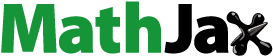
Abstract
Logistics performance is considered an important determinant of supply chain performance that results in carbon neutrality. The main aim of this study is to investigate the influence of logistics performance and green innovation on carbon emissions for selected Asian economies over the period 2007–2020. The 2SLS approach has been employed for empirical tasks, and the robustness of results has been confirmed through the GMM approach. The results indicate that logistics performance significantly reduces carbon emissions. Besides the logistics performance index, five other indicators of logistics performance have been used in the analysis. These indicators include quality of logistics services, tracking and tracing capability, logistics infrastructure quality, cargo transportation timelines, and customs clearance efficiency. All these indicators are negatively correlated with carbon emissions. The results further indicate that green innovation leads to a significant reduction in carbon emissions. Thus, our results emphasize the priorities of green innovation, logistics performance, and environmental sustainability in Asian economies.
1. Introduction
Climate change and global warming indicate market failure that the world has never seen before and pose significant threats to the sustainable future of the world (Yang et al., Citation2020). Underdeveloped and poor countries have to bear the extra cost in the form of adverse impacts of environmental degradation, which can slow the pace of their development process. There are various channels through which climate change can slow down the economic growth of a nation, including low industrial output, damages caused by the rising temperature, high sea level, melting glaciers, storms, droughts, and the issue of refugees (Ullah et al., Citation2020). One of the main culprits in climate change and global warming is the emissions of greenhouse gasses (GHGs). The most dominant greenhouse gas is CO2 emissions because its share is 70% of total GHGs emissions (Ullah et al., Citation2021). Anthropogenic activities are mainly responsible for the emissions of CO2 in several ways. In pursuance of economic goals, various sectors work together, such as power generation, industrial, real state, and transportation, which all require the combustion of fossil fuels and consequently pollute the environment. In recent times, policymakers have started to emphasize the environmental impacts of economic growth (Sun et al., Citation2022). This has induced policymakers to shift their focus from the traditional concept of growth to a more environmentally friendly one, which is more sustainable (Usman et al., Citation2021).
To date, empirics have investigated various determinants of environmental quality. However, there is insufficient evidence of how green logistics impact environmental quality. Logistics means the significant function of all firms and businesses, extending from manufacturing to service, public to private. This is an era of globalization, and countries depend on each other for their needs; hence, countries from different regions are involved in a trade. Therefore, the demand and significance of logistics have increased manifold due to its contribution to trade and the development of the economies (Rezaei et al., Citation2018; Sohail et al., Citation2021). If the countries fully conform to the principles of globalization, their logistics performance can be upgraded in the true sense. In this regard, an index was needed to measure and compare the logistics performance in various countries. The World Bank fulfilled this demand in 2007 by providing an index known as the logistics performance index (LPI) that can estimate the difference in the logistics performance of the countries (Khan et al., Citation2018; Rezaei et al., Citation2018).
On one side, the role of logistics is very significant in shaping the economic development of the nations; on the other side, it has a crucial impact on the ecological balance and other social issues. The logistics industry is among the largest consumer of energy resources and one of the major contributors to CO2 emissions (Rashidi & Cullinane, Citation2019). To keep logistics-driven pollution under control, we need an efficient environmental management structure to keep the environment healthy and green. An increase in globalization cause the logistics industry to become global (Sharma et al., Citation2021), which helps increase the volume of trade and, consequently, the CO2 emissions. Therefore, the pressure is rising on the logistics industry to improve its carbon management structure which is necessary to increase the efficiency of logistics activities. This will increase the pace of economic development and reduce its adverse environmental impact (Chien et al., Citation2021; Herold & Lee, Citation2017).
As a result, the concept of green logistics emerged, which refers to the set of policies and strategies to reduce the environmental damages caused by business-related activities. This logistics concept has transformed many things in the logistics industry, such as the formation of procedures, transport structure, supply chain, and storage areas (Karaman et al., Citation2020). There is a significant difference between the traditional approach to logistics and the approach adopted by green logistics. In the conventional approach, the main focal point is how to improve logistics performance and operation without giving importance to environmental sustainability. On the other side, green logistics also cares about the environment and focuses on maintaining the balance between the economy and ecology (Khan et al., Citation2019).
In addition to green logistics, another important aspect that has helped the economies to attain a sustainable environment is the development of green technologies. According to Li et al. (Citation2022) improving technical efficiency is crucial for long-term and effective control of CO2 emissions. The available literature in this area also supports the idea that green technological innovation is crucial for CO2 reduction (Lin & Zhu, Citation2019; Ullah et al., Citation2020; Wang & Wei, Citation2020; Wei & Ullah, Citation2022). Green technologies when supported by renewable energy technologies become much more effective in controlling the damage of air pollution by curbing CO2 emissions (Chen & Lee, Citation2020; Usman et al., Citation2021). Because renewable technologies and green technologies are crucial for producing clean energy, which put less burden on the environment (Lin & Ma, Citation2022; Wang & Zhu, Citation2020). Thus green technological innovations are considered crucial in reducing the energy demand and improving energy efficiency (Chien et al., Citation2022; Yu & Du, Citation2019).
Our study will contribute to pertaining literature in the following manners. This study aims to investigate the impact of green logistics and green technologies on CO2 emissions in Asia over the period 2007–2020. To the best of our knowledge, this is the first-ever study that has focused on the nexus between green logistics, green innovations, and CO2 emissions in Asian economies. The study discusses possible transmission channels through which green logistics and green innovation help in the attainment of environmental sustainability. Moreover, the selection of the countries is unique and has not been previously used in the context of said relationship. The study also contributes from a methodological perspective, for instance, the econometric methods used for empirical analysis are 2SLS and GMM which can deal with dynamic panel data, which is the third major contribution of the study. Though the data set is small but pooling them together provides more information and observation; thus, the estimates are efficient. Moreover, the study provides important policy directions for environmental sustainability, green logistics, and environmental technology development.
2. Model and methods
The primary objective of the study is to examine the link between CO2 emissions and logistics performance in Asian countries. Some studies have recorded the impact of logistic performance on the international economy (Wong & Tang, Citation2018). For example, Martí et al. (Citation2014) investigated the impact of logistic performance on trade in emerging nations. Their results confirmed the positive impact of logistic performance on the trade of these economies. Similarly, Fernández et al. (Citation2020) presented a model that can calculate the rise in economic growth between 0.011% and 0.034 because of a 1% rise in the logistic performance, known as a dynamic global aggregate function. These empirical findings confirmed the positive impact of logistic performance on economic growth. As rising economic activities are the major cause of environmental degradation; therefore, we hypothesize that logistic performance also has a role in establishing environmental quality. Following the literature, we have developed the following model.
In the above model, CO2 is determined by logistic performance (LP), green innovation (GI), national income (GDP), energy consumption (EC), years of schooling (Education), trade openness (Trade), financial development (FD), country-specific effect (, and random error term (
. To represent logistic performance, we have used six different indicators, detail of which is provided in .
Table 1. Definitions and sources.
The data used in the analysis is based on panel settings. Panel data combines cross-sectional and time-series data and has many benefits over other forms of data. For instance, it increases the number of observations and provides more information and reliable estimates. Several techniques can handle the panel data settings, and the most common among them are fixed effect (FE), random effect (RE), 2SLS, and Generalized Methods of Moments (GMM). Fixed and Random Effect models have their pros and cons. For instance, FE is applicable for the type of sample, which is pre-determined; however, RE is applicable for the randomly selected model. Similarly, FE can’t include the variables that remain fixed across time. Conversely, the RE assumes that there is no correlation between country-specific effects and explanatory variables; otherwise, the RE provides biased estimates. Further, these techniques cannot handle the various problems of panel data, such as heteroskedasticity, endogeneity, and serial correlation. Therefore, for this analysis, we have relied on 2SLS and GMM techniques.
2SLS is a technique that is an extended version of OLS that can perform well in the case of simultaneous equations and provide consistent structural parameters. In this technique, we replace the endogenous independent variables with the same sort of variables known as independent proxy variables, but they do not correlate with residual terms. The 2SLS is a valuable addition to econometrics, but it is more likely to suffer from the problems of endogeneity and heteroscedasticity than GMM (Canh et al., Citation2019; Donou-Adonsou, Citation2019; Li et al., Citation2021). In such a situation, GMM proposed by Arellano and Bond (Citation1991), is more suitable for panel analysis and can counter the common issues of panel data analysis, including heteroskedasticity, endogeneity, and serial correlation. Mainly when the number of cross-sections (N) is greater than the number of time periods (T), GMM is a pretty good technique. In the GMM estimation technique, we must include the lagged dependent variable that is crucial for countering the issue of endogeneity. Two kinds of GMM estimator are available, known as one-step and two-step GMM. In the case of unbalanced data, a two-step GMM is a better estimator because it provides consistent estimates. On the other side, one-step GMM lost many observations in the case of unbalanced data and provided biased estimates (Khan et al., Citation2019). Likewise, two-step GMM performs better in the presence of autocorrelation and heteroscedasticity problems (Chen et al., Citation2022).
3. Data
Our study is exploring the effect of logistics performance and green innovation on carbon neutrality over the period 2007–2020. The details about variables used in the analysis, their symbols, definitions, and sources of data are given in . CO2 emissions have been used to measure the extent of carbon neutrality. Total greenhouse gas emissions is also used as a dependent variable to confirm the robustness of the results. Logistics performance is measured at aggregate and disaggregates levels. At the aggregate level, it is measured through logistics performance index. However, it is measured through five indicators at disaggregated levels namely, quality of logistics services, tracking and tracing capability, logistics infrastructure quality, cargo transportation timelines, and customs clearance efficiency. Patent applications are used to measure green innovation. The study gets assistance from previous literature for the selection of control variables. Various studies highlighted that GDP per capita, energy consumption, and trade openness are important determinants of carbon emissions (Sharma, Citation2011; Usman et al., Citation2021). Li et al. (Citation2022) highlighted the importance of educational attainment and financial development for carbon neutrality. Thus, the study added these determinants as control variables in the model. The required data for empirical analysis have been obtained from the World Development Indicators (WDI) published by the World Bank. The descriptive statistics of each variable are given in . Moreover, shows a higher correlation among LPI, LIQ, QLS, TTC, CTT, and CCE. This high correlation does not infer the existence of correlation among these variables because LIQ, QLS, TTC, CTT, and CCE are 5 different dimensions of logistics performance, which are regressed in separate models.
Table 2. Descriptive statistics.
Table 3. Correlation matrix.
4. Results and discussion
The 2SLS approach is used to find out the impact of green innovation and logistics performance on carbon neutrality and the results are reported in . Six independent 2SLS models have been regressed based on the logistics performance index and the other five indicators of logistics performance. It is reported that green innovation impact on CO2 emissions is found significantly negative in all six models, describing that increases in green innovation help in achieving carbon neutrality in selected Asian economies. In précised manner, it reveals that 1% upsurge in green innovation reduces carbon emissions by 0.162% in model 1, 0.192% in model 2, 0.112% in model 3, 0.171% in model 4, 0.234% in model 5, and 0.233% in model 6. The association between logistics performance index and CO2 emissions is found significantly negative in model 1, displaying that a 1% improvement in logistics performance index tends to reduce CO2 emissions by 1.620% in selected Asian economies. The quality of logistics services (QLS) do not produce any significant impact on carbon emissions in selected Asian economies as displayed in model 2. Tracking and tracing capability (TTC) variables report a significant and negative association with CO2 emissions in model 3, describing that as tracking and tracing capability of selected Asian economies increases, carbon emissions is significantly reduced. It infers that a 1% enhancement in tracking and tracing capability results in reducing CO2 emissions by 0.662% in selected Asian economies.
Table 4. Estimates of CO2 emissions (2SLS).
The association between logistics infrastructure quality (LIQ) and CO2 emissions is found significantly negative in model 4, revealing that a 1% improvement in logistics infrastructure quality tends to reduce CO2 emissions by 1.270% in selected Asian economies. Cargo transportation timelines (CTT) variables report a significant and negative impact on CO2 emissions in model 5, describing that as cargo transportation timelines of selected Asian economies increase, carbon emissions decline. It displays that a 1% enhancement in cargo transportation timelines results in reducing CO2 emissions by 0.841% in selected Asian economies. The correlation between customs clearance efficiency and CO2 emissions is reported significantly negative in model 6, revealing that a 1% improvement in customs clearance efficiency tends to reduce CO2 emissions by 1.518% in selected Asian economies.
The correlation between GDP per capita and CO2 emissions is observed significantly positively in three models denoting that increase in GDP acts as a determining factor of pollution emissions in selected Asian economies. Energy use also contributes significantly in increasing carbon emissions in selected economies as confirmed through a positive association between energy consumption and CO2 emissions in three models. In contrast, trade openness and financial development variables are insignificantly associated with carbon emissions in selected Asian economies. The relationship between education attainment and carbon emissions is reported significantly negative in two models in selected samples of economies.
To confirm the robustness of the results, our study employed the GMM approach and the results are displayed in . The nexus between green innovation and CO2 emissions is reported significantly negative in all six models. It infers that 1% expansion in green innovation reduces carbon emissions by 00.118% in model 1, 0.117% in model 2 and model 3, 0.118% in model 4, 0.116% in model 5, and 0.118% in model 6. Our study reports a positive association between green innovation and carbon neutrality. This finding is supported by Tao et al. (Citation2021) and Ullah et al. (Citation2021), who stated that green innovation is required to enhance operational efficiency and environmental sustainability. It is argued in the literature that green innovation helps economies in switching towards advanced and new methods of energy generation and conservation that help in the reduction of carbon emissions. Sun et al. (Citation2021) denoted that green innovation helps in the reduction of carbon assimilation and environmental degradation. Green innovation initiates a revolution in every segment of the economy that leads to improvement in environmental sustainability. Moreover, green innovation stimulates economies to invest in eco-friendly technologies that are conducive to environmental sustainability.
Table 5. Estimates of CO2 emissions (GMM).
The relationship between the logistics performance index and CO2 emissions is reported as significantly negative in model 1, revealing that a 1% enhancement in logistics performance index reduces CO2 emissions by 1.006% in selected Asian economies. The quality of logistics services (QLS) produces a significant and negative impact on carbon emissions in selected Asian economies as displayed in model 2. A 1% rise in quality of logistics services reduces CO2 emissions by 1.002% in selected Asian economies. Tracking and tracing capability (TTC) produces a significant and negative impact on CO2 emissions as displayed in model 3, describing that a 1% improvement in tracking and tracing capability results in reducing CO2 emissions by 1.006% in selected Asian economies. The connotation between logistics infrastructure quality (LIQ) and CO2 emissions is reported significantly negative in model 4, revealing that a 1% development in logistics infrastructure quality tends to reduce CO2 emissions by 1.015% in selected Asian economies. Cargo transportation timelines (CTT) bring a significant and negative reduction in CO2 emissions as reported in model 5, describing that a 1% enhancement in cargo transportation timelines reduces CO2 emissions by 1.010% in selected Asian economies. The relationship between customs clearance efficiency (CCE) and CO2 emissions is reported significantly negative in model 6, showing that a 1% enhancement in customs clearance efficiency tends to reduce CO2 emissions by 1.013% in selected Asian economies.
Logistics infrastructure is a fundamental determinant of the supply chain. Thus, the improvement of the customs clearance efficiency, logistics services quality, and improvement of transport infrastructure is beneficial for carbon neutrality and economic development. Our findings of the study are supported by the study done by Khan et al. (Citation2018), which denoted that environmental regulations should be imposed on polluting transportation and vehicles to enhance carbon neutrality. Additionally, Mohsin et al. (Citation2022) support our findings by arguing that the consumption of biofuels in vehicles can play a significant role in the reduction of carbon emissions and global warming. Meanwhile, green logistics practices help in the reduction of fuel consumption and carbon emissions that significantly improve environmental sustainability. Liu et al. (Citation2018) show that improvement in logistics performance leads to carbon neutrality and enhances economic performance as well. Our results are consistent with the results of Chen et al. (Citation2022), who denoted those green logistics negatively influence carbon emissions in the case of European countries. The robust model produces almost similar sizes of effects as shown in .
Table 6. Estimates of GHGs (GMM)-robustness.
5. Conclusion and implications
Over the past few decades, the world has witnessed a massive increase in business and trade activities. On one side, the increased business and trade activities improve the living standard of people globally. On the other side, due to the rise in economic activities, the world is suffering unprecedented climate change and global warming. The problem of environmental degradation has come into the limelight in the late 20th century, and it is still the most debated topic at international forums. In this regard, several international treaties such as Kyoto Protocol (1999), Paris Conference (2015), and United Nations Agenda (2030) demand the world community to reduce the amount of CO2 emissions, which is the main instigator of climate change. In this context, the empirics have investigated various determinants of CO2 emissions to reduce carbon emissions, e.g. green innovation, but the role of logistics as a determinant of CO2 emissions is not discussed in detail. In recent times, the importance of logistics has increased manifold due to globalization and rising trade and business relations among nations. Therefore, this study investigated the impact of logistic performance and green innovation on CO2 emissions in Asian economies.
For empirical analysis, we have applied 2SLS and GMM methods. The estimates attached to LPI are significant and negative with both 2SLS and GMM techniques, implying that an improvement in LPI reduces CO2 emissions. Similarly, the analysis has utilized several other proxies for logistic performance, including QLS, TTC, LIQ, CTT, and CCE. The estimates of all these variables are negatively significant, confirming that improved logistics performance improves the environmental quality in Asian economies by reducing CO2 emissions. Similarly, the estimated coefficient of GI is negatively significant in all models, irrespective of the estimation technique, confirming the mitigating effect of green innovations on CO2 emissions. The analysis also controls the effects of GDP, EC, Trade, Education, and FD on CO2 emissions. Among them, the estimated coefficients of GDP and EC are positively significant in almost all models, confirming that a rise in income and electricity consumption exacerbates the environmental problem.
Based on the above findings, we have provided some essential policy suggestions for the concerned stakeholders. Our results imply that improvement in logistics performance can improve environmental quality. Therefore, implementing green practices in the logistics industry may help improve the environment further. For example, introducing plastic-free packing material and the increased use of electric vehicles for delivery can significantly reduce CO2 emissions. The logistics supply chain process should be accomplished in an efficient manner that enhances the operational use of resources and energy. Policymakers and authorities should focus on reconciliation between sustainability and profitability targets of supply chain logistic performance. Additionally, special attention must be given to the timeliness and reliability of the shipment to preserve the environment. Moreover, the use of online catalogues instead of paper registers can reduce the rate of deforestation. Similarly, policymakers should increase the share of environment-related innovations by sponsoring research and development activities to improve environmental quality.
Some limitations are also there in this manuscript. Such as, the study is providing estimates for the Asian region at the aggregate level, however, economy-wise estimates can enhance the significance of this manuscript. Thus, it is suggested that future research should be done at a disaggregated level considering each economy separately. The disaggregated level analysis is required because results might change due to variations in environmental, social, economic, and cultural factors. Another limitation is that the present study is using GMM technique for regression purposes. It will be more appropriate if future studies use a more advanced approach like GMM-PVAR for efficient estimates.
Disclosure statement
No potential conflict of interest was reported by the authors.
References
- Arellano, M., & Bond, S. (1991). Some tests of specification for panel data: Monte Carlo evidence and an application to employment equations. The review of economic studies, 58(2), 277–297.
- Canh, N. P., Schinckus, C., & Thanh, S. D. (2019). Do economic openness and institutional quality influence patents? Evidence from GMM systems estimates. International Economics, 157, 134–169. https://doi.org/10.1016/j.inteco.2018.10.002
- Chen, Y., & Lee, C. C. (2020). Does technological innovation reduce CO2 emissions? Cross-country evidence. Journal of Cleaner Production, 263, 121550. https://doi.org/10.1016/j.jclepro.2020.121550
- Chen, M., Liu, Q., Huang, S., & Dang, C. (2022). Environmental cost control system of manufacturing enterprises using artificial intelligence based on value chain of circular economy. Enterprise Information Systems, 16(8–9), 1856422.
- Chien, F., Ajaz, T., Andlib, Z., Chau, K. Y., Ahmad, P., & Sharif, A. (2021). The role of technology innovation, renewable energy and globalization in reducing environmental degradation in Pakistan: A step towards sustainable environment. Renewable Energy, 177, 308–317. https://doi.org/10.1016/j.renene.2021.05.101
- Chien, F., Hsu, C. C., Andlib, Z., Shah, M. I., Ajaz, T., & Genie, M. G. (2022). The role of solar energy and eco‐innovation in reducing environmental degradation in China: Evidence from QARDL approach. Integrated Environmental Assessment and Management, 18(2), 555–571.
- Donou-Adonsou, F. (2019). Technology, education, and economic growth in Sub-Saharan Africa. Telecommunications Policy, 43(4), 353–360. https://doi.org/10.1016/j.telpol.2018.08.005
- Fernández, X. L., Paz-Saavedra, D., & Coto-Millán, P. (2020). The impact of Brexit on bank efficiency: Evidence from UK and Ireland. Finance Research Letters, 36, 101338.
- Herold, D. M., & Lee, K. H. (2017). Carbon management in the logistics and transportation sector: An overview and new research directions. Carbon Management, 8(1), 79–97. https://doi.org/10.1080/17583004.2017.1283923
- Karaman, A. S., Kilic, M., & Uyar, A. (2020). Green logistics performance and sustainability reporting practices of the logistics sector: The moderating effect of corporate governance. Journal of Cleaner Production, 258, 120718. https://doi.org/10.1016/j.jclepro.2020.120718
- Khan, S. A. R., Jian, C., Zhang, Y., Golpîra, H., Kumar, A., & Sharif, A. (2019). Environmental, social and economic growth indicators spur logistics performance: From the perspective of South Asian Association for Regional Cooperation countries. Journal of Cleaner Production, 214, 1011–1023. https://doi.org/10.1016/j.jclepro.2018.12.322
- Khan, S. A. R., Zhang, Y., Anees, M., Golpîra, H., Lahmar, A., & Qianli, D. (2018). Green supply chain management, economic growth and environment: A GMM based evidence. Journal of Cleaner Production, 185, 588–599. https://doi.org/10.1016/j.jclepro.2018.02.226
- Li, X., Ozturk, I., Ullah, S., Andlib, Z., & Hafeez, M. (2022). Can top-pollutant economies shift some burden through insurance sector development for sustainable development?. Economic Analysis and Policy, 74, 326–336.
- Lin, B., & Ma, R. (2022). Green technology innovations, urban innovation environment and CO2 emission reduction in China: Fresh evidence from a partially linear functional-coefficient panel model. Technological Forecasting and Social Change, 176, 121434. https://doi.org/10.1016/j.techfore.2021.121434
- Lin, B., & Zhu, J. (2019). Determinants of renewable energy technological innovation in China under CO2 emissions constraint. Journal of Environmental Management, 247, 662–671.
- Li, X., Shaikh, P. A., & Ullah, S. (2022). Exploring the potential role of higher education and ICT in China on green growth. Environmental Science and Pollution Research, 1–8.
- Li, X., Sohail, S., Majeed, M. T., & Ahmad, W. (2021). Green logistics, economic growth, and environmental quality: Evidence from one belt and road initiative economies. Environmental Science and Pollution Research International, 28(24), 30664–30674.
- Liu, J., Yuan, C., Hafeez, M., & Yuan, Q. (2018). The relationship between environment and logistics performance: Evidence from Asian countries. Journal of Cleaner Production, 204, 282–291. https://doi.org/10.1016/j.jclepro.2018.08.310
- Martí, L., Puertas, R., & García, L. (2014). The importance of the Logistics Performance Index in international trade. Applied Economics, 46(24), 2982–2992. https://doi.org/10.1080/00036846.2014.916394
- Mohsin, A. K. M., Tushar, H., Hossain, S. F. A., Chisty, K. K. S., Iqbal, M. M., Kamruzzaman, M., & Rahman, S. (2022). Green logistics and environment, economic growth in the context of the Belt and Road Initiative. Heliyon, 8(6), e09641. https://doi.org/10.1016/j.heliyon.2022.e09641
- Rashidi, K., & Cullinane, K. (2019). A comparison of fuzzy DEA and fuzzy TOPSIS in sustainable supplier selection: Implications for sourcing strategy. Expert Systems with Applications, 121, 266–281. https://doi.org/10.1016/j.eswa.2018.12.025
- Rezaei, J., van Roekel, W. S., & Tavasszy, L. (2018). Measuring the relative importance of the logistics performance index indicators using Best Worst Method. Transport Policy, 68, 158–169. https://doi.org/10.1016/j.tranpol.2018.05.007
- Sharma, S. S. (2011). Determinants of carbon dioxide emissions: Empirical evidence from 69 countries. Applied Energy, 88(1), 376–382. https://doi.org/10.1016/j.apenergy.2010.07.022
- Sharma, V. K., Sachdeva, A., & Singh, L. P. (2021). A meta analysis of sustainable supply chain management from different aspects. International Journal of Supply and Operations Management, 8(3), 289–313.
- Sohail, M. T., Ullah, S., Majeed, M. T., & Usman, A. (2021). Pakistan management of green transportation and environmental pollution: A nonlinear ARDL analysis. Environmental Science and Pollution Research International, 28(23), 29046–29055.
- Sun, Y., Li, H., Andlib, Z., & Genie, M. G. (2022). How do renewable energy and urbanization cause carbon emissions? Evidence from advanced panel estimation techniques. Renewable Energy. 185, 996–1005. https://doi.org/10.1016/j.renene.2021.12.112
- Sun, Y., Yesilada, F., Andlib, Z., & Ajaz, T. (2021). The role of eco-innovation and globalization towards carbon neutrality in the USA. Journal of Environmental Management, 299, 113568.
- Tao, R., Umar, M., Naseer, A., & Razi, U. (2021). The dynamic effect of eco-innovation and environmental taxes on carbon neutrality target in emerging seven (E7) economies. Journal of Environmental Management, 299, 113525.
- Ullah, S., Ahmad, W., Majeed, M. T., & Sohail, S. (2021). Asymmetric effects of premature deagriculturalization on economic growth and CO2 emissions: Fresh evidence from Pakistan. Environmental Science and Pollution Research International, 28(47), 66772–66786.
- Ullah, S., Ozturk, I., Usman, A., Majeed, M. T., & Akhtar, P. (2020). On the asymmetric effects of premature deindustrialization on CO2 emissions: Evidence from Pakistan. Environmental Science and Pollution Research International, 27(12), 13692–13702.
- Usman, A., Ozturk, I., Hassan, A., Zafar, S. M., & Ullah, S. (2021). The effect of ICT on energy consumption and economic growth in South Asian economies: An empirical analysis. Telematics and Informatics, 58, 101537. https://doi.org/10.1016/j.tele.2020.101537
- Usman, A., Ozturk, I., Ullah, S., & Hassan, A. (2021). Does ICT have symmetric or asymmetric effects on CO2 emissions? Evidence from selected Asian economies. Technology in Society, 67, 101692. https://doi.org/10.1016/j.techsoc.2021.101692
- Wang, H., & Wei, W. (2020). Coordinating technological progress and environmental regulation in CO2 mitigation: The optimal levels for OECD countries & emerging economies. Energy Economics, 87, 104510. https://doi.org/10.1016/j.eneco.2019.104510
- Wang, Z., & Zhu, Y. (2020). Do energy technology innovations contribute to CO2 emissions abatement? A spatial perspective. The Science of the Total Environment, 726, 138574.
- Wei, L., & Ullah, S. (2022). International tourism, digital infrastructure, and CO2 emissions: Fresh evidence from panel quantile regression approach. Environmental Science and Pollution Research, 29(24), 36273–36280.
- Wong, W. P., & Tang, C. F. (2018). The major determinants of logistic performance in a global perspective: Evidence from panel data analysis. International Journal of Logistics Research and Applications, 21(4), 431–443. https://doi.org/10.1080/13675567.2018.1438377
- Yang, L., Hui, P., Yasmeen, R., Ullah, S., & Hafeez, M. (2020). Energy consumption and financial development indicators nexuses in Asian economies: A dynamic seemingly unrelated regression approach. Environmental Science and Pollution Research International, 27(14), 16472–16483.
- Yu, Y., & Du, Y. (2019). Impact of technological innovation on CO2 emissions and emissions trend prediction on ‘New Normal’economy in China. Atmospheric Pollution Research, 10(1), 152–161. https://doi.org/10.1016/j.apr.2018.07.005