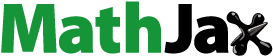
Abstract
Drugs, cosmetics, preservatives, fragrances, pesticides, metals, and other chemicals can cause skin sensitization. The ability to predict the skin sensitization potential and potency of substances is therefore of enormous importance to a host of different industries, to customers’ and workers’ safety. Animal experiments have been the preferred testing method for most risk assessment and regulatory purposes but considerable efforts to replace them with non-animal models and in silico models are ongoing. This review provides a comprehensive overview of the computational approaches and models that have been developed for skin sensitization prediction over the last 10 years. The scope and limitations of rule-based approaches, read-across, linear and nonlinear (quantitative) structure–activity relationship ((Q)SAR) modeling, hybrid or combined approaches, and models integrating computational methods with experimental results are discussed followed by examples of relevant models. Emphasis is placed on models that are accessible to the scientific community, and on model validation. A dedicated section reports on comparative performance assessments of various approaches and models. The review also provides a concise overview of relevant data sources on skin sensitization.
Introduction
Skin sensitizers are substances able to induce T cell-mediated type IV hypersensitivity immunoreactions in susceptible individuals after topical exposure. Repeated exposure eventually results in clinical manifestations such as skin reddening and itchy rashes, commonly termed allergic contact dermatitis (ACD) (Kimber et al. Citation2011). ACD is a commonly observed symptom among the general population. A large meta-study reported a weighted average prevalence of 19.5% of ACD involving at least one allergen, most commonly nickel, preservatives, and fragrances, in the general population (Thyssen et al. Citation2007). ACD is also a major cause of occupational illness (Lushniak Citation2004; Winkler et al. Citation2015), and its pervasiveness among hairdressers and dental technicians, for example, is well described (Goebel et al. Citation2018; Heratizadeh et al. Citation2018). The mechanisms involved in the induction of skin sensitization have been subject to extensive research and are relatively well understood. The currently accepted adverse outcome pathway (AOP) for skin sensitization comprises a total of 11 steps. Of these, four steps are considered to be key events: (i) molecular interaction of the substance with skin peptides and proteins (“haptenization”; this is the molecular initiating event (MIE)), (ii) activation and inflammatory responses of keratinocytes, (iii) activation of the skin’s dendritic cells, and (iv) proliferation of hapten-specific T cells (OECD Citation2012).
Most skin sensitizers are low-molecular-weight xenobiotic chemicals that bind covalently to skin proteins through a Michael addition, Schiff base formation, bimolecular nucleophilic substitution (SN2), nucleophilic aromatic substitution (SNAr), or acyl transfer (Aptula and Roberts Citation2006; Roberts, Aptula, et al. Citation2007; Chipinda et al. Citation2011; Enoch et al. Citation2011; Roberts Citation2013). Another type of common sensitizers is metals that form coordination complexes with skin proteins and, in the case of nickel, can directly interact with receptors (e.g. human Toll-like receptor 4; TCR) on immune cells (Garner Citation2004; Schmidt et al. Citation2010; Martin et al. Citation2011). Although some substances bind to skin proteins directly, others need to undergo activation through autoxidation (pre-haptens) or enzymatic reactions (pro-haptens) (Karlberg et al. Citation2008). The relevance of skin permeability in skin sensitization is a field of active research and is not yet fully understood (Alves et al. Citation2015a; Fitzpatrick et al. Citation2017a, Citation2017b).
Human data on skin sensitization remain sparse and of varying quality and reproducibility. From a regulatory point of view, animal experiments for skin sensitization currently constitute the most authoritative testing method for most risk assessment and regulatory purposes. Three animal experiments are accepted for regulatory purposes by the Organization for Economic Co-operation and Development (OECD): the guinea pig maximization test (GPMT), the Buehler guinea pig test (BGPT), and the rodent local lymph node assay (LLNA). Historically, the GPMT and BGPT have been the methods of choice (Ezendam et al. Citation2016). They have largely been succeeded by the LLNA, which is currently considered the most advanced animal testing system and serves as the primary reference method for the validation of alternatives to animal testing (AATs) (Anderson et al. Citation2011). Recent studies found that the LLNA correctly discriminates human skin sensitizers from non-sensitizers in approximately two out of three (Alves, Capuzzi, et al. Citation2018) to three out of four cases (Hoffmann et al. Citation2018). Besides the identification of a sensitization hazard, the LLNA also allows the determination of the skin sensitization potency of a substance as an EC3 value. The EC3 value is the concentration at which a substance evokes a three-fold stimulation of cell proliferation (measured in draining lymph nodes) in the treated groups compared with the control group. Knowing the potency of a substance is of high interest for risk assessment as this knowledge may allow the application of substances at a safe level of exposure (Adler et al. Citation2011; Goebel et al. Citation2017; Kimber et al. Citation2017).
The LLNA has significant error rates and outcomes can vary considerably. For example, an analysis of 87 substances for which binary LLNA results have been recorded from more than one study (using the same vehicle) identified contradictory outcomes for 19 (22%) of these substances (Dumont et al. Citation2016). Discordance is higher when different solvents or more than two potency classes are considered, as also reported by Hoffmann (Citation2015).
The LLNA and animal experiments, in general, evoke ethical concerns, and their value for human risk assessment is the subject of ongoing debate (Hartung Citation2013; Hartung 2017; Alves, Capuzzi, et al. Citation2018; Hoffmann et al. Citation2018). Effective since 2013, the European Union’s 7th amendment of the cosmetics directive (EUR-Lex Citation2009) prohibits the sale of cosmetics tested on animals. This leaves a challenging environment for the European cosmetics industry as the risk assessment for the qualification of cosmetic ingredients through alternative testing means such as in vitro, in chemico, and in silico methods is a paradigm shift (Goebel et al. Citation2012; Ezendam et al. Citation2016; Goebel et al. Citation2017; EUR-Lex Citation2009). Substantial efforts have been made by academic researchers, individual companies and associations from cosmetics, pharmaceutical and fragrance industries as well as institutional laboratories to replace animal experiments with a combination of alternative methods and assessment strategies in compliance with the 3 R (refinement, reduction, and replacement of animal usage in laboratory procedures) concept (Russell and Burch Citation1959; Basketter et al. Citation2012; Nendza et al. Citation2013; Johansson and Lindstedt Citation2014; Reisinger et al. Citation2015; Bergers et al. Citation2016; Ezendam et al. Citation2016).
Various non-animal testing methods for skin sensitization are available today (Mehling et al. Citation2012; Thyssen et al. Citation2012; Reisinger et al. Citation2015; Ezendam et al. Citation2016). Six testing methods, addressing the first three key events of the AOP, have been accepted by the OECD for regulatory purposes so far: The direct peptide reactivity assay (DPRA) addresses the MIE of the AOP by measuring the reactivity of a compound toward lysine or cysteine-containing peptides (OECD Citation2015a). KeratinoSensTM (CitationEURL ECVAM; KeratinoSens assay for the testing of skin sensitizers.) and LuSens (CitationEURL ECVAM; LuSens Assay) address the second key event of the AOP by measuring the activation of the transcription factor Nrf2 in keratinocytes (OECD Citation2015b). The U937 cell line activation test (U-SENS™), human cell line activation test (h-CLAT), and interleukin-8 reporter gene assay (IL-8 Luc assay) address the third key event of the AOP (OECD Citation2017a). U-SENS™ and h-CLAT assess the induction of cell surface marker (CD54/CD86) expression in dendritic-like cells (U937 and THP-1, respectively) as a measure for immunogenic cell activation, and the IL-8 Luc assay measures dendritic cell activation through changes in IL-8 cytokine secretion. In recent studies, these non-animal testing methods obtained accuracies (or correct classification rates, CCRs) in the range of 65% to 80% when measured against LLNA and human data (Hirota et al. Citation2017; Alves, Capuzzi, et al. Citation2018; Hoffmann et al. Citation2018).
Besides the OECD-accepted assays, several other promising approaches are in development and/or in the process of validation. Some assays, such as the SENS-IS assay (Cottrez et al. Citation2015) and the genomic allergen rapid detection (GARD) assay (Johansson et al. Citation2011, Citation2013) utilize genomic biomarker signatures to discriminate sensitizing from non-sensitizing substances. Genes relevant to the SENS-IS prediction model were identified by a combination of data mining, literature review, and experimental determination and include (i) a selection of 17 genes that contain a Keap1-Nrf2 signaling pathway-activated antioxidant response element in their promotor and (ii) 21 genes associated with several biological processes (inflammation, danger signals, cell migration) relevant to the activation of dendritic cells. Because of the use of skin models, the SENS-IS assay integrates skin penetration and metabolism properties of substances although the epidermis model may not completely reflect the in vivo situation. The prediction model of the GARD assay builds on a gene panel selected by an unbiased, genome-wide profiling of the transcriptional response of MUTZ-3 cells to a training set of 20 sensitizing and 20 non-sensitizing substances. The most descriptive genes were identified by principal component analysis (PCA) of differentially expressed genes and subsequent algorithm-based backward elimination (Johansson et al. Citation2011, Johansson, Rydnert et al. Citation2014). The SENS-IS assay, which is based on the EpiSkin skin model, addresses keratinocyte activation as the second key event of the AOP, whereas the GARD assay assesses the third key event by analyzing gene expression changes in a human myeloid leukemia cell (MUTZ-3)-derived cell line. Both assays were reported to show high accuracy for hazard identification (SENS-IS: 93% and 91% compared with LLNA or human data, respectively (Cottrez et al. Citation2016); GARD: 86% accumulated accuracy compared with LLNA data (Johansson, Rydnert et al. Citation2014; Johansson Citation2017)). Moreover, both assays were reported to indicate in vivo potency. Other approaches to improve predictions are based on the integration of additional parameters to existing testing concepts. For example, the peroxidase peptide reactivity assay (PPRA) adds a peroxidase-dependent oxidation of chemicals with the purpose to improve the detection of pro-haptens with in chemico assays. Potential differences of cell lines and primary cells regarding their metabolic capacity and biological responses to external stimuli motivated the development of an optimized protocol for the use of human peripheral blood monocyte-derived dendritic cells (Reuter et al. Citation2011). Hennen et al. (Citation2011) reported that co-culture of HaCaT cells and THP-1 cells increases the response of THP-1 cells to skin sensitizers compared with that of a monoculture of THP-1 cells. This pertains to the induction of CD54 and CD86, which are readouts essential for the h-CLAT prediction model. The added metabolic capacity of HaCaT cells and the release of keratinocyte danger signals are potential explanations (Hennen et al. Citation2011). Although the metabolic capacities of cell-based in vitro assays are limited, recent findings indicate that non-animal testing methods are also able to identify sensitizers that require activation through autoxidation or metabolism (Patlewicz et al. Citation2016; Urbisch, Becker, et al. Citation2016).
Animal experiments by nature cover the whole process of skin sensitization described in the AOP, including enzymatic or physiological activation of the sensitizer. In contrast, non-animal testing methods focus on single key events of the AOP (Ezendam et al. Citation2016; Casati et al. Citation2018). Therefore, the combination of different non-animal testing methods and integration with in silico methods is recommended, in particular for the task of potency prediction (Raunio Citation2011; Mehling et al. Citation2012; Johansson and Lindstedt Citation2014; Ezendam et al. Citation2016; Goebel et al. Citation2017; OECD Citation2017a; Casati et al. Citation2018). Recent studies indicate that such strategies can yield higher prediction accuracies in human hazard estimation than animal experiments (van der Veen et al. Citation2014; Urbisch et al. Citation2015; Alves, Capuzzi, et al. Citation2016; Benigni et al. Citation2016; Ezendam et al. Citation2016). Addressing each key event of the AOP individually can also be advantageous for the investigation of the underlying mechanisms (Steiling Citation2016).
Computational methods promise the ability to predict the skin sensitization potential of substances based solely on their molecular structures. Compared with experimental methods, computational approaches offer the advantage of producing predictions quickly, thus enabling the interactive optimization of compounds. In addition, these methods are cost-effective (Leontaridou et al. Citation2016), do not require materials for testing, and are not affected by difficulties common to experimental approaches, such as limited solubility, aggregate formation, and evaporation (Hartung Citation2013). Some recent studies suggest that in silico tools could eventually outperform in vitro and in chemico tools, provided that sufficient data will become available for model development (Asturiol et al. Citation2016). In contrast to experimental methods, in silico tools require defined molecular structures, which are not always accessible, such as in the case of some natural products (Kleinstreuer et al. Citation2018). In addition, computational methods are generally not applicable to mixtures and metals. Their predictivity and applicability are limited by the quality and quantity of available human, animal, and non-animal data. Luechtefeld et al. (Luechtefeld, Rowlands, et al. Citation2018) pointed out that future experimental testing efforts should, therefore, focus on the generation of data that can improve model development rather than individual compounds of interest.
In 2008, Patlewicz and Worth produced two reviews that provide a comprehensive overview of computational methods for skin sensitization prediction (Patlewicz and Worth Citation2008; Patlewicz et al. Citation2008). Recently, Alves et al. (Alves, Capuzzi, et al. Citation2018) published a perspective on skin sensitization prediction in which they discuss some of the most relevant computational approaches and data sources.
This work is a comprehensive review of relevant computational approaches for skin sensitization prediction, with a focus on methods and models that have been published after the reviews of Patlewicz et al. and are accessible to the public.
Data sets
Human data on skin sensitization should by nature be most suitable for the development of predictive methods for this endpoint in humans. However, human data remain scarce, vary in quality, and are often difficult to interpret because most of the available human data are no-observed-adverse-effect-levels (NOAELs), which are difficult to interpret and exploit in the context of model development (Politano and Api Citation2008). Deriving potency information from human epidemiological data is more complex than deriving it from animal testing experiments as it is based on the weighted analysis of (aggregated) exposure and the corresponding number of sensitization incidences. In consequence, non-animal testing approaches and in silico models have primarily been developed and validated based on animal data, in particular, LLNA data.
In recent years, a large number of data sets of human, animal, and non-animal data on skin sensitization have been used for model building. However, a closer look reveals that few of these data sets contain significant amounts of new measured data. Most of them are compiled from a few existing sources and thus have substantial overlaps with one another. The most important differences between these data sets is how the data were curated, conflicting information was handled and class labels were assigned.
Here, we will focus on two of the most relevant data sets on skin sensitization: the most comprehensive curated data set on the skin sensitization potential of substances (compiled by Alves et al.; Alves, Capuzzi, et al. Citation2018) and a high-density data set of compounds relevant to cosmetic application (compiled by Cosmetics Europe; Hoffmann et al. Citation2018).
Data set compiled by Alves et al.
The Alves data set includes binary LLNA data for 1000 compounds, DPRA data for 194 compounds, KeratinoSensTM data for 190 compounds, h-CLAT data for 160 compounds, and human data for 138 compounds. The data set was prepared following an elaborate data curation protocol that includes, among many other steps, the removal of entries with discordant biological outcomes for the same data type. The provenance of the data is documented. Data concordance and chemical space analyses provide additional information on the consistency and coverage of the individual subsets. For example, the authors found that 65% to 79% of the binary data recorded for any of the three non-animal testing methods are in agreement with the LLNA outcomes. They also reported that for 801 of the 1000 substances measured in the LLNA no other types of data were available.
The LLNA data that is included in the Alves data set was compiled from the work of Luechtefeld et al. (Citation2016), Jaworska et al. (Citation2013), and from the NICEATM LLNA database (ICCVAM Citation2013). LLNA data on 566 unique compounds (197 sensitizers and 369 non-sensitizers; after curation by Alves et al.) originate from the Luechtefeld data set, representing a collection of publically available in vivo and non-animal data on the skin sensitization potential of (primarily) high-production volume chemicals, all of which have been submitted for the REACH registration process. The REACH data set (as evaluated by Luechtefeld et al.) contains information on close to 20 000 studies conducted on the skin sensitization potential and potency of substances but requires further curation prior to use for model development. The current version of the REACH data set is available on the website of the European Chemical Agency (ECHA; ECHA. Homepage) and can be filtered through the OECD eChemPortal (OECD; eChemPortal). Most recently, Fitzpatrick et al. (Citation2018) extracted GPMT and LLNA data on the skin sensitization potential of 1295 substances mainly from this database.
LLNA data on 145 substances included in the Alves data set originate from the work of Jaworska et al. (Citation2013). The aim of Jaworska et al. was the compilation of a diverse, high-quality data set on the skin sensitization potency of substances for which LLNA, in chemico and in vitro data (i.e. DPRA, KeratinoSens™, and a CD86 activation assay based on the U937 cell line) are available. As such, the substances included in this data set cover different potency classes (from non-sensitizers to extreme sensitizers) and a wide range of physicochemical properties and usage classes (e.g. fragrances, preservatives, dyes, dye precursors, and solvents). Jaworska et al. applied strict quality filters. For example, they only included data derived in agreement with the corresponding OECD protocols and for which either a negative result or a clear dose-response curve is reported.
The LLNA data for 516 substances (332 sensitizers and 184 non-sensitizers) included in the Alves data set originate from the NICEATM LLNA database (ICCVAM Citation2013). The NICEATM LLNA database is one of the most comprehensive collections of EC3 data on a diverse range of chemicals. The database has been compiled from, among other sources, the work of Gerberick et al. (Citation2005), the work of Kern et al. (Citation2010) and donated company data. The documentation of the data provenance allows the lookup of the original sources of individual data points. Importantly, the vehicle of each study is also recorded, which can support the interpretation of discordant data.
The Alves data set also contains human data on the skin sensitization potential of 138 substances. These data originate from the ICCVAM human database (ICCVAM Citation2011) and the Strickland data set (Strickland et al. Citation2017). The ICCVAM human database consists of 302 substances that have been compiled for the evaluation of LLNA potency prediction. The Strickland data set consists of 96 substances covering a wide area of product usages (e.g. manufacturing chemicals, food additives, pharmaceuticals, fragrances, personal care products, pesticides, and cosmetics). In addition to human data, the Strickland data set also contains LLNA data, outcomes from non-animal testing approaches (DPRA, KeratinoSens™, and h-CLAT), as well as (primarily measured) data on six relevant physicochemical properties for the same substances for which human data are provided.
The non-animal testing results collected by Alves et al. originate from the data set of Urbisch et al. (Citation2015). The Urbisch data set includes LLNA-derived EC3 values for 213 substances for which information from at least two non-animal testing methods was available. The data set covers substances from diverse use contexts such as pharmaceuticals, pesticides, fragrances, and preservatives. The LLNA data are accompanied – when available – by human data and results from non-animal testing methods (DPRA, KeratinoSens™, LuSens assay, h-CLAT, myeloid U937 skin sensitization test (MUSST), and modified MUSST (mMUSST)).
Overall, the comprehensiveness and quality of the Alves data set make it one of the most valuable resources for the development of computational models. However, some information that may be of value for the interpretation and modeling of the data has not been transferred from the original source into the Alves data set, such as potency information (e.g. EC values or potency classes), data measured for mixtures, and information on repeated measurements. The documentation of data provenance allows the retrieval of such information from the original sources.
Cosmetics Europe data set
Cosmetics Europe, the trade association of the cosmetics industry in Europe, compiled an almost complete matrix of 128 substances measured with the DPRA, KeratinoSensTM, h-CLAT, U-SENSTM, and SENS-IS assays (Hoffmann et al. Citation2018). The data are accompanied by LLNA-derived potency information, human potency categorization according to Basketter et al. (six classes; Basketter et al. Citation2014), and information on six (primarily measured) physicochemical properties associated with skin penetration and protein binding.
The substances included in this data set are of high relevance to the cosmetic application and include 58 fragrances, 16 preservatives, 9 actives, 7 surfactants, 7 dyes, 6 pharmaceuticals, and 25 substances assigned to other categories. Thirty-eight of these substances are Michael acceptors, 21 are Schiff base electrophiles, 11 are SN2 electrophiles, 9 are acyl transfer agents, and 2 are SNAr electrophiles (41 were not assigned to a reaction domain). The substances have a molecular weight between 30 and 605 Da and a water solubility (logS) between –7 and 2.
The LLNA data included in the Cosmetics Europe data set were retrieved from several sources, including the NICEATM LLNA database and a proprietary database from the Research Institute for Fragrance Materials (RIFM). For 57 substances, EC3 values were collected from more than one LLNA study and merged using a newly developed, median-like location parameter. Human data were collected from Basketter et al. (Citation2014) and Api et al. (Citation2017), whereas non-animal testing data were retrieved from, among others, Urbisch et al. (Citation2015) and Natsch et al. (Citation2013). The non-animal testing data not only comprise binary testing results but also several (partly quantitative) outcomes for each testing method.
All data included in the Cosmetics Europe data set are based on experiments following standard protocols, thereby facilitating the comparability and reliability of the data. Approximately one-third of the non-animal testing data were newly generated by Cosmetics Europe. Any external data were individually reviewed and cross-checked by a second reviewer to ensure the high quality of the data.
The number of substances covered by the Cosmetics Europe data set is comparable with that of other data sets that include different data types (e.g. the data sets of Strickland et al. (Citation2017), Jaworska et al. (Citation2013), and Urbisch et al. (Citation2015)). However, the Cosmetics Europe data set includes significantly more results from non-animal testing methods. Its high quality and consistency make it a valuable resource, in particular for the benchmarking of new theoretical and experimental methods for the prediction of the skin sensitization potency of substances. In comparison with the Alves data set, the Cosmetics Europe data set has a much higher information density for individual substances and a clear focus on substances relevant to the cosmetic application. In contrast, the Alves data set is designed to cover the largest possible chemical space. As such, the Alves data set is particularly valuable for the development of machine learning models for the classification of substances into skin sensitizers and non-sensitizers.
Computational methods for skin sensitization prediction
In this section, we discuss important AATs to predict skin sensitization that either are pure theoretical methods (e.g. rule-based approaches, statistical models, machine learning, models and hybrid models) or (can) include a computational component. A schematic overview of these approaches is provided in . Additional information on the most relevant in silico models is reported in .
Figure 1. Overview of approaches for skin sensitization prediction that are either pure in silico models or can include a computational component.

Table 1. In Silico models for the prediction of skin sensitization potential or potency.
(Q)SAR modeling approaches
(Q)SAR approaches aim to describe the correlation between the structure and activity of compounds (Gleeson et al. Citation2012; Cherkasov et al. Citation2014). In the current context, activity is the skin sensitization potential or potency. Classification models are primarily utilized for the categorization of compounds according to their predicted skin sensitization potential whereas regression models are most commonly used for the quantitative prediction of potency. For risk assessment, quantitative predictions are clearly preferred over categorical models because the latter generally require to adopt the assumption of maximum activity within the predicted category (Adler et al. Citation2011; Goebel et al. Citation2017; Kimber et al. Citation2017). Where possible, a combination of regression and classification models can be of advantage with respect to the accuracy, applicability domain (AD), and interpretability of models.
Depending on the scale of the chemical space covered, (Q)SAR models can either be local or global (Helgee et al. Citation2010). Local models cover a well-defined, narrow chemical space within which they generally obtain high prediction accuracy. In contrast, global models aim to cover the broadest possible chemical space and thus have a large AD, often at the cost of prediction accuracy. As is true for most toxicological endpoints, the relationships observed between chemical structure and skin sensitization potency is not linear, in particular for more diverse sets of data. It is, therefore, generally difficult to develop linear models for toxicity prediction with a large AD. Nonlinear machine learning approaches have proven particularly successful in this context but are more difficult to interpret (Gleeson et al. Citation2012).
The OECD has defined guidelines for (Q)SAR models for use in a regulatory environment (OECD Citation2014b). These include that the models should have a defined endpoint, an unambiguous algorithm, a defined domain of applicability, appropriate measures of goodness-of-fit, robustness and predictivity, and, if possible, should allow a mechanistic interpretation. The latter is also important for the validation of models because it reduces the risk of overfitting due to non-causal but correlated features (Luechtefeld, Rowlands, et al. Citation2018).
Whereas goodness-of-fit (how well the model accounts for the variance of the response in the training data) and robustness (how stable the model is when one or more instances of training data are removed) of a model can be evaluated internally during model development, predictivity (how well the model can predict new data) can only be evaluated externally on the basis of new data not used for model development (OECD Citation2014b). This external evaluation requires the available data to be split into a training and a test set prior to modeling, and the test set must be used for model evaluation only. Unfortunately, for a significant number of available models, no such external tests have been conducted, primarily because of the scarcity of data on skin sensitization. Instead, only results for cross-validation or, in the worst case, only the training data, are reported. The first may lead to an overestimation of model performance; the latter almost certainly will. But even in cases where external validation is carried out, analyses of the representativeness and diversity of the test data with respect to the training data, as well as the AD of a model, are all too often missed.
The AD of a model describes the property and/or structural space of substances for which a model can make reliable predictions. Consideration of the AD is therefore of the essence to the application of any model. The AD is based on the assumption that similar predictivity can be achieved for substances that are similar to those in the training data. It is therefore considered to depend on structural, physicochemical, and response information in the data used for training a model, with the selection of important parameters also depending on the modeling algorithm used (OECD Citation2014b). A large number of different methods are available for the definition of the AD. For an overview of these techniques, the reader is referred to the OECD’s Guidance Document on the Validation of (Quantitative) Structure-Activity Relationship [(Q)SAR] Models (OECD Citation2014b).
Several thousand molecular descriptors are at our disposal today (Todeschini et al. Citation2009). They can be differentiated according to the type of information they encode. 0D descriptors encode properties that can be directly derived from the chemical formula (e.g. molecular weight, number of heavy atoms), whereas 1D descriptors capture the presence or absence of substructures in a molecule. 2D descriptors are derived from the molecular graph and encode the atom connectivity of molecules. Finally, 3D descriptors are derived from the 3D structure of molecules and capture, for example, the molecular surface area or quantum chemical properties such as HOMO-LUMO energies. For models to be mechanistically interpretable and robust, the use of small sets of physically meaningful descriptors is preferred. Therefore, for the development of skin sensitization models, experts often choose to work with descriptors encoding properties related to the ability of a compound to penetrate the skin (e.g. molecular weight, molecular volume, and logP) and react with skin proteins (e.g. HOMO-LUMO energy gap, activation energies, and reaction rates). A plethora of descriptor selection methods also is available that allow the automated selection of small numbers of descriptors with high information content.
Linear models
Chemical class or mechanism-based models. One of the earliest examples of linear QSAR models for skin sensitization prediction is the relative alkylation index (RAI) model developed by Roberts and Williams (Citation1982). This model allowed the quantitative prediction of the sensitization potential of sultones as a function of their reactivity toward proteins. In its most general form, the original RAI model can be formulated as
with D being the molar dose, k the alkylation rate constant, P the partition coefficient between a standard polar/nonpolar solvent, and a and b being constant prefactors.
Since the publication of the initial RAI model, further RAI models, applicable to a defined set of structurally closely related chemicals, and quantitative mechanistic models (QMMs), applicable to a wider range of compounds that share similar reaction chemistry, have been developed. For a comprehensive review of these types of models, the reader is referred to the work of Patlewicz and Worth (Citation2008). More recently, the RAI/QMM concept has been applied to predict the skin sensitization potency of epoxides (Roberts, Aptula, et al. Citation2017), aldehyde Schiff bases (Roberts, Schultz, et al. Citation2017), Michael acceptor electrophiles (Roberts and Natsch Citation2009; Wondrousch et al. Citation2010) and molecules undergoing aromatic substitutions (Roberts et al. Citation2011; Ouyang et al. Citation2014; Roberts and Aptula Citation2014). Although the original RAI model required the experimental measurement of P and k to derive the skin sensitization potential of a compound of interest, more recent models either incorporate calculated p values or neglect the parameter and derive k based on precalculated reactivity parameters (Roberts, Schultz, et al. Citation2017).
Enoch and Roberts (Citation2013) reported a linear model for the prediction of the potency (pEC3 value) of Michael acceptors as a function of the available surface area at the site of reaction and the stability of the expected reaction intermediate (which correlates with the reaction rate k). The latter descriptor is calculated from the sum of the ground state energies of the query molecule and a probe, as well as the energy of the charged intermediate using density functional theory (DFT). The model was developed based on LLNA data for 33 Michael acceptors and predicted pEC3 values with an R2 of 0.79 (after the removal of several outliers).
Linear approaches for the prediction of aromatic substitutions include a model by Promkatkaew et al. (Citation2014), who found a moderate correlation (r2 = 0.64) between the energy barriers (derived by modeling the reaction pathways with DFT) and pEC3 values of 12 sensitizers. Interestingly, no correlation was found between the HOMO−LUMO energy (which is frequently used to encode chemical reactivity) and the pEC3, indicating that the HOMO−LUMO energy is not a relevant attribute for this class of compounds and reactions.
All of these RAI/QMM models have in common that they are based on only a few, mechanistically interpretable features, which minimizes the risk of overfitting. However, the models are derived from very small, focused data sets, which greatly limit their AD. This limitation can be mitigated through combination with other models, provided that the reaction domains and molecular classes of substances of interest can be correctly assigned. Many hybrid approaches combine local linear models, as described in the section “Hybrid in silico models.”
Models applicable to a wider range of chemical classes and mechanisms. Linear models with a broader AD make use of larger and more diverse data sets and often use feature selection algorithms to identify a small subset of relevant descriptors. The descriptors selected by these automated procedures are not necessarily physically meaningful or easily interpretable. Manual refinements based on expert knowledge are therefore generally advised or even necessary. This type of model is also limited by the fact that the relationship between substances and their skin sensitization potency or potential is not linear when observed on a larger scale.
More broadly applicable linear (Q)SAR models include a categorical model for the prediction of skin and respiratory sensitization potential (Warne et al. Citation2009). This model is based on a data set of 119 compounds annotated with GPMT and LLNA, as well as animal and human inhalation data. Most of these data have been obtained from the Annex I of the Dangerous Substances Directive of the European Union (67/548/EEC). Linear regression was performed to select the eight most relevant descriptors (representing molecular orbital energies, differences thereof, and electronegativity) for the discrimination of skin sensitizers and non-sensitizers from a set of 59 descriptors. Although the model was able to correctly identify four of the five skin sensitizers (and both respiratory sensitizers) from a test set of 17 substances, the ability of the model to discriminate skin from respiratory sensitizers was insufficient. The poor discrimination between these types of sensitizers is likely linked to shared chemical properties.
TOPKAT includes several categorical models for skin sensitization. The original TOPKAT models are based on the work of Enslein et al. (Citation1997). A global model combines two linear binary equations, one to distinguish non-sensitizers and sensitizers, and the other to further classify sensitizers into weak and strong categories. This global model is complemented by two local models, one covering aliphatic and single-benzene-ring-containing chemicals, and the second model covering the remaining aromatics. Historically, TOPKAT is one of the first models for skin sensitization to include a definition of the AD. For the original model, a specificity of 79% and a sensitivity of 82% were reported for an independent test set of 25 compounds. In addition to the original models, two newer, extensible models for the prediction of skin sensitization based on a modified Bayesian learning method are available (BIOVIA Citation2017a, Citation2017b). One of these models differentiates sensitizers and non-sensitizers, and the other model differentiates weak and strong sensitizers. Both extensible models make use of seven molecular descriptors, including logP, molecular weight, polar surface area, number of donors, acceptors and rotational bonds, and an atom type fingerprint. The classification model for sensitizers and non-sensitizers is trained on GPMT data for 392 compounds and achieved a ROC score of 0.77 in 10-fold cross-validation, whereas the strong vs weak sensitizer model was trained with GPMT data for 258 compounds and obtained a ROC value of 0.92 during leave-one-out cross-validation.
More recently, Toropova and Toropov (Citation2017) used the Monte Carlo approach implemented in CORAL to derive four continuous linear regression models from a training set of 147 compounds annotated with measured EC3 data. The models were derived based on hybrid optimal descriptors combining information calculated directly from SMILES strings and from hydrogen-suppressed molecular graphs. The best performing model obtained an r2 of 0.86 for the quantitative prediction of EC3 values for an external test set of 29 compounds. The skin sensitization potency of compounds was observed to increase with the presence of five-membered rings, aromatic six-membered rings, and double bonds.
Nonlinear models
In recent years a wide range of nonlinear approaches has been explored to model the complex relationships between substances and their skin sensitization potential and potency. In particular, machine learning algorithms can account for the nonlinear relationships observed in large and diverse data sets. With increasing amounts of data, manual curation by experts is often replaced with automated and less reliable data curation procedures, which can have a negative impact on the quality of data sets used for modeling.
Machine learning algorithms can deal with large numbers of descriptors. Often, a substantial number of descriptors are calculated and subjected to feature selection procedures prior to or as part of the model generation process. The use of large numbers of descriptors entails the risk of model overfitting. In addition, the inclusion of descriptors that are not physically meaningful adds to the black box character of complex machine learning models, making it difficult if not impossible to understand on which basis the algorithm assigns a substance to a certain biological outcome. Taken together, these issues often lead to the neglect or insufficient definition of the AD of models, which is not only problematic for the application of the individual models but also for the perception and reputation of computational methods in general.
Lu et al. (Citation2011) used recursive partitioning to derive a decision tree model for the binary classification of sensitizers and non-sensitizers based on LLNA data for 295 compounds, including, among others, Michael acceptors, SN2 and SNAr electrophiles, Schiff base formers, and acyl transfer agents. Eight quantum chemical and physicochemical descriptors linked to chemical reactivity, hydrophobicity, and electrostatic interaction, as well as a fragment descriptor, served as the input for model building. The fragment descriptor encodes the presence or absence of eight substructural features by a single binary value. The final model correctly classified ∼80% of all (25 and 37) compounds of two test sets.
Alves et al. (Citation2015b) derived random forest models for the classification of skin sensitizers and non-sensitizers from a curated and balanced subset of 127 sensitizers and 127 non-sensitizers extracted from the NICEATM LLNA database. Two types of models were developed, one based on 0D, 1D and 2D descriptors calculated with Dragon (CitationTalete S.r.l. Dragon) and the other based on 2D SiRMS descriptors (simplex representation of molecular structure, encoding molecular structure by tetratomic fragments of fixed composition, structure, chirality, and symmetry; Muratov et al. Citation2010). A consensus model based on models derived from either type of descriptor performed best on an external test set containing 152 sensitizers but only five non-sensitizers, with a CCR of 0.86. Because of the strict definition of the AD applied in this test, predictions were only made for 24% of the compounds of the test set. A consensus model with a less stringent definition of the AD reached slightly lower predictivity (CCR = 0.83) but higher coverage (50%) on the same test set. Five-fold cross-validation on balanced data resulted in comparable classification accuracies but significantly higher coverage (coverage 39% and 70%, depending on the definition of the AD). Based on this work, a free web service called Pred-Skin was developed, which allows the prediction of the skin sensitization potential of substances based on random forest models derived from Morgan2 fingerprints (Braga et al. Citation2017). Two of these models are binary classification models based on human skin sensitization data (for 109 compounds) and LLNA data (for 515 compounds). During five-fold cross-validation, these two models obtained CCRs of 0.80 and 0.84 for the two-thirds of all compounds that were within the AD. A third model discriminating three potency categories based on LLNA data obtained an accuracy of 0.76 and coverage of 78% under the same test scenario.
Yuan et al. (Citation2009) developed a binary support vector machine (SVM) classifier for the prediction of the skin sensitization potential of substances based on LLNA and GPMT data on 108 and 61 organic compounds (including, among others, alkanes, aromatic hydrocarbons, alcohols, amines, acids, and esters), respectively. Particle swarm optimization (PSO) was used for the selection of important 2D molecular descriptors from a set of 926. The final model was based on six molecular descriptors corresponding to the number of chlorine atoms, the molecular electronic structure, molecular size, and hydrophobic properties. It obtained classification accuracies of 89% and 90% on LLNA and GPMT data for 54 and 31 compounds in the two test sets, respectively.
Within the EU-funded CAESAR project (CitationCAESAR. CAESAR project), two global binary categorical models for the prediction of the skin sensitization potential of compounds were developed based on LLNA data compiled for 167 chemicals (Chaudhry et al. Citation2010). One of the models was derived from an in-house adaptive fuzzy partition algorithm. As part of this approach, a hybrid method combining a genetic algorithm with stepwise regression was used to select seven relevant 2 D descriptors calculated with Dragon (i.e. the number of nitrogen, double-bonded oxygen, and non-aromatic conjugated sp2 carbon atoms, as well as descriptors accounting for topological features, charge, and valence connectivity). The model obtained 90% classification accuracy on a test set of 42 compounds (8 non-sensitizers and 34 sensitizers) and is distributed as a component of VEGA (Benfenati et al. Citation2013; CitationCAESAR. Skin sensitization model). The other model was derived with a multilayer perceptron neural network algorithm trained on a slightly modified training set. For the identical test set with a different threshold for the division between sensitizers and non-sensitizers (resulting in 21 non-sensitizers and sensitizers each), an accuracy of 71% was obtained with this model (which has not been implemented in VEGA).
Rule-based approaches
Knowledge-based expert systems have a long record of successful use in ADME (absorption, distribution, metabolism, and excretion) and toxicity prediction. A key component of these systems is dictionaries (sets of rules), which aim to encode existing empirical knowledge distilled from in vitro and in vivo data, as well as from clinical practice. These rules generally link structural fragments to mechanisms of skin sensitization but may also be more complex than simple structural alerts. For example, they may also take into account skin penetration, chemical reactivity, or steric accessibility. Rule-based methods are easily interpretable and inherently subjective.
The relevance of rule-based methods for skin sensitization prediction stems to a significant extent from the sparsity and limited reliability of the available data, which poses a bottleneck in model development. Whereas statistical approaches and machines require a significant number of instances to identify, support, and weigh patterns in the data, experts may be able to derive valid rules from a limited number of observations. In the absence of sufficient hard data, expert knowledge may allow artificial extension of the scope of rule-based approaches. For example, experts may implement rules stating that two chemical substructures behave similarly in a defined context (Enoch, Madden, et al. Citation2008).
On the downside, in certain cases, expert bias may hinder corrections of rule-based systems or even the further data collection. For example, molecules with a molecular weight above 500 Da have generally been assumed to be too large to diffuse into the epidermis and cause skin sensitization. As a consequence of this assumption, only a few compounds with a molecular weight above 500 Da have been evaluated regarding their skin sensitization potential. For example, among the of 211 compounds of the LLNA data set compiled by Gerberick et al. (Citation2005), only two compounds have a molecular weight above 500 Da, one of which is a known skin sensitizer (Fitzpatrick et al. Citation2017a). It is only more recently that awareness about the skin sensitization potential of compounds with a molecular weight above 500 Da has been raised (Roberts et al. Citation2013; Alves et al. Citation2015a; Fitzpatrick et al. Citation2017a; Luechtefeld, Rowlands, et al. Citation2018).
In the context of skin sensitization prediction, rule-based systems are nowadays more often part of hybrid computational models than used as individual models (see section “Hybrid in silico models”). However, several existing platforms allow the screening of substances of interest for the presence of structural features related to skin sensitization. One example is ToxAlerts (Sushko et al. Citation2011, Citation2012), which provides, among others, structural alerts for potential skin sensitizers based on sets of rules distilled from different sources (Barratt et al. Citation1994; Payne and Walsh Citation1994; Gerner et al. Citation2004; Kazius et al. Citation2005). The rule set which was originally implemented in the toxicity prediction model DEREK is also included in ToxAlerts. The current version of DEREK, Derek Nexus, includes additional modules for skin sensitization prediction, for which reason the software is discussed in the section “Hybrid in silico models.”
Another example of a rule-based system for the assignment of substances to one or several skin sensitization reaction domains (the “Skin Sensitisation Reactivity Domain” module) has been implemented in Toxtree (Enoch, Madden, et al. Citation2008; Toxtree). The rule set was derived from LLNA data measured for 208 compounds and encodes substructures associated with the five established skin sensitizing reaction domains. The structural alerts also take metabolism and oxidation (but not bioavailability) into account. Toxtree also features a set of 104 structural alerts for protein binding related to acylation, Michael addition, Schiff base formation, SN2 and SNAr (Enoch et al. Citation2011). As protein binding is the first key event in the AOP for skin sensitization, these structural alerts might also be useful for the prediction of skin sensitization.
The OECD QSAR Toolbox (OECD. The OECD QSAR Toolbox) provides many profilers that may be used for building chemical categories for subsequent read-across (see section “Read-across”) within the software package. In particular, the profilers for skin protein binding and general protein binding are of relevance to the prediction of the skin sensitization potential of compounds. Simulators of autoxidation and skin metabolism are also implemented in the OECD QSAR Toolbox and may be of value to the refinement of predictions. These simulators are also part of TIMES (OASIS-LMC; TIMES-SS Software), which includes additional capabilities for the assessment of biotransformations, information on the AD and metabolic maps.
In general, structural alerts alone are an insufficient predictor of the skin sensitization potential or potency (Alves, Muratov, et al. Citation2016). Toxtree and the profilers of OECD QSAR Toolbox, for example, are not intended to be used as predictors but rather as tools to assign substances of interest to reaction domains (Enoch, Madden, et al. Citation2008; OECD. The OECD QSAR Toolbox). Nevertheless, these tools are frequently investigated as potential predictors, i.e. such that any substance matching structural alerts related to one of the reaction domains is deemed a skin sensitizer (Urbisch, Honarvar, et al. Citation2016; Verheyen et al. Citation2017). However, comparative studies have shown that structural alerts may be able to improve predictions when used in combination with other approaches (Teubner et al. Citation2013; Verheyen et al. Citation2017; see the section “Comparative analyses of the performance of computational models for skin sensitization prediction”).
Read-across
Read-across is an approach for the prediction of endpoint information based on available data on the same endpoint of related substances (Patlewicz et al. Citation2013; OECD Citation2014a; Schultz et al. Citation2015). This method is a pillar of risk assessment for many toxicological endpoints but does not necessarily involve computation. Read-across can either be performed as an analog approach, where – in the absence of a trend or regular pattern of biological properties – a missing property value of a compound of interest is predicted based on one or several other compounds with known values for this property, or as a grouping approach, in which predictions for a compound of interest are derived from several structurally related source compounds with similar properties or properties following a regular pattern (Patlewicz et al. Citation2017). Like rule-based methods, read-across approaches are generally easily interpretable and extendable with new data (e.g. in-house data).
An example of a local read-across tool for the prediction of the skin sensitization potency of alkenes reacting through Michael addition has been implemented in VEGA (Enoch, Cronin, et al. Citation2008). It is based on a database of Michael acceptors with measured EC3 values and DFT-derived electrophilicity indices (ɷ). For any compounds of interest, the potency is derived based on the EC3 values of compounds with similar ɷ.
Recently, Alves et al. (Alves, Golbraikh, et al. Citation2018) published a multi-descriptor read-across (MuDRA) consensus model that integrates read-across based on various types of chemical descriptors and molecular fingerprints. In a test on 217 skin sensitizers and non-sensitizers, of which 42% were within the AD and considered in the analysis, the consensus model obtained a binary classification accuracy of 0.78. It is not entirely clear whether the improved prediction accuracy of the consensus approach over the best-performing individual model justifies the more complex approach.
Hybrid in silico models
Hybrid in silico models combine two or more of the above-mentioned components with the aim to improve prediction accuracy and the applicability of computational methods. For example, the combination of complementary approaches such as a reactivity model based on quantum mechanical calculations with a rule-based approach can be particularly beneficial for potency prediction. Importantly, the agreement or disagreement of predictions from individual components is not necessarily an indicator of reliability. For example, overlaps in the training data, the knowledge base and/or modeling methods of the individual components can lead to correlations (Rorije et al. Citation2013; Fitzpatrick et al. Citation2018). Care must be taken to not wrongly interpret such correlations as founded indications of the high reliability of predictions.
In the context of skin sensitization prediction, the majority of hybrid models are composed of two or more dependent modules that may not be used individually. However, there are also hybrid models in existence that integrate self-sufficient modules for skin sensitization prediction. These are commonly referred to as consensus models.
An example of a hybrid model that integrates several dependent models for the prediction of skin sensitization potency is Derek Nexus (formally Derek for Windows or DEREK; Barratt et al. Citation1994; Payne and Walsh Citation1994; Gerner et al. Citation2004; Kazius et al. Citation2005). The core component of Derek Nexus is an expert system based on 90 structural alerts for the prediction of skin sensitization potential that also includes functionality for verifying negative predictions by (i) comparing them to the structures of compounds that are known to be predicted as false-negatives by the model and (ii) scanning them for substructures not covered by the training data (Williams et al. Citation2016). An additional component predicts EC3 values for any compounds triggering a skin sensitization alert by calculating the weighted average of the EC3 values of 3–10 nearest neighbors (of an LLNA data set containing a total of 465 compounds) matching that alert (Canipa et al. Citation2017). The molecular similarity of individual pairs of molecules is evaluated based on an in-house radial molecular fingerprint. A likelihood level ranging from “certain” to “impossible” is provided together with the predicted EC3 value. For an external test set of 103 compounds, Derek Nexus correctly predicted the EC3 values of half of all tested compounds with less than a five-fold deviation from the LLNA-derived value. In addition, the software correctly assigns 64% of all tested compounds to one of the three categories of the Globally Harmonized System of Classification and Labelling (GHS) recommended by the United Nations Economic Commission for Europe (UNECE) for a standardized classification of skin sensitizers and non-sensitizers according to potency. The error rates differed significantly depending on the skin sensitization alert triggered within Derek Nexus. They were particularly high for metal and metal salts, as well as for substituted phenols and their precursors. In a recent evaluation (Chilton et al. Citation2018), Derek Nexus obtained a sensitivity of 54% and a specificity of 77% when used to discriminate between 302 skin sensitizers and 683 non-sensitizers measured with different animal testing systems. Derek Nexus can be combined with Meteor Nexus to also assess the skin sensitization potential of likely metabolites.
The OECD QSAR Toolbox (OECD. The OECD QSAR Toolbox) offers the combination of several rule-based profilers (see section “Rule-based approaches”) with read-across to find adequate analogs or build chemical categories. The OECD QSAR Toolbox provides experimental data on various endpoints for a large number of substances.
TIMES-SS (Dimitrov et al. Citation2005; Mekenyan et al. Citation2012; CitationOASIS-LMC, TIMES model for skin sensitization prediction) is a hybrid model for the semi-quantitative prediction of the skin sensitization potency of substances. The predictor is part of the TIMES platform for toxicity prediction. TIMES includes a large collection of models for the prediction of human endpoints and metabolism. It also includes modules for the prediction of autoxidation and volatility, which can support the prediction of skin sensitization (Patlewicz, Kuseva, Mehmed, et al. Citation2014). TIMES-SS was developed based on 875 substances annotated with GPMT, LLNA, and human and animal data. The model combines a skin metabolism simulator with several local models for the assignment of three sensitization classes. Substances of interest are analyzed through 420 hierarchical ordered transformations (sorted by probability of occurrence) that link a source to a product structural fragment. The transformations account for abiotic reactions, covalent interaction with proteins and phase I and II metabolic reactions. Whenever a covalent interaction with a skin protein is predicted to occur, the compound is classified as either a strong or a weak sensitizer (depending on the triggered alert), or it is further analyzed by one of the several local 3D QSAR models that differentiate between non-, weak, and strong sensitizers. These 3D QSAR models take parameters such as the HOMO and LUMO energies, the HOMO-LUMO energy gap, molecular weight, electronegativity, hydrophobicity, and acceptor superdelocalizability as input (Mekenyan et al. Citation2004).
The AD of TIMES-SS is defined by the value range of several physicochemical properties and the structural and mechanistic domain covered by the training data. A representative test set of 40 REACH-relevant chemicals was selected from the European Inventory of Existing Commercial Chemical Substances (EINECS), taking into account commercial availability and structural diversity. The 40 compounds (16 sensitizers and 24 non-sensitizers) were evaluated in subsequent LLNA experiments. TIMES-SS correctly classified 30 compounds (9 sensitizers and 21 non-sensitizers) of the 40 compounds (Patlewicz et al. Citation2007; Roberts, Patlewicz, et al. Citation2007).
Several models relevant to skin sensitization prediction have also been implemented in the QSAR software CASE Ultra (Klopman Citation1992; Graham et al. Citation1996; Chakravarti et al. Citation2012; Saiakhov et al. Citation2013). These include models for the prediction of electrophilicity, protein binding (first key event of the skin sensitization AOP; trained on 194 compounds), the activation of the antioxidant response element (ARE) in keratinocytes (second key event of AOP; trained on 185 compounds), the activation of dendritic cells (third key event of AOP; trained on 189 compounds), LLNA outcomes (trained on 587 compounds) and ACD induction in humans and guinea pigs (trained on 1032 compounds). All of these models are purely of statistical nature and were derived with an algorithm based on the MultiCASE methodology (Klopman Citation1992). The algorithm generates a large number of structural fragments from sets of molecules and identifies fragments of statistical relevance for an endpoint of interest. Within this process, a hierarchical approach is applied to divide the training set into logical subsets. In contrast to the structural alerts used in most rule-based approaches, CASE Ultra not only encodes structural fragments related to skin sensitization (“biophores” or positive alerts) but also takes into account fragments that are identified as hindering skin sensitization (“biophobes” or deactivating alerts). Where feasible, local models are developed for each group of compounds sharing the same positive alert by stepwise linear regression incorporating, among others, logP, local charges, vapor pressure or presence or absence of modulating structural fragments as descriptors. The developers of CASE Ultra report 10-fold cross-validation accuracies of 67% to 87% for the different models relevant to skin sensitization prediction. The best performance was obtained with a model for the prediction of human and guinea pig ACD. A tool to perform the read-across analysis is also provided with CASE Ultra.
A quantitative hybrid model for the prediction of the skin sensitization potency of compounds that combines expert knowledge with a linear QSAR approach was developed by Dearden et al. (Citation2015). The hybrid model was developed based on a curated set of 204 known sensitizers annotated with measured EC3 values (non-sensitizers were not considered) from the data sets of Gerberick et al. (Citation2005) and Kern et al. (Citation2010). The compounds were assigned to one of seven different (pro-) reaction domains (i.e. acyl transfer, (pro-) Michael addition, (pro-) Schiff base, SN2, and oxidation potential). Local linear QSAR models were derived for four (pro-) reaction domains for which sufficient data were available. From an initial set of 1600 descriptors (including logP, water solubility, molar refractivity, surface area descriptors, vapor pressure, Gasteiger charges, E-State descriptors, and fragment descriptors), up to six descriptors were selected by a wrapper method of stepwise multiple linear regression (MLR) for the different local models. Although the potency of Michael acceptors was found to be well described by reactivity and (hydrophobic) surface area, the potency of substances in pro-Michael, acyl transfer, and the combination of Schiff base and pro-Schiff domains was found to correlate with several descriptors representing hydrogen bonding. The potency of Schiff bases correlated with polarity and molecular flexibility; the potency of molecules undergoing SN2 reactions increased with hydrophobicity and decreased with electron-donating ability. A range of the values of the descriptors covered by the training data is given for each local model as an indicator of whether a compound of interest is within the AD of the model. An R2 of 0.95 was reported for a set of 37 compounds covering the same chemical space as the training data. The compounds had previously been used for descriptor selection but not for model training. The model is applicable only to skin sensitizers that can be assigned based on expert knowledge to one of the reaction domains for which a local model was retrieved.
Another approach combining a linear QSAR method with an expert-curated set of rules has been implemented in the CADRE-SS model for predicting the skin sensitization potency of compounds (Kostal and Voutchkova-Kostal Citation2016; ToxFix). The three-class categorical hybrid model consists of three modules describing different steps in the sensitization process. In the first module, the permeability coefficient (logKp) is calculated by Monte Carlo simulation. The second module uses a set of rules, encoded in a similar way as those implemented in Toxtree, to assign the most likely reaction domain. Compounds for which no reaction domain could be assigned are assumed to be non-sensitizers. Any compounds predicted as sensitizers are passed on to the third module, which calculates the chemical reactivity of compounds based on ground-state, site-specific, or global physicochemical and quantum mechanical descriptors, depending on the reaction domain assigned. For each reaction domain, a linear model was developed that takes the predictions of modules one (skin permeability) and three (chemical reactivity) as input. In addition, a rule-based approach was implemented to account for the qualitative sensitizing potential of metal salts. CADRE-SS was trained on a set of 384 chemicals annotated with LLNA data. Confidence levels for the individual predictions are derived from the range of the descriptors values observed for the training set. Tested on a set of 100 compounds annotated with human data, animal data or both, the model correctly assigned more than 90% of these compounds to one of the three GHS skin sensitization potency categories. The authors emphasize that, in contrast to other in silico tools for the prediction of skin sensitization potential, CADRE-SS was applicable to all compounds of this test set.
Very recently, Luechtefeld et al. (Luechtefeld, Marsh, et al. Citation2018) reported two binary classifiers of different complexity for the prediction of the skin sensitization potential of compounds. Both classifiers are based on the combination of a fingerprint similarity analysis with machine learning. The basic model generates 2D vectors that describe the similarities of each of the substances in the database to the closest sensitizing and non-sensitizing neighbors. These are analyzed by logistic regression in a second, supervised modeling step. The basic model obtained binary classification accuracies of 68% during leave-one-out cross-validation on a data set of 4783 compounds. The more complex model takes dependencies between 19 different endpoints into account (described by 74 D vectors) and uses a random forest algorithm for prediction. This model was tested with five-fold cross-validation during which it obtained an accuracy of 84% on a data set of 7670 compounds. Because of its ability to handle missing data, the more complex model is more widely applicable. Both models are available within the proprietary platform REACHAcrossTM (Luechtefeld, Rowlands, et al. Citation2018; UL).
An example of a consensus model for the discrimination of sensitizers and non-sensitizers is a weight of evidence approach developed by Ellison et al. (Citation2010) that integrates results from the OECD QSAR Toolbox, Derek for Windows, the SMARTS pattern-based approach of Enoch et al. (Enoch, Madden, et al. Citation2008), and the CAESAR global model. The ability of the model to predict binary LLNA data was tested on 44 compounds that were published shortly before the consensus model was developed, for which reason the authors assume that these compounds are not part of the training data of any of the individual models. The consensus model produced conclusive results for 26 of the 44 test compounds, with 76% correct binary classifications. For 7 of the 44 test compounds, the binary predictions from all four models were in agreement and correct. For 18 compounds, the predictions were inconclusive.
Very recently, Alves et al. (Alves, Capuzzi, et al. Citation2018) reported a naive Bayes binary classification model for the prediction of the skin sensitization potential that integrates predictions from several in silico models provided within the same publication. The model was trained on 138 compounds annotated with human data and obtained a CCR of 89%. The model has been published as a free KNIME workflow (Naive Bayes Skin Sensitization Model v. 1.1).
Whereas hybrid in silico models integrate different computational methods and models, there are also approaches in existence that integrate results from one or several testing methods (mostly in vitro or in chemico assays) and in silico approaches to establish the skin sensitization potential or potency of a compound of interest. These are discussed in the section “Computational methods used in combination with non-animal testing results.”
The SkinSensDB platform (Wang et al. Citation2017; Tung et al. Citation2018) not only provides data relevant to skin sensitization (LLNA, human, DPRA/PPRA, KeratinoSensTM/LuSens, and h-CLAT) but also includes functionality for the prediction of the skin sensitization potential of compounds based on the integration of these experimental data. For compounds of interest, values for missing experimental data are derived by a read-across approach. Using the stored or derived non-animal testing data as input, two different integrated testing strategies can be utilized for the binary prediction of the skin sensitization potential in the LLNA and in humans. Depending on the selected minimum similarity threshold acceptable for the read-across approach, accuracies of up to 81% and 89% were obtained for the prediction of LLNA (∼350 compounds) and human (∼50 compounds) outcomes, respectively.
Comparative analyses of the performance of computational models for skin sensitization prediction
Comparing the performance of computational methods for the prediction of skin sensitization is a non-trivial task. Most models are derived from different, often undisclosed or inaccessible data sets, which prohibit the design of an independent, representative and universal benchmark data set. Nevertheless, several studies have been published in recent years that aim to compare the performance and applicability of current in silico models. When considered with the necessary caution, these reports provide relevant insights on the scope and limitations of the individual models.
Teubner et al. (Citation2013), for example, compared the performance of seven in silico models for the prediction of the skin sensitization potential: VEGA, CASE Ultra, TOPKAT, Toxtree, Derek Nexus, TIMES-SS, and the OECD QSAR Toolbox profilers for protein binding, direct peptide depletion activity, and keratinocyte gene expression. A data set of 100 compounds (55 non-sensitizers and 45 sensitizers) meeting a number of conditions was compiled for testing. The compounds were required to have reliable animal or human data on their skin sensitization potential available (i.e. adequate for GHS classification) and a molecular weight of less than 500 Da. Importantly, to avoid overlaps with the (often inaccessible) training data, only compounds that were not part of a high production volume program and had not been reported in scientific publications in the context of skin sensitization were considered. The tested models correctly classified 23% to 100% of all non-sensitizers and 55% to 100% of all sensitizers that were within the AD of the individual models (i.e. 16% to 100% of the test compounds). The mechanistic models obtained slightly higher success rates than purely statistical models. TIMES-SS turned out to be the most accurate model (100% correct classification) but was, however, applicable to just 16% of the tested compounds. One of the main conclusions drawn by the authors was that the tested models identify skin sensitizers with sufficient accuracy only if they bind to skin proteins without transformation or through a well-established transformation route and do not contain any rare structural features, functional groups, or atoms. None of the models for potency prediction yielded a good correlation with the experimentally determined GHS sensitization subcategories. Overall, the authors concluded that the existing models are not sufficiently accurate and broadly applicable for a widespread application in skin sensitization prediction.
Ellison et al. (Citation2010) developed a weight of evidence approach using results from Derek for Windows, Enoch’s SMARTS rules (Enoch, Madden, et al. Citation2008), OECD QSAR Toolbox and CAESAR global models, which we discuss in section “Hybrid in silico models.” For an LLNA data set of 19 sensitizers and 25 non-sensitizers, the individual models yielded accuracies between 57% (CAESAR global models) and 70% (Derek for Windows). Because the testing data was published only recently before the actual tests were conducted, Ellison et al. assumed that there were no overlaps between the training and testing data.
Urbisch et al. (Urbisch, Honarvar, et al. Citation2016) tested the performance of the OECD QSAR Toolbox and TIMES-SS on a data set of 213 and 111 compounds annotated with LLNA and human skin sensitization data, respectively. Base accuracies for the OASIS profiler (71% and 70%) and the OECD profiler (69% and 67%) – both implemented in the OECD QSAR Toolbox – as well as for TIMES-SS (77% and 71%) were reported according to LLNA and human data, respectively. By a combination of the methods with modules or profilers for predicting metabolism and autoxidation, the accuracy of the best models based on the OECD QSAR Toolbox and TIMES-SS reached 84% and 94%, respectively, in predicting LLNA outcomes. Interestingly, the predictivity of these models was lower for human data, with models based on the OECD QSAR Toolbox and TIMES-SS reaching accuracies of up to 82% and 76%, respectively.
Verheyen et al. (Citation2017) evaluated the ability of VEGA, CASE Ultra, Toxtree, Derek Nexus, and the skin sensitization profiler of the OECD QSAR Toolbox to discriminate skin sensitizers from non-sensitizers. A test set of 160 substances (82 sensitizers and 78 non-sensitizers) annotated with binary animal or human data on skin sensitization was compiled from public sources such as the Priority List of Hazardous Substances (published by the Agency for Toxic Substances and Disease Registry; ATSDR Citation2018) and the OECD’s eChemPortal (OECD, eChemPortal). The major findings of this study are in agreement with those of Teubner et al. The models correctly classified between 48% and 78% of the test compounds to which the models were applicable, with rule-based models obtaining better results than the statistical models. Predictions could be obtained for 38% to 100% of the test compounds, with rule-based models having higher coverage rates than statistical models. The authors agreed with Teubner et al. that coverage and predictivity of current computational models are not satisfactory.
Rorije et al. (Citation2013) studied and compared the ability of the h-CLAT and five in silico models (i.e. MultiCASE, Derek Nexus, TIMES-SS, TOPKAT, and the SMARTS rules of Enoch et al.; Enoch, Madden, et al. Citation2008) to predict LLNA outcomes. An (incomplete) data matrix of 1045 compounds annotated with binary human, LLNA, GPMT, in vitro, and/or in chemico data served as the data basis for this analysis. The authors concluded that neither the performance of h-CLAT nor that of any of the in silico models is currently sufficient for standalone risk assessment (even though the in vitro model performed better than any of the in silico models and reached levels of predictivity close to those of GPMT by LLNA and vice versa). The performance indicators of the individual models indicate that a combination of non-animal testing approaches with in silico methods (see section “Computational methods used in combination with non-animal testing results”) could yield models, which predict LLNA outcomes with an accuracy comparable with the predictivity of GPMT by LLNA and vice versa. For the combinations of these methods, however, the authors found lower than expected accuracies, which are likely related to dependencies between the individual in silico tools caused by overlaps of the knowledge bases or training data.
More recently, Fitzpatrick et al. (Citation2018) evaluated the ability of VEGA, Derek Nexus, and TIMES-SS to predict binary LLNA and GPMT outcomes and compared their performance to the correlation between GPMT and LLNA outcomes. On a test set of 1295 unique compounds derived from eChemPortal, the overall accuracies obtained by VEGA, Derek Nexus, and TIMES-SS were 44%, 71%, and 67%. On a smaller test set of 515 unique compounds derived from the NICEATM LLNA data set, the models obtained accuracies between 57% and 61%. For both data sets, accuracies increased when only considering substances that are within the AD of the models but remained significantly lower than LLNA/GPMT predictivity, which was in the range of 80% to 85%. The low accuracy of VEGA was caused by a high sensitivity combined with a low specificity (which is in agreement with previous findings; Rorije et al. Citation2013). The authors detected 83 compounds for which all three models produced wrong predictions. This may be an indication of dependencies and bias shared among the models and is in the line with previous reports (Rorije et al. Citation2013), which found that the integration of several in silico models does not necessarily lead to the increase in accuracy that would be expected if all models were independent of each other.
Computational methods used in combination with non-animal testing results
When used on their own, modern theoretical and experimental AATs do not yet reach the regulatory acceptance requirements for skin sensitization risk assessment (Casati et al. Citation2018). Therefore, organizations such as the OECD encourage the development of integrated approaches to testing and assessment (IATAs), which can be described as human expert-led, non-formalized weight of evidence approaches amalgamating results obtained from different experimental models and theoretical approaches (e.g. OECD Citation2017b, Citation2017c).
IATAs are designed to be flexible and open for interpretation. New data are introduced in the decision process by an iterative procedure. Defined approaches (DAs) to testing and assessment, on the contrary, integrate information following fixed data interpretation procedures (DIP). As such they do not require or allow expert judgment but provide a defined algorithm that draws conclusions from defined input variables (Kleinstreuer et al. Citation2018). Two types of DAs are established in skin sensitization prediction: integrated testing strategies (ITSs) and sequential testing strategies (STSs) (Ezendam et al. Citation2016). Whereas ITSs combine information from multiple sources to reach a conclusion, STSs collect information in a stepwise manner involving interim decisions. Either type of DAs can be integrated into IATAs.
Obviously, the validity of the predictions made by IATAs and DAs depends on the quality of the individual inputs (Leontaridou et al. Citation2017). Alves et al. (Alves, Capuzzi, et al. Citation2018) recently showed that the binary outcomes of some of the assays most commonly used in IATAs (i.e. h-CLAT, DPRA, and KeratinoSensTM) can be predicted with QSAR models with adequate accuracy. Similarly, Wijeyesakere et al. (Citation2018) reported on a rule-based approach that yielded 89% correct predictions of binary DPRA outcomes of 162 substances. Both of these studies show that further improvement of these models could allow the use of calculated assay outcomes as input variables for IATAs and DAs in the future.
Approaches integrating existing data with experimental and/or computational approaches have been reviewed in several recent publications (Rovida et al. Citation2015; Ezendam et al. Citation2016; Jaworska Citation2016; Kleinstreuer et al. Citation2018). Here, we focus on those using computational methods to support data integration or that have been developed with the support of computational approaches.
An example of a computer-assisted IATA for skin sensitization risk assessment is the model developed by Patlewicz et al. (Patlewicz, Kuseva, Kesova, et al. Citation2014). The expert user is guided through a schematic workflow that collects the available experimental information on skin sensitization potential or potency (i.e. results from animal or non-animal experiments), skin irritation, genotoxicity, and physicochemical properties. As part of this process, the expert user is often requested to interpret the information collected as part of the workflow or to generate additional experimental data. Parts of the described workflow have been implemented as a software prototype called IATA-SS. IATA-SS obtained a binary classification accuracy of 74% on a test set of 100 compounds (consisting of 45 sensitizers and 55 non-sensitizers) that was previously compiled by Teubner et al. (Citation2013).
Most DAs reported for skin sensitization prediction are ITSs. One of the earliest types of ITSs are the so-called “2-out-of-3” approaches (and variants thereof), which are based on majority voting. These include the ITS of Urbisch et al. (Urbisch, Honarvar, et al. Citation2016), which integrates results from either the DPRA, OECD QSAR Toolbox, or TIMES-SS with results from LuSens and h-CLAT. Interestingly, binary classification accuracies did not differ substantially for the different combinations of data. For example, the integration of the OECD QSAR Toolbox with LuSens and h-CLAT reproduced human data in 89% of all cases (covering 92 of the 111 compounds) and LLNA data in 91% of all cases (covering 141 of the 213 compounds). The integration of TIMES-SS with LuSens and h-CLAT resulted in better accuracy (up to 100%) with coverages of only 13 to 20 compounds. Recently, “2-out-of-3” approaches integrating results from several non-animal testing methods have been challenged by “2-out-of-2” approaches, for which comparable accuracies were reached (Otsubo et al. Citation2017; Roberts and Patlewicz Citation2018). These findings are in line with the general concern that the added value of majority voting can be limited if the individual assays differ in their performance (Johansson and Gradin Citation2017) or provide redundant data in terms of the biological mechanisms assessed.
ITSs of higher complexity make use of statistical methods or machine learning, often with the aim to also allow a (semi-) quantitative prediction of skin sensitization potencies. For example, Jaworska et al. (Citation2011, Citation2013, Citation2015) developed a Bayesian integrated testing strategy that takes calculated physico-chemical properties related to bioavailability, in silico potency predictions (performed with TIMES-SS) and results from in vitro (KeratinoSens™ and h-CLAT) and in chemico (DPRA) assays as input in a weight of evidence assessment. The latest published version of this model (ITS-3; Jaworska et al. Citation2015) was trained on LLNA data for a diverse set of 147 fragrances, preservatives, dyes, dye precursors, halogenated alkanes, and solvents. The model was able to predict the correct potency category (of four) for 53 of the 60 compounds of a test set. All wrong predictions were caused by misclassifications into a directly neighboring class. A recent study showed for version 2 of this ITS that the TIMES-SS (a commercial product) can be substituted by the structural alerts set implemented in the protein binding for skin sensitization profiler of the OECD QSAR toolbox (which is free software and the structural alerts are related to those covered by TIMES-SS) without a substantial decrease in prediction accuracy (Fitzpatrick and Patlewicz Citation2017).
Luechtefeld et al. (Citation2015) trained dose-informed random forest/hidden Markov classification models on categorical LLNA data compiled for 145 substances (mainly derived from the Jaworska data set; see section “Data sets”). Up to 10 descriptors derived from different in vitro and in chemico assays, as well as descriptors calculated with Dragon and predictions of skin sensitization performed with TIMES-SS, served as input variables. For the best-performing models, the three most important input variables originated from in vitro and in chemico tests. The consideration of TIMES-SS predictions as input variables had no advantage over the use of Dragon descriptors. Accuracies for predicting the correct or neighboring potency category (of the four categories) were around 92% during stratified shuffle split cross-validation. The correct category was assigned to up to 65% of the compounds, depending on the input variables used.
Asturiol et al. (Citation2016) derived classification trees from LLNA data (partly accompanied by human data) collected for 269 substances (using 80% of the data for training and 20% for testing). In vitro (KeratinoSens™ and h-CLAT) and in chemico data (DPRA), as well as molecular descriptors (calculated with Dragon) and in silico predictions (performed with Toxtree, OECD QSAR Toolbox, Derek Nexus, VEGA, TIMES-SS, and ADMET Predictor) were used as inputs. Interestingly, in contrast to the work of Luechtefeld et al., the prediction of protein binding by TIMES-SS turned out to be the most discriminating node for both of the two best-performing decision tree models, and neither of these models made use of in vitro or in chemico data. The best-performing binary classification model obtained an accuracy of 83% on the test set.
Zang et al. (Citation2017) developed several classification models for the prediction of three categories of skin sensitization potency based on 94 compounds annotated with LLNA or 63 compounds annotated with human data. The models were derived using various machine learning algorithms (i.e. classification and regression tree, linear discriminant analysis, logistic regression, and support vector machine) following either a one-tiered approach (directly assigning one of three potency classes to a compound) or a two-tiered approach (first dividing compounds into non-sensitizers and sensitizers and then differentiating between weak and strong sensitizers). Six physicochemical properties (i.e. logP, water solubility, vapor pressure, melting point, boiling point, and molecular weight) and the results from three non-animal testing methods (i.e. DPRA, h-CLAT and KeratinoSens™) served as input for model building. The best-performing models resulted from a two-tiered SVM approach that took all input variables into account. These models obtained accuracies of 88% and 81% for the prediction of LLNA and human outcomes on two test sets of 63 and 24 compounds, respectively.
Strickland et al. (Citation2017) used different subsets of the same types of input as Zang et al. (i.e. the three non-animal testing methods and six physicochemical properties) in combination with results obtained from the four protein binding profilers implemented in the OECD QSAR Toolbox to develop models based on logistic regression and a SVM for the prediction of the human skin sensitization potential. The models were trained on a set of 72 compounds for which results from DPRA, KeratinoSens™, h-CLAT, and LLNA, as well as human data are available. A random forest model was used for feature selection. Cysteine depletion measured by the DPRA was ranked as the most important feature, followed by other non-animal testing outcomes and the predictions from the OECD QSAR Toolbox. Of the six physicochemical properties, only the use of logP resulted in better performance of the linear regression and SVM model. The best models obtained an accuracy of 92% on a test set of 24 compounds.
Natsch et al. (Citation2015) developed two models based on linear regression for the prediction of pEC3 values based on 244 compounds annotated with pEC3 values and tested on 68 compounds. One of the models used the reaction rate with peptides, Nrf2-induction, and cytotoxicity in KeratinoSensTM as the most distinguishing input variables, leading to an adjusted r2 of 0.62. Interestingly, this model performed better for weak and moderate sensitizers than for strong or extreme sensitizers. The other model (a domain-based model) first groups compounds by their reaction mechanism as predicted by TIMES-SS and as measured by experimental adduct formation, and subsequently applies local regression for the different reaction domains. This model led to the better prediction for well-populated domains but resulted in poor predictivity for less populated ones. Most recently, Natsch et al. (Citation2018) applied the domain-based model within an IATA to 22 existing and 7 new fragrance substances to derive EC3 values. Within that application, the local model for aldehydes was slightly modified compared with the original publication. For 15 compounds with congruent human and LLNA data, an R2 of 0.67 was reported for potency prediction. For each prediction on a substance of interest, uncertainty was assessed by applying the model to a structurally similar molecule with available LLNA data (from a database of more than 400 compounds) and comparing predicted and experimental potency. The no expected sensitization induction level (NESIL) was thereby derived from predicted EC3 values.
Hirota et al. (Citation2017) utilized artificial neural networks for the prediction of LLNA EC3 values (binned into four potency classes) by integrating results obtained from the h-CLAT, DPRA, and KeratinoSensTM with predictions from Toxtree and TIMES. Several models taking into account different combinations of input variables were trained on 134 and tested on 28 compounds annotated with LLNA data. Models taking into account predictions from TIMES (accuracy 71%) or Toxtree alerts (accuracy 64%) obtained higher accuracies than the two models based solely on experimental data (43% and 50%).
ITSs may also be used to address the issue of a limited predictivity of LLNA data for human skin sensitization. For example, Alves et al. (Alves, Capuzzi, et al. Citation2016) combined LLNA outcomes with QSAR results to predict the skin sensitization potential in humans. Several binary QSAR models using radial basis function interpolation and self-consistent regression were developed for this purpose using a data set of 109 compounds annotated with human and LLNA data. A consensus model combining 10 of the best-performing QSAR models in each fold resulted in the correct classification of 71% of all compounds into skin sensitizers and non-sensitizers during five-fold cross-validation. In comparison, the LLNA obtained a CCR of only 63% on this data. When combining QSAR predictions with LLNA outcomes by only considering compounds for which both approaches produced concordant results, the CCR improved to 82% (five-fold cross-validation), at the cost of the applicability of the approach, which was reduced to 52% of the tested compounds.
Most DAs for skin sensitization prediction can be identified as ITS, but STSs are also in existence. For example, van der Veen et al. (Citation2014) proposed an STS for the qualitative prediction of human skin sensitization potential. The three-tiered, independent Bayesian approach integrates results from in silico tools (MultiCASE, CAESAR, Derek Nexus, and OECD QSAR Toolbox) with those from in chemico and in vitro assays (peptide binding, gene signature, KeratinoSens™, and h-CLAT). The model was developed based on a set of 41 compounds annotated with human and LLNA data and designed to reflect various potency classes. In addition, compounds known to cause false-positive or false-negative results in LLNA (compared with human data) were included in the data set. Depending on the results of each tier, results from one to three non-animal testing methods were requested by the approach. Within the first tier, QSAR models were applied and, only if they resulted in an equivocal call, peptide binding was also tested. Depending on the result of this tier, either Keratinosens™ or gene signature was tested in the second tier of the approach. The third tier, comprising h-CLAT results, was only performed when the first and second tier were not in agreement. These interim decision steps not only aim for a reduction of experiments but to account for the predictive performance of the different included methods. For human data, the three-tiered approach obtained classification accuracies of 92% and higher. In contrast, LLNA data only predicted 78% of the human data correctly.
A further example of an STS is a binary classification model for skin sensitization potential based on a decision tree that integrates predictions from Derek Nexus with results from a maximum of two of the five in chemico and in vitro assays (i.e. DPRA, KeratinoSens™, LuSens, h-CLAT, U-SENS™) (Macmillan et al. Citation2016). As part of this STS, for any compound of interest that is within the AD of the assays, a two-tiered majority voting approach is applied that takes into account predictions from Derek Nexus and one or two assays. If the outcome of the first assay is in accordance with the predictions from Derek Nexus, no additional assay is used for majority voting to reduce the number of required experiments. In the case of discordant results, however, the second assay decides the overall result. If a substance is outside of the AD of both assays, only Derek Nexus is used for prediction. The STS was tested with 20 different combinations of in vitro and in chemico assays as input variables on a data set of 213 compounds annotated with LLNA and, where available, also human data. The models reached a classification accuracy of ∼80% to 90%, depending on the assay used (median accuracy 85%). The authors note that the substances evaluated in this study are not independent of the models since 20% of them have been part of the Derek Nexus training set and an unknown portion of them is assumed to be included in the training data of the assays. Nevertheless, the authors state that removal of Derek Nexus training data from the test set did not significantly alter the final results.
Kleinstreuer et al. (Citation2018) evaluated six DAs for the prediction of the skin sensitization potential and potency of compounds based on the Cosmetics Europe data set (see section “Data sets”). The best models reached binary classification accuracies of up to 83 and 80% for LLNA and human data, respectively (in comparison, prediction of human skin sensitization based on LLNA data was correct for only 68% of all tested compounds).
Outlook and conclusions
Today, a large number of in silico models for the prediction of the skin sensitization potential and potency of substances are in existence. The models are based on a variety of approaches, each with its own advantages and disadvantages. Currently, there is no single model or algorithm in existence that consistently outperforms all others.
Rule-based approaches, read-across, and linear statistical models score with good interpretability, and the latter may also provide new mechanistic insights. Machine learning approaches are generally more difficult to interpret or even have the character of a black box, but they are generally most suitable for modeling complex nonlinear relationships such as those observed for most toxicity endpoints, including skin sensitization. Two major strategies that have been followed successfully to increase the prediction accuracy of models are the combination of different computational methods (hybrid models) and the amalgamation of theoretical methods and experimental data (DAs and IATAs). Both strategies have the potential to maximize accuracy and applicability by combining information from different sources. When working with these approaches and interpreting predictions, it is, however, important to carefully consider possible correlations between sources of information.
Molecular descriptors play a crucial role in the performance, applicability, and interpretability of models. Ideally, models should be based on small sets of physically meaningful descriptors to enable the interpretation of models and minimize the risk of overfitting. In the context of machine learning, in particular, feature selection algorithms are commonly utilized to select important features from large sets of descriptors. This can yield better performing models but generally at the cost of interpretability. Among models for the prediction of skin sensitization, a prevalence of descriptors associated with the toxicological endpoint on a mechanistic level is observed. These descriptors include structural fragments or alerts that correspond to the five established reaction domains, as well as descriptors linked to chemical reactivity (e.g. molecular orbital energies) or skin penetration (e.g. logP or molecular volume).
Whereas the existing molecular descriptors and modeling techniques have come of age the limited availability of reliable and relevant data remains a bottleneck for the development of more accurate and widely applicable in silico predictors of skin sensitization, particularly of potency.
Human data on skin sensitization remain extremely rare, are mostly NOAELs that are difficult to interpret and vary in quality. LLNA outcomes have been reported for a total of more than 1000 substances. These are mostly binary data; potency information is available in the public domain for only a subset of a few hundred substances. A recent study has shown that LLNA data populate regions in chemical space not covered by any other type of skin sensitization data (Alves, Capuzzi, et al. Citation2018). For these and other reasons, most in silico models are derived from collections of LLNA data. Although this increases the AD of models, it also caps predictivity of human health to that of animal experiments, which themselves are clearly limited (Alves, Capuzzi, et al. Citation2018; Hoffmann et al. Citation2018). High-quality data sets on skin sensitization, such as those compiled by Hoffmann et al. (Citation2018) or Natsch et al. (Citation2013), are available but small in size. They generally include EC3 values accompanied by information on non-animal testing results and human evidence. These data sets are not sufficiently large for model training but can be of high value as benchmark data sets for theoretical and experimental approaches alike.
Much of the measured data on skin sensitization is proprietary company data. Because of the pressing problem of a lack of data required to advance theoretical and experimental AATs alike, new avenues are being explored that could allow the distribution of proprietary data for model development without contravening the interests of their owners. These strategies include the use of machine learning algorithms on encrypted data (Luechtefeld, Rowlands, et al. Citation2018), as well as the allocation of data by so-called honest brokers. The latter has already resulted, for example, in the contribution of data from nine Lhasa member organizations to the evaluation of Derek Nexus (Chilton et al. Citation2018).
For computational models to be acceptable for use for regulatory purposes, they should comply with the guidelines for linear (Q)SAR models (OECD Citation2014b). Ideally, the data used for model building and testing should be fully disclosed to ensure reproducibility and allow a detailed understanding of the AD, the verification of data, and the testing of models with external data while excluding overlaps. In recent years, significant efforts have been made to develop in silico models for the prediction of skin sensitization that satisfy the requirements for use in a regulatory environment. A growing acceptance of these methods (together with other AATs) in risk assessment is observed. For example, in April 2018 the EPA released a draft for the acceptance of AATs for predicting skin sensitization, reasoning that substantial scientific evidence supports the use of these new methodologies (U.S. EPA Citation2018). In addition, the ECHA has recently promoted the use of AATs in REACH applications (ECHA Citation2017).
A major determinant for the acceptance of AATs for regulatory purposes is their validation with robust protocols complying with defined international standards. However, a substantial number of models, even of those published recently, are still not properly validated. All too often, only evaluation results from cross-validation are reported, or, in the worst case, from predictions on the training data.
Independent studies comparing the performance of models are an important cornerstone on the way to establishing in silico models and other AATs as a major pillar of risk assessment, but these studies are hindered by the scarcity of data available for testing and the often undisclosed training data. The development of well-characterized, high-quality data sets is therefore essential for the robust, comparative evaluation of theoretical and experimental models alike.
Just like any modern AAT, in silico models are not yet sufficiently reliable and broadly applicable to be used as a single prediction method for risk assessment. They are also not capable of predicting skin sensitization caused by mixtures, and few approaches are applicable to metals. However, despite all challenges, several studies have shown that in silico models have the capacity to outperform animal testing experiments, which have been accepted for regulatory purposes for decades. With the increasing availability of experimental data and advances in modeling techniques, computational methods and other AATs are expected to reach levels of accuracy and applicability that will make them a primary tool for risk assessment in the foreseeable future. In particular, integrated approaches combining in vivo, in vitro, in chemico, and in silico data hold the promise to evolve into powerful models for the prediction of the skin sensitization potential and potency of substances in humans with previously unmet accuracy and reach.
Declaration of interest
Beiersdorf AG is a personal care company based in Hamburg, Germany. Beiersdorf’s consumer business focusses on skin care products with brands such as Nivea and Eucerin. The company has a strong background in the development of alternatives to animal testing for about 30 years, being involved in the development of e.g. the in vitro 3T3 NRU Photoxicity test and in KeratinoSensTM ring studies. Within Cosmetics Europe, the European trade association of cosmetics and personal care industry, Beiersdorf is contributing to cooperative efforts to foster the development and implementation of alternatives to animal testing.
HITeC e.V. is the Research and Technology Transfer Center of the Department of Informatics at the University of Hamburg. HITeC is a registered, nonprofit association, which is supported by members of the Department of Informatics at the University of Hamburg. The association is affiliated with the University of Hamburg.
AW is funded by Beiersdorf AG through HITeC e.V. and JKi is supported by the Bergen Research Foundation (BFS) [BFS2017TMT01]. BFS gives grants toward research and research supporting activities at the University of Bergen (UiB) and Haukeland University Hospital (HUS), and other Norwegian research institutions that cooperate with institutions in Bergen. The foundation also gives grants to support research at UiB and HUS at the interface between basic research and clinical research.
Neither JKü, AW, nor JKi have participated in legal proceedings related to the contents of the paper. The authors have sole responsibility for the writing and content of the paper.
Acknowledgments
The authors thank Andreas Schepky from Beiersdorf AG Hamburg, Germany, and Christina de Bruyn Kops and Neann Mathai, both from the Center of Bioinformatics (ZBH) of the University of Hamburg, Germany, for discussion and proofreading of the manuscript. The authors also thank the three anonymous reviewers for the valuable feedback received, which helped the authors to improve this manuscript.
References
- Adler S, Basketter D, Creton S, Pelkonen O, van Benthem J, Zuang V, Andersen KE, Angers-Loustau A, Aptula A, Bal-Price A. 2011. Alternative (non-animal) methods for cosmetics testing: current status and future prospects-2010. Arch Toxicol. 85:367–485.
- Alves VM, Capuzzi SJ, Braga RC, Borba JVB, Silva AC, Luechtefeld T, Hartung T, Andrade CH, Muratov EN, Tropsha A. 2018. A perspective and a new integrated computational strategy for skin sensitization assessment. ACS Sustainable Chem Eng. 6:2845–2859.
- Alves VM, Capuzzi SJ, Muratov E, Braga RC, Thornton T, Fourches D, Strickland J, Kleinstreuer N, Andrade CH, Tropsha A. 2016. QSAR models of human data can enrich or replace LLNA testing for human skin sensitization. Green Chem. 18:6501–6515.
- Alves VM, Golbraikh A, Capuzzi SJ, Liu K, Lam WI, Korn DR, Pozefsky D, Andrade CH, Muratov EN, Tropsha A. 2018. Multi-Descriptor Read Across (MuDRA): a simple and transparent approach for developing accurate quantitative structure-activity relationship models. J Chem Inf Model. 58:1214–1223.
- Alves V, Muratov E, Capuzzi S, Politi R, Low Y, Braga R, Zakharov AV, Sedykh A, Mokshyna E, Farag S, et al. 2016. Alarms about structural alerts. Green Chem. 18:4348–4360.
- Alves VM, Muratov E, Fourches D, Strickland J, Kleinstreuer N, Andrade CH, Tropsha A. 2015a. Predicting chemically-induced skin reactions. Part II: QSAR models of skin permeability and the relationships between skin permeability and skin sensitization. Toxicol Appl Pharmacol. 284:273–280.
- Alves VM, Muratov E, Fourches D, Strickland J, Kleinstreuer N, Andrade CH, Tropsha A. 2015b. Predicting chemically-induced skin reactions. Part I: QSAR models of skin sensitization and their application to identify potentially hazardous compounds. Toxicol Appl Pharmacol. 284:262–272.
- Anderson SE, Siegel PD, Meade BJ. 2011. The LLNA: a brief review of recent advances and limitations. J Allergy. 2011:424203.
- Api AM, Parakhia R, O’Brien D, Basketter DA. 2017. Fragrances categorized according to relative human skin sensitization potency. Dermatitis. 28:299–307.
- Aptula AO, Roberts DW. 2006. Mechanistic applicability domains for nonanimal-based prediction of toxicological end points: general principles and application to reactive toxicity. Chem Res Toxicol. 19:1097–1105.
- Asturiol D, Casati S, Worth A. 2016. Consensus of classification trees for skin sensitisation hazard prediction. Toxicol In Vitro. 36:197–209.
- ATSDR. 2018. Substance priority list. [accessed 2018 Aug 14]. https://www.atsdr.cdc.gov/SPL/.
- Barratt MD, Basketter DA, Chamberlain M, Payne MP, Admans GD, Langowski JJ. 1994. Development of an expert system rulebase for identifying contact allergens. Toxicol In Vitro. 8:837–839.
- Basketter DA, Alépée N, Ashikaga T, Barroso J, Gilmour N, Goebel C, Hibatallah J, Hoffmann S, Kern P, Martinozzi-Teissier S, et al. 2014. Categorization of chemicals according to their relative human skin sensitizing potency. Dermatitis. 25:11–21.
- Basketter D, Clewell H, Kimber I, Rossi A, Blaauboer B, Burrier R, Daneshian M, Eskes C, Goldberg A, Hasiwa N, et al. 2012. A roadmap for the development of alternative (non-animal) methods for skin sensitization testing. In: A roadmap for the development of alternative (non-animal) methods for systemic toxicity testing. ALTEX. 29:3–32.
- Benfenati E, Manganaro A, Gini G. 2013. VEGA-QSAR: AI inside a platform for predictive toxicology. In: Baldoni M, Chesani F, Mello P, Montali M, editors. 2013. Proceedings of the workshop "Popularize Artificial Intelligence 2013"; December 5 2013; Turin, Italy. CEUR Workshop Proceedings Vol 1107.
- Benigni R, Bossa C, Tcheremenskaia O. 2016. A data-based exploration of the adverse outcome pathway for skin sensitization points to the necessary requirements for its prediction with alternative methods. Regul Toxicol Pharmacol. 78:45–52.
- Bergers LIJC, Reijnders CMA, van den Broek LJ, Spiekstra SW, de Gruijl TD, Weijers EM, Gibbs S. 2016. Immune-competent human skin disease models. Drug Discov Today. 21:1479–1488.
- BIOVIA. QSAR, ADMET and predictive toxicology. [accessed 2018 Aug 22]. http://accelrys.com/products/collaborative-science/biovia-discovery-studio/qsar-admet-and-predictive-toxicology.html.
- BIOVIA. 2017a. BIOVIA toxicity prediction model – skin sensitiser vs non sensitiser. (Q)SAR Model Reporting Format Database. [accessed 2018 Jan 16]. https://qsardb.jrc.ec.europa.eu/qmrf/protocol/Q17-46-0042/document?media=application%2Fpdf.
- BIOVIA. 2017b. BIOVIA toxicity prediction model – weak vs strong sensitiser. (Q)SAR Model Reporting Format Database. [accessed 2018 Jan 16]. https://qsardb.jrc.ec.europa.eu/qmrf/protocol/Q17-46-0043/document?media=application%2Fpdf.
- Braga RC, Alves VM, Muratov EN, Strickland J, Kleinstreuer N, Trospsha A, Andrade CH. 2017. Pred-Skin: a fast and reliable web application to assess skin sensitization effect of chemicals. J Chem Inf Model. 57:1013–1017.
- CAESAR. CAESAR project. [accessed 2018 Feb 6]. http://www.caesar-project.eu.
- CAESAR. Skin sensitization model. [accessed 2018 Jan 25]. http://www.caesar-project.eu/index.php?page=results§ion=endpoint&ne=2.
- Canipa SJ, Chilton ML, Hemingway R, Macmillan DS, Myden A, Plante JP, Tennant RE, Vessey JD, Steger-Hartmann T, Gould J, et al. 2017. A quantitative in silico model for predicting skin sensitization using a nearest neighbours approach within expert-derived structure-activity alert spaces. J Appl Toxicol. 37:985–995.
- Casati S, Aschberger K, Barroso J, Casey W, Delgado I, Kim TS, Kleinstreuer N, Kojima H, Lee JK, Lowit A, et al. 2018. Standardisation of defined approaches for skin sensitisation testing to support regulatory use and international adoption: position of the International Cooperation on Alternative Test Methods. Arch Toxicol. 92:611–617.
- Chakravarti SK, Saiakhov RD, Klopman G. 2012. Optimizing predictive performance of CASE Ultra expert system models using the applicability domains of individual toxicity alerts. J Chem Inf Model. 52:2609–2618.
- Chaudhry Q, Piclin N, Cotterill J, Pintore M, Price NR, Chrétien JR, Roncaglioni A. 2010. Global QSAR models of skin sensitisers for regulatory purposes. Chem Cent J. 4(Suppl 1):S5.
- Chembench. Accelerating chemical genomics research. [accessed 2018 July 25]. https://chembench.mml.unc.edu/mudra/.
- Cherkasov A, Muratov EN, Fourches D, Varnek A, Baskin II, Cronin M, Dearden J, Gramatica P, Martin YC, Todeschini R, et al. 2014. QSAR modeling: where have you been? Where are you going to? J Med Chem. 57:4977–5010.
- Chilton ML, Macmillan DS, Steger-Hartmann T, Hillegass J, Bellion P, Vuorinen A, Etter S, Smith BPC, White A, Sterchele P, et al. 2018. Making reliable negative predictions of human skin sensitisation using an in silico fragmentation approach. Regul Toxicol Pharmacol. 95:227–235.
- Chipinda I, Hettick JM, Siegel PD. 2011. Haptenation: chemical reactivity and protein binding. J Allergy. 2011:839682.
- CORAL. Free software for QSAR and nanoQSAR. [accessed 2018 Aug 13]. www.insilico.eu/coral.
- Cottrez F, Boitel E, Auriault C, Aeby P, Groux H. 2015. Genes specifically modulated in sensitized skins allow the detection of sensitizers in a reconstructed human skin model. Development of the SENS-IS assay. Toxicol In Vitro. 29:787–802.
- Cottrez F, Boitel E, Ourlin JC, Peiffer JL, Fabre I, Henaoui IS, Mari B, Vallauri A, Paquet A, Barbry P, et al. 2016. SENS-IS, a 3D reconstituted epidermis based model for quantifying chemical sensitization potency: reproducibility and predictivity results from an inter-laboratory study. Toxicol In Vitro. 32:248–260.
- Dearden JC, Hewitt M, Roberts DW, Enoch SJ, Rowe PH, Przybylak KR, Vaughan-Williams GD, Smith ML, Pillai GG, Katritzky AR. 2015. Mechanism-Based QSAR Modeling of Skin Sensitization. Chem Res Toxicol. 28:1975–1986.
- Dimitrov SD, Low LK, Patlewicz GY, Kern PS, Dimitrova GD, Comber MHI, Phillips RD, Niemela J, Bailey PT, Mekenyan OG. 2005. Skin sensitization: modeling based on skin metabolism simulation and formation of protein conjugates. Int J Toxicol. 24:189–204.
- Dumont C, Barroso J, Matys I, Worth A, Casati S. 2016. Analysis of the Local Lymph Node Assay (LLNA) variability for assessing the prediction of skin sensitisation potential and potency of chemicals with non-animal approaches. Toxicol In Vitro. 34:220–228.
- ECHA. Homepage. [accessed 2018 July 17]. https://echa.europa.eu/en.
- ECHA. 2017. The use of alternatives to testing on animals for the REACH Regulation. [accessed 2018 Aug 27]. https://echa.europa.eu/documents/10162/13639/alternatives_test_animals_2017_en.pdf.
- Ellison CM, Madden JC, Judson P, Cronin MTD. 2010. Using in silico tools in a weight of evidence approach to aid toxicological assessment. Mol Inform. 29:97–110.
- Enoch SJ, Cronin MTD, Schultz TW, Madden JC. 2008. Quantitative and mechanistic read across for predicting the skin sensitization potential of alkenes acting via Michael addition. Chem Res Toxicol. 21:513–520.
- Enoch SJ, Ellison CM, Schultz TW, Cronin MTD. 2011. A review of the electrophilic reaction chemistry involved in covalent protein binding relevant to toxicity. Crit Rev Toxicol. 41:783–802.
- Enoch SJ, Madden JC, Cronin MTD. 2008. Identification of mechanisms of toxic action for skin sensitisation using a SMARTS pattern based approach. SAR QSAR Environ Res. 19:555–578.
- Enoch SJ, Roberts DW. 2013. Predicting skin sensitization potency for Michael acceptors in the LLNA using quantum mechanics calculations. Chem Res Toxicol. 26:767–774.
- Enslein K, Gombar VK, Blake BW, Maibach HI, Hostynek JJ, Sigman CC, Bagheri D. 1997. A quantitative structure-toxicity relationships model for the dermal sensitization guinea pig maximization assay. Food Chem Toxicol. 35:1091–1098.
- EURL ECVAM. KeratinoSens assay for the testing of skin sensitizers [accessed 2018 Aug 29]. https://tsar.jrc.ec.europa.eu/test-method/tm2010-03.
- EUR-Lex. 2009. Regulation (EC) No 1223/2009 of the European Parliament and of the Council of 30 November 2009 on cosmetic products. [accessed 2018 Jul 4]. https://eur-lex.europa.eu/eli/reg/2009/1223/oj.
- EURL ECVAM. LuSens assay. [accessed 2018 Mar 28]. https://tsar.jrc.ec.europa.eu/test-method/tm2011-10.
- Ezendam J, Braakhuis HM, Vandebriel RJ. 2016. State of the art in non-animal approaches for skin sensitization testing: from individual test methods towards testing strategies. Arch Toxicol. 90:2861–2883.
- Fitzpatrick JM, Patlewicz G. 2017. Application of IATA – a case study in evaluating the global and local performance of a Bayesian network model for skin sensitization. SAR QSAR Environ Res. 28:297–310.
- Fitzpatrick JM, Roberts DW, Patlewicz G. 2017a. What determines skin sensitization potency: myths, maybes and realities. The 500 molecular weight cut-off: an updated analysis. J Appl Toxicol. 37:105–116.
- Fitzpatrick JM, Roberts DW, Patlewicz G. 2017b. Is skin penetration a determining factor in skin sensitization potential and potency? Refuting the notion of a LogKow threshold for skin sensitization. J Appl Toxicol. 37:117–127.
- Fitzpatrick JM, Roberts DW, Patlewicz G. 2018. An evaluation of selected (Q)SARs/expert systems for predicting skin sensitisation potential. SAR QSAR Environ Res. 29:439–468.
- Garner LA. 2004. Contact dermatitis to metals. Dermatol Ther. 17:321–327.
- Gerberick GF, Ryan CA, Kern PS, Schlatter H, Dearman RJ, Kimber I, Patlewicz GY, Basketter DA. 2005. Compilation of historical local lymph node data for evaluation of skin sensitization alternative methods. Dermatitis. 16:157–202.
- Gerner I, Barratt MD, Zinke S, Schlegel K, Schlede E. 2004. Development and prevalidation of a list of structure-activity relationship rules to be used in expert systems for prediction of the skin-sensitising properties of chemicals. Altern Lab Anim. 32:487–509.
- Gleeson MP, Modi S, Bender A, Robinson RLM, Kirchmair J, Promkatkaew M, Hannongbua S, Glen RC. 2012. The challenges involved in modeling toxicity data in silico: a review. Curr Pharm Des. 18:1266–1291.
- Goebel C, Aeby P, Ade N, Alépée N, Aptula A, Araki D, Dufour E, Gilmour N, Hibatallah J, Keller D, et al. 2012. Guiding principles for the implementation of non-animal safety assessment approaches for cosmetics: skin sensitisation. Regul Toxicol Pharmacol. 63:40–52.
- Goebel C, Diepgen TL, Blömeke B, Gaspari AA, Schnuch A, Fuchs A, Schlotmann K, Krasteva M, Kimber I. 2018. Skin sensitization quantitative risk assessment for occupational exposure of hairdressers to hair dye ingredients. Regul Toxicol Pharmacol. 95:124–132.
- Goebel C, Kosemund-Meynen K, Gargano EM, Politano V, von Bölcshazy G, Zupko K, Jaiswal N, Zhang J, Martin S, Neumann D, Rothe H. 2017. Non-animal skin sensitization safety assessments for cosmetic ingredients – What is possible today? Curr Opin Toxicol. 5:46–54.
- Graham C, Gealy R, Macina OT, Karol MH, Rosenkranz HS. 1996. QSAR for allergic contact dermatitis. Quant Struct-Act Relat. 15:224–229.
- Hartung T. 2013. Look back in anger – what clinical studies tell us about preclinical work. ALTEX. 30:275–291.
- Hartung T. 2017. Opinion versus evidence for the need to move away from animal testing. ALTEX. 34:193–200.
- Helgee EA, Carlsson L, Boyer S, Norinder U. 2010. Evaluation of quantitative structure-activity relationship modeling strategies: local and global models. J Chem Inf Model. 50:677–689.
- Hennen J, Aeby P, Goebel C, Schettgen T, Oberli A, Kalmes M, Blömeke B. 2011. Cross talk between keratinocytes and dendritic cells: impact on the prediction of sensitization. Toxicol Sci. 123:501–510.
- Heratizadeh A, Werfel T, Schubert S, Geier J. IVDK. 2018. Contact sensitization in dental technicians with occupational contact dermatitis. Data of the Information Network of Departments of Dermatology (IVDK) 2001-2015. Contact Dermatitis. 78:266–273.
- Hirota M, Ashikaga T, Kouzuki H. 2017. Development of an artificial neural network model for risk assessment of skin sensitization using human cell line activation test, direct peptide reactivity assay, KeratinoSensTM and in silico structure alert parameter. J Appl Toxicol. 38:514–526.
- Hoffmann S. 2015. LLNA variability: an essential ingredient for a comprehensive assessment of non-animal skin sensitization test methods and strategies. ALTEX. 32:379–383.
- Hoffmann S, Kleinstreuer N, Alépée N, Allen D, Api AM, Ashikaga T, Clouet E, Cluzel M, Desprez B, Gellatly N, et al. 2018. Non-animal methods to predict skin sensitization (I): the Cosmetics Europe database. Crit Rev Toxicol. 48:344–358.
- ICCVAM. 2011. Test method evaluation report: usefulness and Limitations of the Murine Local Lymph Node Assay for potency categorization of chemicals causing allergic contact dermatitis in humans. [accessed 2018 Apr 16]. https://ntp.niehs.nih.gov/iccvam/docs/immunotox_docs/llna-pot/tmer.pdf.
- ICCVAM. 2013. NICEATM Murine Local Lymph Node Assay (LLNA) Database. [accessed 2017 Nov 24]. https://ntp.niehs.nih.gov/pubhealth/evalatm/test-method-evaluations/immunotoxicity/nonanimal/index.html#NICEATM-Murine-Local-Lymph-Node-Assay-LLNA-Database.
- Jaworska J. 2016. Integrated testing strategies for skin sensitization hazard and potency Assessment—State of the Art and Challenges. Cosmetics. 3:16.
- Jaworska J, Dancik Y, Kern P, Gerberick F, Natsch A. 2013. Bayesian integrated testing strategy to assess skin sensitization potency: from theory to practice. J Appl Toxicol. 33:1353–1364.
- Jaworska J, Harol A, Kern PS, Gerberick GF. 2011. Integrating non-animal test information into an adaptive testing strategy – skin sensitization proof of concept case. ALTEX. 28:211–225.
- Jaworska JS, Natsch A, Ryan C, Strickland J, Ashikaga T, Miyazawa M. 2015. Bayesian integrated testing strategy (ITS) for skin sensitization potency assessment: a decision support system for quantitative weight of evidence and adaptive testing strategy. Arch Toxicol. 89:2355–2383.
- Johansson H, Gradin R, Forreryd A, Agemark M, Zeller K, Johansson A, Larne O, van Vliet E, Borrebaeck C, Lindstedt M. 2017. Evaluation of the GARD assay in a blind Cosmetics Europe study. ALTEX. 34:515–523.
- Johansson H, Albrekt AS, Borrebaeck CA, Lindstedt M. 2013. The GARD assay for assessment of chemical skin sensitizers. Toxicol In Vitro. 27:1163–1169.
- Johansson H, Gradin R. 2017. Skin sensitization: challenging the conventional thinking – a case against 2 out of 3 as integrated testing strategy. Toxicol Sci. 159:3–5.
- Johansson H, Lindstedt M. 2014. Prediction of skin sensitizers using alternative methods to animal experimentation. Basic Clin Pharmacol Toxicol. 115:110–117.
- Johansson H, Lindstedt M, Albrekt A-S, Borrebaeck CAK. 2011. A genomic biomarker signature can predict skin sensitizers using a cell-based in vitro alternative to animal tests. BMC Genomics 12:399.
- Johansson H, Rydnert F, Kühnl J, Schepky A, Borrebaeck CAK, Lindstedt M. 2014. Genomic allergen rapid detection in-house validation–a proof of concept. Toxicol Sci. 139:362–370.
- Karlberg AT, Bergström MA, Börje A, Luthman K, Nilsson JLG. 2008. Allergic contact dermatitis-formation, structural requirements, and reactivity of skin sensitizers. Chem Res Toxicol. 21:53–69.
- Kazius J, McGuire R, Bursi R. 2005. Derivation and validation of toxicophores for mutagenicity prediction. J Med Chem. 48:312–320.
- Kern PS, Gerberick GF, Ryan CA, Kimber I, Aptula A, Basketter DA. 2010. Local lymph node data for the evaluation of skin sensitization alternatives: a second compilation. Dermatitis. 21:8–32.
- Kimber I, Basketter DA, Gerberick GF, Ryan CA, Dearman RJ. 2011. Chemical allergy: translating biology into hazard characterization. Toxicol Sci. 120Suppl 1:S238–S268.
- Kimber I, Frank Gerberick G, Basketter DA. 2017. Quantitative risk assessment for skin sensitization: success or failure? Regul Toxicol Pharmacol. 83:104–108.
- Kleinstreuer NC, Hoffmann S, Alépée N, Allen D, Ashikaga T, Casey W, Clouet E, Cluzel M, Desprez B, Gellatly N, et al. 2018. Non-animal methods to predict skin sensitization (II): an assessment of defined approaches *. Crit Rev Toxicol. 48:359–374.
- Klopman G. 1992. MULTICASE 1. A hierarchical computer automated structure evaluation program. Quant Struct-Act Relat. 11:176–184.
- Kostal J, Voutchkova-Kostal A. 2016. CADRE-SS, an in silico tool for predicting skin sensitization potential based on modeling of molecular interactions. Chem Res Toxicol. 29:58–64.
- LabMol. Pred-Skin 2.0. [accessed 2018 Aug 22]. http://labmol.com.br/predskin/.
- Langton K, Patlewicz GY, Long A, Marchant CA, Basketter DA. 2006. Structure-activity relationships for skin sensitization: recent improvements to Derek for Windows. Contact Dermatitis. 55:342–347.
- Leontaridou M, Gabbert S, Van Ierland EC, Worth AP, Landsiedel R. 2016. Evaluation of non-animal methods for assessing skin sensitisation hazard: a Bayesian Value-of-Information analysis. Altern Lab Anim. 44:255–269.
- Leontaridou M, Urbisch D, Kolle SN, Ott K, Mulliner DS, Gabbert S, Landsiedel R. 2017. The borderline range of toxicological methods: quantification and implications for evaluating precision. ALTEX. 34:525–538.
- Lhasa Limited. Derek Nexus. [accessed 2018 Jan 26]. https://www.lhasalimited.org/products/derek-nexus.htm.
- Luechtefeld T, Maertens A, McKim JM, Hartung T, Kleensang A, Sá-Rocha V. 2015. Probabilistic hazard assessment for skin sensitization potency by dose-response modeling using feature elimination instead of quantitative structure-activity relationships. J Appl Toxicol. 35:1361–1371.
- Luechtefeld T, Maertens A, Russo DP, Rovida C, Zhu H, Hartung T. 2016. Analysis of publically available skin sensitization data from REACH registrations 2008-2014. ALTEX. 33:135–148.
- Luechtefeld T, Marsh D, Rowlands C, Hartung T. 2018. Machine learning of toxicological big data enables read-across structure activity relationships (RASAR) outperforming animal test reproducibility. Toxicol Sci. 165:198–212.
- Luechtefeld T, Rowlands C, Hartung T. 2018. Big-data and machine learning to revamp computational toxicology and its use in risk assessment. Toxicol Res. 7:732–744.
- Lu J, Zheng M, Wang Y, Shen Q, Luo X, Jiang H, Chen K. 2011. Fragment-based prediction of skin sensitization using recursive partitioning. J Comput Aided Mol Des. 25:885–893.
- Lushniak BD. 2004. Occupational contact dermatitis. Dermatol Ther. 17:272–277.
- Macmillan DS, Canipa SJ, Chilton ML, Williams RV, Barber CG. 2016. Predicting skin sensitisation using a decision tree integrated testing strategy with an in silico model and in chemico/in vitro assays. Regul Toxicol Pharmacol. 76:30–38.
- Martin SF, Esser PR, Weber FC, Jakob T, Freudenberg MA, Schmidt M, Goebeler M. 2011. Mechanisms of chemical-induced innate immunity in allergic contact dermatitis. Allergy. 66:1152–1163.
- Mehling A, Eriksson T, Eltze T, Kolle S, Ramirez T, Teubner W, van Ravenzwaay B, Landsiedel R. 2012. Non-animal test methods for predicting skin sensitization potentials. Arch Toxicol. 86:1273–1295.
- Mekenyan O, Dimitrov S, Pavlov T, Dimitrova G, Todorov M, Petkov P, Kotov S. 2012. Simulation of chemical metabolism for fate and hazard assessment. V. Mammalian hazard assessment. SAR QSAR Environ Res. 23:553–606.
- Mekenyan O, Dimitrov S, Pavlov T, Veith G. 2004. A systematic approach to simulating metabolism in computational toxicology. I. The TIMES heuristic modelling framework. Curr Pharm Des. 10:1273–1293.
- MultiCASE. CASE Ultra: QSAR expert system. [accessed 2018 Aug 22]. http://www.multicase.com/case-ultra.
- Muratov EN, Artemenko AG, Varlamova EV, Polischuk PG, Lozitsky VP, Fedchuk AS, Lozitska RL, Gridina TL, Koroleva LS, Sil'nikov VN, et al. 2010. Per aspera ad astra: application of Simplex QSAR approach in antiviral research. Future Med Chem. 2:1205–1226.
- Naive Bayes Skin Sensitization Model v. 1.1. [accessed 2018 Aug 16]. https://figshare.com/articles/Naive_Bayes_Skin_Sensitization_Model/5758644.
- Natsch A, Emter R, Gfeller H, Haupt T, Ellis G. 2015. Predicting skin sensitizer potency based on in vitro data from KeratinoSens and kinetic peptide binding: global versus domain-based assessment. Toxicol Sci. 143:319–332.
- Natsch A, Emter R, Haupt T, Ellis G. 2018. Deriving a no expected sensitization induction level for fragrance ingredients without animal testing: an integrated approach applied to specific case studies. Toxicol Sci. 165:170–185.
- Natsch A, Ryan CA, Foertsch L, Emter R, Jaworska J, Gerberick F, Kern P. 2013. A dataset on 145 chemicals tested in alternative assays for skin sensitization undergoing prevalidation. J Appl Toxicol. 33:1337–1352.
- Nendza M, Gabbert S, Kühne R, Lombardo A, Roncaglioni A, Benfenati E, Benigni R, Bossa C, Strempel S, Scheringer M, et al. 2013. A comparative survey of chemistry-driven in silico methods to identify hazardous substances under REACH. Regul Toxicol Pharmacol. 66:301–314.
- OASIS-LMC. TIMES model for skin sensitization prediction. [accessed 2018 Jan 30]. http://oasis-lmc.org/products/models/human-health-endpoints/skin-sensitization.aspx.
- OASIS-LMC. TIMES-SS Software. [accessed 2018 Jan 30]. http://oasis-lmc.org/products/software/times.aspx.
- OCHEM. Online chemical modeling environment. [accessed 2018 Mar 28]. https://ochem.eu/home/show.do.
- OECD. eChemPortal. [accessed 2018 July 17]. https://www.echemportal.org/.
- OECD. The OECD QSAR Toolbox. [accessed 2018 July 4]. http://www.oecd.org/chemicalsafety/risk-assessment/oecd-qsar-toolbox.htm.
- OECD. 2012. The adverse outcome pathway for skin sensitisation initiated by covalent binding to proteins. [accessed 2018 Apr 17]. http://www.oecd.org/env/the-adverse-outcome-pathway-for-skin-sensitisation-initiated-by-covalent-binding-to-proteins-9789264221444-en.htm.
- OECD. 2014a. OECD series on testing and assessment. Guidance on grouping of chemicals, second edition. [accessed 2018 Apr 17]. https://www.oecd-ilibrary.org/environment/guidance-on-grouping-of-chemicals-second-edition_9789264274679-en.
- OECD. 2014b. OECD series on testing and assessment. Guidance document on the validation of (quantitative) structure-activity relationship [(Q)SAR] models. [accessed 2018 Apr 17]. http://www.oecd.org/env/guidance-document-on-the-validation-of-quantitative-structure-activity-relationship-q-sar-models-9789264085442-en.htm.
- OECD. 2015a. Test No. 442C: In chemico skin sensitisation. [accessed 2018 Apr 17] http://www.oecd.org/env/test-no-442c-in-chemico-skin-sensitisation-9789264229709-en.htm.
- OECD. 2015b. Test No. 442D: In vitro skin sensitisation. [accessed 2018 Apr 17]. http://www.oecd.org/env/test-no-442d-in-vitro-skin-sensitisation-9789264229822-en.htm.
- OECD. 2017a. Test No. 442E: In vitro skin sensitisation. [accessed 2018 Apr 17]. http://www.oecd.org/env/test-no-442e-in-vitro-skin-sensitisation-9789264264359-en.htm.
- OECD. 2017b. Guidance document on the reporting of defined approaches and individual information sources to be used within integrated approaches to testing and assessment (IATA) for skin sensitisation. [accessed 2018 July 4]. https://www.oecd-ilibrary.org/docserver/9789264274822-en.pdf.
- OECD. 2017c. Guidance document on the reporting of defined approaches to be used within integrated approaches to testing and assessment. [accessed 2018 July 4]. https://www.oecd-ilibrary.org/docserver/9789264274822-en.pdf.
- Otsubo Y, Nishijo T, Miyazawa M, Saito K, Mizumachi H, Sakaguchi H. 2017. Binary test battery with KeratinoSens™ and h-CLAT as part of a bottom-up approach for skin sensitization hazard prediction . Regul Toxicol Pharmacol. 88:118–124.
- Ouyang Q, Wang L, Mu Y, Xie X-Q. 2014. Modeling skin sensitization potential of mechanistically hard-to-be-classified aniline and phenol compounds with quantum mechanistic properties. BMC Pharmacol Toxicol. 15:76.
- Patlewicz G, Aptula AO, Roberts DW, Uriarte E. 2008. A minireview of available skin sensitization (Q)SARs/expert systems. QSAR Comb Sci. 27:60–76.
- Patlewicz G, Ball N, Booth ED, Hulzebos E, Zvinavashe E, Hennes C. 2013. Use of category approaches, read-across and (Q)SAR: general considerations. Regul Toxicol Pharmacol. 67:1–12.
- Patlewicz G, Casati S, Basketter DA, Asturiol D, Roberts DW, Lepoittevin JP, Worth AP, Aschberger K. 2016. Can currently available non-animal methods detect pre and pro-haptens relevant for skin sensitization? Regul Toxicol Pharmacol. 82:147–155.
- Patlewicz G, Dimitrov SD, Low LK, Kern PS, Dimitrova GD, Comber MIH, Aptula AO, Phillips RD, Niemelä J, Madsen C, et al. 2007. TIMES-SS – A promising tool for the assessment of skin sensitization hazard. A characterization with respect to the OECD validation principles for (Q)SARs and an external evaluation for predictivity. Regul Toxicol Pharmacol. 48:225–239.
- Patlewicz G, Helman G, Pradeep P, Shah I. 2017. Navigating through the minefield of read-across tools: a review of in silico tools for grouping. Comput Toxicol. 3:1–18.
- Patlewicz G, Kuseva C, Kesova A, Popova I, Zhechev T, Pavlov T, Roberts DW, Mekenyan O. 2014. Towards AOP application-implementation of an integrated approach to testing and assessment (IATA) into a pipeline tool for skin sensitization. Regul Toxicol Pharmacol. 69:529–545.
- Patlewicz G, Kuseva C, Mehmed A, Popova Y, Dimitrova G, Ellis G, Hunziker R, Kern P, Low L, Ringeissen S, et al. 2014. TIMES-SS-recent refinements resulting from an industrial skin sensitisation consortium. SAR QSAR Environ Res. 25:367–391.
- Patlewicz G, Worth A. 2008. Review of Data Sources, QSARs and Integrated Testing Strategies for Skin Sensitisation. [accessed 2018 June 17]. https://eurl-ecvam.jrc.ec.europa.eu/laboratories-research/predictive_toxicology/doc/EUR_23225_EN.pdf.
- Payne MP, Walsh PT. 1994. Structure-activity relationships for skin sensitization potential: development of structural alerts for use in knowledge-based toxicity prediction systems. J Chem Inf Comput Sci. 34:154–161.
- Politano VT, Api AM. 2008. The Research Institute for Fragrance Materials’ human repeated insult patch test protocol. Regul Toxicol Pharmacol. 52:35–38.
- Promkatkaew M, Gleeson D, Hannongbua S, Gleeson PM. 2014. Skin sensitization prediction using quantum chemical calculations: a theoretical model for the SNAr domain. Chem Res Toxicol. 27:51–60.
- Raunio H. 2011. In silico toxicology - non-testing methods. Front Pharmacol. 2:33.
- Reisinger K, Hoffmann S, Alépée N, Ashikaga T, Barroso J, Elcombe C, Gellatly N, Galbiati V, Gibbs S, Groux H, et al. 2015. Systematic evaluation of non-animal test methods for skin sensitisation safety assessment. Toxicol In Vitro. 29:259–270.
- Reuter H, Spieker J, Gerlach S, Engels U, Pape W, Kolbe L, Schmucker R, Wenck H, Diembeck W, Wittern K-P, et al. 2011. In vitro detection of contact allergens: development of an optimized protocol using human peripheral blood monocyte-derived dendritic cells. Toxicol In Vitro. 25:315–323.
- Roberts DW. 2013. Allergic contact dermatitis: is the reactive chemistry of skin sensitizers the whole story? A response. Contact Dermatitis. 68:245–249.
- Roberts DW, Aptula A, Api AM. 2017. Structure-potency relationships for epoxides in allergic contact dermatitis. Chem Res Toxicol. 30:524–531.
- Roberts DW, Aptula AO. 2014. Electrophilic reactivity and skin sensitization potency of SNAr electrophiles. Chem Res Toxicol. 27:240–246.
- Roberts DW, Aptula AO, Patlewicz G. 2007. Electrophilic chemistry related to skin sensitization. Reaction mechanistic applicability domain classification for a published data set of 106 chemicals tested in the mouse local lymph node assay. Chem Res Toxicol. 20:44–60.
- Roberts DW, Aptula AO, Patlewicz GY. 2011. Chemistry-based risk assessment for skin sensitization: quantitative mechanistic modeling for the SNAr domain. Chem Res Toxicol. 24:1003–1011.
- Roberts DW, Mekenyan OG, Dimitrov SD, Dimitrova GD. 2013. What determines skin sensitization potency-myths, maybes and realities. Part 1. The 500 molecular weight cut-off. Contact Dermatitis. 68:32–41.
- Roberts DW, Natsch A. 2009. High throughput kinetic profiling approach for covalent binding to peptides: application to skin sensitization potency of Michael acceptor electrophiles. Chem Res Toxicol. 22:592–603.
- Roberts DW, Patlewicz G. 2018. Non-animal assessment of skin sensitization hazard: Is an integrated testing strategy needed, and if so what should be integrated? J Appl Toxicol. 38:41–50.
- Roberts DW, Patlewicz G, Dimitrov SD, Low LK, Aptula AO, Kern PS, Dimitrova GD, Comber MIH, Phillips RD, Niemelä J, et al. 2007. TIMES-SS – a mechanistic evaluation of an external validation study using reaction chemistry principles. Chem Res Toxicol. 20:1321–1330.
- Roberts DW, Schultz TW, Api AM. 2017. Skin sensitization QMM for HRIPT NOEL data: aldehyde Schiff-base domain. Chem Res Toxicol. 30:1309–1316.
- Roberts DW, Williams DL. 1982. The derivation of quantitative correlations between skin sensitisation and physio-chemical parameters for alkylating agents, and their application to experimental data for sultones. J Theor Biol. 99:807–825.
- Rorije E, Aldenberg T, Buist H, Kroese D, Schüürmann G. 2013. The OSIRIS weight of evidence approach: ITS for skin sensitisation. Regul Toxicol Pharmacol. 67:146–156.
- Rovida C, Alépée N, Api AM, Basketter DA, Bois FY, Caloni F, Corsini E, Daneshian M, Eskes C, Ezendam J, et al. 2015. Integrated testing strategies (ITS) for safety assessment. ALTEX. 32:25–40.
- Russell WMS, Burch RL. 1959. The principles of humane experimental technique. Michigan: Universities Federation for Animal Welfare.
- Saiakhov R, Chakravarti S, Klopman G. 2013. Effectiveness of CASE Ultra expert system in evaluating adverse effects of drugs. Mol Inform. 32:87–97.
- Schmidt M, Raghavan B, Müller V, Vogl T, Fejer G, Tchaptchet S, Keck S, Kalis C, Nielsen PJ, Galanos C, et al. 2010. Crucial role for human Toll-like receptor 4 in the development of contact allergy to nickel. Nat Immunol. 11:814–819.
- Schultz TW, Amcoff P, Berggren E, Gautier F, Klaric M, Knight DJ, Mahony C, Schwarz M, White A, Cronin MT. 2015. A strategy for structuring and reporting a read-across prediction of toxicity. Regul Toxicol Pharmacol. 72:586–601.
- SkinSensDB. [accessed 2018 Mar 29]. http://cwtung.kmu.edu.tw/skinsensdb/.
- Steiling W. 2016. Safety evaluation of cosmetic ingredients regarding their skin sensitization potential. Cosmet Toiletries. 3:14.
- Strickland J, Zang Q, Paris M, Lehmann DM, Allen D, Choksi N, Matheson J, Jacobs A, Casey W, Kleinstreuer N. 2017. Multivariate models for prediction of human skin sensitization hazard. J Appl Toxicol. 37:347–360.
- Sushko I, Novotarskyi S, Körner R, Pandey AK, Rupp M, Teetz W, Brandmaier S, Abdelaziz A, Prokopenko VV, Tanchuk VY, et al. 2011. Online chemical modeling environment (OCHEM): web platform for data storage, model development and publishing of chemical information. J Comput Aided Mol Des. 25:533–554.
- Sushko I, Salmina E, Potemkin VA, Poda G, Tetko IV. 2012. ToxAlerts: a web server of structural alerts for toxic chemicals and compounds with potential adverse reactions. J Chem Inf Model. 52:2310–2316.
- Talete S.r.l. Dragon. [accessed 2018 Apr 16]. http://www.talete.mi.it/products/dragon_description.htm.
- Teubner W, Mehling A, Schuster PX, Guth K, Worth A, Burton J, van Ravenzwaay B, Landsiedel R. 2013. Computer models versus reality: how well do in silico models currently predict the sensitization potential of a substance. Regul Toxicol Pharmacol. 67:468–485.
- Thyssen JP, Giménez-Arnau E, Lepoittevin JP, Menné T, Boman A, Schnuch A. 2012. The critical review of methodologies and approaches to assess the inherent skin sensitization potential (skin allergies) of chemicals. Part I. Contact Dermatitis. 66: 11–24.
- Thyssen JP, Linneberg A, Menné T, Johansen JD. 2007. The epidemiology of contact allergy in the general population-prevalence and main findings. Contact Dermatitis. 57:287–299.
- Todeschini R, Consonni V, Mannhold R. 2009. Molecular descriptors for chemoinformatics: Volume I: Alphabetical listing/Volume II: Appendices, references. Weinheim: Wiley-VCH.
- Toropova AP, Toropov AA. 2017. Hybrid optimal descriptors as a tool to predict skin sensitization in accordance to OECD principles. Toxicol Lett. 275:57–66.
- ToxFix. CADRE-SS skin sensitization model. [accessed 2018 Jan 30]. http://toxfix.com/skin-sensitization.php.
- Toxtree. [accessed 2018 Apr 16]. http://toxtree.sourceforge.net/.
- Tung CW, Wang CC, Wang SS. 2018. Mechanism-informed read-across assessment of skin sensitizers based on SkinSensDB. Regul Toxicol Pharmacol. 94:276–282.
- UL. REACHAcrossTM – satisfy REACH regulations and ECHA submissions with automated read-across. [accessed 2018 July 7]. https://www.ulreachacross.com/index.html.
- Urbisch D, Becker M, Honarvar N, Kolle SN, Mehling A, Teubner W, Wareing B, Landsiedel R. 2016. Assessment of pre- and pro-haptens using nonanimal test methods for skin sensitization. Chem Res Toxicol. 29:901–913.
- Urbisch D, Honarvar N, Kolle SN, Mehling A, Ramirez T, Teubner W, Landsiedel R. 2016. Peptide reactivity associated with skin sensitization: the QSAR Toolbox and TIMES compared to the DPRA. Toxicol in Vitro. 34:194–203.
- Urbisch D, Mehling A, Guth K, Ramirez T, Honarvar N, Kolle S, Landsiedel R, Jaworska J, Kern PS, Gerberick F, et al. 2015. Assessing skin sensitization hazard in mice and men using non-animal test methods. Regul Toxicol Pharmacol. 71:337–351.
- U.S. EPA. 2018 Interim science policy: Use of alternative approaches for skin sensitization as a replacement for laboratory animal testing. [accessed 2018 Aug 30]. https://www.regulations.gov/document?D=EPA-HQ-OPP-2016-0093-0090.
- van der Veen JW, Rorije E, Emter R, Natsch A, van Loveren H, Ezendam J. 2014. Evaluating the performance of integrated approaches for hazard identification of skin sensitizing chemicals. Regul Toxicol Pharmacol. 69:371–379.
- VEGA HUB. VEGA. [accessed 2018 Mar 28]. https://www.vegahub.eu/portfolio-item/vega-qsar/.
- Verheyen GR, Braeken E, Van Deun K, Van Miert S. 2017. Evaluation of in silico tools to predict the skin sensitization potential of chemicals. SAR QSAR Environ Res. 28:59–73.
- Wang CC, Lin YC, Wang SS, Shih C, Lin YH, Tung CW. 2017. SkinSensDB: a curated database for skin sensitization assays. J Cheminform. 9:5.
- Warne MA, Nicholson JK, Lindon JC, Guiney PD, Gartland KPR. 2009. A QSAR investigation of dermal and respiratory chemical sensitizers based on computational chemistry properties. SAR QSAR. Environ Res. 20:429–451.
- Wijeyesakere SJ, Wilson DM, Settivari R, Auernhammer TR, Parks AK, Sue Marty M. 2018. Development of a profiler for facile chemical reactivity using the open-source Konstanz information miner. Appl In Vitro Toxicol. 4:202–213.
- Williams RV, Amberg A, Brigo A, Coquin L, Giddings A, Glowienke S, Greene N, Jolly R, Kemper R, O'Leary-Steele C, et al. 2016. It's difficult, but important, to make negative predictions. Regul Toxicol Pharmacol. 76:79–86.
- Winkler GC, Perino C, Araya SH, Bechter R, Kuster M, Lovsin Barle E. 2015. Classification of dermal sensitizers in pharmaceutical manufacturing. Regul Toxicol Pharmacol. 72:501–505.
- Wondrousch D, Böhme A, Thaens D, Ost N, Schüürmann G. 2010. Local electrophilicity predicts the toxicity-relevant reactivity of Michael acceptors. J Phys Chem Lett. 1:1605–1610.
- Yuan H, Huang J, Cao C. 2009. Prediction of skin sensitization with a particle swarm optimized support vector machine. Int J Mol Sci. 10:3237–3254.
- Zang Q, Paris M, Lehmann DM, Bell S, Kleinstreuer N, Allen D, Matheson J, Jacobs A, Casey W, Strickland J. 2017. Prediction of skin sensitization potency using machine learning approaches. J Appl Toxicol. 37:792–805.