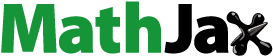
Abstract
It is reasonable to expect that the energy needs of the top emerging economies will increase over the coming decades as their economies expand rapidly. There may be environmental costs to using conventional energy from fossil fuels to meet increased energy demand. Therefore, this study aims to investigate the link between energy efficiency and energy transition along with additional control variables like research and development expenditures, trade, and gross domestic product for the top 10 emerging countries between 1990 and 2021. To study the case of these targeted economies, the authors use panel data and novel panel data econometrics techniques for a long-run relationship like the MMQR to observe changes over time between the variables, which is useful in the empirical evidence. The models’ primary findings are as follows: The panel cointegration tests confirm log-run associations among the targeted variables. Energy efficiency has the largest influence on the energy transition. The control variables like RDR and TRA decrease renewable energy consumption in the targeted economies. The findings also support the notion that GDP drives renewable energy consumption in the leading emerging economies. Numerous important ecological sustainability-related plans are recommended to the concerned governments based on research findings.
JEL CODES:
1. Introduction
Energy is a crucial component of every economy and is used extensively to fulfill the ever-increasing needs of Earth’s various life forms. Despite energy’s centrality to progress, achieving long-term economic growth presents a formidable task for nations worldwide (Tang et al., Citation2016; Umar et al., Citation2022). The current energy system’s reliance on fossil fuels is a major contributor to pollution and climate change (Muhammad et al., Citation2022; Umar et al., Citation2022; Yuan et al., Citation2022). One way human energy use negatively impacts the environment is by releasing greenhouse gases (GHG) and other forms of pollution (Cadoret & Padovano, Citation2016; Tufail et al., Citation2022; Yang et al., Citation2022). Thus, environmental decline and climate change have emerged as the century’s central concerns. Innovative research and development in the energy sector have led to a shift in focus by international organizations and leading economies toward strategies that emphasize efficient stats and power consumption, the use of renewable energy sources, and the reduction of hazardous emissions (Guo et al., Citation2022; Shan et al., Citation2022; Yu et al., Citation2022). 2017 had a worldwide increase in energy efficiency investment of 3% (236 billion USD) 2016. Energy consumption increased by 1.9% worldwide in 2017, driven largely by rising demand in emerging markets, where consumption is expected to increase by 90% by 2035 (OECD2011). The factors that influence a country’s energy consumption include population growth, the efficiency with which resources and energy are allocated, the level of technological advancement, and the level of investment in infrastructure and technology.
In addition, the leading emerging nations need to demonstrate unity with their global efforts for global warming mitigation by taking on the challenges of environmental degradation, notably by reducing emissions of dangerous chemicals into the environment (Chen et al., Citation2022). To assist Paris in achieving its goal of keeping global warming well below 2 °C over pre-industrial levels, these countries have pledged to limit their carbon emissions (Kang et al., Citation2019; Umar et al., Citation2022). To date, however, not a single one of these nations has been able to reduce its emissions of greenhouse gases, either overall or on a per-person basis. Furthermore, practically every one of these nations set goals of becoming carbon neutral and ceasing coal usage throughout the recently ended 26th meeting of events (COP26) in Glasgow (Ji et al., Citation2021). However, these countries are also committed to achieving the UN's 2030 Sustainable Development Goals (SDGs). These economies have shown a commitment to advancing social and economic progress while also enhancing the well-being of their ecosystems by ratifying the SDG agreement (Bansal & Kumar, Citation2021). While the Next Eleven have made progress toward the SDGs by demonstrating respectable economic growth rates, they have failed to ensure environmental sustainability (Ielasi et al., Citation2018). Most of these nations have fallen short in their efforts to encourage green advances by slowing the increase in their CO2 emissions.
Therefore, this research looks at analyzing energy efficiency’s influence on promoting energy transition and mitigating climate change in the world’s top 10 growing economies are all necessary steps toward restoring environmental well-being as soon as possible. These countries’ long-standing reliance on unclean energy is a major contributor to the deterioration of their natural environments. Non-renewable energy sources are heavily relied upon in these nations for electricity generation. In several of these countries, such as Mexico (where natural gas accounts for 59.9% of electricity generation) and Turkey (where it accounts for 37.9%), natural gas is an important contributor to the overall electrical system. In contrast, coal is used at a much higher percentage (55.8%) in Indonesia and 43.1% in South Korea during power production. Furthermore, in the previous 15 years, most of these countries have become more reliant on dirty energy sources, with a corresponding reduction in the proportion of their electrical generation that comes from renewable sources. To alleviate energy-related environmental difficulties and increase the consumption of renewable energy in leading emerging economies, it may be advised that energy use efficiency be increased in light of the challenges that have impeded the shift from non-renewable to renewable energy. However, these nations’ energy efficiency is improving slower than the rest of the world. This may be because they rely so heavily on conventional energy sources that are notoriously inefficient. Finally, it is assumed that these nations can match their CO2 emission-reduction goals with their R&D improvement strategies to encourage renewable energy use. This is since investments in research and development are now acknowledged as a significant factor in carbon dioxide (CO2) emissions.
This study makes three main unique contributions. To start, the majority of the related research describing the influences on environmental quality in the course of the top emerging economies has employed proxies such as energy use, either in terms of total or divided (non-renewable and renewable energy) consumption levels (Kang et al., Citation2019; Sinha et al., Citation2019). Unfortunately, scholars have paid little attention to the part that increased energy efficiency could play in modelling the factors determining environmental quality in these nations. Second, this research considers trade, a key element for energy consumption, rather than merely analyzing the direct effects of energy efficiency and R&D spending on the energy transition (Taghizadeh-Hesary et al., Citation2021). While this cross-effects investigation hasn’t received much attention in previous research, it’s crucial for developing a well-rounded policy. Finally, this investigation employs a novel econometric technique called the method of movement quantile (MMQR), which is one of the advanced econometric tools for examining the long-run connection between the specified variables. There are several positive qualities and benefits associated with the MMQR method. Unlike other quantile regression techniques, the MMQR specification may analyses the impact of independent variables across the entire range of conditional performance in the environment.
2. Literature review
2.1. The literature on energy efficiency and energy transition
There is a lack of research into the impact of increasing energy efficiency on a greener planet. However, few scholars have looked at the connections between these two factors. One such (Akram et al., Citation2020) examines the consequences of improved energy efficiency on the environment in 66 developing nations between 1990 and 2014. The findings showed that reducing CO2 emissions in these nations could be aided by better use of energy resources. Energy efficiency improvements, the authors argue, have a disproportionately big impact on reducing CO2 emissions from highly polluted developing countries and also boost the adoption of renewable energy sources. From the perspective of emerging nations, the authors stressed the necessity of improving energy efficiency in supporting the EKC hypothesis. According to (Balado-Naves et al., Citation2018), there is a chance that the neighbouring countries more efficient energy use will diminish CO2 emissions in the country of concern, which incorporated data from 173 worldwide economies. As a result of these results, scholars stress increasing renewable energy use and implementing measures to increase energy efficiency. A similar set of conclusions was drawn for Chinese provinces in the study cited in Xu et al. (Citation2016). Instead, Akram et al. (Citation2020) failed to develop a foundation for improving energy efficiency in South Africa, China, India, Brazil, and Russia, to reduce CO2 emissions and raise renewable energy consumption (collectively termed BRICS). Meanwhile, some new research has examined how energy intensity (the inverse of energy efficiency) affects the natural world. Energy intensity and carbon dioxide emissions were studied in 27 EU member states for reference (Bekun et al., Citation2021). Greater energy intensity (representing a fall in energy efficiency level) was shown to be associated with increased CO2 emissions and decreased consumption of renewable energy sources throughout Europe. According to the cited study (Shahbaz et al., Citation2015), higher energy intensity in certain African countries is correlated with higher energy consumption per unit of output created, providing support for the positive correlation between energy intensity and carbon emissions. Recently, (Namahoro et al., Citation2021) also discovered that an increase in energy intensity had the same effect on the CO2 emissions of 38 African countries. According to a single-country analysis of American emissions (Ulucak & Khan, Citation2020), higher energy intensity is associated with higher CO2 output. As economic policy uncertainty and non-renewable energy usage rise, the authors note that energy intensity has a greater impact on boosting CO2 emissions.
2.2. The literature on trade and energy transition
The existing research investigates the dynamic interplay between trade and renewable and non-renewable energy sources. This is because, as Akbar et al. (Citation2021) pointed out, freer trade benefits both renewable and non-renewable energy use. Just as strongly (Parsa & Sajjadi, Citation2017) asserts, there is a significant link between trade liberalization and energy use. This is especially true in the South African context, where the importance of trade openness in fostering renewable and non-renewable energy development has been recently underlined (Khoshnevis Yazdi & Shakouri, Citation2017). Similarly, (Guo & Pachauri, Citation2017) argues, with evidence from 78 different nations, that urban expansion raises energy consumption. However, reports from Ghana and the United States (Adom et al., Citation2012) suggest that the Iranian economy’s growth is only tangentially linked to its use of renewable and non-renewable energy sources; more specifically, the research finds that neither reducing energy consumption nor altering the energy portfolio has any effect on the economy’s expansion. Growth in the economy has been shown to have a direct and beneficial impact on using sustainable energy sources in Saudi Arabia (Lu, Citation2017; Mahmood et al., Citation2019). Consumption of energy, including both conventional and renewable sources, has boosted economies throughout East Asia and the Pacific (Hanif, Citation2018; Hdom & Fuinhas, Citation2020) substantiate a bidirectional causality between trade visibility and power usage when it comes to Brazil where the fostering of renewable resources has actually revealed to effectively reduce carbon emissions. Though a related study revealed conflicting outcomes, in general, both forms of energy are beneficial to economic development (Awodumi & Adewuyi, Citation2020).
2.3. The literature on research and development and energy transition
Spending on R&D encourages the use of renewable energy while limiting the use of non-renewable energy. Gan and Smith (Citation2011) revealed a minor role for R&D in promoting renewable energy and biofuel supply in the instance of OECD countries from 1994 to 2013. In contrast, R&D has been found to increase the usage of renewable energy sources while lowering the consumption of non-renewable energy in OECD nations (Yao et al., Citation2019). Using panel regression, (Sun & Kim, Citation2017) examined OECD energy R&D from 1974 to 2012. The findings suggest that the government’s energy R&D budget is influenced by governing policies, refinery output, and gross domestic R&D expenditures. Wu et al. (Citation2020) evaluated government R&D subsidies in China from 2009 to 2015 to promote renewable energy and venture capital. Based on the findings of this study, it is clear that R&D tax credits can encourage the development of renewable energy sources in China. Information spillover and public R&D on renewable energy in Nordic countries were studied by (Miremadi et al., Citation2019). The analysis demonstrates knowledge spillover and R&D boost renewable energy sources. The environmental and financial benefits of R&D investment in advancing renewable energy in South Korea were examined (Sim, Citation2018). According to the findings, renewable energy generation encourages R&D and lowers carbon emissions (Li et al., Citation2020) for the OECD nations showed that eco-innovation boosts renewable energy while reducing the usage of fossil fuels. According to Su et al., technological innovation lowers carbon emissions in the US (2020). In China, it has been found that technological advancement reduces carbon emissions (Umar et al., Citation2020; Alvarez-Herranz et al., Citation2017) discovered that environmental innovation is essential to REC using data from 17 OECD economies. The authors also pointed out that ongoing R&D produces environmental innovation, which converts the non-renewable energy sector into an economy based on renewable energy. From 1965 to 2014, (Yao et al., Citation2019) examined the relationship between gross domestic product (GDP) and the consumption of renewable and non-renewable energy in 18 OECD countries. They found that GDP increased both the consumption of renewable and non-renewable energy as well as total energy consumption. (Apergis & Payne, Citation2014) found that GDP had an impact on the consumption of renewable energy for 25 OECD countries. Similarly, (Przychodzen & Przychodzen, Citation2020) examined renewable energy production in transition economies between 1990 and 2014. In transition countries, there is evidence that GDP positively affects the production of renewable energy. In African countries, (Abanda et al., Citation2012) studied the link between GDP and REP from 1980 to 2008. The authors identified the link between renewable energy generation and GDP. (Gan & Smith, Citation2011) analyzed OECD renewable energy drivers from 1994 to 2003. The result demonstrates GDP boosts bio-energy and renewable energy supplies (RES). Renewable energy consumption in developing nations like India, the Philippines, China, Turkey, and Brazil is influenced by income and pollution levels (Salim & Rafiq, Citation2012; Li et al., Citation2020) investigated the variables influencing the use of renewable energy using OECD data. The scholars observed that GDP, Eco-innovation, and energy productivity affect REC. Similar to (Lin & Omoju, Citation2017) and (Yao et al., Citation2019), GDP is a key REC factor. (Lin & Omoju, Citation2017) China’s GDP increased non-hydro renewable energy use from 1980 to 2011. (Mrabet et al., Citation2019) studied developing and emerging economies’ RGDP and non-renewable energy usage from 1980 to 2014. Real GDP raises non-renewable energy consumption in emerging and developing economies. (Omri & Nguyen, Citation2014) studied renewable energy consumption (REC) from 1990 to 2011 in high, low, and middle-income nations. GDP raises or positively affects renewable energy consumption—a study finds (REC).
3. Theoretical framework
Considering that energy is required for every economic sector, having a steady supply is crucial for progress (Trotta, Citation2020; Umar et al., Citation2022). The environmental implications of energy usage are diverse between alternative sources, even though all sources can stimulate growth. This is because of differences in energy efficiency rates. In contrast to the negative effects on the environment from burning fossil fuels and other non-renewable energy sources, renewable energy sources only have positive effects. As a result, it is reasonable to believe that the environmental challenges associated with economic growth can be mitigated by reducing reliance on non-renewable energy sources while increasing reliance on renewable energy sources within economic systems (Ji et al., Citation2021; Liang et al., Citation2022; Su et al., Citation2022). Therefore, the IEA has emphasized the importance of integrating renewable energy into existing energy infrastructure to steer global economies toward carbon neutrality (Scott & Gössling, Citation2022; Umar et al., Citation2021). However, transitioning to renewable energy sources is not enough to achieve a low-carbon economy. Instead, energy users’ habits need to shift such that no energy is lost unnecessarily and instead is stored for later use. Because reducing energy waste by making better use of energy can be an effective way of combating climate change through energy demand-side channels. This is where the pursuit of energy efficiency improvement comes into play to harness the CO2 emission stifling goals (Trotta, Citation2020).
Research and development (R&D) spending, particularly in the renewable energy industry, is crucial to encourage cleaner energy and fulfil a country’s energy needs while contributing to a more sustainable environment. Greener power isn’t the only benefit of investing in renewable energy R&D; new companies can be created, and existing ones can create more jobs. Research and development must be encouraged to increase energy efficiency and decrease the cost of energy. Research and development (R&D) are crucial to finding new technologies and maximizing investment returns (Ferrat et al., Citation2022; Su et al., Citation2021; Yuan et al., Citation2022). Therefore, public R&D in renewable energy is vital, as it not only influences the future innovation process but also creates information spillover. R&D in renewable energy also promotes rivalry between various energy technologies, which in turn guarantees the delivery of cleaner, more reliable power. R&D in renewable energy is anticipated to enhance the use of renewable energy sources while decreasing the use of non-renewable sources.
Trade liberalization can potentially boost the use of fossil fuels, renewables, or both.
Previous research has established a correlation between international trade and the use of both renewable and non-renewable energy sources. Most prior research has found a positive correlation between energy use and trade. For instance, both (Dogan & Turkekul, Citation2016; Shahbaz et al., Citation2014) report that trade and energy use are positively correlated. Many other researchers have found a favorable correlation between commerce and energy use (Farhani & Shahbaz, Citation2014; Jalil & Mahmud, Citation2009). It has been shown in numerous earlier studies that international trade is a significant factor in the high levels of renewable energy consumption seen in many different nations and areas (Fotros & Maaboudi, Citation2010). However, it is also assumed that trade reduces renewable energy consumption because most emerging economies place a premium on increased trade with other nations and thus continuously increase the use of non-renewable sources to facilitate quick production and economic expansion.
In addition, economic growth is anticipated to boost demand for both renewable and conventional forms of energy. As the world’s economy expands, so does the demand for non-renewable energy sources. Fossil fuels provide a significant share of the energy needed to power the expanding economy (non-renewable energy consumption). Consequently, a rise in GDP will result in a greater demand for non-renewable sources of energy (Tufail et al., Citation2021). On the contrary, a higher GDP shows that a country can afford to invest more in renewable energy and other environmentally friendly forms of development (such as sustainable development). People’s desire for greener safeguards grows in tandem with their disposable money (Sadorsky, Citation2009).
4. Data sources and model specifications
This research aims to analyze the energy efficiency effect on energy transition along with other control variables such as trade, research and development, and gross domestic product in top emerging economies from 1990 to 2021. According to, panel data are a dynamic and rich approach to studying various problems and their components (Tufail et al., Citation2022). It aids in developing a more convincing argument, increases the reliability of the findings, and facilitates the establishment of solid conclusions. The data for all variables are obtained from World Bank (WDI 2021). The model’s general specifications are listed below.
(1)
(1)
In EquationEquation 1(1)
(1) , the cross-sections are denoted '
i.e., Argentina, Brazil, China, India, Indonesia, Mexico, Poland, South Africa, South Korea, and Turkey.
is for some time from 1990 to 2021. The basic regression from EquationEquation 1
(1)
(1) is given below.
(2)
(2)
where
represents energy transition means how much energy is generated from renewable sources and what share of total final energy consumption comes from such sources. The
the measure of R&D investment considers initial investment and recurring expenses across the business, government, higher education, and private, not-for-profit sectors. R&D encompasses a wide range of activities, from basic to apply to experimental.
is defined as Retail prices being arrived at by adding the product taxes levied on the final items to the gross value added by all domestic manufacturers in the economy and subtracting any subsidies that are not included in the final price. Depreciation of manufactured assets and depletion of natural resources are not factored into the estimation. The data are expressed in 2015 constant prices and are in US dollars.
Represents Trade is the total value of all exports plus all imports divided by GDP.
is the cross-section error term, and
is the error term ().
Table 1. Variables and sources’ nomenclature.
Table 2. Descriptive statistics.
5. Econometric methodology
5.1. Panel unit root test
Testing the stability of a single time series at a time using unit root tests was the norm in the past. On the other hand, finding a unit root in a panel structure is an extremely cutting-edge method that relies largely on the assumed structure of the data to be tested and has far more complex asymptotic aspects. A battery of tests covering various criteria and theoretical frameworks has been used to evaluate our findings. Performing a panel unit root test is suggested instead of conducting individual unit root tests on each sample (Levin et al., Citation2002; Khan et al., Citation2021; Khan et al., Citation2020a). An improved passing rate on this examination may be anticipated. A unit root is required for all-time series, and the starting point of all-time series must be the unit root, according to the null hypothesis (the alternative hypothesis). The panel-based framework from the ADF test will also be used because it is similar to the shape of the analyzed structure. This equation can be expressed as:
(3)
(3)
It has been decided that all LLCs must undergo the same set of tests. To check whether or not the unit root is typically 1, (Im et al., Citation2003) recommend allowing a non-uniform coefficient on The model developed by the computation is shown in the EquationEquation (1)
(1)
(1) . The panel is unit-root-complete if and only if the null hypothesis H0 holds, which states that all series in the panel have a unit root. Another hypothesis
proposes that only some of these series have unit roots. The IPS t statistic is calculated by averaging the N and ADF statistics.
(4)
(4)
5.2. Panel cointegration test
From 1990 through 2021, this study will analyze the top emerging economies in trade, R&D expenditures, and gross domestic product to determine if there is a link between energy efficiency and energy transition. The cointegration tests of interest are the panel cointegration tests developed by (Kao, Citation1999). The Kao-type test with residuals is used to conduct long-term tests of relationships between non-heterogeneous groups. The particular is also employed in this examination. DF and ADF statistics disprove the alternative hypothesis, which claims no cointegration exists. To calculate the residuals, we use the following regression model:
(5)
(5)
(Basile et al., Citation2005) came up with one set of ADF numbers:
(6)
(6)
5.3. Method of movement quantile regression
This research used a Moments Quantile Regression (MMQR) method with fixed effects (Machado & Silva, Citation2019). There are two scenarios when this method might be appropriate: when the panel data model has private effects and when the data contains endogenous repressors. In addition, this approach is considered among the most comparable approaches for dealing with heterogeneity and endogeneity, as well as for including crooked and non-linear affiliations. This method provides empirical insight into the distributive heterogeneity of the exhausts-income connection by utilizing fixed variables. This method addresses a problem that standard regressions based on the mean specification quotation cannot: the existence of heterogeneous connections between revenue and emissions in distinct conditional quantile circulations of emissions. Many arguments favour testing the environmental Kuznets hypothesis at various quantiles of temporary exhaust circulation. To begin, conditional quantiles assessments are more resistant to outliers originating from the dependent specification than price quotes of conditional methods are, which are subject to the distorting influence of outliers. Second, the conditional mean price quote is limited in capturing the cumulative impact of the revenue and discharges. The conditional quantile estimates provide more information than the conditional mean price quotes do because they distinguish the distributive influence of these repressors on the dependent variable at various quantile variants, which is something the quantile regression doesn’t do. This is especially true in panel regressions. According to the conditional quantile model, the calculation is as follows:
6. Results and Discussion
Tests for panel unit roots are shown in for all variables used in modelling energy efficiency’s role in mitigating climate change risk (energy transition), as well as other factors, including R&D spending, trade, and GDP in the world’s top 10 emerging nations. To accept the null hypothesis of a unit root, it is sufficient for RDR to be statistically insignificant, as shown by the (Levin et al., Citation2002) test. In particular, the EEF, GDP, RNC, and TRA thresholds of 5% and 10% are noteworthy. All variables, including EEF, GDP, RDR, RNC, and TRA, are shown to be statistically non-significant at the level using the method test developed by (I'm et al., 2003). In addition, the ADF-Fisher Chi-square test is used by (Choi, Citation2001; Maddala & Wu, Citation1999) to demonstrate that all the variables in question do have a unit root. Last but not least, the PP-F fisher chi-square test results demonstrate that while EEF and GDP are significant at the 5 and 10% levels, respectively, RDR, RNC, and TRA are not. According to the panel unit root test results, most of the model’s input variables do, in fact, have unit roots. Results of a panel unit root test using four methods are shown in . All of these methods assume that the variables have been differentiated either first or second. At the 1% significance level, all five tests show that the EEF, GDP, RDR, RNC, and TRA all stationary.
Table 3. Panel unit root testing.
In the second step of our analysis, we examine the possibility of a long-run cointegration among the different variables by employing Kao’s cointegration methods. presents empirical findings that reject the null hypothesis (H0) of the absence of cointegration at the 5% significance level. This indicates that the variables under study, which include energy efficiency, renewable energy consumption, GDP, trade, and research and development expenditures, exhibit a long-run cointegration relationship from 1990 to 2021. The results of the cointegration test are presented in .
Table 4. Panel cointegration test.
After determining the long-run relationships between the variables, this research employs the unique technique of moment’s quantile regression (MMQR), which is more effective in handling non-linear, irregular data and providing statistical estimates at a specific scale, location, and quantile. shows the predicted outcomes of the method above. Empirical evidence demonstrates that EEF positively affects renewable energy consumption throughout the board. The empirical results show that EEF exhibits a strong positive influence on the RNC across all quantiles. Specifically, the EEF increases the RNC in all described quantiles, i.e., Q0.25, Q0.50, Q0.75, and Q0.90, which is significant at a 1% level. It is observed that a 1% increase in energy efficiency boost 0.15%, 0.17%, 0.18%, and 0.19% renewable energy consumption in the mentioned 25th, 50th, 75th, and 90th quantiles, respectively. It is widely predicted that energy efficiency will improve an increase in renewable energy consumption; hence, an improvement in energy efficiency means either less energy will be wasted, or less energy will be required to produce the same result. Both the decrease in demand for energy imports and the reduction in emissions of carbon-based pollutants contribute to an improvement in the environmental sustainability of the targeted economies. This has a positive impact on the country’s energy sector. Including RE in the energy mix also has a secondary, indirect impact on the energy cost (rise) connected to energy efficiency. Our findings are similar to the result of Shadman et al. (Citation2022).
Table 5. Method of moment quantile regressions (MMQRs).
Across all quantiles, there is a negative and statistically significant correlation between R&D spending and renewable energy consumption. In Q 0.25, Q 0.50, Q 0.75, and Q 0.90, a 1% increase in RDR decreased −0.87%, −0.64%, −0.56%, and −0.49% RNC, respectively. Equally important to advancing cleaner energy is funding for research and development. At the same time, human capital and infrastructure growth are essential to R&D and entrepreneurship (Mirza et al., Citation2020; Xu & Lin, Citation2017; Yarovaya et al., Citation2021). Taking into account the advanced level of human capital in the top emerging countries, which aids in a better comprehension of energy consumption and its environmental impact, leading to increased energy efficiency (Vidotto et al., Citation2017), the targeted top emerging countries provide the requisite setting for promoting technological innovations that are both environmentally friendly and energy efficient. Moreover, these developments lower the price of renewable energy (Wong et al., Citation2013), making the switch from NREC to REC easier for the general public (Yao et al., Citation2019). In the wake of the Paris (Climate) Agreement of 2015, the top emerging economies have placed a greater emphasis on the widespread adoption of eco-innovative technologies that not only help reduce emissions but also cut production and environmental costs.
Additionally, trade analysis proves that the use of renewable energy is negatively and significantly correlated across all quantiles. In Q 0.25, Q 0.50, Q 0.75, and Q 0.90, a 1% increase in TRA decreased −0.87%, −1.07%, −1.15%, and −1.21% renewable energy, respectively. This suggests that trade activities are among the most significant consumers of energy derived from non-renewable sources. Furthermore, it suggests that most commercial operations, notably exports, require significant energy consumption. These countries are now emerging economies. They are targeting high economic growth and productivity. For this purpose, they mostly depend on non-renewable energy consumption, which further disturbs the environment. Similar results are found by (Abbasi & Riaz, Citation2016; Farhani & Shahbaz, Citation2014; Jayanthakumaran et al., Citation2012)
The last variable, GDP, shows a positive and significant relationship with renewable energy consumption in all the top emerging economies. It is clearly shown that in the 25th, 50th, 75th, and 90th quantiles, there is a dramatic increase of 0.53%, 0.35%, 0.29%, and 0.24% for renewable energy consumption. The GDP promotes these countries’ capacity to improve their sustainable environment, and it invests in such projects and initiates which promote a green economic system that further strengthens the utilization of renewable energy. When the economic condition of the populous becomes better, they are always eager to protect the environment so, in such circumstances, there is a continuously increasing demand for renewable energy. The same results were obtained by Khan et al. (Citation2020).
The bootstrap quantile regression was used in this study to verify the empirical outcomes of the prior estimator and as a check on the robustness of the estimates. presents the estimated results obtained using the aforementioned estimation methods. This study examined the findings of its investigation on the link between energy efficiency and the usage of renewable energy in the top 10 growing economies. The findings of this research study support that increasing energy efficiency positively impacts the usage of renewable energy sources. As a consequence, the empirical outputs of the panel MMQR approach are validated by the robustness test results ().
Table 6. Bootstrap quantile regression (robustness check).
7. Conclusion and policy recommendations
The impacts of energy efficiency on energy transition are investigated, considering crucial control variables that have been overlooked in prior research. Furthermore, the control variables such as research and development expenditures, trade, and GDP are analyzed for top emerging economies between 1990 and 2021. We apply the most suitable and updated econometric models along with the movement Quantile regression (MMQR) regression method to examine the energy efficiency effect on energy transition. The findings show that RDR and TRA significantly negatively affect the energy transition. However, the GDP confirmed a positive effect and enhanced the selected economies’ energy transition.
7.1. Policy recommendations
Affiliated governments can advocate the following policies for environmental sustainability policymaking based on these findings. Energy efficiency improvement, directly and indirectly, contributes to energy transition by moderating and mediating. Identifying inefficiencies in energy production and use is a must. To reduce energy inefficiency in production, it’s vital to diversify the major energy sources used for power generation. The substitution of less-efficient energy inputs with more efficient alternatives can be an important energy policy reform for these countries. Minimizing transmission and distribution losses help to improve energy efficiency in these countries. On the consumption side, governments should encourage end-users to use less energy-intensive goods. Consumers must alter their habits following IEA recommendations to reduce energy waste and ensure sufficient supplies for the foreseeable future. Another important issue for the affiliated governments to tackle is the implementation of public education campaigns emphasizing the need to reduce energy use and waste. One of these countries’ primary objectives ought to be to increase their investment in R&D to improve energy efficiency and new technologies. Second, reducing the use of non-renewable energy in overall electricity generation can be achieved through greening the traditional energy networks of leading emerging nations. This means they need to put their money into renewable energy sources for power. However, the transition to renewable energy is slowed by the high cost of renewable energy inputs. To hasten the transition from dirty to clean energy, the top emerging nations must lower the levelized cost of renewable electricity. Again, this shows the necessity of technological innovation, as without it is impossible to cut the cost of renewable electricity generation or encourage the renewable energy transition. 3rd, these countries should green their economic operations and export-oriented businesses to counteract the negative environmental externalities of economic growth and international trade involvement.
7.2. Current Deficiencies and future guidelines
Finally, we will discuss the limitations of our work and make recommendations for further research. It was originally thought that all emerging countries would interest the researchers. However, we could only include 10 countries in our final choices due to space constraints. To that end, it would be prudent to think about conducting additional research in the future that takes into account one of the most recent changes, such as the COVID-19 economic crisis. The fact that we focused mostly on developing nations is also a contributing element. This study’s conclusions are generalizable to future gatherings of similar groups of countries, such as the G7, G8, and G20. The role of energy transition and technological advancement can also be considered.
Disclosure statement
No conflict of interest has been reported by the authors.
Additional information
Funding
References
- Abanda, F. H., Ng’ombe, A., Keivani, R., & Tah, J. H. M. (2012). The link between renewable energy production and gross domestic product in Africa: A comparative study between 1980 and 2008. Renewable and Sustainable Energy Reviews, 16(4), 2147–2153. https://doi.org/10.1016/j.rser.2012.01.005
- Abbasi, F., & Riaz, K. (2016). CO2 emissions and financial development in an emerging economy: An augmented VAR approach. Energy Policy, 90, 102–114. https://doi.org/10.1016/j.enpol.2015.12.017
- Adom, P. K., Bekoe, W., Amuakwa-Mensah, F., Mensah, J. T., & Botchway, E. (2012). Carbon dioxide emissions, economic growth, industrial structure, and technical efficiency: Empirical evidence from Ghana, Senegal, and Morocco on the causal dynamics. Energy, 47(1), 314–325. https://doi.org/10.1016/j.energy.2012.09.025
- Akbar, M. W., Yuelan, P., Maqbool, A., Zia, Z., & Saeed, M. (2021). The nexus of sectoral-based CO2 emissions and fiscal policy instruments in the light of Belt and Road Initiative. Environmental Science and Pollution Research, 28(25), 32493–32507. https://doi.org/10.1007/s11356-021-13040-3
- Akram, R., Chen, F., Khalid, F., Ye, Z., & Majeed, M. T. (2020). Heterogeneous effects of energy efficiency and renewable energy on carbon emissions: Evidence from developing countries. Journal of Cleaner Production, 247, 119122. https://doi.org/10.1016/j.jclepro.2019.119122
- Akram, R., Majeed, M. T., Fareed, Z., Khalid, F., & Ye, C. (2020). Asymmetric effects of energy efficiency and renewable energy on carbon emissions of BRICS economies: Evidence from nonlinear panel autoregressive distributed lag model. Environmental Science and Pollution Research International, 27(15), 18254–18268. https://doi.org/10.1007/s11356-020-08353-8
- Alvarez-Herranz, A., Balsalobre-Lorente, D., Shahbaz, M., & Cantos, J. M. (2017). Energy innovation and renewable energy consumption in the correction of air pollution levels. Energy Policy, 105, 386–397. https://doi.org/10.1016/j.enpol.2017.03.009
- Apergis, N., & Payne, J. E. (2014). The causal dynamics between renewable energy, real GDP, emissions and oil prices: Evidence from OECD countries. Applied Economics, 46(36), 4519–4525. https://doi.org/10.1080/00036846.2014.964834
- Awodumi, O. B., & Adewuyi, A. O. (2020). The role of non-renewable energy consumption in economic growth and carbon emission: Evidence from oil producing economies in Africa. Energy Strategy Reviews, 27, 100434. https://doi.org/10.1016/j.esr.2019.100434
- Balado-Naves, R., Baños-Pino, J. F., & Mayor, M. (2018). Do countries influence neighbouring pollution? A spatial analysis of the EKC for CO2 emissions. Energy Policy, 123, 266–279. https://doi.org/10.1016/j.enpol.2018.08.059
- Bansal, M., & Kumar, V. (2021). Forcing responsibility? Examining earnings management induced by mandatory corporate social responsibility: Evidence from India. Review of Accounting and Finance, 20(2), 194–216. https://doi.org/10.1108/RAF-06-2020-0151
- Basile, R., Costantini, M., & Destefanis, S. (2005). Unit root and cointegration tests for cross-sectionally correlated panels. Estimating regional production functions.
- Bekun, F. V., Alola, A. A., Gyamfi, B. A., & Yaw, S. S. (2021). The relevance of EKC hypothesis in energy intensity real-output trade-off for sustainable environment in EU-27. Environmental Science and Pollution Research International, 28(37), 51137–51148. https://doi.org/10.1007/s11356-021-14251-4
- Cadoret, I., & Padovano, F. (2016). The political drivers of renewable energies policies. Energy Economics, 56, 261–269. https://doi.org/10.1016/j.eneco.2016.03.003
- Chen, Z., Mirza, N., Huang, L., & Umar, M. (2022). Green banking—Can financial institutions support green recovery? Economic Analysis and Policy, 75, 389–395. https://doi.org/10.1016/j.eap.2022.05.017
- Choi, I. (2001). Unit root tests for panel data. Journal of International Money and Finance, 20(2), 249–272. https://doi.org/10.1016/S0261-5606(00)00048-6
- Dogan, E., & Turkekul, B. (2016). CO2 emissions, real output, energy consumption, trade, urbanization and financial development: Testing the EKC hypothesis for the USA. Environmental Science and Pollution Research International, 23(2), 1203–1213. https://doi.org/10.1007/s11356-015-5323-8
- Farhani, S., & Shahbaz, M. (2014). What role of renewable and non-renewable electricity consumption and output is needed to initially mitigate CO2 emissions in MENA region? Renewable and Sustainable Energy Reviews, 40, 80–90. https://doi.org/10.1016/j.rser.2014.07.170
- Ferrat, Y., Daty, F., & Burlacu, R. (2022). Short-and long-term effects of responsible investment growth on equity returns. The Journal of Risk Finance, 23(1), 1–13. https://doi.org/10.1108/JRF-07-2021-0107
- Fotros, M. H., & Maaboudi, R. (2010). The impact of trade openness on CO2 emissions in Iran, 1971–2005
- Gan, J., & Smith, C. T. (2011). Drivers for renewable energy: A comparison among OECD countries. Biomass and Bioenergy, 35(11), 4497–4503. https://doi.org/10.1016/j.biombioe.2011.03.022
- Guo, F., & Pachauri, S. (2017). China’s green lights program: A review and assessment. Energy Policy, 110, 31–39. https://doi.org/10.1016/j.enpol.2017.08.002
- Guo, X., Liang, C., Umar, M., & Mirza, N. (2022). The impact of fossil fuel divestments and energy transitions on mutual funds performance. Technological Forecasting and Social Change, 176, 121429. https://doi.org/10.1016/j.techfore.2021.121429
- Hanif, I. (2018). Impact of fossil fuels energy consumption, energy policies, and urban sprawl on carbon emissions in East Asia and the Pacific: A panel investigation. Energy Strategy Reviews, 21, 16–24. https://doi.org/10.1016/j.esr.2018.04.006
- Hdom, H. A., & Fuinhas, J. A. (2020). Energy production and trade openness: Assessing economic growth, CO2 emissions and the applicability of the cointegration analysis. Energy Strategy Reviews, 30, 100488. https://doi.org/10.1016/j.esr.2020.100488
- Ielasi, F., Rossolini, M., & Limberti, S. (2018). Sustainability-themed mutual funds: An empirical examination of risk and performance. The Journal of Risk Finance, 19(3), 247–261. https://doi.org/10.1108/JRF-12-2016-0159
- Im, K. S., Pesaran, M. H., & Shin, Y. (2003). Testing for unit roots in heterogeneous panels. Journal of Econometrics, 115(1), 53–74. https://doi.org/10.1016/S0304-4076(03)00092-7
- Jalil, A., & Mahmud, S. F. (2009). Environment Kuznets curve for CO2 emissions: A cointegration analysis for China. Energy Policy, 37(12), 5167–5172. https://doi.org/10.1016/j.enpol.2009.07.044
- Jayanthakumaran, K., Verma, R., & Liu, Y. (2012). CO2 emissions, energy consumption, trade and income: A comparative analysis of China and India. Energy Policy, 42, 450–460. https://doi.org/10.1016/j.enpol.2011.12.010
- Ji, X., Chen, X., Mirza, N., & Umar, M. (2021). Sustainable energy goals and investment premium: Evidence from renewable and conventional equity mutual funds in the Euro zone. Resources Policy, 74, 102387. https://doi.org/10.1016/j.resourpol.2021.102387
- Ji, X., Zhang, Y., Mirza, N., Umar, M., & Rizvi, S. K. A. (2021). The impact of carbon neutrality on the investment performance: Evidence from the equity mutual funds in BRICS. Journal of Environmental Management, 297, 113228.
- Kang, S. H., Islam, F., & Tiwari, A. K. (2019). The dynamic relationships among CO2 emissions, renewable and non-renewable energy sources, and economic growth in India: Evidence from time-varying Bayesian VAR model. Structural Change and Economic Dynamics, 50, 90–101. https://doi.org/10.1016/j.strueco.2019.05.006
- Kao, C. (1999). Spurious regression and residual-based tests for cointegration in panel data. Journal of Econometrics, 90(1), 1–44. https://doi.org/10.1016/S0304-4076(98)00023-2
- Khan, Z., Malik, M. Y., Latif, K., & Jiao, Z. (2020). Heterogeneous effect of eco-innovation and human capital on renewable & non-renewable energy consumption: Disaggregate analysis for G-7 countries. Energy, 209, 118405. https://doi.org/10.1016/j.energy.2020.118405
- Khan, Z., Ali, S., Dong, K., & Li, R. Y. M. (2021). How does fiscal decentralization affect CO2 emissions? The roles of institutions and human capital. Energy Economics, 94, 105060. https://doi.org/10.1016/j.eneco.2020.105060
- Khan, Z., Hussain, M., Shahbaz, M., Yang, S., & Jiao, Z. (2020a). Natural resource abundance, technological innovation, and human capital nexus with financial development: A case study of China. Resources Policy, 65, 101585. https://doi.org/10.1016/j.resourpol.2020.101585
- Khoshnevis Yazdi, S., & Shakouri, B. (2017). Renewable energy, nonrenewable energy consumption, and economic growth. Energy Sources, Part B: Economics, Planning, and Policy, 12(12), 1038–1045. https://doi.org/10.1080/15567249.2017.1316795
- Levin, A., Lin, C.-F., & Chu, C.-S J. (2002). Unit root tests in panel data: Asymptotic and finite-sample properties. Journal of Econometrics, 108(1), 1–24. https://doi.org/10.1016/S0304-4076(01)00098-7
- Li, J., Zhang, X., Ali, S., & Khan, Z. (2020). Eco-innovation and energy productivity: New determinants of renewable energy consumption. Journal of Environmental Management, 271, 111028.
- Liang, C., Umar, M., Ma, F., & Huynh, T. L. (2022). Climate policy uncertainty and world renewable energy index volatility forecasting. Technological Forecasting and Social Change, 182, 121810. https://doi.org/10.1016/j.techfore.2022.121810
- Lin, B., & Omoju, O. E. (2017). Focusing on the right targets: Economic factors driving non-hydro renewable energy transition. Renewable Energy. 113, 52–63. https://doi.org/10.1016/j.renene.2017.05.067
- Lu, W.-C. (2017). Renewable energy, carbon emissions, and economic growth in 24 Asian countries: Evidence from panel cointegration analysis. Environmental Science and Pollution Research International, 24(33), 26006–26015. https://doi.org/10.1007/s11356-017-0259-9
- Machado, J. A., & Silva, J. S. (2019). Quantiles via moments. Journal of Econometrics, 213(1), 145–173. https://doi.org/10.1016/j.jeconom.2019.04.009
- Maddala, G. S., & Wu, S. (1999). A comparative study of unit root tests with panel data and a new simple test. Oxford Bulletin of Economics and Statistics, 61(S1), 631–652. https://doi.org/10.1111/1468-0084.0610s1631
- Mahmood, H., Alkhateeb, T. T. Y., Al-Qahtani, M. M. Z., Allam, Z. A., Ahmad, N., & Furqan, M. (2019). Energy consumption, economic growth and pollution in Saudi Arabia
- Miremadi, I., Saboohi, Y., & Arasti, M. (2019). The influence of public R&D and knowledge spillovers on the development of renewable energy sources: The case of the Nordic countries. Technological Forecasting and Social Change, 146, 450–463. https://doi.org/10.1016/j.techfore.2019.04.020
- Mirza, N., Rahat, B., Naqvi, B., & Rizvi, S. K. A. (2020). Impact of Covid-19 on corporate solvency and possible policy responses in the EU. The Quarterly Review of Economics and Finance, https://doi.org/10.1016/j.qref.2020.09.002
- Mrabet, Z., Alsamara, M., Saleh, A. S., & Anwar, S. (2019). Urbanization and non-renewable energy demand: A comparison of developed and emerging countries. Energy, 170, 832–839. https://doi.org/10.1016/j.energy.2018.12.198
- Muhammad, S., Pan, Y., Agha, M. H., Umar, M., & Chen, S. (2022). Industrial structure, energy intensity and environmental efficiency across developed and developing economies: The intermediary role of primary, secondary and tertiary industry. Energy, 247, 123576. https://doi.org/10.1016/j.energy.2022.123576
- Namahoro, J. P., Wu, Q., Zhou, N., & Xue, S. (2021). Impact of energy intensity, renewable energy, and economic growth on CO2 emissions: Evidence from Africa across regions and income levels. Renewable and Sustainable Energy Reviews, 147, 111233. https://doi.org/10.1016/j.rser.2021.111233
- Omri, A., & Nguyen, D. K. (2014). On the determinants of renewable energy consumption: International evidence. Energy, 72, 554–560. https://doi.org/10.1016/j.energy.2014.05.081
- Parsa, H., & Sajjadi, S. Z. (2017). Exploring the trade openness, energy consumption and economic growth relationship in Iran by Bayer and Hanck combined cointegration and causality analysis. Iranian Economic Review, 21(4), 829–845.
- Przychodzen, W., & Przychodzen, J. (2020). Determinants of renewable energy production in transition economies: A panel data approach. Energy, 191, 116583. https://doi.org/10.1016/j.energy.2019.116583
- Sadorsky, P. (2009). Renewable energy consumption and income in emerging economies. Energy Policy, 37(10), 4021–4028. https://doi.org/10.1016/j.enpol.2009.05.003
- Salim, R. A., & Rafiq, S. (2012). Why do some emerging economies proactively accelerate the adoption of renewable energy? Energy Economics, 34(4), 1051–1057. https://doi.org/10.1016/j.eneco.2011.08.015
- Scott, D., & Gössling, S. (2022). Destination net-zero: What does the international energy agency roadmap mean for tourism?. Journal of Sustainable Tourism, 30(1), 14–31. https://doi.org/10.1080/09669582.2021.1962890
- Shadman, S., Chin, C. M. M., Sakundarini, N., Yap, E. H., Fairuz, S., Wong, X. Y., Khalid, P. A., Karimi, F., Karaman, C., Mofijur, M., Koyande, A. K., & Show, P. L. (2022). A system dynamics approach to pollution remediation and mitigation based on increasing the share of renewable resources. Environmental Research, 205, 112458. https://doi.org/10.1016/j.envres.2021.112458
- Shahbaz, M., Solarin, S. A., Sbia, R., & Bibi, S. (2015). Does energy intensity contribute to CO2 emissions? A trivariate analysis in selected African countries. Ecological Indicators, 50, 215–224. https://doi.org/10.1016/j.ecolind.2014.11.007
- Shahbaz, M., Uddin, G. S., Rehman, I. U., & Imran, K. (2014). Industrialization, electricity consumption and CO2 emissions in Bangladesh. Renewable and Sustainable Energy Reviews, 31, 575–586. https://doi.org/10.1016/j.rser.2013.12.028
- Shan, S., Umar, M., & Mirza, N. (2022). Can Robo advisors expedite carbon transitions? Evidence from automated funds. Technological Forecasting and Social Change, 180, 121694. https://doi.org/10.1016/j.techfore.2022.121694
- Sim, J. (2018). The economic and environmental values of the R&D investment in a renewable energy sector in South Korea. Journal of Cleaner Production, 189, 297–306. https://doi.org/10.1016/j.jclepro.2018.04.074
- Sinha, A., Gupta, M., Shahbaz, M., & Sengupta, T. (2019). Impact of corruption in public sector on environmental quality: Implications for sustainability in BRICS and next 11 countries. Journal of Cleaner Production, 232, 1379–1393. https://doi.org/10.1016/j.jclepro.2019.06.066
- Su, C.-W., Pang, L.-D., Tao, R., Shao, X., & Umar, M. (2022). Renewable energy and technological innovation: Which one is the winner in promoting net-zero emissions? Technological Forecasting and Social Change, 182, 121798. https://doi.org/10.1016/j.techfore.2022.121798
- Su, C.-W., Umar, M., & Khan, Z. (2021). Does fiscal decentralization and eco-innovation promote renewable energy consumption? Analyzing the role of political risk. The Science of the Total Environment, 751, 142220. https://doi.org/10.1016/j.scitotenv.2020.142220
- Sun, I., & Kim, S. Y. (2017). Energy R&D towards sustainability: A panel analysis of government budget for energy R&D in OECD countries (1974–2012). Sustainability, 9(4), 617. https://doi.org/10.3390/su9040617
- Taghizadeh-Hesary, F., Rasoulinezhad, E., Yoshino, N., Sarker, T., & Mirza, N. (2021). Determinants of the Russia and Asia–Pacific energy trade. Energy Strategy Reviews, 38, 100681. https://doi.org/10.1016/j.esr.2021.100681
- Tang, C. F., Tan, B. W., & Ozturk, I. (2016). Energy consumption and economic growth in Vietnam. Renewable and Sustainable Energy Reviews, 54, 1506–1514. https://doi.org/10.1016/j.rser.2015.10.083
- Trotta, G. (2020). Assessing energy efficiency improvements and related energy security and climate benefits in Finland: An ex post multi-sectoral decomposition analysis. Energy Economics, 86, 104640. https://doi.org/10.1016/j.eneco.2019.104640
- Tufail, M., Song, L., Adebayo, T. S., Kirikkaleli, D., & Khan, S. (2021). Do fiscal decentralization and natural resources rent curb carbon emissions? Evidence from developed countries. Environmental Science and Pollution Research International, 28(35), 49179–49190. https://doi.org/10.1007/s11356-021-13865-y
- Tufail, M., Song, L., Ali, S., Wahab, S., & Hassan, T. (2022). Does more immigration lead to more violent and property crimes? A case study of 30 selected OECD countries. Economic Research-Ekonomska Istraživanja, 1–19.
- Ulucak, R., & Khan, S.-U. (2020). Relationship between energy intensity and CO2 emissions: Does economic policy matter? Sustainable Development, 28(5), 1457–1464.
- Umar, M., Farid, S., & Naeem, M. A. (2022). Time-frequency connectedness among clean-energy stocks and fossil fuel markets: Comparison between financial, oil and pandemic crisis. Energy, 240, 122702. https://doi.org/10.1016/j.energy.2021.122702
- Umar, M., Ji, X., Kirikkaleli, D., & Xu, Q. (2020). COP21 roadmap: Do innovation, financial development, and transportation infrastructure matter for environmental sustainability in China? Journal of Environmental Management, 271, 111026. https://doi.org/10.1016/j.jenvman.2020.111026
- Umar, M., Ji, X., Mirza, N., & Naqvi, B. (2021). Carbon neutrality, bank lending, and credit risk: Evidence from the Eurozone. Journal of Environmental Management, 296, 113156. https://doi.org/10.1016/j.jenvman.2021.113156
- Umar, M., Mirza, N., Hasnaoui, J. A., & Rochoń, M. P. (2022). The nexus of carbon emissions, oil price volatility, and human capital efficiency. Resources Policy, 78, 102876. https://doi.org/10.1016/j.resourpol.2022.102876
- Umar, M., Riaz, Y., & Yousaf, I. (2022). Impact of Russian-Ukraine war on clean energy, conventional energy, and metal markets: Evidence from event study approach. Resources Policy, 79, 102966. https://doi.org/10.1016/j.resourpol.2022.102966
- Vidotto, J. D. F., Ferenhof, H. A., Selig, P. M., & Bastos, R. C. (2017). A human capital measurement scale. Journal of Intellectual Capital, 18(2), 316–329. https://doi.org/10.1108/JIC-08-2016-0085
- Wong, S. L., Chia, W.-M., & Chang, Y. (2013). Energy consumption and energy R&D in OECD: Perspectives from oil prices and economic growth. Energy Policy, 62, 1581–1590. https://doi.org/10.1016/j.enpol.2013.07.025
- Wu, T., Yang, S., & Tan, J. (2020). Impacts of government R&D subsidies on venture capital and renewable energy investment—An empirical study in China. Resources Policy, 68, 101715. https://doi.org/10.1016/j.resourpol.2020.101715
- Xu, B., & Lin, B. (2017). Does the high–tech industry consistently reduce CO2 emissions? Results from nonparametric additive regression model. Environmental Impact Assessment Review, 63, 44–58. https://doi.org/10.1016/j.eiar.2016.11.006
- Xu, S.-C., He, Z.-X., Long, R.-Y., Shen, W.-X., Ji, S.-B., & Chen, Q.-B. (2016). Impacts of economic growth and urbanization on CO2 emissions: Regional differences in China based on panel estimation. Regional Environmental Change, 16(3), 777–787. https://doi.org/10.1007/s10113-015-0795-0
- Yang, S., Chen, Z., Umar, M., & Khursheed, A. (2022). Environmental regulation and high-quality sustainable development of China’s economy—An empirical study based on a spatial durbin model and threshold model. Economic Research-Ekonomska Istraživanja, 35(1), 5699–5718. https://doi.org/10.1080/1331677X.2022.2035243
- Yao, Y., Ivanovski, K., Inekwe, J., & Smyth, R. (2019). Human capital and energy consumption: Evidence from OECD countries. Energy Economics, 84, 104534. https://doi.org/10.1016/j.eneco.2019.104534
- Yarovaya, L., Mirza, N., Abaidi, J., & Hasnaoui, A. (2021). Human capital efficiency and equity funds’ performance during the COVID-19 pandemic. International Review of Economics & Finance, 71, 584–591. https://doi.org/10.1016/j.iref.2020.09.017
- Yu, B., Li, C., Mirza, N., & Umar, M. (2022). Forecasting credit ratings of decarbonized firms: Comparative assessment of machine learning models. Technological Forecasting and Social Change, 174, 121255. https://doi.org/10.1016/j.techfore.2021.121255
- Yuan, X., Su, C.-W., Umar, M., Shao, X., & LobonŢ, O.-R. (2022). The race to zero emissions: Can renewable energy be the path to carbon neutrality? Journal of Environmental Management, 308, 114648. https://doi.org/10.1016/j.jenvman.2022.114648