image of LJX02 on July 19, 2018; (c) optical image of LJX02 on July 4, 2019; (d) optical image of LJX02 on February 8, 2021.

To explore the relationship between the deformation of the LJX02 landslide during the period of 2017-2021 and the reservoir water level and rainfall, three characteristic points (P0, P, P1) distributed along the longitudinal profile of the slope were selected for analysis using the ascending orbit deformation time series, which showed good monitoring results for the LJX02 landslide. As shown in , P0 is located in the lower part of the slope with an average deformation velocity of −28.104 mm/year and a maximum cumulative subsidence of 125.8 mm. P is located in the middle part of the slope with an average deformation velocity of −42.55 mm/year and a maximum cumulative subsidence of 149 mm. P1 is located in the upper part of the slope with an average deformation velocity of −24.169 mm/year and a maximum cumulative subsidence of 105.2 mm.
In order to prevent flood disasters during the wet season, the Liujiaxia Reservoir maintains its lowest water level (1715 m) during the month of June to August., such as the floods that occurred in 1981 and 1998, which resulted in millions of people being displaced. Afterward, the reservoir starts to store water to its highest level (1735 m) at the end of the rainy season in September and maintains the water level for power generation and navigation from November to March of the following year. Finally, during the spring season, a gradual decrease in the water level occurs to the early lowest water level (1715 m), mainly for irrigation purposes in agriculture.
We have analyzed the monthly average rainfall and daily reservoir water level data from March 27, 2017, to December 31, 2021, to examine the relationship between the InSAR time-series deformation of the LJX02 landslide obtained in Section 2.1 and these two factors. The cumulative deformation of the three characteristic points (P0, P, P1) in relation to rainfall and reservoir water level is shown in . From the graph, it can be observed that P0 does not exhibit periodic fluctuations during periods of increased rainfall. However, it shows sudden strong fluctuations after each fluctuation in the reservoir water level. This indicates that P0 is primarily influenced by the reservoir water level. Similarly, point P shows a correlation with both rainfall and reservoir water level, while P1 is more influenced by rainfall.
Figure 10. Relationship curves of rainfall, reservoir water level and displacement of characteristic point.

However, these subjective visual results do not have significant practical reference value and lack objective persuasiveness. Additionally, it is difficult to quantitatively calculate the time lag relationship between reservoir water level changes, rainfall, and the temporal variation of the characteristic points from the graph. Therefore, in the subsequent sections, this study will employ wavelet analysis to further investigate the effects of reservoir water level and rainfall on reservoirs on the LJX02 landslide.
5. Discussion
5.1. Time lag between landslide displacement and reservoir water level and rainfall
This paper presents the application of the wavelet transform tool, derived from the field of signal processing, for landslide analysis. The wavelet transform offers valuable insights into the phase relationship of signals in the time-frequency domain. However, it is important to note that the transformation coefficients generated by the Continuous Wavelet Transform (CWT) can be challenging to interpret and comprehend, making it difficult to extract meaningful features from the results. Additionally, while the Cross Wavelet Transform (XWT) can indicate coherence, it may also lead to false positives, potentially compromising the accuracy of the analysis. To overcome these limitations, Wavelet Transform Coherence (WTC) emerges as a more stable wavelet analysis tool. WTC addresses the shortcomings of previous methods, providing enhanced stability and reliability in landslide analysis. By leveraging the advantages of WTC, researchers can obtain more robust and meaningful insights into the temporal and spatial characteristics of landslides, facilitating a more comprehensive understanding of their behavior and dynamics.(Tomás et al. Citation2016). Therefore, this paper selects XWT and WTC, which can indicate coherence, to complement each other for quantitative analysis of the LJX02 landslide.
XWT (Cross-Wavelet Transform) and WTC (Wavelet Coherence) techniques demonstrate strong signal coupling and resolution capabilities, enabling them to identify common power and relative phase information in the time-frequency domain. These techniques also allow for the assessment of significant coherence and confidence levels under the presence of red noise background. For the three characteristic points of the LJX02 landslide, the cross-wavelet transform and wavelet coherence analyses are conducted with rainfall and reservoir water level. The resulting cross-wavelet energy spectra and cross-wavelet coherence spectra between the three characteristic points and rainfall as well as reservoir water level are depicted in and , respectively.
Figure 11. Wavelet Transform result diagram of the relationship between rainfall and characteristic points of LJX02 landslide. (a) Wavelet Transform result diagram of the relationship between rainfall and point P0; (b) wavelet Transform result diagram of the relationship between rainfall and point P; (c) wavelet Transform result diagram of the relationship between rainfall and point P1.

Figure 12. Wavelet transform result diagram of the relationship between reservoir water level and characteristic points of landslide 2. (a) Wavelet transform result diagram of the relationship between reservoir water level and point P0; (b) wavelet Transform result diagram of the relationship between reservoir water level and point P; (c) wavelet Transform result diagram of the relationship between reservoir water level and point P.

The XWT analysis in reveals a high-energy region between rainfalland point P0, spanning a one-year (30 × 12 days) period. This region is distributed on both sides of the cone of influence, with arrows indicating instability. Similarly, the WTC analysis also indicates the absence of a stable high-energy region, suggesting a weak correlation between the deformation of point P0 and rainfall.
reveals the XWT and WTC analyses between rainfall and point P, indicating the highest energy density in the spectrogram over a one-year period. The WTC analysis verifies the strong correlation between rainfall and point P from 2018 to 2021, as the high-energy region passes the 95% confidence test.
The correlation results of WTC indicate that the cross-wavelet arrows between rainfall and point P align between (0, π/2), suggesting that rainfall precedes the deformation at point P. The measured clockwise angle (Δφ) between the arrow and the horizontal direction is 3/8 π. By substituting this phase difference into Equation (4), it is determined that the deformation at point P lags behind rainfall by 3/16 of a period, corresponding to a lag of approximately 68.33 days.
Likewise, demonstrates that the measured phase angle of point P1 is π/4, indicating that rainfall precedes the deformation at point P1 by 1/8 of a period. Substituting this phase angle into the equation yields a calculated lag of approximately 45.60 days between the landslide displacement and rainfall.
illustrates that the XWT and WTC analyses between reservoir water level and point P0 demonstrate the highest energy density throughout the entire time series, encompassing a one-year (30 × 12 days) period, and successfully pass the 95% confidence test. The WTC analysis reveals that the wavelet arrows between reservoir water level and point P0 align between (π/2, π), and the calculated phase angle is 5/6 π. This implies that the landslide displacement at point P0 lags behind the fluctuations in reservoir water level by 5/12 of a period. By substituting the phase angle into Equation (4), it is determined that the landslide displacement at point P0 lags behind water level fluctuations by approximately 152.10 days.
Likewise, demonstrates that the phase angle of point P in the graph is π, indicating that the landslide displacement at point P occurs after the reservoir water level fluctuations by 1/2 of a period. By substituting this phase angle into Equation (4), it is calculated that point P's landslide displacement lags behind water level fluctuations by approximately 182.50 days.
The XWT and WTC analyses in indicate a lack of significant correlation between point P1 and the reservoir water level. This implies that the deformation of point P1 is primarily influenced by factors other than the reservoir water level.
5.2. Reasons analysis of the time lag
This article used two different wavelet transform tools, Cross Wavelet Transform (XWT) and Wavelet Coherence (WTC), to study the relationship between the temporal displacement of InSAR, reservoir water level, and rainfall time series. In the case of the LJX02 landslide, three feature points P0, P, and P1 were selected to represent the toe, mid-slope, and rear edge of the landslide, respectively. By analyzing the XWT and WTC spectrograms of the reservoir water level and the InSAR temporal deformation of these three points (), the following conclusions were drawn: Firstly, the fluctuations in reservoir water level have a significant impact on the toe and mid-slope positions of the LJX02 landslide, as evidenced by strong correlations in the spectrograms. Secondly, from bottom to top, the deformation of the feature points lags behind the rise in reservoir water level by 152.10 days for point P0 (), 182.50 days for point P (), while point P1 does not exhibit significant correlation (). The specific lag times are shown in . These results indicate that the toe point P0 responds to the rise in reservoir water level with the shortest response time, being the first to be affected. The mid-slope point P responds with a lag of approximately 30.40 days, while the rear edge point P1 shows weak correlation with the reservoir water level.
Table 2. Relationship between rainfall, reservoir water level and feature points of LJX02 landslide.
The phenomena can be explained as follows: During the reservoir filling period, the water in proximity to the reservoir bank exerts a continuous hydrostatic thrust on the slope, thereby enhancing the stability of the adjacent slope. However, as the LJX02 landslide is not in direct contact with the water, the hydrostatic thrust does not significantly influence its stability. Alternatively, the hydrostatic pressure induces the upward movement of groundwater during reservoir filling, resulting in a gradual increase in moisture content and pore water pressure within the LJX02 landslide (the duration of which mainly relies on soil permeability and infiltration paths, as illustrated in ). This, in turn, reduces the effective stress in the soil and the shear strength along the sliding surface (Tomás et al. Citation2016), resulting in a strong correlation between landslide displacement and reservoir water level fluctuations in the XWT and WTC results. The toe position is in closest proximity to the groundwater, and as the elevation rises, the pathway for groundwater to reach the mid-slope and rear edge of the slope lengthens, necessitating the overcoming of gravitational forces. Consequently, in the XWT and WTC results, the toe point P0 exhibits the briefest response time to rising water levels in the reservoir, followed by the mid-slope, whereas the rear edge point P1 remains largely unaffected due to its elevated position. Once the reservoir reaches and sustains its normal water level, the stability of the LJX02 landslide increases. During the reservoir drawdown period, the hydrostatic thrust decreases, and the groundwater level decreases as well (). Similarly, influenced by the soil permeability and infiltration paths, the water level fluctuations have the fastest impact on the toe position and the lag time between displacement and water level fluctuations increases with elevation (Jiang et al. Citation2011, Tomás et al. Citation2014). In summary, variations in reservoir water level directly induce modifications in hydrostatic thrust and groundwater level, subsequently exerting an indirect influence on soil saturation and pore water pressure. These cumulative alterations contribute to the cyclic fluctuations in displacement of the LJX02 landslide, which are directly linked to water level variations. Additionally, owing to the LJX02 landslide’s nonadjacent position to the water, disparities in elevation among different areas and variations in groundwater infiltration pathways, there exists a discernible lag in the influence of reservoir inflow and outflow on the landslide (), as evidenced by the periodicity of approximately one year in the XWT and WTC results.
Figure 13. The principle diagram of time lag and the influence of reservoir water level. (a) The principle of one-year time lag; (b) the effects of hydrostatic thrust and groundwater on the LJX02 landslide during reservoir impoundment; (c) the effects of hydrostatic thrust and groundwater on the LJX02 landslide during reservoir drawdown.

Additionally, an interesting phenomenon is that the XWT and WTC spectrograms of rainfall and the three feature points of the LJX02 landslide () exhibit opposite lag relationships. Feature points P1, P, and P0 are delayed by 45.60 days (), 68.44 days (), and show no significant correlation () with rainfall, respectively. These lag times are listed in . This phenomenon can be explained as follows: Firstly, after the landslide occurs, there is usually a large-scale loosening, fragmentation, and cracking of the soil mass at the rear edge of the landslide (). The permeability and drainage capacity of the soil decrease, and when rainfall occurs, water infiltrates the soil and fills these voids. This means that rainwater has a harder time percolating and draining from the soil, leading to increased soil saturation and reduced shear strength, thus enhancing the landslide’s mobility (Jiang et al. Citation2011). Secondly, due to the downward infiltration of rainwater, the rear edge of the LJX02 landslide (referred to as P1) is most severely affected, with higher moisture content. Subsequently, the rainwater permeates into the middle part of the slope (referred to as P) and may eventually reach the slope toe (referred to as P0), or it may have been absorbed and evaporated by the upper part of the slope. Therefore, there is no apparent correlation observed between point P0 and rainfall in the wavelet transformation. Thirdly, when rainwater passes through the rear edge of the landslide (P1), it erodes and corrodes the surface of the soil, further weakening the stability of the soil. The middle part of the landslide (P) is less affected by direct erosion from rainwater, while the slope toe (P0) is even less affected.
Therefore, based on the above analysis, it can be observed that for the LJX02 landslide, the main factor causing periodic displacement changes at the toe is the variation in reservoir water level, and the lag time increases with the elevation of the feature points. These findings align with the results of Wen et al. (Wen et al. Citation2023) obtained via Pearson correlation analysis, indicating a significant correlation between elevation and lag time. A lower elevation corresponds to a shorter lag time. Hence, rainfall exerts a more substantial influence on the rear edge of the landslide in comparison to changes in reservoir water level, with the impact of reservoir water level on the rear edge being negligible. The slope located in the middle of the landslide, as represented by feature point P, is affected by both reservoir water level and rainfall, as shown in and , where the wavelet coherence values for rainfall and point P are around 0.95, while those for reservoir water level and point P are around 0.8. Consequently, rainfall emerges as the dominant factor influencing deformation in the middle section of the landslide in comparison to reservoir water level.
It is worth mentioning that previous studies mostly focused on the relationship between landslides in direct contact with the reservoir water surface and reservoir water level fluctuations, and many studies have confirmed the influence of reservoir water level fluctuations on such landslides (Wu et al. Citation2021, Yang et al. Citation2019, Yi et al. Citation2022, Zhou et al. Citation2018). In contrast to previous research, this study selected the LJX02 landslide in the reservoir area, which is not directly adjacent to the water, as the research object. The aim was to explore whether landslides in the reservoir area without direct contact with the water would also be affected by reservoir water level fluctuations, which has not been previously investigated. The objective results clearly indicate a strong correlation between the foot and middle positions of the LJX02 landslide and the periodic release of water, influenced by factors such as water thrust, groundwater, and soil infiltration pathways. This demonstrates that landslides in the reservoir area without direct contact with the water are also influenced by periodic water releases. Furthermore, previous studies typically analyzed the nonlinear displacement components of the overall landslide displacement using wavelet analysis in relation to reservoir water level data (Tomás et al. Citation2016). The results obtained could only reflect the correlation between the overall landslide and reservoir water level fluctuations. Inspired by Wen et al. (Wen et al. Citation2023), who analyzed the relationship between point elevation and reservoir water level using Pearson correlation coefficient, this study selected three different elevation points of the landslide and quantified the lag relationship between periodic changes in reservoir water level and different positions of the landslide using wavelet transform. It was demonstrated that the influence of reservoir water level on different elevations of the landslide occurs in a sequential order. Building upon the previous findings regarding the different correlations between reservoir water level and different elevation points, this study further quantitatively verified that these differences are reflected in the lag time of landslide deformation caused by reservoir fluctuation. Lastly, the Liu Jia Xia hydropower station, as a critically important water control hub in the upper reaches of the Yellow River in China, has received very limited research attention over the past forty years since its completion in 1980. Most scholars have focused their studies on landslides in the Three Gorges Reservoir area. In recent years, some researchers have started to identify and monitor landslides in the Longyangxia Reservoir area in the upper reaches of the Yellow River (Fei et al. Citation2023). Inspired by this, this study conducted comprehensive landslide identification and monitoring in the Liu Jia Xia Reservoir area for the first time using InSAR technology. Additionally, wavelet transform was utilized to quantitatively analyze the lag relationship between the LJX02 landslide in the Liu Jia Xia Reservoir area and reservoir water level. These findings are of significant importance for the relevant water management departments in the upper reaches of the Yellow River for landslide prevention and mitigation.
6. Conclusions
This study employs the SBAS-InSAR technique in conjunction with optical imagery to detect landslides in the Liu Jiaxia reservoir area, resulting in the identification of 20 landslides. Focusing on the LJX02 landslide, which exhibits significant deformation on both sides of the reservoir, the study utilizes XWT and WTC to investigate the relationship between the InSAR-derived displacement time series and the impacting factors such as rainfall and reservoir water level. Through this analysis, the study successfully establishes the time lag relationship between different elevation zones of the LJX02 landslide and the aforementioned factors.
Specifically, the temporal deformation at the lowest point (P0) of the LJX02 landslide demonstrates a strong correlation with the reservoir water level, with a time lag of 152.10 days. On the other hand, the highest point (P) exhibits a substantial correlation with rainfall, with a time lag of 45.60 days. The intermediate point (P) shows correlations with both reservoir water level and rainfall, with time lags of 182.50 and 68.44 days, respectively.
The analysis results indicate that the impact of reservoir water level gradually weakens from low to high elevation zones of the LJX02 landslide, while the influence of rainfall decreases from high to low elevation zones. This variation can be attributed to the different effects of hydrostatic thrust, soil saturation, and groundwater caused by the periodic changes in reservoir water level and seasonal rainfall across the different elevation zones. These discrepancies are evident in the XWT and WTC results, revealing distinct time lag relationships.
In summary, by using the LJX02 landslide as an example, this study showcases the feasibility of examining the correlation and time lag relationship between landslide impact factors (such as rainfall and reservoir water level) and landslides through the combined analysis of InSAR time series and wavelet analysis. Furthermore, the study introduces a novel quantitative approach utilizing wavelet transform to interpret InSAR time series, emphasizing the advantages of wavelet transform tools and their significance in analyzing potential correlations and time lag relationships between signals.
Acknowledgments
I would like to express my gratitude to myself for fighting this battle alone.
Data availability statement
The data presented in this study are available on request from the first or corresponding authors.
Disclosure statement
No potential conflict of interest was reported by the author(s).
Additional information
Funding
References
- Bales S. 2022. Policy uncertainty and the Sovereign-Bank Nexus: a time-frequency analysis using wavelet transformation. Finance Res Lett. 44(1544-6123):102038. doi: 10.1016/j.frl.2021.102038.
- Dai K, Deng J, Xu Q, Li Z, Shi X, Hancock C, Wen N, Zhang L, Zhuo G. 2022. Interpretation and sensitivity analysis of the insar line of sight displacements in landslide measurements. GISci Remote Sens. 59(1):1226–1242. doi: 10.1080/15481603.2022.2100054.
- Dai K, Li Z, Xu Q, Burgmann R, Milledge DG, Tomas R, Fan X, Zhao C, Liu X, Peng J, et al. 2020. Entering the era of earth observation-based landslide warning systems: a novel and exciting framework. IEEE Geosci Remote Sens Mag. 8(1):136–153. doi: 10.1109/MGRS.2019.2954395.
- Ding YX, Peng SZ. 2020. Spatiotemporal trends and attribution of drought across China from 1901 - 2100. Sustainability. 12(2):477. doi: 10.3390/su12020477.
- Dong J, Zhang L, Liao M, Gong J. 2019. Improved correction of seasonal tropospheric delay in insar observations for landslide deformation monitoring. Remote Senting of Environ. 233(0034-4257):1–18. doi: 10.1016/j.rse.2019.111370.
- Dun J, Feng W, Yi X, Zhang G, Wu M. 2021. Detection and mapping of active landslides before impoundment in the Baihetan Reservoir Area (China) based on the time-series insar method. Remote Senting 13. doi: 10.3390/rs13163213.
- Dykes AP, Bromhead EN. 2018. New, simplified and improved interpretation of the vaiont landslide mechanics. Landslides. 15(10):2001–2015. doi: 10.1007/s10346-018-0998-9.
- Fei XF, Tian Y, Zhao CY, Liu HM, Chen HW. 2023. Ldentification and deformation monitoring of unstable slopes in Longyangxia Reservoir Area. The upper reach of Yellow River, China based on multi- temporal Lnsar technology. J Earth Sci Environ. 45(1672-6561):578–589. doi: 10.19814/j.jese.2022.11042.
- Fu BJ, Xia WR, Zhu Z. J 1989. Study on the Stability of the Left Shoulder Slope of the Liujiaxia Hydropower Station. Chinese J Rock Mech Engin. 1000-0860:43–51. doi: 10.13928/j.cnki.wrahe.
- Gabriel AK, Goldstein RM, Zebker HA. 1989. Mapping small elevation changes over large areas; Differential radar interferometry. J Geophys Res. 94(B7):9183–9191. doi: 10.1029/JB094iB07p09183.
- Gao BH, He Y, Zhang LF, Yao S, Yang W, Chen Y, He X, Zhao ZA, Chen HS. 2023. Dynamic evaluation of landslide susceptibility by CNN considering insar deformation: A case study of Liujiaxia Reservoir. Chinese J Rock Mech Engin. 42(1000-6915):450–465. doi: 10.13722/j.cnki.jrme.2022.0266.
- Grinsted A, Moore JC, Jevrejeva S. 2004. Application of the cross wavelet transform and wavelet coherence to geophysical time series. Nonlin Processes Geophys. 11(5/6):561–566. doi: 10.5194/npg-11-561-2004.
- Issartel J, Bardainne T, Gaillot P, Marin L. 2014. The relevance of the cross-wavelet transform in the analysis of human interaction - a tutorial. Front Psychol. 5(1664-1078):1566. doi: 10.3389/fpsyg.2014.01566.
- Jiang J, Ehret D, Xiang W, Rohn J, Huang L, Yan S, Bi R. 2011. Numerical simulation of Qiaotou landslide deformation caused by drawdown of the three Gorges Reservoir, China. Environ Earth Sci. 62(2):411–419. doi: 10.1007/s12665-010-0536-0.
- Kang Y, Zhao C, Zhang Q, Lu Z, Li B. 2017. Application of Insar techniques to an analysis of the Guanling landslide. Remote Senting. 9:1–17. doi: 10.3390/rs9101046.
- Li X,Ma J,Hu G. 2007. Genetic analysis on huge landslides alongthe section from Longyang Gorge to Liujia Gorge of the Yellow River. The Chinese Journal of Geological Hazard and Control. 18(01):28–32. doi: 10.3969/j.issn.1003-8035.2007.01.007.
- Liu P, Li ZH, Hoey T, Kincal C, Zhang JF, Zeng QM, Muller JP. 2013. Using advanced Insar time series techniques to monitor landslide movements in Badong of the three Gorges region, China. Int J Appl Earth Obs Geoinf. 21(0303-2434):253–264. doi: 10.1016/j.jag.2011.10.010.
- Matsuura S, Asano S, Okamoto T. 2008. Relationship between rain and/or meltwater, pore-water pressure and displacement of a reactivated landslide. Engin Geol. 101(1–2):49–59. doi: 10.1016/j.enggeo.2008.03.007.
- Ngui WK, Leong M, Hee LM, Abdelrhman AM. 2013. Wavelet analysis: mother wavelet selection methods. Appl Mech Mat. 393(2013):953–958. doi: 10.4028/www.scientific.net/AMM.393.953.
- Peng SZ, Ding YX, Wen ZM, Chen YM, Cao Y, Ren JY. 2017. Spatiotemporal change and trend analysis of potential evapotranspiration over the Loess plateau of China during 2011-2100. Agric for Meteorol. 233(0168-1923):183–194. doi: 10.1016/j.agrformet.2016.11.129.
- Peng SZ, Gang CC, Cao Y, Chen YM. 2018. Assessment of climate change trends over the loess plateau in China from 1901 to 2100. Intl J Climatol. 38(5):2250–2264. doi: 10.1002/joc.5331.
- Peng S. 2020. 1-Km monthly precipitation dataset for China (1901-2022). National Tibetan Plateau Data Center. doi: 10.5281/zenodo.3185722.
- Peng S, Ding Y, Liu W, Li Z. 2019. 1 Km monthly temperature and precipitation dataset for China from 1901 to 2017. Earth Syst Sci Data. 11(4):1931–1946. doi: 10.5194/essd-11-1931-2019.
- Rateb A, Kuo CY. 2019. Quantifying vertical deformation in the Tigris - Euphrates basin due to the groundwater abstraction: insights from grace and sentinel-1 satellites. Water. 11(8):1658. doi: 10.3390/w11081658.
- Shi X, Yang C, Zhang L, Jiang H, Liao M, Zhang L, Liu X. 2019. Mapping and characterizing displacements of active loess slopes along the upstream Yellow River with multi-temporal insar datasets. Sci Total Environ. 674(00489697):200–210. doi: 10.1016/j.scitotenv.2019.04.140.
- Sloukia FE, Bybi A, Drissi H. 2017. Selection of mother wavelets for analyzing bearing vibration signals. 3rd International Conference on Electrical and Information Technologies. IEEE; p. 1–6.
- Su L, Miao CY, Borthwick AGL, Duan QY. 2017. Wavelet-based variability of Yellow River discharge at 500 -, 100 -, and 50-year timescales. Gondwana Res. 49(1342-937X):94–105. doi: 10.1016/j.gr.2017.05.013.
- Sun Q, Zhang L, Ding X, Hu J, Li ZW, Zhu JJ. 2015. Slope deformation prior to Zhouqu, China landslide from insar time series analysis. Remote Senting of Environ. 156(0034-4257):45–57. doi: 10.1016/j.rse.2014.09.029.
- Tang HM, Wasowski J, Juang CH. 2019. Geohazards in the three gorges reservoir Area, China lessons learned from decades of research. Eng Geol. 261(0013-7952):105267. doi: 10.1016/j.enggeo.2019.105267.
- Tomás R, Li Z, Liu P, Singleton A, Hoey T, Cheng X. 2014. Spatiotemporal characteristics of the Huangtupo landslide in the three Gorges region (China) constrained by radar interferometry. Geophys J Int. 197(1):213–232. doi: 10.1093/gji/ggu017.
- Tomás R, Li Z, Lopez-Sanchez JM, Liu P, Singleton A. 2016. Using wavelet tools to analyse seasonal variations from insar time-series data: A case study of the Huangtupo landslide. Landslides. 13(3):437–450. doi: 10.1007/s10346-015-0589-y.
- Tomás R, Pastor JL, Béjar-Pizarro M, Bonì R, Ezquerro P, Fernández-Merodo JA, Guardiola-Albert C, Herrera G, Meisina C, Teatini P, et al. 2020. Wavelet analysis of land subsidence time-series: Madrid tertiary aquifer case study. Proc IAHS. 382(2199-899X):353–359. doi: 10.5194/piahs-382-353-2020.
- Torrence C, Compo GP. 1998. A practical guide to wavelet analysis. Bull Amer Meteor Soc. 79(1):61–78. doi: 10.1175/1520-0477(1998)079<0061:APGTWA>2.0.CO;2.
- Wang LZ, Song HL, An J, Dong B, Wu XY, Wu YZ, Wang Y, Li B, Liu QJ, Yu WN. 2023. Nutrients and environmental factors cross wavelet analysis of river Yi in East China: A multi-scale approach. Int J Environ Res Public Health. 20(1):1–19. doi: 10.3390/ijerph20010496.
- Wen NL, Dai K, Tomas R, Wu MT, Chen C, Deng J, Shi XL, Feng WK. 2023. Revealing the time lag between slope stability and reservoir water fluctuation from Insar observations and wavelet tools - a case study in Maoergai Reservoir (China). GISci Remote Sens. 60(1):1–18. doi: 10.1080/15481603.2023.2170125.
- Wu SS, Hu XL, Zheng WB, He CC, Zhang GC, Zhang H, Wang X. 2021. Effects of reservoir water level fluctuations and rainfall on a landslide by two-way anova and K-means clustering. Bull Eng Geol Environ. 80(7):5405–5421. doi: 10.1007/s10064-021-02273-8.
- Yang BB, Yin KL, Lacasse S, Liu ZQ. 2019. Time series analysis and long short-term memory neural network to predict landslide displacement. Landslides. 16(4):677–694. doi: 10.1007/s10346-018-01127-x.
- Yi XY, Feng WK, Wu MT, Ye ZP, Fang YF, Wang P, Li RJ, Dun JW. 2022. The initial impoundment of the Baihetan Reservoir Region (China) exacerbated the deformation of the Wangjiashan landslide: characteristics and mechanism. Landslides. 19(8):1897–1912. doi: 10.1007/s10346-022-01898-4.
- Zhao CY, Kang Y, Zhang Q, Lu Z, Li B. 2018. Landslide identification and monitoring along the Jinsha River Catchment (Wudongde Reservoir Area), China, using the Insar method. Remote Senting. 10(2072-4292):1–16. doi: 10.3390/rs10070993.
- Zhou C,Yin K,Cao Y,Ahmed B. 2016. Application of time series analysis and PSO–SVM model in predicting the Bazimen landslide in the Three Gorges Reservoir, China. Eng. Geol. 204:108–120. doi: 10.1016/j.enggeo.2016.02.009.
- Zhou C,Cao Y,Hu Xie,Yin K,Wang Yue,Catani F. 2022. Enhanced dynamic landslide hazard mapping using MT-InSAR method in the Three Gorges Reservoir Area. Landslides. 19(7):1585–1597. doi: 10.1007/s10346-021-01796-1.
- Zhou C, Yin KL, Cao Y, Intrieri E, Ahmed B, Catani F. 2018. Displacement prediction of step-like landslide by applying a novel Kernel extreme learning machine method. Landslides. 15(11):2211–2225. doi: 10.1007/s10346-018-1022-0.