Abstract
Long non-coding RNAs (lncRNAs) are recently-discovered transcripts that regulate vital cellular processes and are crucially connected to diseases. Despite their unprecedented molecular complexity, it is emerging that lncRNAs possess distinct structural motifs. Remarkably, the 3D shape and topology of full-length, native lncRNAs have been visualized for the first time in the last year. These studies reveal that lncRNA structures dictate lncRNA functions. Here, we review experimentally determined lncRNA structures and emphasize that lncRNA structural characterization requires synergistic integration of computational, biochemical and biophysical approaches. Based on these emerging paradigms, we discuss how to overcome the challenges posed by the complex molecular architecture of lncRNAs, with the goal of obtaining a detailed understanding of lncRNA functions and molecular mechanisms in the future.
Classes of long non-coding RNA (lncRNA) structures
Mammalian genomes are broadly transcribed beyond protein coding genes (Carninci et al. Citation2005; Cheng J et al. Citation2005). Currently, the GENCODE database (version v35) counts 60,656 genes, of which only 19,954 are protein-coding genes, and 229,580 transcripts, of which only 84,485 are protein-coding transcripts (Frankish et al. Citation2019, https://www.gencodegenes.org). Among non-protein coding genes, long non-coding RNAs (lncRNAs) are a recently-defined category of transcripts longer than 200 nucleotides (nt) and not translated into proteins (Rinn and Chang Citation2012; Bonasio and Shiekhattar Citation2014). But, what defines an “lncRNA” exactly? Non-coding transcripts longer than 200 nt are ubiquitously expressed in bacteria and archaea, besides eukaryotes (Weinberg et al. Citation2009; Weinberg et al. Citation2010; Trewhella Citation2016). Moreover, evolutionarily-conserved molecular machines, such as ribosomal and spliceosomal RNAs, group I and II self-splicing introns, the telomerase RNA, and RNase P, also represent prominent examples of >200-nt-long non-coding transcripts (Pyle Citation2014). However, neither the former large prokaryotic transcripts nor the latter ribozymes are generally associated with the acronym “lncRNA”. So, what are the characteristic properties of lncRNAs?
Typically, the term lncRNA is reserved for large eukaryotic transcripts that play a role in the regulation of gene expression at either the transcriptional or translational level (Rinn and Chang Citation2012). In practice, lncRNAs collectively gather a very conspicuous number of transcripts. It is estimated that humans have tens of thousands lncRNA genes. As of September 8th 2020, GENCODE v35 annotates 17,957 lncRNA genes and 48,684 lncRNA transcripts (Frankish et al. Citation2019, https://www.gencodegenes.org), LNCipedia v5.2 annotates 56,946 lncRNA genes and 127,802 lncRNA transcripts (Volders et al. Citation2019, https://lncipedia.org), and NONCODE v5 annotates 96,308 lncRNA genes and 172,216 lncRNA transcripts (Fang S et al. Citation2018, http://www.noncode.org). Thus, lncRNAs inevitably have very diverse characteristics (). Based on their loci of origin, lncRNAs can be classified as enhancer (eRNAs), intergenic (lincRNAs), promoter-associated (pRNAs), or genic/intronic RNAs in sense or antisense orientation (gsRNAs and gaRNAs, respectively) (Bonasio and Shiekhattar Citation2014). Based on their cellular localization, lncRNAs can be nuclear, cytoplasmic, or both (Cabili et al. Citation2015). Based on their maturation process, lncRNAs can be capped, spliced, and polyadenylated, like all mRNAs transcribed by RNA polymerase II, or monoexonic and non-polyadenylated (Bonasio and Shiekhattar Citation2014). Mechanistically, lncRNAs can act as protein decoys, protein scaffolds, or protein guides (Rinn and Chang Citation2012). Some act at their transcription site (in cis), while others act far from their transcription site (in trans) (Ulitsky and Bartel Citation2013). Furthermore, lncRNAs also have highly variable half-lives (Clark et al. Citation2012; Tani et al. Citation2012) and are expressed at different levels in the cell (Ulitsky and Bartel Citation2013; Cabili et al. Citation2015). Finally, certain lncRNAs are tissue-specific, others are ubiquitously expressed (Cabili et al. Citation2011; Mattioli et al. Citation2019), and certain lncRNAs are expressed in early development, others in adult tissues, and others throughout the entire life span of a cell or an organism (Sarropoulos et al. Citation2019).
Table 1. LncRNA classes.
The current definition of lncRNAs is thus loose and combines many different types of RNA. Necessarily, transcripts belonging to such a heterogeneous class also possess very diverse properties at the molecular and structural level. Biochemical and biophysical studies on specific lncRNA targets are thus needed to understand their structural diversity.
It actually took more than two decades since the discovery of human XIST and mouse H19 in the early 1990s (Brannan et al. Citation1990; Brown CJ et al. Citation1992), before the functional importance of lncRNA structures started to be appreciated (). LncRNA structures started to be characterized by chemical and enzymatic probing (Novikova et al. Citation2012b; Ilik et al. Citation2013; Somarowthu et al. Citation2015) when the phenotypic investigation of lncRNA knock-outs showed correlations between these targets and human pathologies (Wapinski and Chang Citation2011; Sauvageau et al. Citation2013) and when an increasing number of cellular studies connected specific lncRNAs with well-defined biological pathways (Rinn et al. Citation2007; Zhou et al. Citation2007; Csorba et al. Citation2014). On the basis of those early structural studies, the following hypotheses were initially proposed to classify the lncRNA architectures (Novikova et al. Citation2012a). LncRNAs may possess a highly compact tertiary core, similar to ribozymes such as the ribosome or self-splicing introns. Alternatively, lncRNAs may possess structured protein binding sites, arranged in a de-centralized scaffold without a compact core. Finally, lncRNAs may possess an overall unstructured architecture, with loosely organized protein binding domains and several long stretches of disordered single-stranded RNA (Novikova et al. Citation2012a). These three hypotheses need not be mutually exclusive, and it is likely that there exist examples of lncRNAs for each of these defined classes (). Since such lncRNAs structural classification was proposed by the Sanbonmatsu lab, however, our understanding of the lncRNA molecular properties has advanced significantly, and we have achieved new milestones, including the characterization of the 3D shape and topology of the first full-length, native lncRNAs (Uroda et al. Citation2019; Kim DN et al. Citation2020) (). Consequently, some new general principles of lncRNA structural organization are emerging. Here, we will review experimentally determined lncRNA structures and the methodologies used to characterize them, and present a perspective for future research and innovation in the field of lncRNA structural biology.
Figure 1. Milestones in lncRNA structural characterization. After the discovery of human XIST and mouse H19 in the early 1990s, it took almost twenty years before systematic structural studies on lncRNAs started. Now, the first studies have been published that characterize full-length, native lncRNA 3D structures. These studies set the ground for future high-resolution investigation on these challenging targets.
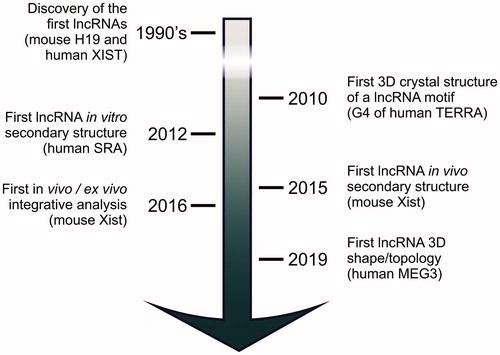
Rationale behind lncRNA structural and mechanistic studies
The functional importance of lncRNA structures has been underappreciated until recently, partly because it has been difficult to assess whether or not lncRNAs are evolutionarily conserved (Rivas et al. Citation2017; Tavares et al. Citation2019). The general paradigm that similar sequences determine similar structures, and similar structures are in turn responsible for similar biological functions is barely applicable to lncRNAs, because evolutionary pressure does not only act on lncRNA sequence, but also on their structure, function, and genomic synteny (Diederichs Citation2014). As a result, the molecular properties of homologous lncRNAs often differ significantly (Chillon and Pyle Citation2016; Kirk et al. Citation2018; Noviello et al. Citation2018). But these considerations should not surprise, because the sequence-structure-function paradigm has numerous exceptions in proteins, too (Martin et al. Citation1998). For example, human α-lactalbumin and chicken egg-white lysozyme are homologous, sharing 40% sequence identity and the same structural fold, but lactalbumin does not possess the hydrolase activity of lysozyme (Kumagai et al. Citation1992). Moreover, “moonlighting proteins” (Jeffery Citation1999) and “promiscuous enzymes” (Khersonsky et al. Citation2006) possess 100% identical protein sequences, but different functions. Finally, “chameleon sequences” are polypeptides that can adopt different secondary and tertiary folds depending on the surrounding environment (Minor and Kim Citation1996). In short, sequence-structure-functional relationships are very diverse in biological macromolecules, and lncRNAs are no exception.
LncRNA structural studies have also been neglected based on the misconception that lncRNAs must be flexible, unstructured molecules, and – as such – their structures would not carry functional information (Zappulla and Cech Citation2004; Blythe et al. Citation2016). Indeed, many large RNAs have flexible regions (Patel et al. Citation2017). For instance, the group II intron self-splicing ribozyme has required extensive engineering to remove unstructured regions and enable crystallization (Toor et al. Citation2008; Marcia and Pyle Citation2012; Marcia et al. Citation2013; Zhao C et al. Citation2015; Marcia Citation2016). Nonetheless, RNA flexibility did not compromise the feasibility of structural studies, including high-resolution structure determination (Pyle Citation2014). In turn, structural characterization of RNA engineered to maximize structural rigidity has provided unmatched details on the functional properties of these transcripts (Manigrasso et al. Citation2020). As a matter of fact, also many eukaryotic proteins contain disordered regions, and both their structured and unstructured segments are functionally important (van der Lee et al. Citation2014). Because intrinsically disordered proteins (IDPs) do not form well-organized hydrophobic cores typical of structured domains, their functionality follows different paradigms than those of globular, structured proteins (van der Lee et al. Citation2014). But the characterization of the structural architecture of IDPs has enormous potential to provide useful functional and mechanistic insights (Jensen et al. Citation2013). It is thus important to perform structural studies both on evolutionarily-conserved and apparently non-conserved molecules, and independent on whether these targets adopt compact and globular, or flexible and disordered architectures.
But what is the rationale that justifies structural studies specifically on lncRNAs? And what can be expected from such an investigation? The answer to these questions lies primarily in what we currently know about the function and mechanism of action of lncRNAs. LncRNAs regulate fundamental cellular processes playing key roles in epigenetics, transcriptional and translational regulation, and scaffolding of key subcellular structures or compartments (Mattick and Rinn Citation2015). As such, lncRNAs are crucially involved in human diseases, such as cancers, infections and developmental disorders (Mattick and Rinn Citation2015). Their functions inherently require that lncRNAs recognize molecular targets in the cell, modulate protein function, contribute to shaping the nuclear architecture and ensure correct targeting of transcription and translation factors (Quinodoz and Guttman Citation2014). In this context, lncRNA structures guarantee efficient gene expression regulation by shaping RNA-protein recognition interfaces and by providing a scaffold for the assembly of ribonucleoprotein complexes. Independent of their exact structural architecture, studying the biochemical and biophysical properties of specific lncRNAs is the only way of experimentally determining the molecular properties of these targets and of facilitating their structure-functional understanding.
Evidence of discrete structural organization in lncRNAs
Interestingly, in vivo genome-wide mapping studies indicate that lncRNAs contain structured regions (Wan et al. Citation2012; Ding et al. Citation2014). Moreover, targeted approaches – some of which have correlated in vivo with in vitro data – suggest that these structures can be confidently reproduced and experimentally probed. Indeed, both in vitro and in vivo secondary structure probing methods appear well-reproducible. Experimental replicas are highly correlated (Smola et al. Citation2016), and there is also high correlation between different chemical and enzymatic probing methods. For instance, human MEG3, mouse Braveheart, mouse RepA, and human HOTAIR were probed with both selective 2′-hydroxyl acylation analyzed by primer extension (SHAPE) reagents and dimethyl sulfide (DMS), human SRA was probed with SHAPE reagents, DMS, and RNase V1, Arabidopsis thaliana COOLAIR was probed with SHAPE reagents and 1-cyclohexyl-(2-morpholinoethyl)carbodiimide metho-p-toluene sulfonate (CMCT) (Novikova et al. Citation2012b; Somarowthu et al. Citation2015; Hawkes et al. Citation2016; Xue et al. Citation2016; Liu F et al. Citation2017; Uroda et al. Citation2019). For each of these lncRNAs, the different probing techniques generally agree by >90% (Kim DN et al. Citation2020). Remarkably, for those rare cases where 3D structure homologues of lncRNA motifs are known, the chemically probed secondary structures are compatible with the available high-resolution models (Bindewald et al. Citation2011; Weeks and Mauger Citation2011; Chillon and Pyle Citation2016). Last but not least, lncRNA structural characterization has guided toward the identification of functionally important motifs, which would have otherwise gone undetected (Xue et al. Citation2016; Uroda et al. Citation2019). If the lncRNAs studied by these structural approaches did not possess a well-regulated structural pattern, probing data would have been non-reproducible and would not have correlated to functionally meaningful features or to 3D experimental data (Kim DN et al. Citation2020). Considering that out of the many thousands of lncRNAs only a handful has been studied from a structural perspective, and that all these structurally-probed lncRNAs possess well-defined and functionally-meaningful molecular architectures, it is evident that lncRNA structural studies will play a major role in the future. However, lncRNAs present unique challenges and require specific working pipelines and technologies for their structure-functional properties to be elucidated at the molecular level in a systematic way.
Challenges in lncRNA structural studies
Challenges in lncRNA structural studies derive from the unavailability of robust computational pipelines for analyzing lncRNA sequences, from the technical limitations posed by the size of lncRNAs on biochemical and biophysical studies, and from the biological complexity of lncRNA and lncRNA cellular pathways, which are typically difficult to probe at the functional level with high-throughput phenotypic assays ().
Figure 2. Technology and challenges in lncRNA structural investigation. LncRNA structural studies can be performed by bioinformatics, biochemical assays, secondary and tertiary structure techniques, and functional assays. Each of these approaches have specific informative potential (listed as “information”) and limitations (listed as “challenges”), but when integrated synergistically they can provide a comprehensive molecular characterization of the lncRNA target of interest, and unearth molecular properties that transcriptomic and high-throughput studies would otherwise leave undetected. A color version of this figure is available online.
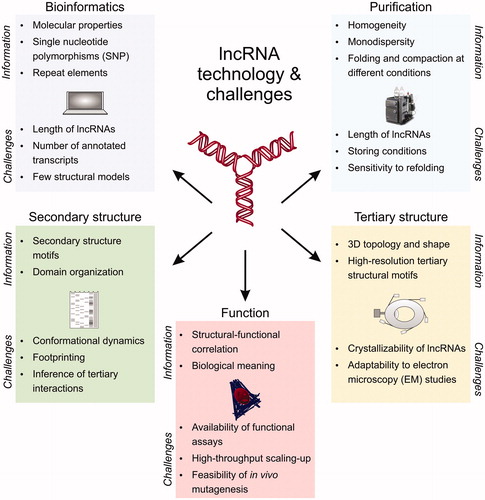
Computational analysis relies on available databases that catalog lncRNA sequences and structures. However, sequence databases are not yet comprehensive, because the number of transcriptomics data is still limited. For instance, despite the number of lncRNA genes being significantly higher than that of protein-coding genes (see above), as of September 8th 2020 Rfam counts only 3,024 entries, mostly derived from tRNAs, rRNAs, snoRNAs, or snRNAs (Kalvari et al. Citation2018, https://rfam.xfam.org), whereas Pfam counts 18,259 entries (El-Gebali et al. Citation2019, https://pfam.xfam.org). Lack of annotated lncRNA sequences – particularly homologous sequences from different organisms – impairs sequence alignments. Especially in the case of multiexonic lncRNAs, this limitation implies that putative homologs need to be identified manually via targeted genomic alignment [i.e. in BLAT (Kent Citation2002)], rather than by high-throughput sequence alignments typically used for proteins [i.e. in BLAST (Altschul et al. Citation1990)] (Chakraborty et al. Citation2014; Uroda et al. Citation2019). There is also no unique database for depositing experimentally mapped secondary structures, which have anyway only been determined for a few targets so far. The low number of annotated lncRNA secondary structures makes ab initio, sequence-based secondary structure calculations challenging, because prediction software does not have access to a sufficient number of sequences for performing comparative analysis, for scoring thermodynamic folding parameters, or for training machine learning algorithms (Zhao et al. Citation2018; Singh et al. Citation2019). For example, the ab initio prediction of the E.coli 16S rRNA secondary structure results in only 52% of correctly identified helices, compared to 98% when quantitative, nucleotide-resolution SHAPE information is used (Deigan et al. Citation2009). Computational predictions also suffer from the complexity inherent in RNA structures, which can interchangeably form localized or long-range interactions (stem-loops vs pseudoknots), and admit a wide range of canonical and non-canonical base-pairs (Leontis et al. Citation2002), besides base triplets and other complex tertiary interactions (Butcher and Pyle Citation2011). Some software can predict RNA secondary structures with pseudoknots [pknotsRG (Reeder and Giegerich Citation2004), Probknot and ShapeKnots (Bellaousov and Mathews Citation2010), IPknot (Sato et al. Citation2011), Knotty (Jabbari et al. Citation2018)], while other algorithms can predict non-canonical base pairs [MC-Fold (Parisien and Major Citation2008), MC-Fold-DP (Honer zu Siederdissen et al. Citation2011), CycleFold (Sloma and Mathews Citation2017)], but none of these computational tools can account for both options (Zhao et al. Citation2018). Computational methods become particularly unreliable when longer sequences need to be analyzed. Folding an RNA of n nucleotides requires ∼8n2 bytes of RAM and computation time increases as n3 (Markham and Zuker Citation2008; Hajdin et al. Citation2010). Thus, some tools impose a length limit for the analysis, which may be problematic for analyzing certain lncRNAs. For instance, RNAfold accepts only RNA sequences up to 7,500 nt for partition function calculations and 10,000 nt for minimum free energy only predictions (Gruber et al. Citation2015). These limitations are more pronounced when the software is embedded in structural prediction pipelines or when operated via online graphical user interfaces (GUI). For instance, mFold is limited to 1400 nt long sequences in GCG (Devereux et al. Citation1984), while RNAStructure modules are limited to 200–4000-nt-long sequences when operated via the online GUI (https://rna.urmc.rochester.edu/RNAstructureWeb/Information/Limitations.html). In these cases, a windowed modeling approach is used by some tools, in which the structure calculations are broken into stages to increase computational efficiency (Siegfried et al. Citation2014). If lncRNA secondary structure prediction is challenging in the absence of experimental data, tertiary structure prediction is currently impossible. There is still no experimental high-resolution 3D structure of any full-length lncRNA, and the number of lncRNA motifs of known 3D structure is still extremely limited. In general, only <0.01% of the >14 million non-coding RNAs collected in RNAcentral possess experimentally determined 3D structures, and these represent only ∼3% of all entries annotated in the Protein Data Bank (PDB). Mostly, these structures correspond to short RNAs or RNA fragments of <200 nt in length. Thus, despite ongoing efforts, current de novo RNA 3D structure prediction are accurate only for short segments (Hajdin et al. Citation2010; Humphris-Narayanan and Pyle Citation2012; Magnus et al. Citation2019; Yesselman et al. Citation2019; Watkins et al. Citation2020).
Production, purification, and storage of lncRNAs is also challenging. These targets are difficult to produce in a homogeneous conformation and canonical methods used for purification of short RNAs may not be appropriate. For instance, because of their large size, lncRNAs are difficult to purify or analyze using electrophoretic methods and they require the use of specific chromatography matrices, generally not commercially available in prepacked, high-resolution and fast flow format (Chillon et al. Citation2015; Uroda et al. Citation2020). Biophysical analysis is equally impacted by lncRNA size. For instance, small-angle X-ray scattering (SAXS) analysis on human MEG3 (∼1600 nt long, 540 kDa in molecular weight) used scattering data at the limits of conventional synchrotron beamlines, so SAXS on lncRNAs larger than MEG3 would require beamlines adapted to very large macromolecular complexes – which are rare – or new technological implementation (Uroda et al. Citation2019; Uroda et al. Citation2020). Moreover, lncRNAs – especially those longer than ∼1000 nt – do not tolerate denaturation and refolding or freezing, so that they are best produced by native purification methods and stored at room temperature to be analyzed rapidly (Chillon et al. Citation2015; Uroda et al. Citation2020).
Last but not least, functional assays on lncRNAs may not always be possible, because cellular and mechanistic data are lacking, or may involve complex cellular or developmental assays, and thus not be easily performable at the throughput that would be required by structure-driven mutagenesis (see below). In vitro functional assays, by contrast, are challenging due to the promiscuity of lncRNA interactions with nuclear proteins and to the difficulty of producing and purifying these protein complexes (Davidovich et al. Citation2013; Davidovich et al. Citation2015). This is perhaps the most important set-back because functional probing is a very useful and informative approach to guide the structural, evolutionary and mechanistic analysis of lncRNAs (Bassett et al. Citation2014; Kashi et al. Citation2016).
Emerging structural motifs in lncRNAs
Despite these challenges, recent studies have unearthed common motifs and patterns of lncRNA structural organization.
Structural motifs that can be identified by sequence analysis
Certain lncRNA structured motifs have been recognized by sequence homology, i.e. because of their conservation with previously characterized viral or mRNA elements ().
Figure 3. LncRNA structured motifs identified by sequence analysis. Top) RACE sequencing of hLincRNA-p21 revealed that this lncRNA comprises IRAlu elements (left: primary structure). Structure probing of these IRAlus in the context of the full-length hLincRNA-p21 (middle: experimental secondary structure map) surprisingly revealed an architecture similar to that of independently-transcribed Alus, i.e., the Alu of the 7SL subunit of the signal recognition particle, which has been previously crystallized (right: crystal structure from PDB 5AOX). Bottom) Identification of a PAN-like triple helix-forming motif in MALAT1 (left: sequence) fostered the secondary (middle) and high-resolution 3D (right: crystal structure from PDB 4PLX) structure characterization of this short lncRNA motif. A color version of this figure is available online.
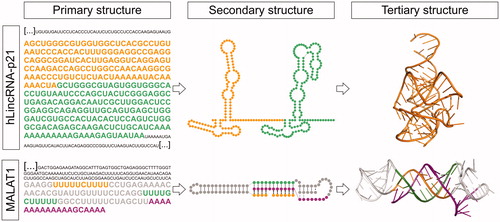
A prominent example of a viral structural motif recognizable at the sequence level is the 3′-end triple helix of the polyadenylated nuclear non-coding RNA (PAN) produced by the Kaposi’s sarcoma-associated herpesvirus [KSHV, (Mitton-Fry et al. Citation2010)]. This triple helical structure is characteristically recognized by the presence of two U-rich motifs separated by a stem-loop structure upstream of the poly(A) tail. It is functionally important, because it protects the poly(A) tail from degradation by deadenylation, thus conferring stability to the RNA. In 2012, the Sharp and Steitz groups identified a PAN-like sequence at the 3′-end of human nuclear lncRNAs MALAT1 and NEAT1 (Brown JA et al. Citation2012; Wilusz et al. Citation2012). Differently from the KSHV mRNA, neither MALAT1 nor NEAT1 contain a canonical poly(A) tail. Instead, they possess a genomically-encoded A-rich tract, which forms the 3′-end of the mature lncRNAs after RNase P cleavage (Wilusz et al. Citation2008; Sunwoo et al. Citation2008). However, similarly to KSHV, the MALAT1 and NEAT1 A-rich motif is preceded by two homologous U-rich motifs separated by a predicted stem-loop-forming sequence. This PAN-like motif is highly conserved in MALAT1 and NEAT1 from humans to reptiles, which facilitated the identification and 3D structure characterization (Brown JA et al. Citation2012; Wilusz et al. Citation2012). The crystal structure of the MALAT1 PAN-like triple helix showed the presence of characteristic U•A-U triplets interrupted by a central C+•G-C triplet and a C-G doublet, thus revealing a key molecular difference between lncRNA triplexes and the KSHV viral triplex, which is shorter (Brown JA et al. Citation2014). This helical reset showed that RNA triple helices are restricted to a limited number of successive base triples to avoid steric clashes, which occur when consecutive stacked base triples accumulate (Brown JA et al. Citation2014) ().
Like any other gene, lncRNA genes have also been invaded by transposable elements (TEs), which – similarly to the PAN-like motifs – are also recognizable by sequence analysis (Lubelsky and Ulitsky Citation2018). TEs have been preserved over evolution, likely because they provide new functionalities to the lncRNAs host, for instance, controlling their cellular localization (Hu et al. Citation2016; Carlevaro-Fita and Johnson Citation2019). The most abundant TE in the human genome is the Alu retrotransposon (Alu element). Alu elements are primate-specific TEs from the short interspersed nuclear element (SINE) family. They count about 300 nt in length and generally contain two repeats ancestrally derived from the 7SL RNA, separated by a short A-rich region, and ending in a longer A-rich tract (Kriegs et al. Citation2007; Deininger Citation2011). Alu elements are found in nature as stand-alone transcripts produced by RNA polymerase III, as well as embedded in mRNA or lncRNAs, which are instead transcribed by RNA polymerase II (Walters et al. Citation2009; Kramerov and Vassetzky Citation2011; Kim EZ et al. Citation2016). One example of an Alu-containing lncRNA is the human lincRNA-p21 (hLincRNA-p21), which contains two inverted-repeat Alu elements (IRAlus) (Chillon and Pyle Citation2016). Using chemical probing and structure-driven covariation analysis, the Pyle lab observed that the hLincRNA-p21 IRAlus are conserved in sequence and in secondary structure in primates. The higher conservation of the Alu elements suggested that these elements are functionally important for hLincRNA-p21, which is in line with previous observations that TE sequences evolve under greater evolutionary constraint than non–TE sequences in lncRNAs (Kapusta et al. Citation2013). Thus not surprisingly, mutagenesis on the hLincRNA-p21 IRAlu elements showed that disrupting the helical structure of these motifs affects the cellular localization of hLincRNA-p21 and prevents this lncRNA from co-localizing with NEAT1 in specific nuclear bodies called paraspeckles (Chillon and Pyle Citation2016). Importantly, compensatory mutagenesis that regenerated the helical structure of the IRAlus also restored the physiological cellular localization of this lncRNA (Chillon and Pyle Citation2016). This IRAlu-mediated mechanism of hLincRNA-p21 nuclear retention may be important during the cellular stress response, via a yet unknown molecular mechanism. Remarkably, the secondary structure of the hLincRNA-p21 IRAlus obtained by chemical probing from this ∼4kb long lncRNA is nearly identical to that derived by crystallography for the stand-alone functional Alu element of the 7SL RNA (Ahl et al. Citation2015; Chillon and Pyle Citation2016) ().
Specific RNA motifs like G-quadruplexes (G4) are also recurrent in lncRNAs and can be predicted by sequence analysis, i.e. using the QGRS Mapper algorithm (Kikin et al. Citation2006). These predictions are less robust than predictions of PAN-like triple helices or TEs, because the presence of a G4 consensus sequence does not necessarily imply its formation in vivo or a functional role (Weldon et al. Citation2017; Yang SY et al. Citation2018). It is thus necessary to confirm G4 formation experimentally, i.e. with 7-deazaguanine-substituted RNA (Weldon et al. Citation2017) or G4-specific immunoprecipitation and sequencing (Yang SY et al. Citation2018), and to assess the functional contribution of G4 to the particular lncRNA of interest. In lncRNAs, G4 may affect translation or splicing, as the G4 present in mRNAs (Weldon et al. Citation2017), but they may also increase lncRNA stability (Yang SY et al. Citation2018), introduce structural plasticity, or modulate lncRNA interactions with chromatin-modifying Polycomb repressive complexes (PRCs) (Wang et al. Citation2017). For instance, using enzymatic probing and 1H nuclear magnetic resonance (NMR) analysis, it was suggested that a G4 structure in equilibrium with a duplex structure regulates the interaction between a minimal construct derived from human HOTAIR and PRC2 (Wu et al. Citation2013). The most prominent G4-containing lncRNAs are the G-rich telomeric repeat-containing RNAs TERRA, which varies in size from 0.2–10 kb in humans and mice and is transcribed from subtelomeric regions toward the chromosome ends (Azzalin et al. Citation2007). For this reason, TERRA transcripts consist of subtelomeric-derived sequences at their 5′ end and terminate with extended tandem repeats of the sequence UUAGGG [reviewed in Bettin et al. (Citation2019)]. Due to its repetitive nature, TERRA can form RNA:DNA duplex structures (R-loops) by hybridizing to the complementary single-stranded C-rich telomeric DNA. These hybrid structures participate in the regulation of the telomere length, as they promote recombination-mediated elongation after the inactivation of the telomerase in differentiated cells (Balk et al. Citation2013; Arora et al. Citation2014). However, the tandem repeats of TERRA can also adopt stable parallel-stranded G4 structures (Xu et al. Citation2008). The crystal structure of TERRA G4 showed that this motif differs from the G4 formed by the telomeric DNA counterpart because of the presence of the 2′ hydroxyl groups, which can establish not only inter-molecular interactions with water molecules but also intra-molecular hydrogen bonds (Collie et al. Citation2010). These TERRA G4 structures have become particularly attractive from a biomedical perspective, as their stabilization with small molecules represents a potential tumor-selective target for chemotherapy (Hirashima and Seimiya Citation2015) ().
Finally, lncRNA sequence motifs important for structural and functional interactions can also be recognized because of their base complementarity to known functional motifs of other RNAs and/or via consensus sequence analysis. For instance, recent work has identified sequences responsible for lncRNA nuclear localization (Zhang B et al. Citation2014; Lubelsky and Ulitsky Citation2018). Other studies have revealed that many lncRNAs possess sequences complementary to a recognition motif of the U1 snRNP (Yin et al. Citation2020). The interaction between these motifs by intermolecular sequence complementarity modulates the localization of the lncRNA on chromatin in a U1 snRNP dependent manner (Yin et al. Citation2020). The functional implications of such interaction were studied in detail for human and mouse MALAT1, whose nuclear localization was disrupted when its interaction with U1 snRNP was abolished (Yin et al. Citation2020). These results reveal how the lncRNA sequence can specifically recognize cellular partners and, in turn, connect lncRNA localization with crucial splicing and transcription factors for coordinated regulation of gene expression (Yin et al. Citation2020).
In summary, from sequence analysis it may be possible to identify lncRNA functional motifs, but it is important to stress that such analysis will likely only unearth the presence of localized structured domains, and not be sufficient to comprehensively dissect the structural-functional relationships of the entire lncRNA molecule of interest.
Recurrent patterns of lncRNA secondary structure organization
The majority of functional lncRNA motifs cannot be detected at the sequence level, but require experimental investigation of the corresponding lncRNA secondary structure. These experiments provide a detailed cartography of the target and enable the identification of recurrent lncRNA substructures (). Examples of lncRNAs for which an experimental secondary structure has been determined are reported in .
Figure 4. LncRNA structured motifs identified by secondary and tertiary structure analysis. Top) Secondary structure probing of human lncRNA MEG3 (center), revealed the presence of highly conserved intra-molecular interactions, or pseudoknots (complementary sequences highlighted in the left panel), which are essential for conferring MEG3 a surprisingly compact 3D topology (AFM image in the right panel) and its ability to stimulate p53-target gene expression. Bottom) Secondary structure probing of mouse lncRNA Braveheart (middle panel) revealed the presence of a rare RHT motif, named AGIL (sequence highlighted in the left panel). AGIL is crucial for ensuring Braveheart recognition of its partner CNBP, which is the mechanism by which this lncRNA promotes cardiomyocyte differentiation and the correct development of the heart. The 3D structure of Braveheart with CNBP has been studied by SAXS in solution (right panel). MEG3 and Braveheart primary and secondary structures are color coded by exons. A color version of this figure is available online.
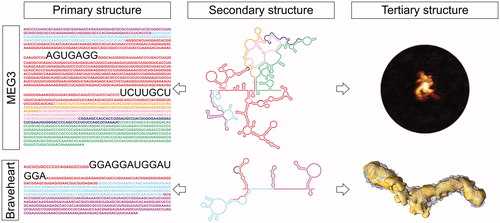
Table 2. Examples of lncRNA secondary structure characterization.
First, these studies have revealed that lncRNAs possess a modular architecture. Each module is an RNA domain of several tens to hundreds of nucleotides in length, which possesses an independently-folding structure. The modular organization of several lncRNAs, i.e. human SRA, A. thaliana COOLAIR, and mouse Braveheart, has been proven experimentally using the so-called 3S shotgun approach developed in the Sanbonmatsu lab (Novikova et al. Citation2013). For other lncRNAs, like human MEG3, structural modularity was confirmed by structure-based alignments and extensive functional probing (Uroda et al. Citation2019). Besides forming independently-folding units, the secondary structure modules also often correspond to independent functional units. For instance, in human HOTAIR, the 5′-terminal module (domain 1, D1) corresponds to the portion of this lncRNA devoted to the interaction with PRC2, whereas the 3′-terminal motif (D4) interacts with lysine demethylase LSD1 (Tsai et al. Citation2010; Li et al. Citation2013; Somarowthu et al. Citation2015). In certain cases, a close correspondence between the modular boundaries and the exon boundaries of the target lncRNA has also been observed, i.e. for mouse Braveheart (Xue et al. Citation2016) and human MEG3 (Uroda et al. Citation2019).
Second, experimental lncRNA secondary structures describe the architecture of each target in terms of secondary structure motifs, such as helical stems, internal and terminal loops, bulges and multi-way junctions (Lescoute and Westhof Citation2006; Laing and Schlick Citation2009) and critically reveal the presence of non-canonical base pairs (GU wobble, GA imino or GA sheared), which are often abundant at functionally important regions (Leontis and Westhof Citation2001; Leontis et al. Citation2002). Interestingly, the number of these secondary structure elements in lncRNAs is as high as in rRNAs or ribozymes, proportionally to the length of the RNA, suggesting similar structural complexity (Novikova et al. Citation2012b; Somarowthu et al. Citation2015; Chillon and Pyle Citation2016; Hawkes et al. Citation2016; Xue et al. Citation2016; Lin et al. Citation2018; Uroda et al. Citation2019). For instance, human SRA, which is organized in four domains, contains 25 helices, 16 terminal loops, 15 internal loops, and 5 junction regions, together with several CU and CA non-Watson–Crick pairs (Novikova et al. Citation2012b); human HOTAIR, which is also organized in four independently-folding domains, possess 56 helical segments, 38 terminal loops, 34 internal loops, and 19 junction regions (Somarowthu et al. Citation2015); and human MEG3 v1, which forms 5 structured domains, possesses 16 multi-way junctions, 35 terminal loops, 40 internal loops, and 51 helices (Uroda et al. Citation2019). A particularly interesting example of secondary structural organization is represented by mouse RepA and human XIST, because RepA is homologous to the A- and F-repeat units of XIST, but is transcribed as an independent molecule (Wutz et al. Citation2002; Zhao J et al. Citation2008; Minks et al. Citation2013). Interestingly, RepA is composed of three independently-folding modules radiating from a central junction (Liu F et al. Citation2017) and thus differs from the tandem dual stem-loop predicted model (Wutz et al. Citation2002) or the dimerization models of nonconsecutive individual repeats (Maenner et al. Citation2010; Duszczyk et al. Citation2011) proposed for XIST. Specifically, the RepA structure obtained by the Pyle lab showed that, within the A-repeat units located in domain 1, only repeat 5 adopts the dual stem-loop structure, whereas repeats 1, 2 and 6 form diverse types of stem-loop motifs, and repeats 3 and 4, and 7 and 8 base-pair with each other, respectively (Liu F et al. Citation2017).
Besides the helical architecture, the topology of multiway junctions also deserves particular attention, because it is critical for defining the lncRNA 3D topology and the consequent formation of peripheral long-range tertiary interactions (Butcher and Pyle Citation2011; Zhao C et al. Citation2015). For instance, in human SRA, the topology of a four-way junction in D1 is critical for orienting functional stems H4 and H5 and that of a three-way junction in D3 is critical for orienting functional stems H15-H17 (Novikova et al. Citation2012b). In A. thaliana COOLAIR, a 3-way and a 5-way junctions were shown to be evolutionarily conserved in Brassicaceae and thus likely carry functional importance (Hawkes et al. Citation2016). In human MEG3, the three-way junction J3 is strategically formed in D2 to orient the highly conserved and functional H10 and H11 stems (Uroda et al. Citation2019). Notably, the function of multi-way junctions may not always be evident from or easy to probe by cellular assays. For instance, mutations in a 5-way junction in the central module of mouse Braveheart did not reveal any phenotypic defects (Xue et al. Citation2016). However, a more detailed investigation of the Braveheart 3D structure by SAXS in isolation and in complex with its protein effector CNBP, suggested that the topology of this 5-way junction may be important for the correct binding of the protein partner (Kim DN et al. Citation2020).
While junctions confer rigidity, internal loops are critical to modulate the flexibility of the helical stems, crucially determining the potential of the RNA to engage in peripheral intra- or inter-molecular interactions (Zhao C et al. Citation2015). Recurrent internal loop structures that are emerging as particularly important for lncRNA function are the asymmetric right-hand turns (r-turns, or RHTs). RTHs are internal loops characterized by a single-stranded segment of 13–20 nucleotides on the 5′-side (N5’) and a single-stranded segment of 0–4 nucleotides on the 3′-side (N3’), so that N5’/N3’ ≥ 4, respectively (Hawkes et al. Citation2016). Adjacent to the loop region, RTHs typically contain pairs of non-canonical GA or GU interactions (Hawkes et al. Citation2016). RTHs are recurrent in the U6 snRNP and pistol ribozyme, where they act as the receptor for protein PRP24 or for an intramolecular pseudoknot interaction, respectively (Hawkes et al. Citation2016). In lncRNAs, RTHs have been identified in human SRA (Novikova et al. Citation2012b), A. thaliana COOLAIR (Hawkes et al. Citation2016), and mouse Braveheart (Xue et al. Citation2016). In the latter lncRNA, an RTH called the 5′ asymmetric G-rich internal loop (AGIL) motif was shown to be particularly crucial for function by the Sanbonmatsu and Boyer groups (Xue et al. Citation2016). Braveheart is an lncRNA that act in trans to regulate cardiovascular lineage commitment (Klattenhoff et al. Citation2013). Deletion of the AGIL motif by CRISPR/Cas9-mediated homology-directed repair showed that the AGIL motif is necessary for cardiomyocyte differentiation, due to its interaction with the single-stranded G-rich binding protein CNBP, which participates in cardiac development (Xue et al. Citation2016) ().
Finally, secondary structure elements identified in lncRNAs may display analogies to other known nucleic acid motifs and thus give insights into lncRNA functions. For example, the lncRNA-E2 – which is involved in pluripotency maintenance in embryonic stem cells – displays similarity to a DNA segment recognized by the transcription factor Sox2 (Ng et al. Citation2012; Holmes et al. Citation2020). Indeed, this segment recognizes the Sox2 DNA-binding HMG domain, using a partially overlapping set of amino acids than that used to interact with DNA, so that the interaction of Sox2 with DNA or RNA is mutually exclusive (Holmes et al. Citation2020). A similar lncRNA-protein interaction has been described for the recognition of steroid receptors (SRs) by human GAS5, which promotes the inhibition of the steroid-mediated transcriptional activity (Kino et al. Citation2010). This repression is mediated through sequence-specific protein RNA-contacts within an A-form double-helical structure with a widened major groove, which interacts with the DNA-binding domain of the SR (Hudson et al. Citation2014). Protein binding can also be altered due to the presence of single-nucleotide polymorphism (SNP) or post-transcriptional modifications (PTMs). For instance, the presence of an N6-methyladenosine (m6A) in a hairpin-stem on MALAT1 produces a local structural rearrangement due to the destabilization of the duplex structure in the vicinity of the modification (Liu et al. Citation2015). As a consequence, a U-tract is exposed, facilitating the binding of the m6A reader protein hnRNP-C, which is involved in several post-transcriptional gene-regulatory processes (Liu et al. Citation2015).
Structural elements that dictate the 3D topology and shape of lncRNAs
Secondary structure analysis may finally reveal potential topological 3D elements, unpredictable by sequence analysis and whose characterization requires tertiary structure analysis (). Typical tertiary interactions in RNA comprise minor groove triples and A-minor interactions, tetraloop receptor interactions, kissing loops and pseudoknots, kink and hook turns, ribose zippers, and T-loops (Butcher and Pyle Citation2011), besides the triple-helical and G4 structures discussed above.
The most prominent example of lncRNA long-range tertiary interactions is currently constituted by the recently determined pseudoknots in human MEG3 (Uroda et al. Citation2019) (). MEG3 is an abundant alternatively spliced nuclear lncRNA that induces cell cycle arrest and apoptosis in adult cells in a p53-dependent manner (Zhang X et al. Citation2003; Zhou et al. Citation2007). The experimentally-determined secondary structures of three different MEG3 splice variants revealed a common structural core that is evolutionarily conserved across mammals (D2-D3) (Uroda et al. Citation2019). Using an in vivo p53-dependent cell-based functional assay, two RNA motifs showed to be necessary for p53 stimulation, namely a highly-conserved hairpin (H11) in D2 and a region comprising H25-H27 in D3. Surprisingly, the terminal loop of H11 is complementary to six repeated sequences in H27, which thus form six mutually-exclusive long-range pseudoknot structures (or “kissing loops”). This long-range tertiary interaction was confirmed by mutagenesis and comparative secondary and tertiary structure investigation, using SHAPE, hydroxyl radical footprinting (HRF), sedimentation velocity analytical ultracentrifugation (SV-AUC), and atomic force microscopy (AFM) (Uroda et al. Citation2019; Uroda et al. Citation2020). AFM remarkably showed that the formation of the H11-H27 pseudoknot in MEG3 is essential to fold this lncRNA into a compact conformation. Compensatory mutagenesis, coupled with cell-based p53-reporter assays, finally proved the functional importance of these MEG3 pseudoknots (Uroda et al. Citation2019). Interestingly, MEG3 also displays a GNRA tetraloop, a putative T-loop (in H10), and multiple bulges (Uroda et al. Citation2019), and may form triple helices between a single-stranded GA-rich repeat sequence at its 5′ end and genomic DNA at selected loci for chromatin targeting (Mondal et al. Citation2015), but the structural and functional importance of these motifs remains to be characterized experimentally.
Besides MEG3, long-range tertiary interactions have also been proposed based on cross-linking results for mouse RepA (Liu F et al. Citation2017), and on gel shift assays for human and mouse NEAT1 (Lin et al. Citation2018). These interactions do not correspond to any canonical RNA tertiary interactions, suggesting that they are either novel types of interactions or that they are in proximity of yet unidentified canonical interactions. Also, in this case, further experimental characterization is needed to elucidate the precise functional roles of these tertiary contacts (Liu F et al. Citation2017; Lin et al. Citation2018).
Technology and available experimental pipelines for lncRNA structural studies
The studies discussed above show that structural investigation on lncRNAs typically requires a combination of computational, biochemical and biophysical analyses. While the experimental approaches used to characterize lncRNAs are individually inherited from previous studies on large ribozymes (Pyle Citation2014), these techniques are often integrated together in unique and unconventional ways to fit to the specific requirements and increased complexity of lncRNAs. The recent advances in secondary structure probing and tertiary structure analysis have introduced new experimental opportunities for lncRNA characterization, and it is thus important to discuss what the informative potential and current limits of these techniques are ().
The computational and experimental approaches that we revise below are intended for the characterization of specific lncRNAs at the molecular level. Suitable targets for such investigation are lncRNAs that fulfill the following requirements: (i) their expression in the biological system of interest has been tested, i.e. by northern blot or transcriptomic approaches (Lowe et al. Citation2017), (ii) their mature full-length sequence has been determined, i.e. by rapid amplification of cDNA ends (5′-/3′-RACE) or long-read sequencing, including annotation of splicing isoforms (Frohman et al. Citation1988; Sharon et al. Citation2013), and (iii) their genomic and cellular environment has been analyzed, i.e. by data mining genome browsers, by studying their cellular localization, and by confirming their non-coding nature (Kashi et al. Citation2016). The correct lncRNA sequence annotation is particularly important, as changes in the transcript organization will have a deep effect on structure, inducing local or global effects with direct consequences on function (Lewis et al. Citation2017). Additionally, characterizing the PTMs and associated proteome of the target lncRNA, i.e. by long read sequencing, hybridization capture and mass spectrometry (Chu et al. Citation2011; Simon MD et al. Citation2011; Smith et al. Citation2019), would also be beneficial before setting up an lncRNA structural study, because PTMs can affect lncRNA structural organization. For example, N6-methyladenosine (m6A) increases the accessibility of its surrounding RNA sequence to bind heterogeneous nuclear ribonucleoprotein G (hnRNP-G), with implications in the selection of alternative splice variants (Liu N et al. Citation2017).
Bioinformatics tools for analyzing lncRNA sequences
The first step in the structural characterization of a lncRNA is the analysis of its sequence. Although bioinformatics of large RNAs presents many challenges (see above), several useful pieces of information can be derived by computation on a novel lncRNA target sequence, which are informative for the planning of wet-lab experiments ().
First, sequence analysis determines the molecular parameters of the target lncRNA, i.e. its molecular weight, nucleotide composition, and extinction coefficient. These parameters are critical in deciding which chromatography column, electrophoresis gel matrices, and centrifugal filters to use for purification (see below), to determine the lncRNA concentration at various stages of purification, and to set up the appropriate experimental conditions for biochemical and biophysical investigation, i.e. by SV-AUC and SAXS. Several online tools are available for calculating such parameters from the sequence of the lncRNA of interest (i.e.: from https://molbiol-tools.ca). Independent of the tool used, the values obtained in silico are estimates of experimental values, which can later be obtained by mass spectrometry or size exclusion chromatography coupled with multi-angle laser light scattering (SEC-MALLS) (Uroda et al. Citation2020), but are sufficient to ensure data reproducibility, provided that the same parameters are used consistently, throughout an entire lncRNA structure project.
Besides molecular parameters, sequence analysis also informs about SNPs and PTMs. This information can be obtained by data mining existing databases via genome browsers, and is important because SNPs and PTMs often correlate with potentially interesting functional regions, or regions of medical relevance, which are important targets for functional probing by structure-based mutagenesis, once the lncRNA structure has been determined.
Moreover, sequence analysis can be used to identify conserved homologs of the target lncRNA in different organisms. This evolutionary analysis, although still limited by the number of annotated lncRNA sequences (see above), is critical to enable structure-based covariation studies, once the experimentally-probed secondary structure is obtained (Nawrocki and Eddy Citation2013), and to guide structure-based mutagenesis and functional probing. Evolutionary analyses could also lead to the identification of shorter, more compact, and potentially more easily tractable lncRNA homologs to facilitate high-resolution 3D structural analysis. In this respect, the experience matured in the group II intron field is informative. Group II introns are self-splicing ribozymes that range between 400 and >1000 nt in length. Although the medical implications of fungal introns are of a more immediate impact for human health and thus most initial studies targeted the ∼800-nt long ai5γ intron expressed in fungal mitochondria, the first high-resolution 3D crystal structures of the intron were obtained using the bacterial homolog from Oceanobacillus iheyensis, which is much shorter, i.e. ∼400 nt long (Toor et al. Citation2008; Pyle Citation2010). Two approaches can be followed to identify lncRNA sequence conservation. First, one should mine the Rfam database to identify families associated with the lncRNA of interest (Kalvari et al. Citation2018). Second, using the full-length sequence of the target, one can use BLAT to identify putatively homologous regions in the genome of other annotated organisms, and extract those putatively similar sequences for pairwise or multiple sequence alignments (Kent Citation2002; Uroda et al. Citation2019). Importantly, before performing experimental probing on these homologs, one needs to confirm experimentally by transcriptomics analysis and RACE or long-read sequencing that these lncRNA are actually expressed, and determine what their exact 5′- and 3′-boundaries are (see above).
Finally, some online tools allow for the identification of known domains, such as PAN-like triple helices or TE-derived repeat regions like the Alus (see above), or repeats, which is useful to detect putative intra-molecular interactions. Identification of repeats can be performed with a number of algorithms, such as RepeatMasker (http://www.repeatmasker.org), REPuter (Kurtz et al. Citation2001), the modules palindrome and equicktandem of the EMBOSS suite (Rice P et al. Citation2000), or Dfam, which searches for repeats in genomic DNA (Hubley et al. Citation2016).
Procedures to purify and fold lncRNAs
After sequence analysis, the target lncRNA needs to be produced in pure and homogenous forms for experimental structural studies (Pyle Citation2014, Citation2016). This experimental task differs significantly from protein analysis and from the analysis of short RNAs (). For instance, purification from endogenous sources – which is typical for proteins – is rarely used for lncRNAs, i.e. only for structure probing approaches that monitor folding in the cellular context (i.e. in vivo or ex vivo SHAPE) or for transcriptome-wide studies (Wan et al. Citation2012; Ding et al. Citation2014). In these approaches, the RNA is extracted by cell lysis and phenol-chloroform phase separation. Such extraction, which generally involves denaturation of the target, can also be performed in mild non-denaturing conditions, i.e. by maintaining physiological ionic concentrations throughout the procedure (Smola et al. Citation2015). However, lncRNA production is most commonly done by in vitro transcription, because the levels of endogenous abundance are generally too low, thus making endogenous purification inefficient. Importantly, in vitro transcription allows the production of lncRNAs without tags, which is an advantage with respect to the requirements for protein purification, because under these conditions the target lncRNAs preserve their native scaffold (Chillon et al. Citation2015). Importantly, in vitro transcribed lncRNAs lack PTMs. However, this need not be a limitation. PTMs generally do not occur homogeneously on the entire cellular population of a lncRNA. We thus consider important to first obtain a lncRNA structure map without PTMs, and use such map as a guide to study the structural and functional effect of modifications at a later stage. Following in vitro transcription, lncRNAs can be purified by denaturing or non-denaturing methods. These procedures have been optimized for large housekeeping RNAs, such as tRNAs or self-splicing ribozymes and riboswitches, and can be applied to lncRNAs with minimal optimization. Denaturing purification studies that involve heat denaturation steps and refolding and are employed for small ribozymes with usually high GC sequence composition (Waldsich and Pyle Citation2008; Chillon et al. Citation2014) can be applied to lncRNAs smaller than ∼1000 nt, as proven for human SRA, mouse Braveheart, and human GAS5 (Novikova et al. Citation2012b; Hudson et al. Citation2014; Xue et al. Citation2016). However, for lncRNAs longer than 1,000 nt, RNA denaturation and refolding usually yields inhomogeneous samples, as proven for human HOTAIR and MEG3 (Somarowthu et al. Citation2015; Uroda et al. Citation2019; Uroda et al. Citation2020). For these samples, non-denaturing purification methods are necessary, similar to those used for the crystallization of tRNAs or the group II intron (Toor et al. Citation2008). An lncRNA-customized non-denaturing purification pipeline typically involves transcription by T7 RNA polymerase, enzymatic treatments with DNase and proteinase K, purification by sequential filtering through spin columns (i.e. Amicon centrifugal filter devices), and a final polishing size-exclusion chromatography step using a fast performance liquid chromatography system (FPLC) (Chillon et al. Citation2015; Adams et al. Citation2019; Uroda et al. Citation2020). This approach is grounded on the observation that many RNAs fold co-transcriptionally and that their final fold is sensitive to the order of events occurring during RNA synthesis (Frieda and Block Citation2012; Watters et al. Citation2016). Other non-denaturing methods include the use of tagged RNAs followed by affinity purification (Batey and Kieft Citation2007; Said et al. Citation2009) and purification through weak anion-exchange FPLC (Easton et al. Citation2010), which also preserve the co-transcriptionally folded RNA and avoid folding heterogeneity (Pereira et al. Citation2010), but they have not been used for lncRNA purification yet. These approaches would thus require testing if the non-native tags affect the lncRNA structural and functional integrity.
An important factor to consider when purifying lncRNAs in vitro is the concentration of cations, particularly magnesium, because this parameter crucially affects lncRNA folding. Too low magnesium concentrations will not ensure folding, while too high magnesium concentrations may induce sample aggregation. It is essential to optimize ionic conditions experimentally for each new lncRNA target, but interestingly it is emerging that lncRNAs can be studied at near-physiological concentrations of mono and divalent cations (∼1–10 mM magnesium chloride, ∼100–150 mM potassium chloride), which closely mimic the intracellular environment (Uroda et al. Citation2020). We specifically recommend performing folding studies by titrating magnesium concentrations using sedimentation-velocity analytical ultracentrifugation (Uroda et al. Citation2020).
Secondary structure analysis: lncRNA mapping guides folding prediction algorithms
A pure and homogenous population of lncRNA is suitable for secondary and tertiary structure characterization (Uroda et al. Citation2020). Secondary structure probing can be performed with multiple reagents (, ).
Enzymatic vs. chemical probing of lncRNA structure
RNA has been traditionally probed using structure-specific RNases (Palangat et al. Citation1998; Beniaminov et al. Citation2008; Diaz-Toledano et al. Citation2009). The combined use of the endoribonucleases RNase T1, which recognizes single-stranded RNA at G residues, and RNase V1, which preferentially cleaves between nucleotides in double-stranded regions of the RNA without base specificity, provides an initial estimation of the structural configuration of different viral and house-keeping RNAs (Knapp Citation1989). The additional use of RNase A, which cleaves at the 3′ side of single-stranded C and U residues, increases the accuracy of the folding predictions (Knapp Citation1989). Although broadly used, there are two main problems associated with this enzymatic probing approach. One problem is reproducibility, as secondary-structure footprinting is achieved using partial digestion with the aforementioned RNases (Merino et al. Citation2005). Another limitation is the difficulty of quantifying the extent of the cleavage so that positions are only classified as either reactive or not reactive (Merino et al. Citation2005). Moreover, certain RNases are increasingly difficult to obtain commercially. Due to these limitations, the modern use of enzymatic probing in RNA secondary structure determination has been reduced in favor of complementary techniques, such as chemical probing. For instance, the secondary structure of human SRA and D. melanogaster roX – among the first lncRNAs to have been probed – included RNase probing data (Novikova et al. Citation2012b; Ilik et al. Citation2013), but no other lncRNA has been probed enzymatically since then.
Chemical probing allows for more accurate and quantitative analysis of the reaction products (Costa et al. Citation1998; Ruschak et al. Citation2004; Cordero et al. Citation2012; Tomezsko et al. Citation2020). Some of the chemicals react with the nucleobase moieties of RNA, such as DMS, diethylpyrocarbonate (DEPC), and CMCT. These reagents react largely at nucleophilic positions in the bases that are not engaged in base pairs, with a preference for purines. DMS reacts at N1 in adenosine and N3 in cytidine; DEPC reacts at N7 in adenosine, and; CMCT reacts at N1 in guanidine and N3 in uridine. Despite the uneven modification of nucleobases, DMS is still one of the most broadly used reagents in lncRNA structure determination. For example, the full-length structure of mouse Xist was obtained in vivo using DMS, as this reagent is cell-permeable and reacts rapidly (Fang R et al. Citation2015). A different approach exploits the observation that the reactivity of an RNA ribose hydroxyl group is particularly sensitive to local nucleotide flexibility. Thus, the reaction with an appropriate electrophile forms a 2′-O-adduct that can be used to monitor local structure independent of nucleotide identity (Merino et al. Citation2005). The use of these electrophiles coupled with the detection of the reaction readout at single-nucleotide resolution is the foundation of SHAPE, a technique developed in the Weeks lab (Merino et al. Citation2005). Different SHAPE reagents are available and possess slightly different properties. One of the first reagents to be used was N-methylisatoic anhydride (NMIA). NMIA reacts slowly with its substrate at the 2′OH group at flexible sites in RNA, which translates into a 10-fold difference between ssRNA and dsRNA and a reduction in reactivity by diverse local tertiary interactions, including Hoogsteen paring, base triples, and kissing-loop interactions (Merino et al. Citation2005). One of the most broadly used reagents is the NMIA derivative 1M7, which shows significantly shorter reaction times with respect to NMIA (1M7 reacts to completion in ∼70 s whereas NMIA, in 20 min) and a reaction rate independent of the magnesium concentration. The change in reaction rate from 0 to 20 mM Mg2+ for 1M7 is negligible, while for NMIA, the change is greater than 2-fold (Mortimer and Weeks Citation2007). To distinguish whether constrained nucleotides are part of base-pairing or tertiary interactions, the use of 1M7 in SHAPE has been complemented with two other chemicals to introduce structure-selective differential reactivities: NMIA, which due to its long reactive time, can identify nucleotides in the rare C2’ endo conformation, and 1-methyl-6-nitroisatoic anhydride (1M6), which is able to stack with RNA nucleobases, identifies long-range stacking interactions or backbone turns (Steen et al. Citation2012; Rice GM et al. Citation2014). The use of the differential SHAPE reactivity has been successfully applied to the determination of the secondary structure map of human MEG3 (Uroda et al. Citation2019). For example, the observation that a key terminal loop in MEG3 showed low chemical reactivity helped in the identification of this motif as part of a complex pseudoknot with a distal portion of the molecule (Uroda et al. Citation2019). SHAPE probes can also be readily used for in vivo probing, similarly to DMS. Among these reagents, besides 1M7 (Takahashi et al. Citation2016), also 2-methylnicotinic acid imidazolide (NAI), 2-(azidomethyl)nicotinic acid acyl imidazole (NAI-N3) (Spitale et al. Citation2015; Lee et al. Citation2017) and 5-nitroisatoic anhydride (5NIA) (Busan et al. Citation2019) can be used, as they display improved solubility with respect to 1M7 and a higher signal-to-background ratio (Busan et al. Citation2019).
Despite the prevalence of SHAPE, most lncRNAs have been analyzed using multiple probing techniques, as a tool for cross-validation of the structural reactivity and to avoid possible sample-specific biases in the measurements (Somarowthu et al. Citation2015; Uroda et al. Citation2019). An alternative validation approach is the so-called 3S shotgun approach developed in the Sanbonmatsu lab (Novikova et al. Citation2013), which was used to probe human SRA (Novikova et al. Citation2012b), A. thaliana COOLAIR (Hawkes et al. Citation2016), mouse Braveheart (Xue et al. Citation2016), human and mouse NEAT1_1 (Lin et al. Citation2018), and mouse RepA (Liu F et al. Citation2017). This approach consists of the production of multiple overlapping fragments of the target lncRNA, which are then independently probed along with the full-length species (Novikova et al. Citation2013). A comparison of the reactivity profiles of full-length and fragments enables the identification of fragments that show similar reactivity profiles to the full-length RNA. These fragments are likely to adopt a similar structure both in isolation and in the context of the entire target molecule, and are thus considered to fold as independent modules or structural domains (Novikova et al. Citation2012b).
From manual to high-throughput readout
Detection of enzymatic or chemical modifications in the target lncRNA can be analyzed by primer extension followed by RNA or cDNA fragment separation on a polyacrylamide gel (Fernandez et al. Citation2011) and manual or semi-automated quantification of electrophoretic bands (Das et al. Citation2005). The introduction of capillary electrophoresis (Mitra et al. Citation2008) coupled to the automated quantification of fluorescent peaks (Vasa et al. Citation2008; Karabiber et al. Citation2013) considerably reduced the manual load during the analysis of the structural probing. Now, the introduction of massive parallel sequencing (MPS) technologies has accelerated even further the analysis of structural probing data in an unbiased way, providing a diversified pool of tools available for lncRNA study (). For example, human MEG3 has been recently studied using SHAPE-Map, a technique based on the incorporation of non-complementary nucleotides during cDNA synthesis, which is then measured by MPS (Sherpa et al. Citation2018; Uroda et al. Citation2019).
How to study tertiary structures
Tertiary structure investigation by biochemical and probing methods
Besides secondary structure architecture, chemical probing can also be used to inform on the tertiary structure of the target (Merino et al. Citation2005). For instance, the “mutate-and-map read out through next-generation sequencing” method (M2-seq) introduces mutations in vitro or in vivo at sites of structural interactions and analyzes these mutations by DMS-seq and mutational profiling. Although not yet applied to lncRNAs, M2-seq has the potential to capture not only secondary but also tertiary structural interactions, i.e. Watson-Crick base pairs formed in pseudoknots, connect these structural motifs to function, and facilitate 3D modeling (Kladwang et al. Citation2011; Cheng CY et al. Citation2017; Kappel et al. Citation2020).
Further tertiary structural information can also be obtained using chemical probes different from those used in secondary structure mapping (, ).
Table 3. Examples of lncRNA tertiary structure characterization.
Nucleotide solvent accessibility, and thus the degree of tertiary compaction of the target, is commonly measured using HRF, because hydroxyl radicals are small in size and highly reactive with surface residues of the target lncRNA (Swisher J et al. Citation2001). Hydroxyl radicals are mostly produced by incubating the target lncRNA with Fe-EDTA and hydrogen peroxide in solution, because this technique is inexpensive and easily accessible (Shcherbakova et al. Citation2006; Uroda et al. Citation2019). Alternatively, hydroxyl radicals can be produced by X-ray exposure, i.e. at synchrotrons (Woodson et al. Citation2001), by radiolysis (Ottinger and Tullius Citation2000), by photolysis (Sharp et al. Citation2004), or by peroxynitrite probing (Swisher JF et al. Citation2002), but these methods have not yet been used for lncRNAs. Reactivity can then be measured by primer extension and capillary electrophoresis, or MPS, as for SHAPE (see above). HRF studies have the potential to probe the kinetics of lncRNA folding in the millisecond scale under time-resolved conditions (Brenowitz et al. Citation2002; Shcherbakova et al. Citation2006), or conformational differences between different lncRNA species at equilibrium (Shcherbakova and Mitra Citation2009). The latter approach was used to produce the single-nucleotide resolution solvent accessibility map of human MEG3 in different folding states and to prove the in vitro formation of its functional H11-H27 pseudoknot by comparing wild type and mutated constructs (Uroda et al. Citation2019).
While HRF provides solvent accessibility information and thus a global insight into the folded architecture of RNA, it does not inform on what residues are specifically involved in mediating long-range tertiary interactions. Such interactions can instead be obtained using alternative biochemical and probing approaches. On the one hand, long-range base-pairing can be detected by RNA antisense purification (RAP) to systematically map RNA-RNA interactions (Engreitz et al. Citation2014) or by psoralen analysis of RNA interactions and structures [PARIS, (Lu et al. Citation2016)]. Because its resolution and throughput are low, RAP has been used so far only to map interactions of the U1 snRNA with pre-mRNAs (Lu et al. Citation2016), but not lncRNAs. Instead, PARIS, which combines in vivo crosslinking, purification of RNA duplexes, and proximity ligation, was used to determine the structure of the A-repeat of human and mouse XIST, revealing a complex inter-repeat duplex organization necessary to mediate the interaction with protein SPEN (Lu et al. Citation2016). Similar long-range interactions were also detected in human and mouse MALAT1 (Lu et al. Citation2016). On the other hand, non-canonical tertiary interactions were detected in mouse RepA by UV crosslinking and primer extension. Three long-range cross-links between RepA D2 and D3 were identified. According to this model, D2 and D3 interact and establish a relatively rigid scaffold, which could facilitate the proper orientation of RepA for protein binding (Liu F et al. Citation2017). Finally, on the basis of the experimentally mapped secondary structure of human and mouse NEAT1_1 and of in silico predictions, putative long-range interactions have been proposed for NEAT1_2. In vitro gel shift assays were used in this case to assess the potential of the two putatively-interacting fragments to form a stable complex (Lin et al. Citation2018).
High-resolution methods
Despite informing on lncRNA globularity and tertiary structure interactions, chemical probing cannot offer a direct 3D atomic visualization of the target lncRNA, which instead requires structural biology approaches. Of the most frequently-used high-resolution structural methods, NMR and X-ray crystallography have been employed successfully on lncRNAs, but so far only for the characterization of short structural elements (, ).
NMR has been used to solve the structure of a 14-nt fragment from the ∼17,000-nt long human XIST [PDB id. 2Y95, (Duszczyk et al. Citation2011)]. However, NMR suffers loss in sensitivity and increased spectral complexity with large (i.e. > 50 kDa) macromolecular targets (Furtig et al. Citation2003). For reference, the largest RNA structure determined by NMR so far is the HIV-1 core packaging signal, which counts 155 nt [PDB id 2N1Q, (Keane et al. Citation2015)]. As a consequence, at least in the immediate future, the contribution that NMR will offer to the characterization of lncRNA will remain limited to structure determination of short motifs and their dynamics (Marusic et al. Citation2019).
By contrast, X-ray crystallography is, in principle, applicable to large macromolecules. However, X-ray crystallography for RNA is more challenging than for proteins, particularly with respect to construct engineering for obtaining well-diffracting crystals (Wiryaman and Toor Citation2017; Gomez and Toor Citation2018) and to phasing (Marcia et al. Citation2013; Marcia Citation2016). Except for the ribosome, the spliceosome, RNase P, and group I and II introns (Pyle Citation2014), RNA crystal structures are available only for short molecules (<200 nt long). In the lncRNA field, X-ray crystallography has thus only been used so far to solve the structures of the 76-nt long PAN-like triple helix from the ∼8,000-nt long human MALAT1 [PDB id 4PLX, 3.1 Å resolution, (Brown JA et al. Citation2014)], of the 12-nt long G4 motif of the ∼200–10,000-nt long human TERRA [PDB 3IBK, 2.2 Å resolution, (Collie et al. Citation2010)], and of a 20-nt long duplex from the ∼4,000-nt long human GAS5 [PDB id 4MCE and 4MCF, 1.9 Å resolution, (Hudson et al. Citation2014)]. In the future, X-ray crystallography of entire lncRNAs or larger lncRNA domains will be possible, provided that folded lncRNAs forming intramolecular tertiary contacts are identified and a robust, medium-to-high throughput functional assay is developed to assist structural engineering of crystal constructs.
Finally, electron microscopy (EM) is another well-established technique for high-resolution structural biology, which is gaining momentum, particularly since the development of direct electron detectors (Kuhlbrandt Citation2014). This technique has been used successfully in the characterization of RNA-protein complexes, including large ribozymes, i.e. the group II intron bound to its maturase partner (Haack et al. Citation2019). Until recently, the pri-miRNA miR-17 ∼ 92 and the SAM-IV riboswitch were the only RNA systems studied in the absence of any protein, at ∼20 Å resolution by negative-staining and at 3.7 Å resolution by cryo-EM, respectively (Chaulk et al. Citation2011; Zhang K et al. Citation2019), but the Das and Chiu labs have now demonstrated that structured RNAs of 100–400 nt in length can be imaged by cryoEM (Kappel et al. Citation2020). However, EM has not been used to solve high-resolution structures of lncRNAs yet. In our hands, predominant challenges consist in the tendency of lncRNAs to aggregate when deposited either on negative staining or cryo-EM grids, which prevents obtaining well-defined reference-free 2d class averages (Uroda et al. Citation2020). Specific optimization is likely required to image lncRNAs by EM in the future [see below and (Uroda et al. Citation2020)].
Low-resolution methods and integrated structural biology approaches
While high-resolution methods have been employed only on short lncRNA segments, three recent studies have now succeeded in characterizing the 3D topology of full-length, native lncRNAs using low-resolution methods (∼15–20 Å), such as SAXS and AFM (, ).
Human MEG3 has been studied with an integrated approach that coupled secondary probing by SHAPE and tertiary probing by HRF (see above) with the visualization of its 3D shape in solution by SAXS and of its 3D topology by single-particle AFM in dry mode (Uroda et al. Citation2019; Uroda et al. Citation2020). These 3D approaches, which are compatible with the inherent structural flexibility and plasticity of full-length, non-engineered lncRNAs and which are not limited by size, have captured MEG3 (1,595 nt long) in different structural conformations and at different stages of folding, which is modulated by the formation/disruption of its functional H11-H27 kissing loop interactions (Uroda et al. Citation2019; Uroda et al. Citation2020). Human HOTAIR has also been studied by AFM, capturing its dynamics in solution, but only in an unfolded form, i.e. at magnesium concentrations (0.5 mM) significantly lower than those that ensure compaction in solution (8.6 mM) (Spokoini-Stern et al. Citation2020). Finally, mouse Braveheart (∼500 nt long) has also been imaged in 3D in solution by SAXS, capturing alternative folding conformations derived from the modulation of its functional AGIL RHT in the presence and absence of its binding protein CNBP (Xue et al. Citation2016; Kim DN et al. Citation2020).
Interestingly, the folding behavior of MEG3 and Braveheart appears rather different. MEG3 shows a distinct folding transition becoming ∼10–20% more compact in the presence of magnesium, than when deprived of magnesium or of its functional pseudoknot structures (Uroda et al. Citation2019). Instead, Braveheart, despite being rigid and possessing well-defined modular domains, adopts an extended conformation that does not change significantly in the presence or absence of magnesium or of its interacting partner CNBP (Kim DN et al. Citation2020).
Independent of the exact folding behaviors of MEG3 and Braveheart, these studies reveal how integrated structural biology can powerfully enable the characterization of novel classes of very large and difficult-to-handle biological macromolecules, such as lncRNAs. Moreover, when coupled with functional assays – as in the cases of MEG3 and Braveheart – these studies have enormous potential in identifying functional and evolutionary-conserved regions that would otherwise go unrecognized. These studies thus have the potential to prepare the ground for future high-resolution characterization of lncRNAs (Uroda et al. Citation2020).
Correlating lncRNA structure with lncRNA function
Functional analysis is crucial for supporting and guiding structural investigation, but may be challenging for lncRNAs. As a result, many lncRNA secondary structures have not yet systematically been probed for function, i.e. SRA (Novikova et al. Citation2012b), COOLAIR (Hawkes et al. Citation2016), RepA (Liu F et al. Citation2017), and NEAT1 (Lin et al. Citation2018). However, for at least some lncRNAs, functional studies are available, and can be performed in vitro, in cell, or in model organisms, with different experimental approaches ().
Figure 5. Approaches for functional probing of lncRNA structures. Left) In vitro assays, such as protein binding assays via RIP/qRT-PCR (top) or EMSA (bottom), can be used to test the ability of lncRNA structure mutants to recognize their molecular targets in the test tube. These approaches have been used to probe the structures of Braveheart, MEG3, GAS5, and HOTAIR. Middle) Cell-based assays, such as cell proliferation assays or cellular localization, can be used if lncRNA structural mutants preserve their cellular functions. These approaches have been used to probe the structures of MEG3, GAS5, XIST, and hLincRNA-p21. Right) LncRNA structures can also be probed by genetically engineering structure-based mutations into model organisms and performing viability or developmental assays. These assays are generally more complex and have lower throughput, and have thus so far only been used for roX (N/A = not available). A color version of this figure is available online.
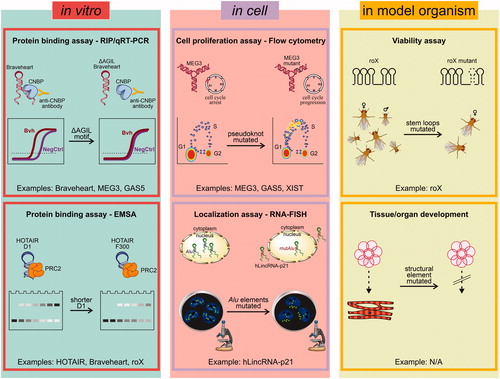
In vitro, lncRNA are typically tested for protein binding, when specific protein partners are known. These assays ensure that the in vitro purified and chemically probed lncRNA adopts a functional conformation that preserves interactions with its known cellular partners. The same assays can then also be applied to structural mutants to help identify the precise motifs responsible for protein recognition. In vitro assays can be performed using purified lncRNAs and proteins by electrophoretic mobility shift assays (EMSA), as for human HOTAIR with PRC2 (Somarowthu et al. Citation2015) and mouse Braveheart with CNBP (Xue et al. Citation2016); by glutathione sepharose RNA affinity chromatography (GNRA), as for D. melanogaster roX with MLE (Ilik et al. Citation2013); or by fluorescence polarization, as for human GAS5 with glucocorticoid receptor (GR) (Hudson et al. Citation2014). In vitro assays on purified lncRNAs can also be performed using cell extracts, i.e. by performing pull-down and specific antibody detection, when the protein partner is known, as for human MEG3 with p53 (Uroda et al. Citation2019), or using microarrays, when the protein partner is not known, as for initial studies on mouse Braveheart (Xue et al. Citation2016). Studies complementary to pull-downs that also make use of cell extracts are RNA immunoprecipitation (RIP) studies. RIP was used to study the binding of wild type and structure-based mutants of human MEG3 with p53 (Uroda et al. Citation2019), mouse Braveheart with CNBP (Xue et al. Citation2016), and human GAS5 with GR (Kino et al. Citation2010).
A number of approaches are then available to test the functionality, stability, or localization of lncRNAs and their corresponding structure mutants in cell. For instance, the p53 stimulation potential of human MEG3 structural mutants and the GR activation potential of human GAS5 structural mutants were characterized by gene reporter assays and by flow cytometric cell cycle/apoptosis assays (Kino et al. Citation2010; Hudson et al. Citation2014; Uroda et al. Citation2019). The cardiovascular lineage commitment potential of mouse Braveheart AGIL mutants was characterized using flow cytometry, immunofluorescence, and qRT-PCR (Xue et al. Citation2016). The X-chromosome inactivation potential of human XIST AUCG tetraloop hairpin mutants was tested by cell viability assays (Duszczyk et al. Citation2011). The RNA stabilization potential of human MALAT1 PAN-like triple helix mutants was studied by Northern blots (Brown JA et al. Citation2014). The ability of D. melanogaster roX mutants to target the MSL complex was characterized by polytene chromosomal immunostaining (Ilik et al. Citation2013). Finally, the nuclear localization potential of hLincRNA-p21 Alu mutants was characterized by RNA fluorescence in situ hybridization (RNA-FISH) (Chillon and Pyle Citation2016).
Finally, although more elaborate and time-consuming, lncRNA functional assays can also be performed in organoids or in model organisms, because lncRNA mutagenesis has the potential to induce functional phenotypes (Sauvageau et al. Citation2013). An example of this functional probing approach is represented by viability studies performed on D. melanogaster roX mutants directly on fly embryos (Ilik et al. Citation2013).
Applicability of lncRNA methods to other classes of large RNAs
The importance of lncRNA structural studies and the correlated technological development is not only useful for advancing the lncRNA field itself, but it is also synergistic and promotes development in other important sectors of RNA biology.
For instance, in vivo genome-wide mapping studies captured not only lncRNAs, but also mRNAs and viral RNAs, showing their tendency to form structured regions in the cell (Wan et al. Citation2012; Ding et al. Citation2014). In this context, chemical probing and structural analysis of coding RNAs could lead to the identification of important regulatory motifs for translation or cellular localization. For instance, TE motifs like the Alu elements are equally present in coding and non-coding transcripts (Walters et al. Citation2009; Kramerov and Vassetzky Citation2011; Kim EZ et al. Citation2016).
Moreover, structural probing methodologies described here for lncRNAs have also had important applications in the field of infection biology, particularly enabling the secondary structure characterization of the genome of many RNA viruses. For instance, the secondary structure of the hepatitis C virus (HCV) genome has been determined by SHAPE and probed by in vivo replication and infectivity assays (Mauger et al. Citation2015; Pirakitikulr et al. Citation2016), the secondary structure of the influenza A virus genome has been determined by in vitro and in vivo DMS-MaP-seq and probed by plaque assays (Simon LM et al. Citation2019), the secondary structure of the genomes of different human immunodeficiency virus (HIV) strains have been characterized by SHAPE/SHAPE-MaP (Watts et al. Citation2009; Lavender et al. Citation2015), and the secondary structure of the 5′-UTR region of the mouse hepatitis virus (MHV) – a SARS-CoV-2 related coronavirus – has been characterized by in vitro and in virio SHAPE and probed by replication assays (Yang D et al. Citation2015). Certain functional segments of viral RNA genomes have also been characterized by structural biology, either at high-resolution, as for the crystal structure of the beet western yellow virus (BWYV) ribosomal frameshifting pseudoknot [PDB id 437 D, 28-nt long, 1.6 Å resolution, (Su et al. Citation1999)], or at low resolution, i.e. by SAXS and AFM, as in the case of the full-length HCV internal ribosome entry site (IRES) (Perard et al. Citation2013; Garcia-Sacristan et al. Citation2015). Finally, AFM has been used to characterize non-naturally-occurring RNAs, i.e. de novo-designed RNA origami nanostructures with potential biomedical applications (Lyubchenko et al. Citation2011; Yu et al. Citation2015).
Future directions
Considering the vast number of lncRNAs identified so far and the rapid development of the field in the last decade, it can be expected that exciting new opportunities will arise for the molecular and mechanistic characterization of these targets in the future. These future directions will require a coordinated effort not just of molecular biologists, biochemists, and structural biologists but of a broader community that will certainly include computational scientists, genome and developmental biologists, and possibly pharmacologists and physicians.
Certainly, with an increasing number of secondary structures being determined by probing, it would be important to establish a unique and dedicated open-access repository for the deposition of secondary structure probing data and related models. A more systematic assessment of secondary structure probing data quality would also be useful. So far, the statistical analysis evaluates SHAPE data in terms of signal/noise ratio (Vaziri et al. Citation2018), but it would be important to have a statistical, unbiased procedure to assess the quality of the derived structure models in terms of their match to the experimental results. For this purpose, an approach similar to the Rfactor approach used in crystallography (Brunger Citation1997) or Fourier shell correlation (FSC) used in cryo-electron microscopy (Afonine et al. Citation2018) could be useful. Since probing is often done with complementary approaches that use different probing agents, a thorough, robust pipeline to integrate data from different sources would also be useful. Pipelines like RNAStructure (Reuter and Mathews Citation2010) or SHAPE-Mapper (Busan et al. Citation2019), already partly address this problem, being capable of integrating SHAPE data with DMS data, but they could be further developed to introduce tertiary structure probes, such as hydroxyl radicals or crosslinking agents. Specifically, it would be beneficial to integrate these pipelines with tools for lncRNA structural visualization, such as VARNA (Darty et al. Citation2009) or the integrative genome viewer (Busan and Weeks Citation2017), for lncRNA conservation, such as Infernal (Nawrocki and Eddy Citation2013), RScape (Rivas et al. Citation2017), and R2R (Weinberg and Breaker Citation2011), and for lncRNA 3D atomistic modeling, such as ERNWIN (Kerpedjiev et al. Citation2015) or RNAComposer (Popenda et al. Citation2012). Moreover, for the analysis of heterogeneous, dynamic lncRNAs, it will be important to customize pipelines that disentangle complex probing results and derive conformational ensembles, rather than unique individual structures, i.e. by principal component or expectation maximization analysis, as already done for large viral RNAs (Eubanks et al. Citation2017; Tomezsko et al. Citation2020). Finally, it would be necessary to better integrate in vitro with in vivo probing, which are complementary approaches. While in vitro probing captures very pure conformations of the target, in vivo approaches capture the target in its physiological cellular environment. Therefore, it should be possible to design pipelines that combine in vitro and in vivo probing data to discern structured lncRNA cores preserved in both environments and thus likely stable and potentially amenable to high-resolution studies, from lncRNA structural regions implicated in cellular interactions and subject to conformational diversity induced by the cellular environment.
Besides computational improvements, advances in biochemical approaches will also set important milestones. For instance, improvements and broader applicability of long-read sequencing technology will enable high-throughput identification of lncRNA PTMs, which can crucially affect lncRNA structure (see above). The systematic identification of lncRNA PTMs will in turn stimulate the development of new technology to selectively introduce these modifications onto in vitro purified lncRNAs for biochemical, biophysical and structural analysis. Moreover, long-read sequencing and in general transcriptomic approaches will enable high-confidence identification of lncRNA splicing variability and enrich lncRNA annotations transcribed in many organisms. From a structural perspective, these advances will be significant, because they will improve and accelerate sequence and structural alignments and thus the identification of homologous transcripts with improved structural properties (i.e. shorter unstructured segments, fewer repeat elements, etc.).
Improvements will also be needed in structural biology for 3D visualization and modeling. So far, low-resolution approaches like SAXS and AFM have been the most successful in visualizing entire lncRNAs (see above). These approaches are, however, limited by throughput and imaging resolution, precluding 3D atomic modeling. High-speed AFM microscopes (Miyagi and Scheuring Citation2018) or the advent of highly automated SAXS beamlines at fourth-generation synchrotrons in the next decade will possibly improve throughput and enable to capture a more complete spectrum of lncRNA conformations, i.e. in different folding states, with different partner proteins, and/or with different mutations. To improve resolution, it will be necessary to produce crystallizable lncRNAs or to adapt EM analysis to these targets. In this respect, optimization of grid preparation (Palovcak et al. Citation2018; Naydenova et al. Citation2019) or vitrification with automatized or semi-automatized methods (Feng et al. Citation2017; Schmidli et al. Citation2019) could help lncRNA deposition on EM supports, preventing their tendency to aggregate (Uroda et al. Citation2020). Improvements in target homogeneity and structural robustness, i.e. by the production of minimal lncRNAs functional cores encompassing only the most highly-structured regions, will also certainly be beneficial, as recently shown by the Das and Chiu labs for a set of 100–400-nt long structured RNAs (Kappel et al. Citation2020; Uroda et al. Citation2020). Finally, the integration of these different low and high-resolution techniques will be useful to obtain more complete molecular views of these challenging targets. For instance, it can be envisaged to use AFM topographic single-particle images as experimental constraints to guide the reconstitution of lncRNA 3D volumes, as for proteins (Trinh et al. Citation2012; Dasgupta et al. Citation2020; Niina et al. Citation2020).
More broadly, it is tempting to speculate that lncRNAs may reserve “molecular surprises”, i.e. they may carry active functions, such as enzymatic catalysis or metabolite recognition, rather than exclusively scaffolding or modulating protein effectors. Considering their medical implications, proving that lncRNAs have the ability to recognize small ligands would open the door to lncRNA-targeted drug development, significantly boosting currently ongoing efforts from private and academic labs to develop RNA-directed compounds (Petrone and DeFrancesco Citation2018).
In summary, it is likely that we are just at the beginning of an exciting era of discoveries on lncRNA structures, functions, and mechanisms. The coordinated effort of an ever-increasing number of researchers investigating the molecular properties of specific lncRNAs will unveil the structural diversity of these complex targets and promote exciting technological developments with important fallouts on many different sectors of RNA biology.
Author contributions
Isabel Chillón and Marco Marcia wrote the manuscript.
Abbreviations: Acronyms of lncRNAs mentioned in this review | ||
GAS5 | = | Growth Arrest-Specific 5 |
HOTAIR | = | HOX Transcript Antisense Intergenic RNA |
LincRNA-p21 | = | Long Intergenic Non-Coding RNA p21 |
MALAT1 | = | Metastasis-Associated Lung Adenocarcinoma Transcript 1 |
MEG3 | = | Maternally Expressed Gene 3 |
NEAT1_1 | = | Nuclear Paraspeckle Assembly Transcript 1 short isoform |
NEAT1_2 | = | Nuclear Paraspeckle Assembly Transcript 1 long isoform |
RepA | = | Repeat A |
roX | = | RNA on the X |
SRA | = | Steroid Receptor RNA activator |
TERRA | = | Telomeric Repeat-containing RNA |
XIST | = | X-Inactive Specific Transcript. |
Other abbreviations | ||
1M6 | = | 1-Methyl-6-Nitroisatoic Anhydride |
1M7 | = | 1-Methyl-7-Nitroisatoic Anhydride |
5NIA | = | 5-Nitro-Isatoic Anhydride |
AFM | = | Atomic Force Microscopy |
CMCT | = | 1-Cyclohexyl-(2-Morpholinoethyl) Carbodiimide metho-p-Toluene sulfonate |
DEPC | = | Diethylpyrocarbonate |
DMS | = | Dimethyl Sulfate |
EM | = | Electron Microscopy |
EMSA | = | Electrophoretic Mobility Shift Assays |
FPLC | = | Fast Performance Liquid Chromatography |
G4 | = | G-quadruplex |
GNRA | = | Glutathione Sepharose RNA Affinity chromatography |
GR | = | Glucocorticoid Receptor |
HCV | = | Hepatitis C Virus |
HIV | = | Human Immunodeficiency Virus |
HRF | = | Hydroxyl Radical Footprinting |
IRAlu | = | Inverted-Repeat Alu Element |
KSHV | = | Kaposi’s Sarcoma-associated Herpes Virus |
MHV | = | Mouse Hepatitis Virus |
Mod-seq | = | RNA chemical modification using high-throughput sequencing |
MPS | = | Massive Parallel Sequencing |
NAI | = | 2-methyl-Nicotinic Acid Imidazolide |
NAI-N3 | = | 2-(azidomethyl)-Nicotinic Acid Acyl Imidazole |
NMIA | = | N-Methyl-Isatoic Anhydride |
NMR | = | Nuclear Magnetic Resonance |
PAN | = | Poly-Adenylated Nuclear non-coding RNA |
PARIS | = | Psoralen analysis of RNA interactions and structures |
PARS | = | Parallel Analysis of RNA Structure |
PDB | = | Protein Data Bank |
PTM | = | Post-Transcriptional Modifications |
qRT-PCR | = | Quantitative Real Time Polymerase Chain Reaction |
RACE | = | Rapid Amplification of cDNA Ends |
RAP | = | RNA antisense purification |
RIP | = | RNA Immuno-Precipitation |
SAXS | = | Small Angle X-ray Scattering |
SAXSBDB | = | Small Angle Scattering Biological Data Bank |
SEC-MALLS | = | Size Exclusion Chromatography coupled to Multi-Angle Laser Light Scattering |
SHAPE | = | Selective 2'-hydroxyl Acylation Analyzed by Primer Extension |
SHAPE-MaP | = | SHAPE and Mutational Profiling |
SINE | = | Short Interspersed Nuclear Element |
SNP | = | Single-Nucleotide Polymorphism |
SR | = | steroid receptor |
SV-AUC | = | Sedimentation Velocity Analytical Ultracentrifugation |
TE | = | Transposable Element |
U1 snRNP | = | U1 small nuclear Ribonucleoprotein. |
Acknowledgements
We would like to thank Dr. Janosch Hennig for critical reading of our manuscript.
Disclosure statement
The authors report no declarations of interest.
Additional information
Funding
References
- Adams RL, Huston NC, Tavares RCA, Pyle AM. 2019. Chapter Twelve - sensitive detection of structural features and rearrangements in long, structured RNA molecules. In: Hargrove AE, editor. Methods Enzymol. Cambridge (UK), Massachusetts (MA): Academic Press, Elsevier; p. 249–289. DOI: 10.1016/bs.mie.2019.04.002. https://en.wikipedia.org/wiki/Methods_in_Enzymology
- Afonine PV, Klaholz BP, Moriarty NW, Poon BK, Sobolev OV, Terwilliger TC, Adams PD, Urzhumtsev A. 2018. New tools for the analysis and validation of cryo-EM maps and atomic models. Acta Crystallogr D Struct Biol. 74(Pt 9):814–840.
- Ahl V, Keller H, Schmidt S, Weichenrieder O. 2015. Retrotransposition and crystal structure of an alu RNP in the ribosome-stalling conformation. Mol Cell. 60(5):715–727.
- Altschul SF, Gish W, Miller W, Myers EW, Lipman DJ. 1990. Basic local alignment search tool. J Mol Biol. 215(3):403–410.
- Arora R, Lee Y, Wischnewski H, Brun CM, Schwarz T, Azzalin CM. 2014. RNaseH1 regulates TERRA-telomeric DNA hybrids and telomere maintenance in ALT tumour cells. Nat Commun. 5:5220.
- Azzalin CM, Reichenbach P, Khoriauli L, Giulotto E, Lingner J. 2007. Telomeric repeat containing RNA and RNA surveillance factors at mammalian chromosome ends. Science. 318(5851):798–801.
- Balk B, Maicher A, Dees M, Klermund J, Luke-Glaser S, Bender K, Luke B. 2013. Telomeric RNA-DNA hybrids affect telomere-length dynamics and senescence. Nat Struct Mol Biol. 20(10):1199–1205.
- Bassett AR, Akhtar A, Barlow DP, Bird AP, Brockdorff N, Duboule D, Ephrussi A, Ferguson-Smith AC, Gingeras TR, Haerty W, et al. 2014. Considerations when investigating lncRNA function in vivo. Elife. 3:e03058.
- Batey RT, Kieft JS. 2007. Improved native affinity purification of RNA. RNA. 13(8):1384–1389.
- Bellaousov S, Mathews DH. 2010. ProbKnot: fast prediction of RNA secondary structure including pseudoknots. RNA. 16(10):1870–1880.
- Beniaminov A, Westhof E, Krol A. 2008. Distinctive structures between chimpanzee and human in a brain noncoding RNA. RNA. 14(7):1270–1275.
- Bettin N, Oss Pegorar C, Cusanelli E. 2019. The emerging roles of TERRA in telomere maintenance and genome stability. Cells. 8(3):246.
- Bindewald E, Wendeler M, Legiewicz M, Bona MK, Wang Y, Pritt MJ, Le Grice SF, Shapiro BA. 2011. Correlating SHAPE signatures with three-dimensional RNA structures. RNA. 17(9):1688–1696.
- Blythe AJ, Fox AH, Bond CS. 2016. The ins and outs of lncRNA structure: how, why and what comes next? Biochim Biophys Acta. 1859(1):46–58.
- Bonasio R, Shiekhattar R. 2014. Regulation of transcription by long noncoding RNAs. Annu Rev Genet. 48:433–455.
- Brannan CI, Dees EC, Ingram RS, Tilghman SM. 1990. The product of the H19 gene may function as an RNA. Mol Cell Biol. 10(1):28–36.
- Brenowitz M, Chance MR, Dhavan G, Takamoto K. 2002. Probing the structural dynamics of nucleic acids by quantitative time-resolved and equilibrium hydroxyl radical "footprinting. Curr Opin Struct Biol. 12(5):648–653.
- Brown CJ, Hendrich BD, Rupert JL, Lafreniere RG, Xing Y, Lawrence J, Willard HF. 1992. The human xist gene - analysis of a 17 Kb inactive X-specific RNA that contains conserved repeats and is highly localized within the nucleus. Cell. 71(3):527–542.
- Brown JA, Bulkley D, Wang J, Valenstein ML, Yario TA, Steitz TA, Steitz JA. 2014. Structural insights into the stabilization of MALAT1 noncoding RNA by a bipartite triple helix. Nat Struct Mol Biol. 21(7):633–640.
- Brown JA, Valenstein ML, Yario TA, Tycowski KT, Steitz JA. 2012. Formation of triple-helical structures by the 3'-end sequences of MALAT1 and MENbeta noncoding RNAs. Proc Natl Acad Sci USA. 109(47):19202–19207.
- Brunger AT. 1997. Chapter 19, free R value: cross-validation in crystallography. In Methods in enzymology. Cambridge (UK), Massachusetts (MA): Academic Press, Elsevier; p. 366–396. DOI: 10.1016/s0076-6879(97)77021-6. https://en.wikipedia.org/wiki/Methods_in_Enzymology
- Busan S, Weeks KM. 2017. Visualization of RNA structure models within the integrative genomics viewer. RNA. 23(7):1012–1018.
- Busan S, Weidmann CA, Sengupta A, Weeks KM. 2019. Guidelines for SHAPE reagent choice and detection strategy for RNA structure probing studies. Biochemistry. 58(23):2655–2664.
- Butcher SE, Pyle AM. 2011. The molecular interactions that stabilize RNA tertiary structure: RNA motifs, patterns, and networks. Acc Chem Res. 44(12):1302–1311.
- Cabili MN, Dunagin MC, McClanahan PD, Biaesch A, Padovan-Merhar O, Regev A, Rinn JL, Raj A. 2015. Localization and abundance analysis of human lncRNAs at single-cell and single-molecule resolution. Genome Biol. 16(1):20.
- Cabili MN, Trapnell C, Goff L, Koziol M, Tazon-Vega B, Regev A, Rinn JL. 2011. Integrative annotation of human large intergenic noncoding RNAs reveals global properties and specific subclasses. Genes Dev. 25(18):1915–1927.
- Carlevaro-Fita J, Johnson R. 2019. Global positioning system: understanding long noncoding rnas through subcellular localization. Mol Cell. 73(5):869–883.
- Carninci P, Kasukawa T, Katayama S, Gough J, Frith MC, Maeda N, Oyama R, Ravasi T, Lenhard B, Wells C, et al. 2005. The transcriptional landscape of the mammalian genome. Science. 309(5740):1559–1563.
- Chakraborty S, Deb A, Maji RK, Saha S, Ghosh Z. 2014. LncRBase: an enriched resource for lncRNA information. PloS One. 9(9):e108010.
- Chaulk SG, Thede GL, Kent OA, Xu Z, Gesner EM, Veldhoen RA, Khanna SK, Goping IS, MacMillan AM, Mendell JT, et al. 2011. Role of pri-miRNA tertiary structure in miR-17 ∼ 92 miRNA biogenesis. RNA Biol. 8(6):1105–1114.
- Cheng CY, Kladwang W, Yesselman JD, Das R. 2017. RNA structure inference through chemical mapping after accidental or intentional mutations. Proc Natl Acad Sci USA. 114(37):9876–9881.
- Cheng J, Kapranov P, Drenkow J, Dike S, Brubaker S, Patel S, Long J, Stern D, Tammana H, Helt G, et al. 2005. Transcriptional maps of 10 human chromosomes at 5-nucleotide resolution. Science. 308(5725):1149–1154.
- Chillon I, Marcia M, Legiewicz M, Liu F, Somarowthu S, Pyle AM. 2015. Native purification and analysis of long RNAs. Methods Enzymol. 558:3–37.
- Chillon I, Molina-Sanchez MD, Fedorova O, Garcia-Rodriguez FM, Martinez-Abarca F, Toro N. 2014. In vitro characterization of the splicing efficiency and fidelity of the RmInt1 group II intron as a means of controlling the dispersion of its host mobile element. RNA. 20(12):2000–2010.
- Chillon I, Pyle AM. 2016. Inverted repeat Alu elements in the human lincRNA-p21 adopt a conserved secondary structure that regulates RNA function. Nucleic Acids Res. 44(19):9462–9471.
- Chu C, Qu K, Zhong FL, Artandi SE, Chang HY. 2011. Genomic maps of long noncoding RNA occupancy reveal principles of RNA-chromatin interactions. Mol Cell. 44(4):667–678.
- Clark MB, Johnston RL, Inostroza-Ponta M, Fox AH, Fortini E, Moscato P, Dinger ME, Mattick JS. 2012. Genome-wide analysis of long noncoding RNA stability. Genome Res. 22(5):885–898.
- Collie GW, Haider SM, Neidle S, Parkinson GN. 2010. A crystallographic and modelling study of a human telomeric RNA (TERRA) quadruplex. Nucleic Acids Res. 38(16):5569–5580.
- Cordero P, Kladwang W, VanLang CC, Das R. 2012. Quantitative dimethyl sulfate mapping for automated RNA secondary structure inference. Biochemistry. 51(36):7037–7039.
- Costa M, Christian EL, Michel F. 1998. Differential chemical probing of a group II self-splicing intron identifies bases involved in tertiary interactions and supports an alternative secondary structure model of domain V. RNA. 4(9):1055–1068.
- Csorba T, Questa JI, Sun Q, Dean C. 2014. Antisense COOLAIR mediates the coordinated switching of chromatin states at FLC during vernalization. Proc Natl Acad Sci USA. 111(45):16160–16165.
- Darty K, Denise A, Ponty Y. 2009. VARNA: interactive drawing and editing of the RNA secondary structure. Bioinformatics. 25(15):1974–1975.
- Das R, Laederach A, Pearlman SM, Herschlag D, Altman RB. 2005. SAFA: semi-automated footprinting analysis software for high-throughput quantification of nucleic acid footprinting experiments. RNA. 11(3):344–354.
- Dasgupta B, Miyashita O, Tama F. 2020. Reconstruction of low-resolution molecular structures from simulated atomic force microscopy images. Biochim Biophys Acta Gen Subj. 1864(2):129420.
- Davidovich C, Wang X, Cifuentes-Rojas C, Goodrich KJ, Gooding AR, Lee JT, Cech TR. 2015. Toward a consensus on the binding specificity and promiscuity of PRC2 for RNA. Molecular Cell. 57(3):552–558.
- Davidovich C, Zheng L, Goodrich KJ, Cech TR. 2013. Promiscuous RNA binding by Polycomb repressive complex 2. Nat Struct Mol Biol. 20(11):1250–1257.
- Deigan KE, Li TW, Mathews DH, Weeks KM. 2009. Accurate SHAPE-directed RNA structure determination. Proc Natl Acad Sci USA. 106(1):97–102.
- Deininger P. 2011. Alu elements: know the SINEs. Genome Biol. 12(12):236.
- Devereux J, Haeberli P, Smithies O. 1984. A comprehensive set of sequence analysis programs for the VAX. Nucleic Acids Res. 12(1 Pt 1):387–395.
- Diaz-Toledano R, Ariza-Mateos A, Birk A, Martinez-Garcia B, Gomez J. 2009. In vitro characterization of a miR-122-sensitive double-helical switch element in the 5' region of hepatitis C virus RNA. Nucleic Acids Res. 37(16):5498–5510.
- Diederichs S. 2014. The four dimensions of noncoding RNA conservation. Trends Genet. 30(4):121–123.
- Dimitrova N, Zamudio JR, Jong RM, Soukup D, Resnick R, Sarma K, Ward AJ, Raj A, Lee JT, Sharp PA, et al. 2014. LincRNA-p21 activates p21 in cis to promote Polycomb target gene expression and to enforce the G1/S checkpoint. Mol Cell. 54(5):777–790.
- Ding Y, Tang Y, Kwok CK, Zhang Y, Bevilacqua PC, Assmann SM. 2014. In vivo genome-wide profiling of RNA secondary structure reveals novel regulatory features. Nature. 505(7485):696–700.
- Duszczyk MM, Wutz A, Rybin V, Sattler M. 2011. The Xist RNA A-repeat comprises a novel AUCG tetraloop fold and a platform for multimerization. RNA. 17(11):1973–1982.
- Easton LE, Shibata Y, Lukavsky PJ. 2010. Rapid, nondenaturing RNA purification using weak anion-exchange fast performance liquid chromatography. RNA. 16(3):647–653.
- El-Gebali S, Mistry J, Bateman A, Eddy SR, Luciani A, Potter SC, Qureshi M, Richardson LJ, Salazar GA, Smart A, et al. 2019. The Pfam protein families database in 2019. Nucleic Acids Res. 47(D1):D427–D432.
- Engreitz JM, Sirokman K, McDonel P, Shishkin AA, Surka C, Russell P, Grossman SR, Chow AY, Guttman M, Lander ES. 2014. RNA-RNA interactions enable specific targeting of noncoding RNAs to nascent Pre-mRNAs and chromatin sites. Cell. 159(1):188–199.
- Eubanks CS, Forte JE, Kapral GJ, Hargrove AE. 2017. Small molecule-based pattern recognition to classify RNA structure. J Am Chem Soc. 139(1):409–416.
- Fang R, Moss WN, Rutenberg-Schoenberg M, Simon MD. 2015. Probing xist RNA structure in cells using targeted structure-seq. PLoS Genet. 11(12):e1005668.
- Fang S, Zhang L, Guo J, Niu Y, Wu Y, Li H, Zhao L, Li X, Teng X, Sun X, et al. 2018. NONCODEV5: a comprehensive annotation database for long non-coding RNAs. Nucleic Acids Res. 46(D1):D308–D314.
- Feng X, Fu Z, Kaledhonkar S, Jia Y, Shah B, Jin A, Liu Z, Sun M, Chen B, Grassucci RA, et al. 2017. A fast and effective microfluidic spraying-plunging method for high-resolution single-particle cryo-EM. Structure. 25(4):663–670. e663.
- Fernandez N, Garcia-Sacristan A, Ramajo J, Briones C, Martinez-Salas E. 2011. Structural analysis provides insights into the modular organization of picornavirus IRES. Virology. 409(2):251–261.
- Frankish A, Diekhans M, Ferreira AM, Johnson R, Jungreis I, Loveland J, Mudge JM, Sisu C, Wright J, Armstrong J, et al. 2019. GENCODE reference annotation for the human and mouse genomes. Nucleic Acids Res. 47(D1):D766–D773.
- Frieda KL, Block SM. 2012. Direct observation of cotranscriptional folding in an adenine riboswitch. Science. 338(6105):397–400.
- Frohman MA, Dush MK, Martin GR. 1988. Rapid production of full-length cDNAs from rare transcripts: amplification using a single gene-specific oligonucleotide primer. Proc Natl Acad Sci USA. 85(23):8998–9002.
- Furtig B, Richter C, Wohnert J, Schwalbe H. 2003. NMR spectroscopy of RNA. Chembiochem. 4(10):936–962.
- Garcia-Sacristan A, Moreno M, Ariza-Mateos A, Lopez-Camacho E, Jaudenes RM, Vazquez L, Gomez J, Martin-Gago JA, Briones C. 2015. A magnesium-induced RNA conformational switch at the internal ribosome entry site of hepatitis C virus genome visualized by atomic force microscopy. Nucleic Acids Res. 43(1):565–580.
- Gomez A, Toor N. 2018. Selecting new RNA crystal contacts. Structure. 26(9):1166–1167.
- Gruber AR, Bernhart SH, Lorenz R. 2015. The ViennaRNA web services. Methods Mol Biol. 1269:307–326.
- Haack DB, Yan XD, Zhang C, Hingey J, Lyumkis D, Baker TS, Toor N. 2019. Cryo-EM structures of a group II intron reverse splicing into DNA. Cell. 178(3):612–623.e12. +.
- Hajdin CE, Ding F, Dokholyan NV, Weeks KM. 2010. On the significance of an RNA tertiary structure prediction. RNA. 16(7):1340–1349.
- Hawkes EJ, Hennelly SP, Novikova IV, Irwin JA, Dean C, Sanbonmatsu KY. 2016. COOLAIR antisense RNAs form evolutionarily conserved elaborate secondary structures. Cell Rep. 16(12):3087–3096.
- Hirashima K, Seimiya H. 2015. Telomeric repeat-containing RNA/G-quadruplex-forming sequences cause genome-wide alteration of gene expression in human cancer cells in vivo. Nucleic Acids Res. 43(4):2022–2032.
- Holmes ZE, Hamilton DJ, Hwang T, Parsonnet NV, Rinn JL, Wuttke DS, Batey RT. 2020. The Sox2 transcription factor binds RNA. Nat Commun. 11(1):1805.
- Honer zu Siederdissen C, Bernhart SH, Stadler PF, Hofacker IL. 2011. A folding algorithm for extended RNA secondary structures. Bioinformatics. 27(13):i129–136.
- Hu S, Wang X, Shan G. 2016. Insertion of an Alu element in a lncRNA leads to primate-specific modulation of alternative splicing. Nat Struct Mol Biol. 23(11):1011–1019.
- Huarte M, Guttman M, Feldser D, Garber M, Koziol MJ, Kenzelmann-Broz D, Khalil AM, Zuk O, Amit I, Rabani M, et al. 2010. A large intergenic noncoding RNA induced by p53 mediates global gene repression in the p53 response. Cell. 142(3):409–419.
- Hubley R, Finn RD, Clements J, Eddy SR, Jones TA, Bao W, Smit AF, Wheeler TJ. 2016. The Dfam database of repetitive DNA families. Nucleic Acids Res. 44(D1):D81–89.
- Hudson WH, Pickard MR, de Vera IM, Kuiper EG, Mourtada-Maarabouni M, Conn GL, Kojetin DJ, Williams GT, Ortlund EA. 2014. Conserved sequence-specific lincRNA-steroid receptor interactions drive transcriptional repression and direct cell fate. Nat Commun. 5:5395.
- Humphris-Narayanan E, Pyle AM. 2012. Discrete RNA libraries from pseudo-torsional space. J Mol Biol. 421(1):6–26.
- Hutchinson JN, Ensminger AW, Clemson CM, Lynch CR, Lawrence JB, Chess A. 2007. A screen for nuclear transcripts identifies two linked noncoding RNAs associated with SC35 splicing domains. BMC Genomics. 8:39.
- Ilik IA, Quinn JJ, Georgiev P, Tavares-Cadete F, Maticzka D, Toscano S, Wan Y, Spitale RC, Luscombe N, Backofen R, et al. 2013. Tandem stem-loops in roX RNAs act together to mediate X chromosome dosage compensation in Drosophila. Mol Cell. 51(2):156–173.
- Jabbari H, Wark I, Montemagno C, Will S. 2018. Knotty: efficient and accurate prediction of complex RNA pseudoknot structures. Bioinformatics. 34(22):3849–3856.
- Jeffery CJ. 1999. Moonlighting proteins. Trends Biochem Sci. 24(1):8–11.
- Jensen MR, Ruigrok RW, Blackledge M. 2013. Describing intrinsically disordered proteins at atomic resolution by NMR. Curr Opin Struct Biol. 23(3):426–435.
- Kalvari I, Argasinska J, Quinones-Olvera N, Nawrocki EP, Rivas E, Eddy SR, Bateman A, Finn RD, Petrov AI. 2018. Rfam 13.0: shifting to a genome-centric resource for non-coding RNA families. Nucleic Acids Res. 46(D1):D335–D342.
- Kaneko S, Bonasio R, Saldana-Meyer R, Yoshida T, Son J, Nishino K, Umezawa A, Reinberg D. 2014. Interactions between JARID2 and noncoding RNAs regulate PRC2 recruitment to chromatin. Mol Cell. 53(2):290–300.
- Kappel K, Zhang K, Su Z, Watkins AM, Kladwang W, Li S, Pintilie G, Topkar VV, Rangan R, Zheludev IN, et al. 2020. Accelerated cryo-EM-guided determination of three-dimensional RNA-only structures. Nat Methods. 17(7):699–707.
- Kapusta A, Kronenberg Z, Lynch VJ, Zhuo X, Ramsay L, Bourque G, Yandell M, Feschotte C. 2013. Transposable elements are major contributors to the origin, diversification, and regulation of vertebrate long noncoding RNAs. PLoS Genet. 9(4):e1003470.
- Karabiber F, McGinnis JL, Favorov OV, Weeks KM. 2013. QuShape: rapid, accurate, and best-practices quantification of nucleic acid probing information, resolved by capillary electrophoresis. RNA. 19(1):63–73.
- Kashi K, Henderson L, Bonetti A, Carninci P. 2016. Discovery and functional analysis of lncRNAs: methodologies to investigate an uncharacterized transcriptome. Biochim Biophys Acta. 1859(1):3–15.
- Keane SC, Heng X, Lu K, Kharytonchyk S, Ramakrishnan V, Carter G, Barton S, Hosic A, Florwick A, Santos J, et al. 2015. RNA structure. Structure of the HIV-1 RNA packaging signal. Science. 348(6237):917–921.
- Kent WJ. 2002. BLAT--the BLAST-like alignment tool. Genome Res. 12(4):656–664.
- Kerpedjiev P, Honer Zu Siederdissen C, Hofacker IL. 2015. Predicting RNA 3D structure using a coarse-grain helix-centered model. RNA. 21(6):1110–1121.
- Khersonsky O, Roodveldt C, Tawfik DS. 2006. Enzyme promiscuity: evolutionary and mechanistic aspects. Curr Opin Chem Biol. 10(5):498–508.
- Kikin O, D'Antonio L, Bagga PS. 2006. QGRS Mapper: a web-based server for predicting G-quadruplexes in nucleotide sequences. Nucleic Acids Res. 34:W676–682.
- Kim DN, Thiel BC, Mrozowich T, Hennelly SP, Hofacker IL, Patel TR, Sanbonmatsu KY. 2020. Zinc-finger protein CNBP alters the 3-D structure of lncRNA Braveheart in solution. Nat Commun. 11(1):148.
- Kim EZ, Wespiser AR, Caffrey DR. 2016. The domain structure and distribution of Alu elements in long noncoding RNAs and mRNAs. RNA. 22(2):254–264.
- Kino T, Hurt DE, Ichijo T, Nader N, Chrousos GP. 2010. Noncoding RNA gas5 is a growth arrest- and starvation-associated repressor of the glucocorticoid receptor. Sci Signal. 3(107):ra8.
- Kirk JM, Kim SO, Inoue K, Smola MJ, Lee DM, Schertzer MD, Wooten JS, Baker AR, Sprague D, Collins DW, et al. 2018. Functional classification of long non-coding RNAs by k-mer content. Nat Genet. 50(10):1474–1482.
- Kladwang W, Cordero P, Das R. 2011. A mutate-and-map strategy accurately infers the base pairs of a 35-nucleotide model RNA. RNA. 17(3):522–534.
- Klattenhoff CA, Scheuermann JC, Surface LE, Bradley RK, Fields PA, Steinhauser ML, Ding H, Butty VL, Torrey L, Haas S, et al. 2013. Braveheart, a long noncoding RNA required for cardiovascular lineage commitment. Cell. 152(3):570–583.
- Knapp G. 1989. Enzymatic approaches to probing of RNA secondary and tertiary structure. Meth Enzymol. 180:192–212.
- Kramerov DA, Vassetzky NS. 2011. Origin and evolution of SINEs in eukaryotic genomes. Heredity. 107(6):487–495.
- Kriegs JO, Churakov G, Jurka J, Brosius J, Schmitz J. 2007. Evolutionary history of 7SL RNA-derived SINEs in Supraprimates. Trends Genet. 23(4):158–161.
- Kuhlbrandt W. 2014. Cryo-EM enters a new era. eLife. 3:e03678.
- Kumagai I, Takeda S, Miura K. 1992. Functional conversion of the homologous proteins alpha-lactalbumin and lysozyme by exon exchange. Proc Natl Acad Sci USA. 89(13):5887–5891.
- Kurtz S, Choudhuri JV, Ohlebusch E, Schleiermacher C, Stoye J, Giegerich R. 2001. REPuter: the manifold applications of repeat analysis on a genomic scale. Nucleic Acids Res. 29(22):4633–4642.
- Laing C, Schlick T. 2009. Analysis of four-way junctions in RNA structures. J Mol Biol. 390(3):547–559.
- Lanz RB, McKenna NJ, Onate SA, Albrecht U, Wong J, Tsai SY, Tsai M-J, O’Malley BW. 1999. A steroid receptor coactivator, SRA, functions as an RNA and is present in an SRC-1 complex. Cell. 97(1):17–27.
- Lavender CA, Gorelick RJ, Weeks KM. 2015. Structure-based alignment and consensus secondary structures for three HIV-related RNA genomes. PLoS Comput Biol. 11(5):e1004230.
- Lee B, Flynn RA, Kadina A, Guo JK, Kool ET, Chang HY. 2017. Comparison of SHAPE reagents for mapping RNA structures inside living cells. RNA. 23(2):169–174.
- Leontis NB, Stombaugh J, Westhof E. 2002. The non-Watson-Crick base pairs and their associated isostericity matrices. Nucleic Acids Res. 30(16):3497–3531.
- Leontis NB, Westhof E. 2001. Geometric nomenclature and classification of RNA base pairs. RNA. 7(4):499–512.
- Lescoute A, Westhof E. 2006. Topology of three-way junctions in folded RNAs. RNA. 12(1):83–93.
- Lewis CJ, Pan T, Kalsotra A. 2017. RNA modifications and structures cooperate to guide RNA-protein interactions. Nat Rev Mol Cell Biol. 18(3):202–210.
- Li L, Liu B, Wapinski OL, Tsai M-C, Qu K, Zhang J, Carlson JC, Lin M, Fang F, Gupta RA, et al. 2013. Targeted disruption of hotair leads to homeotic transformation and gene derepression. Cell Rep. 5(1):3–12.
- Lin Y, Schmidt BF, Bruchez MP, McManus CJ. 2018. Structural analyses of NEAT1 lncRNAs suggest long-range RNA interactions that may contribute to paraspeckle architecture. Nucleic Acids Res. 46(7):3742–3752.
- Liu F, Somarowthu S, Pyle AM. 2017. Visualizing the secondary and tertiary architectural domains of lncRNA RepA. Nat Chem Biol. 13(3):282–289.
- Liu N, Dai Q, Zheng G, He C, Parisien M, Pan T. 2015. N(6)-methyladenosine-dependent RNA structural switches regulate RNA-protein interactions. Nature. 518(7540):560–564.
- Liu N, Zhou KI, Parisien M, Dai Q, Diatchenko L, Pan T. 2017. N6-methyladenosine alters RNA structure to regulate binding of a low-complexity protein. Nucleic Acids Res. 45(10):6051–6063.
- Lowe R, Shirley N, Bleackley M, Dolan S, Shafee T. 2017. Transcriptomics technologies. PLoS Comput Biol. 13(5):e1005457.
- Lu Z, Zhang QC, Lee B, Flynn RA, Smith MA, Robinson JT, Davidovich C, Gooding AR, Goodrich KJ, Mattick JS, et al. 2016. RNA duplex map in living cells reveals higher-order transcriptome structure. Cell. 165(5):1267–1279.
- Lubelsky Y, Ulitsky I. 2018. Sequences enriched in Alu repeats drive nuclear localization of long RNAs in human cells. Nature. 555(7694):107–111.
- Lyubchenko YL, Shlyakhtenko LS, Ando T. 2011. Imaging of nucleic acids with atomic force microscopy. Methods. 54(2):274–283.
- Maenner S, Blaud M, Fouillen L, Savoye A, Marchand V, Dubois A, Sanglier-Cianferani S, Van Dorsselaer A, Clerc P, Avner P, et al. 2010. 2-D structure of the A region of Xist RNA and its implication for PRC2 association. PLoS Biol. 8(1):e1000276.
- Magnus M, Kappel K, Das R, Bujnicki JM. 2019. RNA 3D structure prediction guided by independent folding of homologous sequences. BMC Bioinformatics. 20(1):512.
- Manigrasso J, Chillon I, Genna V, Vidossich P, Somarowthu S, Pyle AM, De Vivo M, Marcia M. 2020. Visualizing group II intron dynamics between the first and second steps of splicing. Nat Commun. 11(1):2837.
- Marcia M. 2016. Using molecular replacement phasing to study the structure and function of RNA. Methods Mol Biol. 1320:233–257.
- Marcia M, Humphris-Narayanan E, Keating KS, Somarowthu S, Rajashankar K, Pyle AM. 2013. Solving nucleic acid structures by molecular replacement: examples from group II intron studies. Acta Crystallogr D Biol Crystallogr. 69(Pt 11):2174–2185.
- Marcia M, Pyle AM. 2012. Visualizing group II intron catalysis through the stages of splicing. Cell. 151(3):497–507.
- Markham NR, Zuker M. 2008. UNAFold: software for nucleic acid folding and hybridization. Methods Mol Biol. 453:3–31.
- Martin AC, Orengo CA, Hutchinson EG, Jones S, Karmirantzou M, Laskowski RA, Mitchell JB, Taroni C, Thornton JM. 1998. Protein folds and functions. Structure. 6(7):875–884.
- Marusic M, Schlagnitweit J, Petzold K. 2019. RNA dynamics by NMR spectroscopy. Chembiochem. 20(21):2685–2710.
- Mattick JS, Rinn JL. 2015. Discovery and annotation of long noncoding RNAs. Nat Struct Mol Biol. 22(1):5–7.
- Mattioli K, Volders PJ, Gerhardinger C, Lee JC, Maass PG, Mele M, Rinn JL. 2019. High-throughput functional analysis of lncRNA core promoters elucidates rules governing tissue specificity. Genome Res. 29(3):344–355.
- Mauger DM, Golden M, Yamane D, Williford S, Lemon SM, Martin DP, Weeks KM. 2015. Functionally conserved architecture of hepatitis C virus RNA genomes. Proc Natl Acad Sci USA. 112(12):3692–3697.
- McCown PJ, Wang MC, Jaeger L, Brown JA. 2019. Secondary structural model of human MALAT1 reveals multiple structure–function relationships. IJMS. 20(22):5610.
- Merino EJ, Wilkinson KA, Coughlan JL, Weeks KM. 2005. RNA structure analysis at single nucleotide resolution by selective 2'-hydroxyl acylation and primer extension (SHAPE). J Am Chem Soc. 127(12):4223–4231.
- Minks J, Baldry SE, Yang C, Cotton AM, Brown CJ. 2013. XIST-induced silencing of flanking genes is achieved by additive action of repeat a monomers in human somatic cells. Epigenet Chromatin. 6(1):23.
- Minor DL, Jr., Kim PS. 1996. Context-dependent secondary structure formation of a designed protein sequence. Nature. 380(6576):730–734.
- Mitra S, Shcherbakova IV, Altman RB, Brenowitz M, Laederach A. 2008. High-throughput single-nucleotide structural mapping by capillary automated footprinting analysis. Nucleic Acids Res. 36(11):e63.
- Mitton-Fry RM, DeGregorio SJ, Wang J, Steitz TA, Steitz JA. 2010. Poly(A) tail recognition by a viral RNA element through assembly of a triple helix. Science. 330(6008):1244–1247.
- Miyagi A, Scheuring S. 2018. A novel phase-shift-based amplitude detector for a high-speed atomic force microscope. Rev Sci Instrum. 89(8):083704.
- Miyoshi N, Wagatsuma H, Wakana S, Shiroishi T, Nomura M, Aisaka K, Kohda T, Surani MA, Kaneko-Ishino T, Ishino F. 2000. Identification of an imprinted gene, Meg3/Gtl2 and its human homologue MEG3, first mapped on mouse distal chromosome 12 and human chromosome 14q. Genes Cells. 5(3):211–220.
- Mondal T, Subhash S, Vaid R, Enroth S, Uday S, Reinius B, Mitra S, Mohammed A, James AR, Hoberg E, et al. 2015. MEG3 long noncoding RNA regulates the TGF-beta pathway genes through formation of RNA-DNA triplex structures. Nat Commun. 6:7743.
- Mortimer SA, Weeks KM. 2007. A fast-acting reagent for accurate analysis of RNA secondary and tertiary structure by SHAPE chemistry. J Am Chem Soc. 129(14):4144–4145.
- Nawrocki EP, Eddy SR. 2013. Infernal 1.1: 100-fold faster RNA homology searches. Bioinformatics. 29(22):2933–2935.
- Naydenova K, Peet MJ, Russo CJ. 2019. Multifunctional graphene supports for electron cryomicroscopy. Proc Natl Acad Sci USA. 116(24):11718–11724.
- Ng SY, Johnson R, Stanton LW. 2012. Human long non-coding RNAs promote pluripotency and neuronal differentiation by association with chromatin modifiers and transcription factors. Embo J. 31(3):522–533.
- Niina T, Fuchigami S, Takada S. 2020. Flexible Fitting of biomolecular structures to atomic force microscopy images via biased molecular simulations. J Chem Theory Comput. 16(2):1349–1358.
- Noviello TMR, Di Liddo A, Ventola GM, Spagnuolo A, D’Aniello S, Ceccarelli M, Cerulo L. 2018. Detection of long non-coding RNA homology, a comparative study on alignment and alignment-free metrics. BMC Bioinf. 19(1):407.
- Novikova IV, Dharap A, Hennelly SP, Sanbonmatsu KY. 2013. 3S: shotgun secondary structure determination of long non-coding RNAs. Methods. 63(2):170–177.
- Novikova IV, Hennelly SP, Sanbonmatsu KY. 2012a. Sizing up long non-coding RNAs: do lncRNAs have secondary and tertiary structure?. Bioarchitecture. 2(6):189–199.
- Novikova IV, Hennelly SP, Sanbonmatsu KY. 2012b. Structural architecture of the human long non-coding RNA, steroid receptor RNA activator. Nucleic Acids Res. 40(11):5034–5051.
- Ottinger LM, Tullius TD. 2000. High-resolution in vivo footprinting of a protein − DNA complex using γ-radiation. J Am Chem Soc. 122(24):5901–5902.
- Palangat M, Meier TI, Keene RG, Landick R. 1998. Transcriptional pausing at +62 of the HIV-1 nascent RNA modulates formation of the TAR RNA structure. Mol Cell. 1(7):1033–1042.
- Palovcak E, Wang F, Zheng SQ, Yu Z, Li S, Betegon M, Bulkley D, Agard DA, Cheng Y. 2018. A simple and robust procedure for preparing graphene-oxide cryo-EM grids. J Struct Biol. 204(1):80–84.
- Parisien M, Major F. 2008. The MC-Fold and MC-Sym pipeline infers RNA structure from sequence data. Nature. 452(7183):51–55.
- Patel TR, Chojnowski G, Astha Koul A, McKenna SA, Bujnicki JM. 2017. Structural studies of RNA-protein complexes: a hybrid approach involving hydrodynamics, scattering, and computational methods. Methods. 118-119:146–162.
- Perard J, Leyrat C, Baudin F, Drouet E, Jamin M. 2013. Structure of the full-length HCV IRES in solution. Nat Commun. 4(1):1612.
- Pereira MJ, Behera V, Walter NG. 2010. Nondenaturing purification of co-transcriptionally folded RNA avoids common folding heterogeneity. PloS One. 5(9):e12953.
- Petrone J, DeFrancesco L. 2018. Small molecules get the message. Nat Biotechnol. 36(9):787–790.
- Pirakitikulr N, Kohlway A, Lindenbach BD, Pyle AM. 2016. The coding region of the HCV genome contains a network of regulatory RNA structures. Mol Cell. 62(1):111–120.
- Popenda M, Szachniuk M, Antczak M, Purzycka KJ, Lukasiak P, Bartol N, Blazewicz J, Adamiak RW. 2012. Automated 3D structure composition for large RNAs. Nucleic Acids Res. 40(14):e112.
- Pyle AM. 2010. The tertiary structure of group II introns: implications for biological function and evolution. Crit Rev Biochem Mol Biol. 45(3):215–232.
- Pyle AM. 2014. Looking at LncRNAs with the ribozyme toolkit. Mol Cell. 56(1):13–17.
- Pyle AM. 2016. Group II intron self-splicing. Annu Rev Biophys. 45:183–205.
- Quinodoz S, Guttman M. 2014. Long noncoding RNAs: an emerging link between gene regulation and nuclear organization. Trends Cell Biol. 24(11):651–663.
- Reeder J, Giegerich R. 2004. Design, implementation and evaluation of a practical pseudoknot folding algorithm based on thermodynamics. BMC Bioinformatics. 5:104.
- Reuter JS, Mathews DH. 2010. RNAstructure: software for RNA secondary structure prediction and analysis. BMC Bioinformatics. 11(1):129.
- Rice GM, Leonard CW, Weeks KM. 2014. RNA secondary structure modeling at consistent high accuracy using differential SHAPE. RNA. 20(6):846–854.
- Rice P, Longden I, Bleasby A. 2000. EMBOSS: the European molecular biology open software suite. Trends Genet. 16(6):276–277.
- Rinn JL, Chang HY. 2012. Genome regulation by long noncoding RNAs. Annu Rev Biochem. 81:145–166.
- Rinn JL, Kertesz M, Wang JK, Squazzo SL, Xu X, Brugmann SA, Goodnough LH, Helms JA, Farnham PJ, Segal E, et al. 2007. Functional demarcation of active and silent chromatin domains in human HOX loci by noncoding RNAs. Cell. 129(7):1311–1323.
- Rivas E, Clements J, Eddy SR. 2017. A statistical test for conserved RNA structure shows lack of evidence for structure in lncRNAs. Nat Methods. 14(1):45–48.
- Ruschak AM, Mathews DH, Bibillo A, Spinelli SL, Childs JL, Eickbush TH, Turner DH. 2004. Secondary structure models of the 3' untranslated regions of diverse R2 RNAs. RNA. 10(6):978–987.
- Said N, Rieder R, Hurwitz R, Deckert J, Urlaub H, Vogel J. 2009. In vivo expression and purification of aptamer-tagged small RNA regulators. Nucleic Acids Res. 37(20):e133.
- Sarropoulos I, Marin R, Cardoso-Moreira M, Kaessmann H. 2019. Developmental dynamics of lncRNAs across mammalian organs and species. Nature. 571(7766):510–514.
- Sato K, Kato Y, Hamada M, Akutsu T, Asai K. 2011. IPknot: fast and accurate prediction of RNA secondary structures with pseudoknots using integer programming. Bioinformatics. 27(13):i85–93.
- Sauvageau M, Goff LA, Lodato S, Bonev B, Groff AF, Gerhardinger C, Sanchez-Gomez DB, Hacisuleyman E, Li E, Spence M, et al. 2013. Multiple knockout mouse models reveal lincRNAs are required for life and brain development. Elife. 2:e01749.
- Schmidli C, Albiez S, Rima L, Righetto R, Mohammed I, Oliva P, Kovacik L, Stahlberg H, Braun T. 2019. Microfluidic protein isolation and sample preparation for high-resolution cryo-EM. Proc Natl Acad Sci USA. 116(30):15007–15012.
- Sharon D, Tilgner H, Grubert F, Snyder M. 2013. A single-molecule long-read survey of the human transcriptome. Nat Biotechnol. 31(11):1009–1014.
- Sharp JS, Becker JM, Hettich RL. 2004. Analysis of protein solvent accessible surfaces by photochemical oxidation and mass spectrometry. Anal Chem. 76(3):672–683.
- Shcherbakova I, Mitra S. 2009. Hydroxyl-radical footprinting to probe equilibrium changes in RNA tertiary structure. Meth Enzymol. 468:31–46.
- Shcherbakova I, Mitra S, Beer RH, Brenowitz M. 2006. Fast Fenton footprinting: a laboratory-based method for the time-resolved analysis of DNA, RNA and proteins. Nucleic Acids Res. 34(6):e48.
- Sherpa C, Rausch JW, Le Grice SF. 2018. Structural characterization of maternally expressed gene 3 RNA reveals conserved motifs and potential sites of interaction with polycomb repressive complex 2. Nucleic Acids Res. 46(19):10432–10447.
- Siegfried NA, Busan S, Rice GM, Nelson JA, Weeks KM. 2014. RNA motif discovery by SHAPE and mutational profiling (SHAPE-MaP). Nat Methods. 11(9):959–965.
- Simon LM, Morandi E, Luganini A, Gribaudo G, Martinez-Sobrido L, Turner DH, Oliviero S, Incarnato D. 2019. In vivo analysis of influenza A mRNA secondary structures identifies critical regulatory motifs. Nucleic Acids Res. 47(13):7003–7017.
- Simon MD, Wang CI, Kharchenko PV, West JA, Chapman BA, Alekseyenko AA, Borowsky ML, Kuroda MI, Kingston RE. 2011. The genomic binding sites of a noncoding RNA. Proc Natl Acad Sci USA. 108(51):20497–20502.
- Singh J, Hanson J, Paliwal K, Zhou Y. 2019. RNA secondary structure prediction using an ensemble of two-dimensional deep neural networks and transfer learning. Nat Commun. 10(1):5407.
- Sloma MF, Mathews DH. 2017. Base pair probability estimates improve the prediction accuracy of RNA non-canonical base pairs. PLoS Comput Biol. 13(11):e1005827.
- Smith AM, Jain M, Mulroney L, Garalde DR, Akeson M. 2019. Reading canonical and modified nucleobases in 16S ribosomal RNA using nanopore native RNA sequencing. PloS One. 14(5):e0216709.
- Smola MJ, Calabrese JM, Weeks KM. 2015. Detection of RNA-protein interactions in living cells with SHAPE. Biochemistry. 54(46):6867–6875.
- Smola MJ, Christy TW, Inoue K, Nicholson CO, Friedersdorf M, Keene JD, Lee DM, Calabrese JM, Weeks KM. 2016. SHAPE reveals transcript-wide interactions, complex structural domains, and protein interactions across the Xist lncRNA in living cells. Proc Natl Acad Sci USA. 113(37):10322–10327.
- Somarowthu S, Legiewicz M, Chillon I, Marcia M, Liu F, Pyle AM. 2015. HOTAIR forms an intricate and modular secondary structure. Mol Cell. 58(2):353–361.
- Spitale RC, Flynn RA, Zhang QC, Crisalli P, Lee B, Jung J-W, Kuchelmeister HY, Batista PJ, Torre EA, Kool ET, et al. 2015. Structural imprints in vivo decode RNA regulatory mechanisms. Nature. 519(7544):486–490.
- Spokoini-Stern R, Stamov D, Jessel H, Aharoni L, Haschke H, Giron J, Unger R, Segal E, Abu-Horowitz A, Bachelet I. 2020. Visualizing the structure and motion of the long noncoding RNA HOTAIR. RNA. 26(5):629–636.
- Steen KA, Rice GM, Weeks KM. 2012. Fingerprinting noncanonical and tertiary RNA structures by differential SHAPE reactivity. J Am Chem Soc. 134(32):13160–13163.
- Su L, Chen L, Egli M, Berger JM, Rich A. 1999. Minor groove RNA triplex in the crystal structure of a ribosomal frameshifting viral pseudoknot. Nat Struct Biol. 6(3):285–292.
- Sunwoo H, Dinger ME, Wilusz JE, Amaral PP, Mattick JS, Spector DL. 2008. MEN epsilon/beta nuclear-retained non-coding RNAs are up-regulated upon muscle differentiation and are essential components of paraspeckles. Genome Res. 19(3):347–359.
- Swisher J, Duarte CM, Su LJ, Pyle AM. 2001. Visualizing the solvent-inaccessible core of a group II intron ribozyme. Embo J. 20(8):2051–2061.
- Swisher JF, Su LJ, Brenowitz M, Anderson VE, Pyle AM. 2002. Productive folding to the native state by a group II intron ribozyme. J Mol Biol. 315(3):297–310.
- Takahashi MK, Watters KE, Gasper PM, Abbott TR, Carlson PD, Chen AA, Lucks JB. 2016. Using in-cell SHAPE-Seq and simulations to probe structure-function design principles of RNA transcriptional regulators. RNA. 22(6):920–933.
- Tani H, Mizutani R, Salam KA, Tano K, Ijiri K, Wakamatsu A, Isogai T, Suzuki Y, Akimitsu N. 2012. Genome-wide determination of RNA stability reveals hundreds of short-lived noncoding transcripts in mammals. Genome Res. 22(5):947–956.
- Tavares RCA, Pyle AM, Somarowthu S. 2019. Phylogenetic analysis with improved parameters reveals conservation in lncRNA structures. J Mol Biol. 431(8):1592–1603.
- Tomezsko PJ, Corbin VDA, Gupta P, Swaminathan H, Glasgow M, Persad S, Edwards MD, McIntosh L, Papenfuss AT, Emery A, et al. 2020. Determination of RNA structural diversity and its role in HIV-1 RNA splicing. Nature. 582(7812):438–442.
- Toor N, Keating KS, Taylor SD, Pyle AM. 2008. Crystal structure of a self-spliced group II intron. Science. 320(5872):77–82.
- Trewhella J. 2016. Small-angle scattering and 3D structure interpretation. Curr Opin Struct Biol. 40:1–7.
- Trinh MH, Odorico M, Pique ME, Teulon JM, Roberts VAT, Eyck LF, Getzoff ED, Parot P, Chen SW, Pellequer JL. 2012. Computational reconstruction of multidomain proteins using atomic force microscopy data. Structure. 20(1):113–120.
- Tripathi V, Ellis JD, Shen Z, Song DY, Pan Q, Watt AT, Freier SM, Bennett CF, Sharma A, Bubulya PA, et al. 2010. The nuclear-retained noncoding RNA MALAT1 regulates alternative splicing by modulating SR splicing factor phosphorylation. Mol Cell. 39(6):925–938.
- Tsai MC, Manor O, Wan Y, Mosammaparast N, Wang JK, Lan F, Shi Y, Segal E, Chang HY. 2010. Long noncoding RNA as modular scaffold of histone modification complexes. Science. 329(5992):689–693.
- Ulitsky I, Bartel DP. 2013. lincRNAs: genomics, evolution, and mechanisms. Cell. 154(1):26–46.
- Uroda T, Anastasakou E, Rossi A, Teulon JM, Pellequer JL, Annibale P, Pessey O, Inga A, Chillon I, Marcia M. 2019. Conserved pseudoknots in lncRNA MEG3 are essential for stimulation of the p53 pathway. Mol Cell. 75(5):982–995.
- Uroda T, Chillon I, Annibale P, Teulon JM, Pessey O, Karuppasamy M, Pellequer JL, Marcia M. 2020. Visualizing the functional 3D shape and topography of long noncoding RNAs by single-particle atomic force microscopy and in-solution hydrodynamic techniques. Nat Protoc. 15(6):2107–2139.
- van der Lee R, Buljan M, Lang B, Weatheritt RJ, Daughdrill GW, Dunker AK, Fuxreiter M, Gough J, Gsponer J, Jones DT, et al. 2014. Classification of intrinsically disordered regions and proteins. Chem Rev. 114(13):6589–6631.
- Vasa SM, Guex N, Wilkinson KA, Weeks KM, Giddings MC. 2008. ShapeFinder: a software system for high-throughput quantitative analysis of nucleic acid reactivity information resolved by capillary electrophoresis. RNA. 14(10):1979–1990.
- Vaziri S, Koehl P, Aviran S. 2018. Extracting information from RNA SHAPE data: Kalman filtering approach. PloS One. 13(11):e0207029.
- Volders PJ, Anckaert J, Verheggen K, Nuytens J, Martens L, Mestdagh P, Vandesompele J. 2019. LNCipedia 5: towards a reference set of human long non-coding RNAs. Nucleic Acids Res. 47(D1):D135–D139.
- Waldsich C, Pyle AM. 2008. A kinetic intermediate that regulates proper folding of a group II intron RNA. J Mol Biol. 375(2):572–580.
- Walters RD, Kugel JF, Goodrich JA. 2009. InvAluable junk: the cellular impact and function of Alu and B2 RNAs. IUBMB Life. 61(8):831–837.
- Wan Y, Qu K, Ouyang Z, Kertesz M, Li J, Tibshirani R, Makino DL, Nutter RC, Segal E, Chang HY. 2012. Genome-wide measurement of RNA folding energies. Mol Cell. 48(2):169–181.
- Wang X, Goodrich KJ, Gooding AR, Naeem H, Archer S, Paucek RD, Youmans DT, Cech TR, Davidovich C. 2017. Targeting of polycomb repressive complex 2 to RNA by short repeats of consecutive guanines. Mol Cell. 65(6):1056–1067.
- Wapinski O, Chang HY. 2011. Long noncoding RNAs and human disease. Trends Cell Biol. 21(6):354–361.
- Watkins AM, Rangan R, Das R. 2020. FARFAR2: improved de novo rosetta prediction of complex global RNA folds. Structure. 28(8):963–976.
- Watters KE, Strobel EJ, Yu AM, Lis JT, Lucks JB. 2016. Cotranscriptional folding of a riboswitch at nucleotide resolution. Nat Struct Mol Biol. 23(12):1124–1131.
- Watts JM, Dang KK, Gorelick RJ, Leonard CW, Bess JW, Jr., Swanstrom R, Burch CL, Weeks KM. 2009. Architecture and secondary structure of an entire HIV-1 RNA genome. Nature. 460(7256):711–716.
- Weakley SM, Wang H, Yao Q, Chen C. 2011. Expression and function of a large non-coding RNA gene XIST in human cancer. World J Surg. 35(8):1751–1756.
- Weeks KM, Mauger DM. 2011. Exploring RNA structural codes with SHAPE chemistry. Acc Chem Res. 44(12):1280–1291.
- Weinberg Z, Breaker RR. 2011. R2R--software to speed the depiction of aesthetic consensus RNA secondary structures. BMC Bioinformatics. 12:3.
- Weinberg Z, Perreault J, Meyer MM, Breaker RR. 2009. Exceptional structured noncoding RNAs revealed by bacterial metagenome analysis. Nature. 462(7273):656–659.
- Weinberg Z, Wang JX, Bogue J, Yang J, Corbino K, Moy RH, Breaker RR. 2010. Comparative genomics reveals 104 candidate structured RNAs from bacteria, archaea, and their metagenomes. Genome Biol. 11(3):R31.
- Weldon C, Behm-Ansmant I, Hurley LH, Burley GA, Branlant C, Eperon IC, Dominguez C. 2017. Identification of G-quadruplexes in long functional RNAs using 7-deazaguanine RNA. Nat Chem Biol. 13(1):18–20.
- Wilusz JE, Freier SM, Spector DL. 2008. 3' end processing of a long nuclear-retained noncoding RNA yields a tRNA-like cytoplasmic RNA. Cell. 135(5):919–932.
- Wilusz JE, JnBaptiste CK, Lu LY, Kuhn CD, Joshua-Tor L, Sharp PA. 2012. A triple helix stabilizes the 3' ends of long noncoding RNAs that lack poly(A) tails. Genes Dev. 26(21):2392–2407.
- Wiryaman T, Toor N. 2017. Structure determination of group II introns. Methods (San Diego, Calif. 125:10–15.
- Woodson SA, Deras ML, Brenowitz M. 2001. Time-resolved hydroxyl radical footprinting of RNA with X-rays. In: Serge L Beaucage, et al., editors. Current protocols in nucleic acid chemistry. Hoboken (NJ): John Wiley & Sons, Inc, Chapter 11:Unit 11 16. DOI: 10.1002/0471142700.nc1106s06
- Wu L, Murat P, Matak-Vinkovic D, Murrell A, Balasubramanian S. 2013. Binding interactions between long noncoding RNA HOTAIR and PRC2 proteins. Biochemistry. 52(52):9519–9527.
- Wutz A, Rasmussen TP, Jaenisch R. 2002. Chromosomal silencing and localization are mediated by different domains of Xist RNA. Nat Genet. 30(2):167–174.
- Xu Y, Kaminaga K, Komiyama M. 2008. G-quadruplex formation by human telomeric repeats-containing RNA in Na + solution. J Am Chem Soc. 130(33):11179–11184.
- Xue Z, Hennelly S, Doyle B, Gulati AA, Novikova IV, Sanbonmatsu KY, Boyer LA. 2016. A G-Rich motif in the lncRNA Braveheart interacts with a zinc-finger transcription factor to specify the cardiovascular lineage. Mol Cell. 64(1):37–50.
- Yang D, Liu P, Wudeck EV, Giedroc DP, Leibowitz JL. 2015. SHAPE analysis of the RNA secondary structure of the Mouse Hepatitis Virus 5' untranslated region and N-terminal nsp1 coding sequences. Virology. 475:15–27.
- Yang F, Zhang H, Mei Y, Wu M. 2014. Reciprocal regulation of HIF-1alpha and lincRNA-p21 modulates the Warburg effect. Mol Cell. 53(1):88–100.
- Yang SY, Lejault P, Chevrier S, Boidot R, Robertson AG, Wong JMY, Monchaud D. 2018. Transcriptome-wide identification of transient RNA G-quadruplexes in human cells. Nat Commun. 9(1):4730.
- Yesselman JD, Eiler D, Carlson ED, Gotrik MR, d'Aquino AE, Ooms AN, Kladwang W, Carlson PD, Shi X, Costantino DA, et al. 2019. Computational design of three-dimensional RNA structure and function. Nat Nanotechnol. 14(9):866–873.
- Yin Y, Lu JY, Zhang X, Shao W, Xu Y, Li P, Hong Y, Cui L, Shan G, Tian B, et al. 2020. U1 snRNP regulates chromatin retention of noncoding RNAs. Nature. 580(7801):147–150.
- Yu J, Liu Z, Jiang W, Wang G, Mao C. 2015. De novo design of an RNA tile that self-assembles into a homo-octameric nanoprism. Nat Commun. 6(1):5724.
- Zappulla DC, Cech TR. 2004. Yeast telomerase RNA: a flexible scaffold for protein subunits. Proc Natl Acad Sci USA. 101(27):10024–10029.
- Zhang B, Gunawardane L, Niazi F, Jahanbani F, Chen X, Valadkhan S. 2014. A novel RNA motif mediates the strict nuclear localization of a long noncoding RNA. Mol Cell Biol. 34(12):2318–2329.
- Zhang K, Li S, Kappel K, Pintilie G, Su Z, Mou TC, Schmid MF, Das R, Chiu W. 2019. Cryo-EM structure of a 40 kDa SAM-IV riboswitch RNA at 3.7 A resolution. Nat Commun. 10(1):5511.
- Zhang X, Zhou Y, Mehta KR, Danila DC, Scolavino S, Johnson SR, Klibanski A. 2003. A pituitary-derived MEG3 isoform functions as a growth suppressor in tumor cells. J Clin Endocrinol Metab. 88(11):5119–5126.
- Zhao C, Rajashankar KR, Marcia M, Pyle AM. 2015. Crystal structure of group II intron domain 1 reveals a template for RNA assembly. Nat Chem Biol. 11(12):967–972.
- Zhao J, Sun BK, Erwin JA, Song JJ, Lee JT. 2008. Polycomb proteins targeted by a short repeat RNA to the mouse X chromosome. Science. 322(5902):750–756.
- Zhao Y, Wang J, Zeng C, Xiao Y. 2018. Evaluation of RNA secondary structure prediction for both base-pairing and topology. Biophys Rep. 4(3):123–132.
- Zhou Y, Zhong Y, Wang Y, Zhang X, Batista DL, Gejman R, Ansell PJ, Zhao J, Weng C, Klibanski A. 2007. Activation of p53 by MEG3 non-coding RNA. J Biol Chem. 282(34):24731–24742.