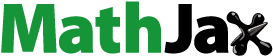
Abstract
The continuous growth of the transport sector and the increase in transportation carbon emissions attract policymakers’ attention. It is of great importance to understand the determinants of pollution from transportation. This study explores the dynamic nexus between transportation, growth, and environmental degradation in China. The QARDL approach is used for the empirical investigation of data series from 1995 to 2018. The findings exposed mixed results in both the long and short run. The result for freight transportation only improves the environment at upper extreme quantiles, while the results are insignificant in the short run. The results show that passenger transportation reduces CO2 emissions at the lower bottom quantiles in the long run, while the results are significant at upper extreme quantiles in the short run. In the case of GDP, the results endorsed the EKC hypothesis in the long run, while in short-run dynamics, the results for GDP2 are found insignificant, which elaborates that China’s economic growth enhances the CO2 emissions. Besides, the quantile causality test showed a bi-directional causality between all variables. The findings of this study provide concrete evidence to the policymakers of China to strengthen the sustainable transportation system by promoting eco-friendly and energy-efficient modes of transportation.
1. Introduction
The transportation sector is considered to be an important engine of economic growth and contributes greatly to the socio-economic development of countries. It enables the mobility of people and goods from one place to another (Berg & Ihlström, Citation2019; Umar et al., Citation2020). According to (Ozkan et al., Citation2019), a safe and environment-friendly transportation system is essential for sustainable economic growth. Although transportation is inevitable for globalization, it is also one of the biggest sources of environmental degradation (Liu et al., Citation2020; Umar et al., Citation2021). Especially in the cities, pollutant emissions produced by the transportation are a big concern for the environmentalists. The transportation sector is one of the largest consumers of fossil fuels, and such energy consumption makes a significant contribution to greenhouse gas emissions (C.-W. Su et al., Citation2022; Xu et al., Citation2020; Yan et al., Citation2022). (J. Zheng et al., Citation2018) argued that transportation is necessary for economic growth, but conversely, its impact on the environment cannot be ignored.
In 2011, the World Energy Commission (WEC) report unveiled that the transportation system consumed almost 19% of global energy and consequently has a share of 23% of carbon emissions worldwide. Another report released by UNCTAD (Development (UNCTAD),), Citation2015) stated that 64% of the world’s oil and fossil fuel is used for transportation. All sorts of modern transportation mean, including road, rail, air, and sea transport, have an adverse impact on the environment as they depend on energy from fossil fuels (Guo et al., Citation2022; Talbi, Citation2017; Q.-S. Wang et al., 2021). According to (Organization & W. H, Citation2006) report, more than 2 million people die prematurely due to air pollution each year, and the burning of fossil fuels in transportation contributes the most. In addition, the same reference data shows that 98% of residents in developing countries are exposed to environments with high levels of air pollution (Ji et al., Citation2021; R. Wang et al., Citation2020). To this extent, policymakers are increasingly aware of finding effective policy tools to solve traffic-oriented environmental pollution problems.
In this study, we emphasized China, one of the country’s leading carbon emissions globally. China has witnessed unprecedented economic growth in the previous 40 years since the reforms of 1978. As per the International Monetary Fund (IMF) data, China continues to become the largest economy worldwide after surpassing the United States in 2014 (Boumphrey, Citation2014; Monaghan, Citation2014; C. W. Su et al., Citation2022). With rapid economic development, China has also experienced accelerated urbanization, resulting in an increased number of vehicles on the road (Reddy et al., Citation2021; K.-H. Wang et al., Citation2022). By 2013, more than 50% of China’s population lived in urban areas. (Darido et al., Citation2014) evidenced that the car ownership average growth rate per capita is 10 to 25 percent annually. The fast growth in automobile ownership and usage related to urbanization cause to increase in global warming and other allied issues (Beaudoin et al., Citation2015; Brownstone & Golob, Citation2009; Kahn, Citation2000; Lin & Zeng, Citation2014; Si et al., Citation2021; Van Heddeghem et al., Citation2014). Along with the economic growth, China is the largest emitter of CO2, with 28% of carbon emissions reported in 2017 globally (Lobato et al., Citation2021; Shan et al., Citation2018). From 1978 to 2017, China’s per capita CO2 emissions amplified from 1.53 metric tons to 7.53 metric tons (Bank, Citation2019). Under such circumstances, policymakers and researchers must effectively analyze transportation-induced environmental pollution factors in China. Considering environmental pollution in China, a sustainable emission-free transportation system has become a crucial phenomenon (K.-H. Wang et al., Citation2021). Even though China has insisted on using legal carbon technology, its transportation system relies on fossil fuels. Even modern transportation technology provides many advantages, but it is not environmentally sustainable.
Many former studies have looked at the dynamic link between transportation and the environment using various economic estimation methods. (Huang et al., Citation2021) used the new cross-sectionally augmented autoregressive distributed lags (CS-ARDL) methods to look at the link between transportation, economic growth, urbanization, and emissions of greenhouse gases in the countries of ASEAN from 1995 to 2018 (Tao et al., Citation2022). According to the study, emissions grow with mobility, economic expansion, and urbanization but fall with the introduction of environmental levies (C.-W. Su et al., Citation2020; X.-T. Zhang et al., Citation2020). Similarly, (Shahbaz et al., Citation2015) used a Vector error correction model to assess the association between transportation and carbon emissions in Tunisia. They discovered a decoupling association of energy consumption with carbon emissions. (Neves et al., Citation2017) utilized the autoregressive distributed lag model (ARDL) to illustrate that fossil fuels in transport represent a significant contributor to economic development in 15 OECD nations (C.-W. Su et al., Citation2022). Similarly, Saidi and Hammami (Saidi & Mbarek, Citation2017) utilized a sample of 75 nations to examine the connection between GDP, transportation, and environmental deterioration from 2000 to 2014. In the countries of low, middle, and high-income countries, a two-way link is found between GDP and environmental degradation, and a one-way link is found between the transportation sector and environmental degradation that appears only in economies of high income (Umar et al., Citation2021). In contrast, reverse weaker causality is found in low and middle-income economies (Kaiser & Welters, Citation2019; Umar et al., Citation2022).
However, because research on China’s transportation, growth, and environmental degradation is relatively scarce, the current study focuses on the dynamic interplay between transportation, growth, and environmental degradation in China. With growing CO2 emissions from transportation and residential sources, China is one of the most important global emerging markets (Ielasi et al., Citation2018). This is due to several Chinese areas’ fast growth that requires energy. Consequently, establishing policies for China and other emerging countries can help them to rethink their strategies to stimulate sustainable growth without damaging the environment (Ji et al., Citation2021). In addition, an appropriate policy framework requires an appropriate methodological approach. Therefore, unlike traditional methods, the current study uses the Quantile Autoregressive Distribution Lag (QARDL) developed by (Cho et al., Citation2015) to design robust conclusions. The advantage of this method is that it simultaneously refers to the association between multiple time series of conditional quantiles of multiple quantile points.
The paper’s organization is as follows: Section 2 encloses the literature review; Section 3 explains the methodology. Section 4 discourses the outcomes, and Section 5 offers conclusions and policy suggestions.
2. Literature review
This section provides prior literature that identifies the relationship between transportation, economic growth, and carbon emissions in different contexts and prospects. Researchers and economists discussed the causal relationship between transportation-related energy consumption and environmental degradation. Such as (Georgatzi et al., Citation2020; González et al., Citation2019; Nasreen et al., Citation2018; Saidi & Mbarek, Citation2016; Sasana & Aminata, Citation2019) demonstrate that CO2 emissions from the transportation sector gradually increase with travel, energy use, and oil imports (Umar et al., Citation2021). In addition, it is argued that the use of transportation energy has accelerated environmental degradation without any restrictions or effective countermeasures. (Näslin & Sverrisdottir, Citation2020), considering the problem of structural fracture and cross-section dependence, it studied the causal relationship between transportation energy consumption, environmental degradation, and economic growth in 18 Asian countries from 1980 to 2017. The authors conclude that there is a two-way long-term Granger causality between transportation energy consumption and carbon dioxide emissions. (Banister & Stead, Citation2002) examine the affiliation between transportation and economic activities and carbon emissions. They confirmed that transportation and economic activities extensively amplified energy demand and eventually increased CO2 emissions (Neves et al., Citation2017; Tongwane et al., Citation2015) revealed that in South Africa, trucks and cars generate 70.6% of whole road transport pollutants.
The empirical study of (Ridhosari & Rahman, Citation2020) shows that transportation is ranked as the second-largest contributor to carbon emissions. (Georgatzi et al., Citation2020) use various econometric methods to investigate the main determinants of carbon emissions caused by traffic activities in 12 European economies from 1994 to 2014. They argued that transportation-linked carbon emissions are considerably affected by environmental regulations, new technologies, and the value addition of the transportation sector. (González et al., Citation2019) employed a dynamic panel data model to investigate the main determinants of CO2 emissions in European countries from 1990 to 2015. The study found that 92% of carbon emissions are directly linked to road transportation. (Li et al., Citation2020) applied autoregressive distribution lag and the VECM method to analyze the long-term relationship between China’s (1985-2013) carbon dioxide emissions and various transportation modes. They found that the expansion of China’s domestic road, aviation, and waterway infrastructure has experienced a long-term increase in environmental degradation. Similarly, (Li et al., Citation2020) compare the main determinants of carbon dioxide emissions in the United States and China from 1993 to 2013. They found that China’s transportation, power, natural gas, and construction industries significantly impact carbon dioxide emissions.
In the case of ASEAN, (Huang et al., Citation2021) reported that greenhouse gas emissions upsurge with transportation, economic expansion, and urbanization but decline with environmental-related levies. From 1995 to 2017, (Shafique et al., Citation2021) utilized multi-variate panel data analysis to evaluate the long-run connection between variables in ten Asian economies. From 1995 to 2017, they utilized ARDL and panel pool means group model to assess the link between economic growth, transportation, and environmental deterioration in ten Asian economies with CO2 emissions. The findings reveal that transportation, economic growth, and CO2 emissions have an endogenous link and that economic expansion and the transportation sector are primarily involved in environmental deterioration. In the United States, (Tiwari et al., Citation2021) looked at the Granger-causality between the transportation sector and CO2 emissions. Across all periods, they identified a weak link between traffic pollution and transportation infrastructure. Similarly, (Alam et al., Citation2021) examined the relationship between transportation infrastructure and economic development in Pakistan from 1971 to 2017. They utilized the ARDL and VECM methods. The results imply a long-term causal link between transportation infrastructure and economic progress.
Furthermore, take Pakistan and India as examples. (Tariq et al., Citation2017) used OLS regression to study the link between total capital formation, carbon dioxide emissions, and growth. Their research results show that energy use, real growth, and total capital formation positively impact carbon dioxide emissions (Berger, Citation2022; Hmaittane et al., Citation2019; Rauf et al., Citation2018) consider the ‘Belt and Road’ initiative and used the panel ARDL method to study the link between energy use, CO2 emissions, and growth. It turns out that energy usage and actual growth positively impact carbon emissions (C.-W. Su et al., Citation2020; C.-W. Su et al., Citation2022). This is primarily because economic growth accumulates resource consumption and increases people’s demand for environmental quality and technology change. (Dinda et al., Citation2000) apprehend the up gradation and optimization of the industrial structure, so it improves the environmental quality. (Stern & van Dijk, Citation2017) considered the impact of economic growth on PM2.5 pollution and institute that economic growth condensed the concentration of pollutant emissions and enhanced the environment. (Dong et al., Citation2019) argued that economic growth might be a vast driving force in recovering haze pollution.
Based on the preceding literature, it is noticeable that further research is needed in China on the link between economic growth, transportation, and CO2 emissions. Several studies examined various relationships between economic growth, transportation, and pollution emissions for various countries. Furthermore, a range of empirical estimating approaches has been applied by several scholars. To the authors’ knowledge, no research has looked at the entire dynamic link of transportation and economic growth with CO2 emissions by employing the unique Quantile Autoregressive Lagged (QARDL) technique, especially in the case of China. The study by (Sharif et al., Citation2020) also used QARDL to examine the impact of non-renewable and renewable energy consumption on the ecological footprint in Turkey, finding that the consumption of renewable energy decreases the ecological footprint on each quantile in the long run. Economic expansion and non-renewable energy, on the other hand, have a long-term and short-term positive influence on the ecological footprint at all quantiles. (Godil et al., Citation2020) used QARDL to look at the link between transportation services (i.e. passenger and freight) and carbon emissions in the US economy. However, because research on China is still few, the current study fills the gap by determining the dynamic relationship between transportation and economic growth with CO2 emissions in China.
3. Data and methods
3.1. Data and variables
This study explores the dynamic relationship between China’s transportation, growth, and environmental degradation. The variable Transportation is measured by including passenger transport (PT) and freight transport (FT). The economic growth measured as total nominal GDP and environmental degradation is proxied by CO2 emissions. The data is collected from the World Development Indicators (WDI) spanning the years 1995 to 2018. The annual data of the variables are converted into quarterly data through the quadratic matching and summation method, which is in line with (Arain et al., Citation2020; Aziz et al., Citation2020; Sharif et al., Citation2020). The above method effectively transforms low-frequency data and solves periodic divergence by discarding end-to-end data deviation.
3.2. Estimations
There is a need for a robust methodological approach as orthodox methods such as the Ordinary Least Squares (OLS) technique is only efficient in assessing the effects of variables only at the median. Besides, the relationship among variables may not be symmetric as claimed by the OLS. Since variables do not always exhibit a linear trend, a methodological adaptation is required to ameliorate the deficiencies of previous studies. Therefore, to achieve the objective of this study, the QARDL model is utilized to explore the dynamic nexus between transportation, economic growth, and environmental degradation in China. Introduced by (Cho et al., Citation2015), the QARDL is superior to the ARDL model, mainly due to its ability to expose asymmetries between key variables (such as FT, PT, GDP, and CO2 in our case). In many respects, the QARDL theory is compelling. For example, it enables locational asymmetries, which implies that the variables can be decided by the location of the dependent variable, which is enclosed by its conditional distribution. Second, the QARDL method can manage the long-term connection and past and present dynamics at different quantiles. Finally, classic econometric approaches have shown that time series variables do not co-integrate. This may be explained by the manifestation of cointegration coefficients varying at quantiles throughout prior and present dynamics, even while the parameters are moving together in the long run (X.-P. Zhang & Cheng, Citation2009)? The cointegration coefficient might diverge over the new quantiles created by shocks using this method. Other non-linear models, such as (Shin et al., Citation2014)NARDL model, in which nonlinearity is demarcated exogenously as the cutoff is fixed at zero rather than being formed through a data-driven method, so these characteristics make the QARDL the critical and most precise tool for detecting nonlinearity and asymmetries in China’s transportation, economic development, and carbon emissions. Further, the Wald test associated with the QARDL procedure gives information on the time period-varying integration association and the steadiness of integrating coefficients throughout the quantiles. The latter will ease the estimation of short and long-run symmetry. By moving from general to specific, the ARDL model associated with this study is given as:
(1)
(1)
Where are the various lag orders based on the Schwarz information criteria (SIC), while
is the error term. (Cho et al., Citation2015) modified EquationEq. (1)
(1)
(1) to include quantiles. As such, the QARDL (
form of EquationEq. (1)
(1)
(1) is given as:
(2)
(2)
From EquationEq. (2)(2)
(2) ,
Also, the range
indicates quantile. It is necessary to generalize the QARDL displayed in EquationEq. (2)
(2)
(2) due to the probability of serial correlation. As such, Eq. (3) is presented as;
(3)
(3)
Now, to account for the QARDL-ECM model, Eq. (3) needs to be reformulated into the generalized form shown in EquationEq. (4)(4)
(4) below:
(4)
(4)
The cumulative short-run impact of the preceding degradation on contemporary degradation via the delta method is determined by the same goes for FT, PT, and GDP, represented by
and
respectively. Now, the long-run parameters of FT, PT, and GDP are calculated as:
It is expected for (ECM parameter) to be significantly negative. Thereafter, as early mentioned, there is a need for a Wald test to evaluate the long and short-run asymmetric influence of FT, PT, and GDP on CO2. Therefore, EquationEq. (5)
(5)
(5) has been specified to explore the null hypotheses
parameter, while EquationEq. (6)
(6)
(6) presents the alternative hypothesis.
(5)
(5)
(6)
(6)
In a similar vein, these hypotheses are examined on the remaining parameters ( and also on
Be that as it may, the QARDL technique is not infallible. The only known weakness of the method is that the QARDL cannot analyze parameters at the quantile-on-quantile level. However, this does not reduce the quality of our study since we are only interested in non-linear inter-quantile analysis, not quantile-on-quantile analysis.
4. Results and discussions
4.1. Descriptive statistics
The descriptive statistical results of urbanization, carbon dioxide emissions, ecological innovation, trade opening, and renewable energy are shown in . From the results, the mean of all variables is positive. The average value of CO2 is 1.234, and the minimum and maximum values are 0.684 and 2.001, respectively. In addition, the average value of FT is 0.739, the minimum value is 0.400, and the maximum value is 1.202. The maximum value of PT is 2.002, the minimum value is 1.130, and the maximum value is 3.020. Except for transportation, GDP has the second-highest average value at 1.630, and the maximum and minimum values are 2.03 and 0.20, respectively. Finally, from , the Jarque-Bera statistics show that the variables are not normally distributed, which confirms the asymmetry and fat tails in the data series. Now, these justify the reasons for adopting QARDL technology.
Table 1. Results of descriptive statistics.
4.2. Unit root test
The non-stationarity of the time series is an important prerequisite for testing the long-term relationship between variables. In this study, we used the traditional unit root test (ADF) and unit root test and the (Zivot & Andrews, Citation2002) unit root test with a structural fracture to study non-stationarity. Using the traditional unit root test, the results show that all variables are non-stationary. However, the enhanced Dickey-Fuller (ADF) test in shows that it becomes stable in the first-order difference that is, all variables are integrated at the first-order difference according to the enhanced dickey fuller test. As many studies have shown, these traditional unit root tests may provide biased and false results because they do not consider the outage date information that appears in the time-series data. The interruption years in the data series are estimated using the ZA test. The results demonstrate that none of the variables is stationary but stable at the first difference. In addition, the ZA unit root test results showed strong evidence of structural fracture in all variables.
Table 2. Results of unit root tests.
4.3. QARDL results
presents the QARDL results showing the interactions between freight transport, passenger transport, economic growth, and CO2 emissions. Statistically, ρ (representing the speed of adjustment) is expected to be negative; however, it is highly significant at lower to medium quantiles, 0.05 to 0.70 but turns insignificant at higher quantiles. The long-run nexus of FT-CO2 is positively significant at lower quantiles to upper quantiles but becomes negatively significant at the upper extreme quantiles that inferring that the increase in freight transport in China deteriorates the environment unfavorably at the lower to medium quantiles. Exhaustion from freight transport is the major composition of pollutants that give rise to CO2 emissions. Freight transport is mainly reliant on consuming fossil fuels that dominate the energy mix in China, and China is not only the largest coal producer but also the leading fossil fuel consumer globally (Ahmed et al., Citation2020). While on the other side, the negatively significant results at the upper extreme quantiles reflect that China has been adhering to clean technology in freight transport to amend the environment. It suggests that increasing the consumption of clean energy will assist in declining CO2 emissions in the long run. Besides, this confirms that clean energy consumption is a veritable tool to curb environmental deterioration and the threat associated with climate change in China. Environmental rules and technologies have been introduced to clean the environment and make the ecosystem carbon-free due to China’s attempts to achieve sustainability. The result contradicts the study of (Godil et al., Citation2020), which showed that freight transportation is not advantageous to environmental protection in the United States at the highest quantile levels.
Table 3. Results of quantile autoregressive distributed lag (QARDL) for CO2 emission.
On the other side, the results in the case of passenger transport in china are negatively significant at the lower half quantile but turn insignificant at the upper quantiles. The results infer that passenger transport is efficient in abating emissions in China. This outcome is appealing; it exposes the efficacy of the existing energy sources employed in passenger transportation to curb environmental degradation. Moreover, it has been found that the consumption of renewable will help curb environmental deterioration. This corroborates the earlier findings of (Adedoyin et al., Citation2021; Aziz et al., Citation2020; Aziz et al., Citation2020; Y. Cheng & Yao, Citation2021; Tiba & Belaid, Citation2021; X. Wu et al., Citation2021). The insignificant results at the later quantiles infer that the rising population and expansion of urbanization in China increased the number of vehicles dramatically. The traffic jams during rush hours also cause vehicles to emit more gases, further exacerbating pollutants (Z. Cheng et al., Citation2020). The insignificant findings contradicted the conclusions of (Godil et al., Citation2020), who stated that passenger transportation in the US reduces carbon emissions more efficiently and considerably at quantiles 0.50 to 0.80. According to the research, the US transportation policies and environmental agencies are well prepared to protect and sustain the environment.
Except for freight and passenger transportation, the results of GDP infer that GDP is significantly positive in the upper extreme quantile, while GDP2 is negative and significant, supporting EKC and revealing the expansion of economic output. Expanding GDP compels resource-intensive industries to use more energy, increasing pollutant emissions. Contrarily, an increase in the population size could lead to a congestion effect, which might intensify electronic pollution. It reflects that the technological level at the early stage of development is not enough to support the improvement of energy efficiency or the effective use of clean technology, which ultimately leads to serious environmental problems. Though the economic growth enters the later phase, the marginal efficacy of environment quality continues to climb, recognizing future sustainable and green development. The up-gradation and optimization of the manufacturing structure speed up and increase energy efficacy. Also, the swift growth of the high-tech industry encourages the improvement of cleaner production technology, which eventually leads to a better environment. This indicates that China’s economic growth has increasingly accomplished structural and technological shifts to improve the environment. The results of the study are similar to those of previous studies. Prior studies also indicate there is an inverted U-shaped association between economic growth and the environment in China (Z. Wang et al., Citation2021; W. Wu et al., Citation2020; Xie et al., Citation2019). Sharif et al. (Citation2020) observed higher significant coefficients in the upper quantile in Malaysia, indicating that economic expansion impacts on emissions are more robust. The long-run coefficient for squared GDP, on the other hand, found a negative association with CO2 emissions, confirming the integrity of the EKC relationship and implying that a GDP growth first harms the environmental quality of the area before finally improving it.
For transportation, (Ozkan et al., Citation2019) confirmed a similar result. Considering the EKC, the result for the transportation sector reveals the effect of China’s income level on the sector’s environmental impact. The economic cost of sustainable investment focusing on the transportation sector is directly related to income level. In addition, it is understood that in China, the transportation industry, like many industries in the early stages of development, is dependent on fossil fuels. In the later stages of development, the consciousness of sustainable development of freight and passenger transportation is expected to be appeared. Moreover, biofuel is a vital substitute that will help countries achieve their carbon neutrality targets to improve the environment. Biofuel will replace traditional oil products in the United States and China by 2050; as per (X. Zheng et al., Citation2016), biofuels, fuel cell technologies, and decreasing the cost of low-carbon power generation should all be given more attention to minimize transportation-related emissions.
Turning to the short-run dynamics, it is found that the variations in current CO2 are positively and significantly affected by their own past across all quantiles. Also, the results for freight transportation are insignificant across all quantiles. Unlike freight transportation, the results of passenger transportation effectively decline CO2 emissions from medium to higher quantiles. It reflects that most passenger vehicles use clean-based energy that halts the noxious substances emitting into the surroundings. Moreover, private cars are also inevitably clean, and energy exhaust less emissions and eventually improving China’s environmental quality. The results show that the intensive consumption of clean energy helps regenerate the environment of China. In GDP, the results are positively significant at lower quantiles, while results for GDP2 are unexpectedly insignificant. The results provide insight that GDP2 cannot sustain a good quality environment in China over short-run dynamics.
4.4. Wald test
shows the corresponding Wald tests’ outputs and suggests that the constancy of parameters, null of linearity of the speed of adjustment parameter, is rejected. Also, the hypotheses of the parameter constancy across all quintiles for the long-run integrating parameters of βFT, βPT, and βGDP are not accepted. These results show that the co-integrating parameter between CO2 emissions and freight transport, CO2 emissions and passenger transport, CO2 emissions, and economic growth is dynamic in all quantiles, affirming asymmetry. The results could be justified on the grounds that the Chinese economy has undergone distinct changes in freight transportation, passenger transportation, and economic growth. Correspondingly, the Wald test in the lower portion of rejects the null hypothesis of linearity of the short-run influence of the past levels of CO2 emissions around the grid of considered quantiles.
Table 4. Results of the Wald test for the constancy of parameters.
4.5. Granger causality
The Granger-causality test results are presented in . The probability values suggest a two-way causality between all the variables such as FT and CO2, PT and CO2 emissions, GDP, and CO2 emission exist at a 1% significance level. The results infer that all variables granger causes each other. Thus, it is inevitable that a change in freight transport, passenger transport, and economic growth affect the CO2 emission, and the CO2 emission, in turn, influences these variables.
Table 5. Granger causality in Quantile test results.
5. Conclusions and policy suggestions
Transportation is considered to be a key economic sector that promotes the socio-economic development of a country. Although it is an important contributor to economic growth, it plays an important role in generating carbon emissions, which is a major obstacle to promoting a sustainable transportation system. This study uses QARDL technology to investigate the dynamic relationship between China’s transportation, growth, and environmental degradation. The findings exposed mixed results in both the long run and short run. The findings exposed that freight transportation only improves the environment at upper extreme quantiles while the results for freight transportation are insignificant in the short run. The results in passenger transportation show that passenger transportation reduces the CO2 emissions at the lower bottom quantiles in the long run, while in the short run, the results are significant at upper extreme quantiles. In the case of GDP, the results endorsed the EKC hypothesis in the long run, while in short-run dynamics, the results for GDP2 are found insignificant, elaborating that China’s economic growth cannot reduce CO2. Besides, the quantile causality test showed a bi-directional causality between all variables.
Considering that convenient transportation is an integral part of the quality of life and being fast, reliable, and diverse, sustainability is also an important point. However, as technology advances, every step that may be taken to enhance the quality of the environment is possible, especially for transportation. Based on empirical findings, it is confirmed that transportation is a high energy consumption sector and significantly contributes to China’s carbon emissions. It is needed to reinforce the sustainability of the transportation system by encouraging energy-saving and environment-friendly modes of transportation. Though China is moving towards electric vehicles, this is still a fraction considering the size of the country. In addition, necessary measures should be taken to increase people’s environmental awareness and the use of clean energy for transportation to create a clean environment. The future studies can further examine the transport system of China, considering provincial data with regard to the environment.
This paper has some shortcomings that point to a future research direction. In this study, we exclusively used freight and passenger transportation data. Other variables could be included in future research to estimate the overall influence of transportation on carbon emissions in China. For instance, future studies could include transportation infrastructure, energy prices, and road energy use. Moreover, future research could go deeper into China’s transportation system by considering provincial environmental statistics.
Disclosure statement
No potential conflict of interest was reported by the author.
Additional information
Funding
References
- Adedoyin, F. F., Ozturk, I., Agboola, M. O., Agboola, P. O., & Bekun, F. V. (2021). The implications of renewable and non-renewable energy generating in Sub-Saharan Africa: The role of economic policy uncertainties. Energy Policy, 150, 112115. https://doi.org/10.1016/j.enpol.2020.112115
- Ahmed, Z., Asghar, M. M., Malik, M. N., & Nawaz, K. (2020). Moving towards a sustainable environment: The dynamic linkage between natural resources, human capital, urbanization, economic growth, and ecological footprint in China. Resources Policy, 67, 101677. https://doi.org/10.1016/j.resourpol.2020.101677
- Alam, M. S., Islam, M. S., Shahzad, S. J. H., & Bilal, S. (2021). Rapid rise of life expectancy in Bangladesh: Does financial development matter? International Journal of Finance & Economics, 26(4), 4918–4931. https://doi.org/10.1002/ijfe.2046
- Arain, H., Han, L., Sharif, A., & Meo, M. S. (2020). Investigating the effect of inbound tourism on FDI: The importance of quantile estimations. Tourism Economics, 26(4), 682–703. https://doi.org/10.1177/1354816619859695
- Aziz, N., Mihardjo, L. W., Sharif, A., & Jermsittiparsert, K. (2020). The role of tourism and renewable energy in testing the environmental Kuznets curve in the BRICS countries: Fresh evidence from methods of moments quantile regression. Environmental Science and Pollution Research International, 27(31), 39427–39441.
- Aziz, N., Sharif, A., Raza, A., & Rong, K. (2020). Revisiting the role of forestry, agriculture, and renewable energy in testing environment Kuznets curve in Pakistan: Evidence from Quantile ARDL approach. Environmental Science and Pollution Research International, 27(9), 10115–10128.
- Banister, D., & Stead, D. (2002). Reducing transport intensity. European Journal of Transport and Infrastructure Research, 2(4), 161–178.
- Bank, W. (2019). Global financial development report 2019/2020: Bank regulation and supervision a decade after the global financial crisis. The World Bank.
- Beaudoin, J., Farzin, Y. H., & Lawell, C.-Y C. L. (2015). Public transit investment and sustainable transportation: A review of studies of transit’s impact on traffic congestion and air quality. Research in Transportation Economics, 52, 15–22. https://doi.org/10.1016/j.retrec.2015.10.004
- Berg, J., & Ihlström, J. (2019). The importance of public transport for mobility and everyday activities among rural residents. Social Sciences, 8(2), 58. https://doi.org/10.3390/socsci8020058
- Berger, D. (2022). Investor sentiment: A retail trader activity approach. Review of Accounting and Finance, 21(2), 61–82. https://doi.org/10.1108/RAF-06-2021-0152
- Boumphrey, S. (2014). China Overtakes the US as the World’s Largest Economy: Impact on industries and consumers worldwide. Euro Monitor International. Retrieved 20 December, 2020, from https://www.Iimk.Ac.in/Libportal/Reports/China-Overtakes-US-Worlds-Largest-Economy-White-Paper-Euromonitor-Report.Pdf.
- Brownstone, D., & Golob, T. F. (2009). The impact of residential density on vehicle usage and energy consumption. Journal of Urban Economics, 65(1), 91–98. https://doi.org/10.1016/j.jue.2008.09.002
- Cheng, Y., & Yao, X. (2021). Carbon intensity reduction assessment of renewable energy technology innovation in China: A panel data model with cross-section dependence and slope heterogeneity. Renewable and Sustainable Energy Reviews, 135, 110157. https://doi.org/10.1016/j.rser.2020.110157
- Cheng, Z., Li, L., & Liu, J. (2020). Natural resource abundance, resource industry dependence and economic green growth in China. Resources Policy, 68, 101734. https://doi.org/10.1016/j.resourpol.2020.101734
- Cho, J. S., Kim, T., & Shin, Y. (2015). Quantile cointegration in the autoregressive distributed-lag modeling framework. Journal of Econometrics, 188(1), 281–300. https://doi.org/10.1016/j.jeconom.2015.05.003
- Darido, G., Torres‐Montoya, M., & Mehndiratta, S. (2014). Urban transport and CO 2 emissions: Some evidence from C hinese cities. Wiley Interdisciplinary Reviews: Energy and Environment, 3(2), 122–155. https://doi.org/10.1002/wene.71
- Development (UNCTAD). (2015). Information economy report 2015: Unlocking the potential of E-commerce for developing countries. UN.
- Dinda, S., Coondoo, D., & Pal, M. (2000). Air quality and economic growth: An empirical study. Ecological Economics, 34(3), 409–423. https://doi.org/10.1016/S0921-8009(00)00179-8
- Dong, F., Zhang, S., Long, R., Zhang, X., & Sun, Z. (2019). Determinants of haze pollution: An analysis from the perspective of spatiotemporal heterogeneity. Journal of Cleaner Production, 222, 768–783. https://doi.org/10.1016/j.jclepro.2019.03.105
- Georgatzi, V. V., Stamboulis, Y., & Vetsikas, A. (2020). Examining the determinants of CO2 emissions caused by the transport sector: Empirical evidence from 12 European countries. Economic Analysis and Policy, 65, 11–20. https://doi.org/10.1016/j.eap.2019.11.003
- Godil, D. I., Sharif, A., Agha, H., & Jermsittiparsert, K. (2020). The dynamic nonlinear influence of ICT, financial development, and institutional quality on CO2 emission in Pakistan: New insights from QARDL approach. Environmental Science and Pollution Research International, 27(19), 24190–24200.
- González, R. M., Marrero, G. A., Rodríguez-López, J., & Marrero, Á. S. (2019). Analyzing CO2 emissions from passenger cars in Europe: A dynamic panel data approach. Energy Policy, 129, 1271–1281. https://doi.org/10.1016/j.enpol.2019.03.031
- Guo, X., Liang, C., Umar, M., & Mirza, N. (2022). The impact of fossil fuel divestments and energy transitions on mutual funds performance. Technological Forecasting and Social Change, 176, 121429. https://doi.org/10.1016/j.techfore.2021.121429
- Hmaittane, A., Bouslah, K., & M’Zali, B. (2019). Does corporate social responsibility affect the cost of equity in controversial industry sectors? Review of Accounting and Finance, 18(4), 635–662. https://doi.org/10.1108/RAF-09-2018-0184
- Huang, H., Wang, F., Song, M., Balezentis, T., & Streimikiene, D. (2021). Green innovations for sustainable development of China: Analysis based on the nested spatial panel models. Technology in Society, 65, 101593. https://doi.org/10.1016/j.techsoc.2021.101593
- Ielasi, F., Rossolini, M., & Limberti, S. (2018). Sustainability-themed mutual funds: An empirical examination of risk and performance. The Journal of Risk Finance, 19(3), 247–261. Article 3. https://doi.org/10.1108/JRF-12-2016-0159
- Ji, X., Chen, X., Mirza, N., & Umar, M. (2021). Sustainable energy goals and investment premium: Evidence from renewable and conventional equity mutual funds in the Euro zone. Resources Policy, 74, 102387. https://doi.org/10.1016/j.resourpol.2021.102387
- Ji, X., Zhang, Y., Mirza, N., Umar, M., & Rizvi, S. K. A. (2021). The impact of carbon neutrality on the investment performance: Evidence from the equity mutual funds in BRICS. Journal of Environmental Management, 297, 113228.
- Kahn, M. E. (2000). The environmental impact of suburbanization. Journal of Policy Analysis and Management, 19(4), 569–586. https://doi.org/10.1002/1520-6688(200023)19:4<569::AID-PAM3>3.0.CO;2-P
- Kaiser, L., & Welters, J. (2019). Risk-mitigating effect of ESG on momentum portfolios. The Journal of Risk Finance, 20(5), 542–555. Article 5. https://doi.org/10.1108/JRF-05-2019-0075
- Li, Y., Xu, D., Wang, J., Zhang, Y., & Yu, X. (2020). Industrial path analysis for CO2 transfers induced by final consumption: A comparison of the United States and China. Journal of Cleaner Production, 251, 119604. https://doi.org/10.1016/j.jclepro.2019.119604
- Lin, C.-Y C., & Zeng, J. (2014). The optimal gasoline tax for China. Theoretical Economics Letters, 04(04), 270–278. https://doi.org/10.4236/tel.2014.44037
- Liu, W., Zhang, Q., Chen, J., Xiang, R., Song, H., Shu, S., Chen, L., Liang, L., Zhou, J., You, L., Wu, P., Zhang, B., Lu, Y., Xia, L., Huang, L., Yang, Y., Liu, F., Semple, M. G., Cowling, B. J., … Liu, Y. (2020). Detection of Covid-19 in children in early January 2020 in Wuhan, China. The New England Journal of Medicine, 382(14), 1370–1371. https://doi.org/10.1056/NEJMc2003717
- Lobato, M., Rodríguez, J., & Romero, H. (2021). A volatility-match approach to measure performance: The case of socially responsible exchange traded funds (ETFs). The Journal of Risk Finance, 22(1), 34–43. https://doi.org/10.1108/JRF-04-2020-0066
- Monaghan, A. (2014). China poised to overtake US as world’s largest economy, research shows. The Guardian, 30. https://www.theguardian.com/business/2014/apr/30/china-overtake-us-worlds-largest-economy
- Näslin, C., & Sverrisdottir, S. (2020). Hallå, är det någon där?: En fallstudie om digitalisering som en organisationsförändring
- Nasreen, S., Saidi, S., & Ozturk, I. (2018). Assessing links between energy consumption, freight transport, and economic growth: Evidence from dynamic simultaneous equation models. Environmental Science and Pollution Research International, 25(17), 16825–16841. https://doi.org/10.1007/s11356-018-1760-5
- Neves, A. C., Harnedy, P. A., O'Keeffe, M. B., Alashi, M. A., Aluko, R. E., & FitzGerald, R. J. (2017). Peptide identification in a salmon gelatin hydrolysate with antihypertensive, dipeptidyl peptidase IV inhibitory and antioxidant activities. Food Research International, 100, 112–120. https://doi.org/10.1016/j.foodres.2017.06.065
- Organization, W. H. (2006). The world health report 2006: Working together for health. World Health Organization.
- Ozkan, T., Yanginlar, G., & Kalayci, S. (2019). Testing the transportation-induced environmental Kuznets curve hypothesis: Evidence from eight developed and developing countries. International Journal of Energy Economics and Policy, 9(1), 174.
- Rauf, A., Liu, X., Amin, W., Ozturk, I., Rehman, O. U., & Hafeez, M. (2018). Testing EKC hypothesis with energy and sustainable development challenges: A fresh evidence from belt and road initiative economies. Environmental Science and Pollution Research International, 25(32), 32066–32080. https://doi.org/10.1007/s11356-018-3052-5
- Reddy, K., Qamar, M. A. J., Mirza, N., & Shi, F. (2021). Overreaction effect: Evidence from an emerging market (Shanghai stock market). International Journal of Managerial Finance, 17(3), 416–437. https://doi.org/10.1108/IJMF-01-2019-0033
- Ridhosari, B., & Rahman, A. (2020). Carbon footprint assessment at Universitas Pertamina from the scope of electricity, transportation, and waste generation: Toward a green campus and promotion of environmental sustainability. Journal of Cleaner Production, 246, 119172. https://doi.org/10.1016/j.jclepro.2019.119172
- Saidi, K., & Mbarek, M. B. (2016). Nuclear energy, renewable energy, CO2 emissions, and economic growth for nine developed countries: Evidence from panel Granger causality tests. Progress in Nuclear Energy, 88, 364–374. https://doi.org/10.1016/j.pnucene.2016.01.018
- Saidi, K., & Mbarek, M. B. (2017). The impact of income, trade, urbanization, and financial development on CO2 emissions in 19 emerging economies. Environmental Science and Pollution Research, 24(14), 12748–12757. https://doi.org/10.1007/s11356-016-6303-3
- Sasana, H., & Aminata, J. (2019). Energy subsidy, energy consumption, economic growth, and carbon dioxide emission: Indonesian case studies. International Journal of Energy Economics and Policy, 9(2), 117.
- Shafique, M., Azam, A., Rafiq, M., & Luo, X. (2021). Investigating the nexus among transport, economic growth and environmental degradation: Evidence from panel ARDL approach. Transport Policy, 109, 61–71. https://doi.org/10.1016/j.tranpol.2021.04.014
- Shahbaz, M., Farhani, S., & Ozturk, I. (2015). Do coal consumption and industrial development increase environmental degradation in China and India? Environmental Science and Pollution Research International, 22(5), 3895–3907.
- Shan, Y., Guan, D., Zheng, H., Ou, J., Li, Y., Meng, J., Mi, Z., Liu, Z., & Zhang, Q. (2018). China CO2 emission accounts 1997–2015. Scientific Data, 5(1), 1–14. https://doi.org/10.1038/sdata.2017.201
- Sharif, A., Baris-Tuzemen, O., Uzuner, G., Ozturk, I., & Sinha, A. (2020). Revisiting the role of renewable and non-renewable energy consumption on Turkey’s ecological footprint: Evidence from Quantile ARDL approach. Sustainable Cities and Society, 57, 102138. https://doi.org/10.1016/j.scs.2020.102138
- Shin, Y., Yu, B., & Greenwood-Nimmo, M. (2014). Modelling asymmetric cointegration and dynamic multipliers in a nonlinear ARDL framework. In Festschrift in honor of Peter Schmidt (pp. 281–314). Springer.
- Si, D.-K., Li, X.-L., & Huang, S. (2021). Financial deregulation and operational risks of energy enterprise: The shock of liberalization of bank lending rate in China. Energy Economics, 93, 105047. https://doi.org/10.1016/j.eneco.2020.105047
- Stern, D. I., & van Dijk, J. (2017). Economic growth and global particulate pollution concentrations. Climatic Change, 142(3-4), 391–406. https://doi.org/10.1007/s10584-017-1955-7
- Su, C. W., Meng, X.-L., Tao, R., & Umar, M. (2022). Policy turmoil in China: A barrier for FDI flows? International Journal of Emerging Markets, 17(7), 1617–1634. https://doi.org/10.1108/IJOEM-03-2021-0314
- Su, C.-W., Naqvi, B., Shao, X.-F., Li, J.-P., & Jiao, Z. (2020). Trade and technological innovation: The catalysts for climate change and way forward for COP21. Journal of Environmental Management, 269, 110774. https://doi.org/10.1016/j.jenvman.2020.110774
- Su, C.-W., Song, Y., Tao, R., & Hao, L.-N. (2020). Does political conflict affect bilateral trade or vice versa? Evidence from Sino-U.S. relations. Economic Research-Ekonomska Istraživanja, 33(1), 3238–3257. https://doi.org/10.1080/1331677X.2019.1694559
- Su, C.-W., Xie, Y., Shahab, S., Faisal, C. M. N., Hafeez, M., & Qamri, G. M. (2021). Towards achieving sustainable development: role of technology innovation, technology adoption and CO2 emission for BRICS. International Journal of Environmental Research and Public Health, 18(1), 277. Article 1. https://doi.org/10.3390/ijerph18010277
- Su, C.-W., Yuan, X., Tao, R., & Umar, M. (2021). Can new energy vehicles help to achieve carbon neutrality targets? Journal of Environmental Management, 297, 113348.
- Talbi, B. (2017). CO2 emissions reduction in road transport sector in Tunisia. Renewable and Sustainable Energy Reviews, 69, 232–238. https://doi.org/10.1016/j.rser.2016.11.208
- Tao, R., Su, C.-W., Naqvi, B., & Rizvi, S. K. A. (2022). Can Fintech development pave the way for a transition towards low-carbon economy: A global perspective. Technological Forecasting and Social Change, 174, 121278. https://doi.org/10.1016/j.techfore.2021.121278
- Tariq, S., Ul-Haq, Z., Imran, A., Mehmood, U., Aslam, M. U., & Mahmood, K. (2017). CO2 emissions from Pakistan and India and their relationship with economic variables. Applied Ecology and Environmental Research, 15(4), 1301–1312. https://doi.org/10.15666/aeer/1504_13011312
- Tiba, S., & Belaid, F. (2021). Modeling the nexus between sustainable development and renewable energy: The African perspectives. Journal of Economic Surveys, 35(1), 307–329. https://doi.org/10.1111/joes.12401
- Tiwari, A. K., Boachie, M. K., Suleman, M. T., & Gupta, R. (2021). Structure dependence between oil and agricultural commodities returns: The role of geopolitical risks. Energy, 219, 119584. https://doi.org/10.1016/j.energy.2020.119584
- Tongwane, M., Piketh, S., Stevens, L., & Ramotubei, T. (2015). Greenhouse gas emissions from road transport in South Africa and Lesotho between 2000 and 2009. Transportation Research Part D: Transport and Environment, 37, 1–13. https://doi.org/10.1016/j.trd.2015.02.017
- Umar, M., Ji, X., Kirikkaleli, D., & Alola, A. A. (2021). The imperativeness of environmental quality in the United States transportation sector amidst biomass-fossil energy consumption and growth. Journal of Cleaner Production, 285, 124863. https://doi.org/10.1016/j.jclepro.2020.124863
- Umar, M., Ji, X., Kirikkaleli, D., & Xu, Q. (2020). COP21 Roadmap: Do innovation, financial development, and transportation infrastructure matter for environmental sustainability in China? Journal of Environmental Management, 271, 111026.
- Umar, M., Ji, X., Mirza, N., & Naqvi, B. (2021). Carbon neutrality, bank lending, and credit risk: Evidence from the Eurozone. Journal of Environmental Management, 296, 113156.
- Umar, M., Mirza, N., Rizvi, S. K. A., & Naqvi, B. (2022). ESG scores and target price accuracy: Evidence from sell-side recommendations in BRICS. International Review of Financial Analysis, 84, 102389. https://doi.org/10.1016/j.irfa.2022.102389
- Van Heddeghem, W., Lambert, S., Lannoo, B., Colle, D., Pickavet, M., & Demeester, P. (2014). Trends in worldwide ICT electricity consumption from 2007 to 2012. Computer Communications, 50, 64–76. https://doi.org/10.1016/j.comcom.2014.02.008
- Wang, K.-H., Su, C.-W., Xiao, Y., & Liu, L. (2022). Is the oil price a barometer of China’s automobile market? From a wavelet-based quantile-on-quantile regression perspective. Energy, 240, 122501. https://doi.org/10.1016/j.energy.2021.122501
- Wang, K.-H., Xiong, D.-P., Mirza, N., Shao, X.-F., & Yue, X.-G. (2021). Does geopolitical risk uncertainty strengthen or depress cash holdings of oil enterprises? Evidence from China. Pacific-Basin Finance Journal, 66, 101516. https://doi.org/10.1016/j.pacfin.2021.101516
- Wang, Q.-S., Su, C.-W., Hua, Y.-F., & Umar, M. (2022). How can new energy vehicles affect air quality in China?—From the perspective of crude oil price. Energy & Environment, 33(8), 1524–1544. https://doi.org/10.1177/0958305X211044388
- Wang, R., Mirza, N., Vasbieva, D. G., Abbas, Q., & Xiong, D. (2020). The nexus of carbon emissions, financial development, renewable energy consumption, and technological innovation: What should be the priorities in light of COP 21 Agreements? Journal of Environmental Management, 271, 111027. https://doi.org/10.1016/j.jenvman.2020.111027
- Wang, Z., Zhao, N., Wei, W., & Zhang, Q. (2021). A differentiated energy Kuznets curve: Evidence from mainland China. Energy, 214, 118942. https://doi.org/10.1016/j.energy.2020.118942
- Wu, W., Wang, W., & Zhang, M. (2020). Using China’s provincial panel data exploring the interaction between socio-economic and eco-environment system. Ecological Complexity, 44, 100873. https://doi.org/10.1016/j.ecocom.2020.100873
- Wu, X., Li, C., Shao, L., Meng, J., Zhang, L., & Chen, G. (2021). Is solar power renewable and carbon-neutral: Evidence from a pilot solar tower plant in China under a systems view. Renewable and Sustainable Energy Reviews, 138, 110655. https://doi.org/10.1016/j.rser.2020.110655
- Xie, Q., Xu, X., & Liu, X. (2019). Is there an EKC between economic growth and smog pollution in China? New evidence from semiparametric spatial autoregressive models. Journal of Cleaner Production, 220, 873–883. https://doi.org/10.1016/j.jclepro.2019.02.166
- Xu, Q., Umar, M., & Ji, X. (2020). Time-frequency analysis between renewable and nonrenewable energy consumption, economic growth, and CO2 emissions in the United States: Evidence from the transportation sector. In CICTP 2020 (pp. 2964–2974). https://doi.org/10.1061/9780784483053.250
- Yan, L., Mirza, N., & Umar, M. (2022). The cryptocurrency uncertainties and investment transitions: Evidence from high and low carbon energy funds in China. Technological Forecasting and Social Change, 175, 121326. https://doi.org/10.1016/j.techfore.2021.121326
- Zhang, X.-P., & Cheng, X.-M. (2009). Energy consumption, carbon emissions, and economic growth in China. Ecological Economics, 68(10), 2706–2712. https://doi.org/10.1016/j.ecolecon.2009.05.011
- Zhang, X.-T., Liu, X.-H., Su, C.-W., & Umar, M. (2020). Does asymmetric persistence in convergence of the air quality index (AQI) exist in China? Environmental Science and Pollution Research, 27(29), 36541–36569. https://doi.org/10.1007/s11356-020-09498-2
- Zheng, J., Tan, G., Shan, P., Liu, T., Hu, J., Feng, Y., Yang, L., Zhang, M., Chen, Z., Lin, Y., Lu, J., Neuefeind, J. C., Ren, Y., Amine, K., Wang, L.-W., Xu, K., & Pan, F. (2018). Understanding thermodynamic and kinetic contributions in expanding the stability window of aqueous electrolytes. Chem, 4(12), 2872–2882. https://doi.org/10.1016/j.chempr.2018.09.004
- Zheng, X., Pang, L., Chen, G., Huang, C., Liu, L., & Zhang, L. (2016). Challenge of population aging on health. In Public health challenges in contemporary China (pp. 43–53). Springer.
- Zivot, E., & Andrews, D. W. K. (2002). Further evidence on the great crash, the oil-price shock, and the unit-root hypothesis. Journal of Business & Economic Statistics, 20(1), 25–44. https://doi.org/10.1198/073500102753410372