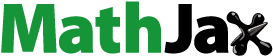
Abstract
This study examined the effect of digital financial inclusion in reducing the urban–rural income inequality in China. Based on city-level panel data, the results showed that digital financial inclusion narrowed the urban–rural income gap significantly by boosting economic growth. The results were robust when the core explained variables were replaced. Heterogeneity analysis showed that digital financial inclusion indicates regional differences in narrowing the urban–rural income gap. This study puts forward corresponding countermeasures for the development of digital financial inclusion and adds to the research on this very topical subject.
1. Introduction
China’s economy has expanded rapidly since its reform and opening up to foreign investment and trade (Huang et al., Citation2019; Sheng et al., Citation2021; Wuttke, Citation2017). However, with this rapid economic growth, the unbalanced development between urban and rural areas, which may deteriorate social stability and fair governance, has gradually become prominent (Koh et al., Citation2020). Urban–rural income inequality plays an important role in economic shocks on democracy (Kotschy & Sundeshow, Citation2021) and may lead to labour–market polarisation (Furusawa et al., Citation2020). Thus, the focus of the Chinese government shifted to balanced and coordinated development, and narrowing the gap between urban and rural areas has become an important goal (Yu & Wang, Citation2021).
Existing research generally concurs that financial development can help alleviate enterprises’ external financing constraints (Levine, 2005). Financial support is an effective means to reduce poverty and increase income, and a lack of finance may lead to a widening income gap owing to financial constraints (Yu & Lu, Citation2021). Finance can optimise resource allocation (Tian et al., Citation2021), reduce financing constraints (Madrid-Guijarro et al., Citation2016), promote household consumption (Li et al., Citation2020), and increase economic growth (Ye et al., Citation2021).
Financial inclusion refers to a financial system that can effectively and comprehensively provide services to all social classes and groups. Financial inclusion emphasises a wide range of service targets and low-cost financial service products. The main service targets include rural residents, small and medium-sized enterprises, urban low-income groups, and older adults. Its original intention was to enhance financial accessibility services by improving financial infrastructure and providing convenient and low-cost financial services in underdeveloped areas and to low-income individuals (Guo et al., Citation2019). Financial inclusion increases low-income groups’ access to finance, thereby increasing their income and narrowing the urban–rural income gap (Satya & Chakravarty, Citation2013; Schmied & Marr, Citation2016). An inclusive financial market provides affordable and equitable access to financial products to all households and entrepreneurs, especially to the most marginalised ones (Corrado & Corrado, Citation2017).
Digital technologies have played a positive role in making finance more inclusive (Ji et al., Citation2021; Khera et al., Citation2022), improving farmers’ income (Liu et al., Citation2021) as well as leading to inclusive economic growth (Yi & Zhou, Citation2018). The resultant new business model that combines finance and digital technologies is called ‘digital finance’ (Huang & Huang, Citation2018). Differing from traditional financial services, digital finance can overcome geographical barriers (Zheng, Citation2014) and enhance the economic correlation between regions (Guo et al., Citation2019). Moreover, it stimulates the entrepreneurial behaviour of rural residents and equalises entrepreneurial opportunities (Zhang et al., Citation2019).
As the core of information and communication technology, the Internet has developed rapidly since the 1990s (Li et al., Citation2020). It has penetrated all aspects of economic development, and a digital economic development model has gradually been established (Bican & Brem, Citation2020; Cruz-Jesus et al., Citation2017). The rapid development of digital technologies such as big data and cloud computing has laid a solid foundation for Digital Financial Inclusion (DFI). The concept of DFI was first introduced in 2016 at the G20 Summit in Hangzhou. As a combination of digital technology and financial inclusion, DFI refers to ‘all actions that promote financial inclusion through digital financial services’.Footnote1 In 2019, the Ministry of Industry and Information Technology of China defined DFI as ‘the provision of equal, effective, comprehensive and convenient financial products and services to all people, especially to the low-income people in urban areas, people in rural and remote areas and micro and small enterprises, under the premise of cost control and sustainable models, with various digital technologies as a condition for realisation’.
DFI is a new type of financial industry that uses digital technologies on the basis of financial inclusion; it broadens the service scope, improves the accessibility, and reduces the cost of financial inclusion and makes it more commercially sustainable. DFI enhances the risk management ability based on technology innovations, reduces information asymmetry, lowers financing thresholds by recording customers’ payments of utility bills and living expenses, benefits users of financial services and economic sustainability (Ozili, Citation2018), and leads to social inclusion (Cnaan et al., Citation2021).
DFI has experienced rapid development in China. At the provincial level, the DFI index has grown from around 50 in 2011 to nearly 320 in 2019. In addition to this inclusive financial index, China’s digitalisation level has risen rapidly. The incomes of urban and rural residents have also increased considerably following the development of the economy. In terms of the urban–rural disparity shown in , the Theil index and the urban–rural income gap at the provincial level peaked in 2004, and has been showing an annual decreasing trend annually ever since.
Figure 1. Provincial Digital Financial Inclusion and Theil index 2011-2019.
Source: created by the authors based on the annual China statistical yearbooks.

According to the 2020 China Inclusive Finance Indicators Analysis Report, inclusive financial services like small and micro loans have grown rapidly. As shown in , the balance of inclusive small and micro loans was approximately $2.16 trillion by the end of 2020, having increased by 30.3% since 2019. In 2020, inclusive small and micro loans supported a total of 32.28 million small and micro business entities, a figure that had also increased by 19.4% since 2019.
Figure 2. Inclusive finance loan balance and small and micro financial inclusion growth rate.
Source: created by the authors based on the annual China statistical yearbooks.

The literature has hitherto considered DFI from various perspectives. Zhang et al. (Citation2019) postulated that DFI could effectively promote innovation and entrepreneurship, whereas Yi and Zhou (Citation2018) noted that DFI could increase household consumption. Li and Feng (Citation2020) and Song (Citation2017) opined that DFI narrows the income gap between urban and rural areas. Ren and Li (Citation2019) asserted that DFI could promote the inclusive development of rural areas. Based on the financial deepening theory, Wang et al. (Citation2022) showed that financial inclusion positively enhances green economic efficiency.
Almost all existing studies related to the urban–rural income gap in China are based on empirical analyses at the provincial level, with only a few studies focusing on the city level. In addition, when existing studies remeasure the urban–rural income gap, they mainly use the absolute income gap, which is the difference between urban and rural residents’ per capita disposable income, and the Gini coefficient derived from Lorenz curve as explained variables. However, these variables may ignore the income gap among regions as well as the dynamic changes caused by urban–rural population mobility. The Theil index is an important tool and a widely used indicator to study the regional unevenness, income distribution discrepancy, and carbon inequality and it appears frequently in the academic literature (Clarke-Sather et al., Citation2011; Du et al., Citation2020; Remuzgo & Sarabia, Citation2020). The property of decomposability of the index makes it particularly useful in measuring socio–economic inequality (Sarabia et al., Citation2017). Thus, this study used city-level panel data to study the effect of DFI on the urban–rural income gap by using the Theil index to measure this gap, as this index can better reflect the dynamic changes that occur. Factors affecting income inequality have been widely investigated in existing studies (C. Chen, Citation2016; D. Chen & Ma, Citation2022; Greaney & Li, Citation2017; Zhu et al., Citation2020). As digital finance, which relies on innovative technologies such as intelligent algorithms, big data and cloud computing, further expands the reach and depth of services of financial inclusion, DFI may become a new path for narrowing the urban–rural income gap and addressing regional imbalances. Based on panel data of DFI in China that were collected at the city level, we hope to analyse the possible impact of DFI on narrowing the urban–rural income gap from a new perspective and provide experiences for other developing countries, which is the motivation of this research. This study further analysed the mechanism by which DFI narrows the urban–rural income gap.
The primary contribution of this study to the literature is that, to the best of our knowledge, this study is the first to consider the effect of DFI on both alleviating poverty and narrowing the urban–rural gap by using city-level panel data based on the Theil index during 2011–2018. Second, this study is expected to provide insightful information and act as a beneficial reference for other countries and regions.
2. Theoretical framework and hypothesis (H)
2.1. Assumptions of the model
Smith’s theorem states that labour division and specialisation enable people to produce different products to meet diverse needs (Kamei, Citation2014; Legros et al., Citation2014; Smith, Citation2008). Producers must pay transaction fees during product transactions, and people are looking for an equilibrium point between specialisation and transaction costs. Transaction cost is a key factor in labour division and specialisation, and labour division will lead to a geometric increase in transactions costs (Becker & Murphy, Citation1992). When the benefits of labour division and specialisation outweigh the transaction fees, an autarky will evolve through an economy of labour division and specialisation, as people will conduct transactions through market behaviour to meet their diversified consumption needs.
This study assumes that an economy consists of a total number of producer-consumers (P), with no pure producers or pure consumers, and people produce final product (Y) by inputting labour (L) and capital (C). Among them, capital is an intermediate product provided by financial institutions, which financial institutions need to invest in labour L when providing financial products and services to consumers. Under the specialised division of labour, there are transaction costs for the transaction of product Y and capital product C. Assuming e∈(0,1) is the transaction efficiency coefficient, the higher the transaction efficiency, the lower the transaction cost will become. Assuming that each producer-consumer’s monetary income from selling products is equal to the sum of monetary expenditure for buying goods (i.e., no savings and no credit), there would be little incentive for production, as the entrepreneur would not be making any profit.
2.2. Model establishment
2.2.1. Benchmark model
Following Yang (Citation2001) and Yang and Ng (Citation1993), the following benchmark model of specialised decision-making by producer-consumers has been constructed, based on the above assumptions:
Objective function:
(1)
(1)
Production function:
(2)
(2)
Aggregate output:
(3)
(3)
(4)
(4)
Time constraints:
(5)
(5)
(6)
(6)
In function (1), u represents the utility of the producer-consumer. The utility maximisation of the producer-consumer depends on self-sufficiency y of the final product, and purchase amount
is the transaction efficiency of the final product.
In function (2), is the amount of labour required to produce y,
is the learning cost during the specialised production of final product y (0 < a < 1),
is the amount of labour required to produce capital product c, and b is the learning cost in the process of specialised production of capital goods (0 < b < 1).
In function (3), is the total output of the final product, including self-sufficiency y plus market sales
According to the Leontief production function, total output depends on the minimum value of capital and labour input, where c and represent the self-sufficiency and market supply of capital goods, respectively, and
is the transaction efficiency of capital goods.
In function (4), capital product includes the self-sufficiency c plus market supply
In function (5), labour is equitably allocated between capital goods and final products; that is, time constraint is 1 owing to the limited labour endowment.
In function (6), according to Walras’s law, the sum of the monetary income of each producer-consumer from the sale of the product is equal to the sum of the monetary expenditure of the purchased product. and
denote the prices of capital and final goods, respectively.
Producer-consumers need to consider two decision-making models: the self-sufficient decision-making model (Autarky, A) and specialised division of labour decision-making model (Professionalisation, P). As this study analyses the effect of DFI on incentivising consumers-producers, it mainly considers the specialised division of labour model; that is, producer-consumers use financial services to execute a specialised division of labour to generate more income.
DFI improves transaction efficiency of capital products and services. This study further conducted an ultra-marginal analysis of the model to examine the effect of financial product efficiency
on producer-consumer utility u.
2.2.2. Ultra-marginal decision model
Under the specialised production decision-making model, the super-marginal decision-making mode of the manufactured goods’ production department is The production department produces y by purchasing financial product c from financial institutions. Then,
= 1,
The producer’s target utility functions for product y are as follows:
(7)
(7)
(8)
(8)
(9)
(9)
These can be further denoted as follows:
(10)
(10)
(11)
(11)
(12)
(12)
Under the specialised production decision-making model, the ultra-marginal decision-making model of financial institutions is Financial institutions satisfy the producer-consumer purchasing y’s consumption demand by providing self-produced products and services c. At this stage,
= 1,
and the decision-making objective utility function of the financial institutions are as follows:
(13)
(13)
(14)
(14)
(15)
(15)
This can be further denoted as follows:
(16)
(16)
(17)
(17)
According to the equality of utility and market clearing conditions at general equilibrium, production sector y and financial institution c must satisfy ,
or
where
and
represent the number of labourers in the financial and productive sectors, respectively. To achieve increased income under the condition of satisfying general equilibrium, there must be an increase in the income of people with the same per capita income. The formula for solving people’s per capita income under general equilibrium conditions is as follows:
(18)
(18)
It can be concluded that per capita utility u is an increasing function of transaction efficiency of financial institutions; that is, the improvement of transaction efficiency of financial institutions will increase people’s per capita income. Therefore, DFI reduces the cost of financial services by facilitating accessibility for small- and medium-sized market players and low-income persons, thereby increasing the disposable income of low- and middle-income individuals and narrowing the urban–rural gap. Macroeconomic theory holds that finance, as one of the production factors, can effectively promote economic growth, which can significantly increase national income. Therefore, H1 is proposed:
H1. DFI is conducive to narrowing the urban–rural income gap.
H2. Different DFI levels generate different effects in narrowing the urban–rural income gap.
H3. DFI narrows urban–rural income gap via boosting economic growth by benefiting most rural households.
H4. DFI may lead to a disequilibrium effect on urban–rural income inequality among regions.
H5. Coverage breadth, depth of use, and level of digitisation of DFI may generate different effects on narrowing urban–rural income inequality.
3. Empirical design
3.1. Model construction
To empirically test the theoretical hypothesis listed above, it is necessary to test whether DFI can narrow the urban–rural income gap by increasing the income of rural households. The mediating effects model is widely used in empirical studies in the field of social science research (Rucker et al., Citation2011; Zhao et al., Citation2010). Compared to similar studies that simply analyse the effects of independent variables on dependent variables, mediating effect models allow for the analysis of the processes and mechanisms of independent variables on dependent variables, and therefore mediating effect models are not only methodologically sound but also usually yield more in-depth results (Zhang, Citation2019). Following Liu et al. (Citation2021), the mediating effect model below is established:
(19)
(19)
(20)
(20)
(21)
(21)
Function (19) analyses the relationship between the urban–rural income gap and DFI. In function (19), is the explained variable, expressed as the urban–rural income gap measured by the Theil index.
is the core explanatory variable, represented by the development level of DFI in each city.
is a set of control variables.
and
are the random disturbance terms of each model, respectively. i represents the city, and t the year. To verify the mechanism that digital inclusive finance can narrow the urban–rural income gap, the mechanism models, and function (20) and (21) are established.
in function (20) is the intermediary variable, which is set as economic growth in this study, and other variables are consistent with formula (19).
3.2. Data and variables
The data in this study are mainly from The Institute of Digital Finance of Peking University, which selected hundreds of millions of pieces of micro-data from a representative digital financial institution in China to compile The Peking University Digital Financial Inclusion IndexFootnote2 of China, covering 337 cities in Mainland China from 2011–2018. The index charts DFI development trends in different regions of China. The data used to calculate the Thiel index and proxies for control variables were obtained from the China Urban Statistical Yearbook, which provides a comprehensive dataset of the economic and social development of Chinese cities from 2012 to 2019. The period of this study is 2011–2018, and after removing sample cities with incomplete data, we obtained a panel data of 209 cities for 8 years, with a total population of 1,672 samples. The variables used in the study are as follows.
3.2.1. Explained variables and explanatory variables
The absolute or relative value of income gap or Gini coefficient are usually adopted as indicators of income gap between urban and rural sectors. This study used the Theil index to measure the urban–rural income gap among Chinese cities, as it can reflect the sensitivity of population changes to the income changes of both high- and low-income groups. The specific calculation method is as follows:
(22)
(22)
In function (22), j = 1, 2 represents urban and rural residents, respectively; is the disposable income of urban (j = 1) or rural (j = 2) in year t
represents the total disposable income in year t;
is the urban (j = 1) or rural (j = 2) population in year t; and
represents the total population in year t. Generally, the smaller the Theil index is, the smaller the urban–rural income gap will be.
The DFI index is based on Peking University’s DFI index, based on big data from the Ant Financial Services Group. Released in 2019, the index covers the DFI index for various Chinese cities from 2011 to 2018. It objectively and comprehensively analyses the development of DFI in China from the multi-level and diversity perspectives and makes the development of DFI in China more visible. It also includes provincial, municipal, and county-level indices, thereby describing the development of DFI in China in detail.
The index includes three sub-dimensions of digital financial service: coverage, depth of use, and degree of digitisation. Among them, coverage considers third-party payment accounts bound to bank cards. First, because the account holder can better manage materials and financing through the third-party payment function after binding the bank card, the coverage of financial services will be wider. Second, the depth of use considers account holders’ use of digital finance in conducting services such as payment, credit, investment, purchase of monetary funds or insurance, and uses customer activity and actual total usage to measure the real use of digital finance. Third, the degree of digitalisation results from the convenience of inclusive finance and its low cost, whereas the credit perspective reflects the value of DFI. The larger the index, the better the development of DFI, and the more opportunities for farmers and low-income groups to enjoy financial products and services, which are more likely to increase income and narrow the urban–rural income gap.
The time span of the city-level DFI index is 2011–2018. Overall, China’s digital finance has grown rapidly during this period. According to the trend of the index from 2012 to 2018 (), the country’s municipal DFI index shows decreasing development from the east to the west. The development of the DFI in the northeast region is higher than that in the western region.
3.2.2. Controlled variables
Based on the existing research on inclusive finance and the urban–rural income gap, this study selected economic growth, urbanisation rate, fiscal expenditure structure, foreign investment, and fiscal expenditure structure as control variables as follows:
Regional economic development was measured by the per capita gross domestic product (GDP) of each region;
Urbanisation was calculated by the proportion of the permanent urban population in the total population in each region;
Government fiscal expenditure was measured by the proportion of a city government’s fiscal expenditure of GDP;
Foreign investment was expressed as the logarithm of foreign direct investment in each city;
Finance was represented by each city’s loan balance; and
The urban–rural income gap was measured as the difference between the disposable incomes of urban and rural residents ().
Table 1. Variable list.
4. Empirical analysisFootnote3
4.1. Statistical description
The statistical characteristics of all variables are shown in . To avoid spurious regression analysis caused by data instability, LLC test method is adopted to conduct panel unit-root test before empirical analysis, and the result is shown in Appendix . All variables have passed the significance test and are stationary. Then, Kao test is used to conduct panel co-integration test. The result in Appendix shows there is a long-term stable relationship among variables.
Table 2. Statistical characteristics of the variables.
Additionally, this study evenly divided the DFI index into four intervals, as shown in , for which the minimum value was 17.02 and the maximum 302.98. In the above four intervals, multiple differences were observed in the DFI index, regional economic development, Theil index, and urban–rural income in terms of mean and sample size. It can be gleaned from that, with the improvement of the DFI index, local economic development has gradually increased on average, whereas the Theil index and the urban–rural income gap have declined steadily. The DFI index of most cities in China was between 88.51 and 231.51, accounting for 71% of all cities.
Table 3. Group description of digital financial inclusion and Theil index.
4.2. Baseline regression analysis
Based on the above theoretical analysis, shows the benchmark regression analysis results of the DFI index on the urban–rural income gap. The effects of DFI on regional economic growth and the Theil index were tested and are presented in columns (1) and (2) of , respectively. According to the results in column (1), the total utility of DFI in promoting economic growth is significantly positive, which is consistent with the correlation between DFI and regional economic growth in . Column (2) indicates that DFI significantly reduces the urban–rural income gap, denoted by the Theil index. To deal with endogeneity, we introduced the first-order lag value of DFI as an instrumental variable in columns (3) and (4) of , and the results were consistent with the basic regression analysis.
Table 4. Benchmark regression.
From the regression analysis results of models (1) to (4), DFI has a significant effect on narrowing the urban–rural income gap regardless of methods applied or control variables added. Therefore, H1 is supported.
4.3. Group test
To investigate the effect of DFI on the promotion of economic development and narrowing the urban–rural income gap under different development conditions, we divided the overall sample into two groups according to the average value of the DFI index: a lower degree of DFI development and a higher degree of DFI development. According to the results in columns (1) and (2) of , DFI still positively promotes economic growth and narrows the urban–rural income gap in cities with low DFI development. Columns (3) and (4) show the regression analysis results after adding the first-order lag of DFI as an instrumental variable, which is consistent with the previous conclusion in .
Table 5. Regression result of low DFI group.
According to the results in columns (1) and (2) of , the advancement of DFI in cities with better DFI development has significantly promoted economic growth and narrowed the urban–rural income gap. Columns (3) and (4) present the regression analysis results after adding the first-order lag of DFI as an instrumental variable, which is consistent with the previous conclusions. A comparison between and reveal that DFI promotes regional economic growth more successfully in cities with a lower degree of DFI development and indicates the existence of different effects of DFI levels on narrowing urban–rural income gap. This verifies H2.
Table 6. Regression result of high DFI group.
4.4. Robustness test
To test the robustness of the regression analysis results, we adopted three robustness tests. First, to test whether the empirical results are equally robust across variables and samples, we replaced the explained variable with another variable, gap, which represents the per capita disposable income of urban residents divided by the per capita disposable income of rural residents. Moreover, we used gap as a moderator variable to test the effect of DFI on regional economic growth when there was a gap between urban and rural areas. Columns (1)–(3) of show that the promotion effect of DFI on regional economic growth was still significant and had a significant effect in narrowing the urban–rural income gap.
Table 7. Robustness test.
Second, to avoid potential endogeneity of the regression results, we adopted the instrumental variable regression method. The key to the instrumental variables approach is to find the right instrumental variables; following Lokshin and Mohnen (Citation2013), we used the first-order lag of DFI as the instrumental variable. Columns (4)–(6) display the results when the first-order lag of DFI was added as an instrumental variable, which is consistent with the previous regression analysis results, thus indicating that the regression analysis results are robust.
Third, the year 2015 was added as a policy variable to exclude possible interference from other factors and to test whether the impact of DFI on narrowing the urban–rural income gap might have been overestimated. Rural entrepreneurship is an important way to increase farmers’ income (Ji et al., Citation2021; Zhu et al., Citation2022). The push for mass entrepreneurship and innovation (MEI) was first put forward by the Premier Li Keqiang at the Summer Davos Forum held in Tianjin in 2014. The goal of MEI was to encourage people nationwide to start their own businesses, especially to promote farmers’ entrepreneurship, increases farmers’ income, and reduces the urban–rural income gap.Footnote4 Due to possible delays in the implementation of the policy across China and the fact that the Chinese government launched the policy towards the end of 2014, we used the year 2015 as the policy year to analyse whether the implementation of the policy would affect the role of DFI in reducing the urban–rural income gap. According to the regression results, the effect of DFI on narrowing the urban–rural income gap remained significant after we add the 2014 policy, which shows that the regression results above are stable (see Appendix for details).
Fourth, we examined the impact of DFI on narrowing the rural–urban gap at different sample periods by artificially dividing the sample period into three time periods: 2011–2014, 2015–2018, and 2013–2016 (see Appendix for details). It can be seen that DFI significantly reduced the urban–rural income gap in all time periods, again validating the robustness of the empirical results.
5. Mechanism and heterogeneity analysis
5.1. Mechanism analysis
The benchmark regression analysis and robustness tests above demonstrate the significant effect of DFI in narrowing the urban–rural income gap. The theoretical part showed that DFI increases the accessibility and coverage of financial services for low-income groups. On this basis, this study examined the mechanism by which DFI narrowed the urban–rural income gap from the perspective of economic growth. Column (1) of shows that DFI significantly promotes regional economic growth; specifically, in columns (2) and (3), the regression analysis coefficients of DFI and GDP are both significantly positive, indicating that the development of DFI has effectively promoted economic growth, and then economic growth has contributed to an increase in the average income of rural people. The regression analysis coefficients of DFI and GDP in columns (4) and (5) are both significantly negative, indicating that the development of DFI significantly promotes economic growth. Subsequently, economic growth has effectively narrowed the urban–rural gap. This finding supports H3, indicating that digital inclusive finance narrows urban–rural income gap via boosting economic growth, where most households in rural areas benefit from.
Table 8. Mechanism channel analysis.
5.2. Heterogeneity analysis
5.2.1. Regional heterogeneity analysis
Considering regional fixed effects, the area characteristic variable (area) was assigned values from 1 to 4 for the east, middle, west, and northeast, respectively, according to the official government division method. According to columns (1)–(4) of , the impact of DFI on the Theil index of the cities in eastern, central, western, and north-eastern China shows different effects. DFI has the greatest effect on the urban–rural income gap in the central region, and the effects in the eastern and western regions are analogous. However, DFI is not significant in reducing the urban–rural income gap in north-eastern China, thus verifying H4.
Table 9. Heterogeneity test.
5.2.2. Heterogeneity analysis of sub-indicators
The main purpose of inclusive finance is to expand the scope and coverage of financial services so that farmers and low-income individuals can enjoy financial products and services at a lower cost, thereby narrowing the urban–rural income gap through rural poverty reduction. Therefore, this study used the three sub-indicators of the DFI index—coverage breadth, depth of use, and digitalisation degree—to test the heterogeneous effect of DFI in narrowing the urban–rural income gap. Columns (1), (3), and (5) of examine the effects of coverage, usage, and digitisation level, respectively, on narrowing the urban–rural gap when no control variables were added. In columns (2), (4), and (6), after adding control variables, DFI significantly narrowed the urban–rural income gap in each city through the effects of coverage, usage, and digitisation level. Overall, the effect of two sub-indicators of DFI, coverage breadth and depth of use, has a more pronounced effect on narrowing the urban–rural income gap than that of the digitisation level.
Table 10. Heterogeneity Test.
6. Discussion
The significant negative relationship between DFI and the Theil index indicates that DFI has an actual effect on narrowing the urban–rural income gap, thus verifying H1. By dividing DFI into high and low development levels to study the effect of the different development levels on the urban–rural income gap, this study finds that, in cities with low DFI development levels, DFI can better promote economic growth and reduce the urban–rural income gap to a greater extent. Therefore, the above findings validate H2.
The possible explanation is three-fold. First, DFI not only increases the coverage of financial services and improves the financial service network, but also reduces the risks and costs of financial services and improves the availability of financial services at the individual level. Cities with higher DFI development level typically have a better economic development foundation and development momentum. Compared to cities with a higher DFI development level, those with a lower DFI development level cannot achieve stable economic growth because of a lack of economic momentum. As a new driving force for development, DFI has brought a new development impetus to relatively underdeveloped regions. When the financing needs of innovative small- and medium-sized enterprises in underdeveloped regions are improved, the production vitality of the private economy will be stimulated, and the supply structure of the real economy will be optimised. Second, when the capital needs of low-income groups are met, the consumption vitality of residents will also be stimulated, thereby stimulating economic growth by increasing domestic demand. Therefore, under the balance of supply and demand in the real economy, DFI can not only optimise the financial supply structure but also realise the ideal co-development of finance and the real economy by optimising the supply and demand structure of the real economy. Third, it is possible that the lower the level of economic development in a region, the wider and deeper the financial exclusion. Therefore, the greater the space for DFI to develop, the better the effect of promoting economic growth and narrowing the urban–rural income gap in the region.
To address the concern of narrowing the urban–rural income gap, the per capita income of residents in rural areas must be increased. The mediating effect model shows that DFI narrows the urban–rural income gap by increasing per capita income in rural areas, thus validating H3. By dividing all cities into eastern, central, western, and north-eastern regions, this study was able to examine the regional heterogeneity effect of DFI on narrowing the urban–rural income gap. We find that DFI has the greatest effect on narrowing the urban–rural income gap in the central region. The effect is similar in the eastern and western regions. However, it is smallest and most insignificant in the north-eastern region. Therefore, H4 is also verified.
By analysing the effect of the three sub-dimensions of DFI, coverage, depth of use, and degree of digitalisation on narrowing the urban–rural income gap, this study finds that the two sub-indicators, namely breadth of coverage and depth of use, have similar effects on narrowing the urban–rural income gap. The effect of the degree of digitisation on narrowing the urban–rural income gap is smaller than that on the other two sub-indicators; thus, H5 is verified.
The results of this study are consistent with the findings of previous studies (Li et al., Citation2022; Li et al., Citation2022; Ji et al., Citation2021). Unlike existing studies, based on datasets from the Digital Finance Research Centre of Peking University and the China Urban Statistics Yearbook, this study investigates the impact of DFI on narrowing the urban–rural income gap based on the Thiel index in China. We attempted to explore a new path to narrow the urban–rural income gap from the perspective of new emerging DFI, revealing the regional heterogeneity of the impact of DFI on the urban–rural income gap.
7. Conclusions and policy implications
Based on the panel data of 209 Chinese prefecture-level cities from 2011 to 2018, this study used the Peking University DFI index to empirically analyse whether DFI can narrow the urban–rural income gap. We found that (1) the development of DFI has significantly improved regional economic growth and narrowed the urban–rural income gap; (2) compared with cities with more advanced DFI, the development of DFI was relatively low, and in low-level cities, DFI played a greater role in narrowing the urban–rural gap; and (3) the mechanism test showed that DFI mainly increased the income of rural residents by promoting regional economic development, thus effectively narrowing the urban–rural income gap. A qualitative analysis found regional differences in the effect of DFI on narrowing the urban–rural gap. Among them, DFI had the greatest effect on narrowing the urban–rural income gap in the central region, followed by the gap in the eastern and western regions; however, it had no significant effect on the gap in the north-eastern region. The three sub-dimensions of DFI—coverage, usage, and digitisation level—had a significant effect on narrowing the income gap between urban and rural areas. Coverage and usage had a greater effect on narrowing the gap than the digitisation level.
Accordingly, our policy recommendations are as follows. First, DFI in the central and western regions should be further encouraged to increase the availability of financial services for the low- and middle-income groups, especially for people in rural areas. Second, the construction of information platforms should be strengthened to reduce financial institutions’ cost of acquiring customers through improving the credit recognition of low- and middle-income groups. Third, while improving the accessibility and affordability of financial services for rural people with a relatively low social status, it is suggested that financial institutions should retain the non-performing loan rate at a low level.
However, our study is not without limitations. The China Urban Statistical Yearbook includes data on the disposable income of urban and rural residents but does not contain relevant information on entrepreneurship. Thus, in terms of mechanism testing, this study cannot verify whether DFI can effectively increase farmers’ income by helping rural residents to start businesses, thereby narrowing the urban–rural income gap. Therefore, in future studies, the mechanism of DFI on narrowing the urban–rural gap based on the entrepreneurship of urban and rural residents should be explored.
Authors’ contributions
Hongcheng Shen designed this study, carried out the empirical work and the data analysis, and drafted the manuscript. Ting Luo wrote the literature review. Xia Zhang and Zhixiong Gao participated in the design of the study, Weikun Zhang and Yenching Chuang made revisions and finalized this study. All authors read and approved the final manuscript.
Acknowledgements
The authors thank the Young Talent Program of Taizhou University and the 211 Third-Level Talents Program of Taizhou City for their support. We also thank Editage (www.editage.cn) for English language editing.
Disclosure statement
The authors report there are no competing interests to declare.
Data availability statement
The data that support the findings of this study are available from the corresponding author upon reasonable request.
Additional information
Funding
Notes
1 https://www.gpfi.org/publications/g20-high-level-principles-digital-financial-inclusion (accessed on 19 Oct. 2022).
2 We collected and sorted the DFI data from the following link: https://en.idf.pku.edu.cn/achievements/seriesofdigitafinanceindexes/490847.htm
3 Empirical analyses were conducted using STATA MP Version 16.0.
4 http://english.www.gov.cn/premier/news/2017/07/12/content_281475723086902.htm (accessed on 19 Oct. 2022).
References
- Becker, G., & Murphy, K. (1992). The division of labor, coordination costs, and knowledge. The Quarterly Journal of Economics, 107(4), 1137–1160. https://doi.org/10.2307/2118383
- Bican, P. M., & Brem, A. (2020). Digital business model, digital transformation, digital entrepreneurship: Is there a sustainable "digital"? Sustainability, 12(13), 5239. https://doi.org/10.3390/su12135239
- Chen, C. (2016). The impact of foreign direct investment on urban-rural income inequality Evidence from China. China Agricultural Economic Review, 8(3), 480–497. https://doi.org/10.1108/CAER-09-2015-0124
- Chen, D., & Ma, Y. (2022). Effect of industrial structure on urban-rural income inequality in China. China Agricultural Economic Review, 14(3), 547–566. https://doi.org/10.1108/CAER-05-2021-0096
- Clarke-Sather, A., Qu, J., Wang, Q., Zeng, J. J., & Li, Y. (2011). Carbon inequality at the sub-national scale: A case study of provincial-level inequality in CO2 emissions in China 1997–2007. Energy Policy, 39(9), 5420–5428. https://doi.org/10.1016/j.enpol.2011.05.021
- Cnaan, R. A., Scott, M. L., Heist, H. D., & Moodithaya, M. S. (2021). Financial inclusion in the digital banking age: Lessons from rural India. Journal of Social Policy, 1–22. https://doi.org/10.1017/S0047279421000738
- Corrado, G., & Corrado, L. (2017). Inclusive finance for inclusive growth and development. Current Opinion in Environmental Sustainability, 24, 19–23. https://doi.org/10.1016/j.cosust.2017.01.013
- Cruz-Jesus, F., Oliveira, T., Bacao, F., & Irani, Z. (2017). Assessing the pattern between economic and digital development of countries. Information Systems Frontiers, 19(4), 835–854. https://doi.org/10.1007/s10796-016-9634-1
- Du, Q., Li, J., Li, Y., Huang, N., Zhou, J., & Li, Z. (2020). Carbon inequality in the transportation industry: Empirical evidence from. Environmental Science and Pollution Research International, 27(6), 6300–6311.
- Furusawa, T., Konishi, H., & Duong, T. L. A. (2020). International trade and income inequality. The Scandinavian Journal of Economics, 122(3), 993–1026. https://doi.org/10.1111/sjoe.12360
- Greaney, T. M., & Li, Y. (2017). Multinational enterprises and regional inequality in China. Journal of Asian Economics, 48, 120–133. https://doi.org/10.1016/j.asieco.2016.09.003
- Guo, F., Wang, J. Y., Wang, F., Kong, T., Zhang, X., & Cheng, Z. Y. (2019). Measuring China’s digital finance inclusion: Index compilation and spatial characteristics. China Economic Quarterly, 19(4), 1401–1418.
- Huang, J. B., Liu, C. H., Chen, S. X., Huang, X., & Hao, Y. (2019). The convergence characteristics of China’s carbon intensity: Evidence from a dynamic spatial panel approach. The Science of the Total Environment, 668, 685–695.
- Huang, Y., & Huang, Z. (2018). The development of digital finance in China: Present and future. China Economic Quarterly, 17(4), 1489–1502.
- Ji, X., Wang, K., Xu, H., & Li, M. (2021). Has digital financial inclusion narrowed the urban-rural income gap: The role of entrepreneurship in China. Sustainability, 13(15), 8292–8292. https://doi.org/10.3390/su13158292
- Kamei, K. (2014). Pro-competitive effect, division of labor, and firm productivity. Economics Letters, 124(1), 132–135. https://doi.org/10.1016/j.econlet.2014.05.001
- Khera, P., Ng, S., Ogawa, S., & Sahay, R. (2022). Measuring digital financial inclusion in emerging market and developing economies: A new index. Asian Economic Policy Review, 17(2), 213–230. https://doi.org/10.1111/aepr.12377
- Koh, S. G. M., Lee, G. H. Y., & Bomhoff, E. J. (2020). The income inequality, financial depth and economic growth nexus in China. World Economy, 43(2), 412–427. https://doi.org/10.1111/twec.12825
- Kotschy, R., & Sunde, U. (2021). Income Shocks, Inequality, and Democracy*. Scandinavian Journal of Economics, 123(1), 295–326. https://doi.org/10.1111/sjoe.12398
- Legros, P., Newman, A. F., & Proto, E. (2014). Smithian growth through creative organization. Review of Economics and Statistics, 96(5), 796–811. https://doi.org/10.1162/REST_a_00421
- Li, J., Wu, Y., & Xiao, J. J. (2020). The impact of digital finance on household consumption: Evidence from China. Economic Modelling, 86, 317–326. https://doi.org/10.1016/j.econmod.2019.09.027
- Li, M., & Feng, S. X. (2020). Digital inclusive finance and urban-rural income gap: A literature based analysis. Contemporary Economic Management, 42, 93–102.
- Li, M.-c., Feng, S.-x., & Xie, X. (2022). Spatial effect of digital financial inclusion on the urbanrural income gap in Chinaanalysis based on path dependence. Economic Research-Ekonomska Istrazivanja, 1–22. https://doi.org/10.1080/1331677X.2022.2106279
- Li, Y., Wang, M., Liao, G., & Wang, J. (2022). Spatial spillover effect and threshold effect of digital financial inclusion on farmers' income growth-based on provincial data of China. Sustainability, 14(3). https://doi.org/10.3390/su14031838
- Liu, Y., Liu, C., & Zhou, M. (2021). Does digital inclusive finance promote agricultural production for rural households in China? China Agricultural Economic Review, 13(2), 475–494. https://doi.org/10.1108/CAER-06-2020-0141
- Lokshin, B., & Mohnen, P. (2013). Do R&D tax incentives lead to higher wages for R&D workers? Evidence from The Netherlands. Research Policy, 42(3), 823–830. https://doi.org/10.1016/j.respol.2012.12.004
- Madrid-Guijarro, A., Garcia-Perez-de-Lema, D., & Van Auken, H. (2016). Financing constraints and SME innovation during economic crises. Academia-Revista Latinoamericana de Administracion, 29(1), 84–106. https://doi.org/10.1108/ARLA-04-2015-0067
- Ozili, P. K. (2018). Impact of digital finance on financial inclusion and stability. Borsa Istanbul Review, 18(4), 329–340. https://doi.org/10.1016/j.bir.2017.12.003
- Remuzgo, L., & Sarabia, J. M. (2020). Desigualdad en la distribución mundial de emisiones de CO2 por sectores: Descomposición y estudio de sensibilidad. Studies of Applied Economics, 31(1), 65–92. https://doi.org/10.25115/eea.v31i1.3261
- Ren, B. Y., & Li, L. Y. (2019). Does digital inclusion finance promote inclusive growth in rural areas? A study on the survey data from 2114 rural residents in Beijing, Tianjin and Hebei provinces. Modern Finance and Economics -Journal of Tianjin University of Finance and Economics, 4, 1–14.
- Rucker, D. D., Preacher, K. J., Tormala, Z. L., & Petty, R. E. (2011). Mediation analysis in social psychology: Current practices and new recommendations. Social and Personality Psychology Compass, 5(6), 359–371. https://doi.org/10.1111/j.1751-9004.2011.00355.x
- Satya, R., & Chakravarty, R. P. (2013). Financial inclusion in India: An axiomatic approach. Journal of Policy Modeling, 35(5), 813–837. https://doi.org/10.1016/j.jpolmod.2012.12.007
- Sarabia, J. M., Jorda, V., & Remuzgo, L. (2017). The Theil indices in parametric families of income distributions: a short review. Review of Income and Wealth, 63(4), 867–880. https://doi.org/10.1111/roiw.12260
- Schmied, J., & Marr, A. (2016). Financial Inclusion And Poverty: The Case of Peru. Regional and Sectoral Economic Studies, 16, 29–40.
- Sheng, P., Li, J., Zhai, M., & Majeed, M. U. (2021). Economic growth efficiency and carbon reduction efficiency in China: Coupling or decoupling. Energy Reports, 7, 289–299. https://doi.org/10.1016/j.egyr.2020.12.043
- Smith, A. (2008). An inquiry into the nature and the causes of the wealth of nations. University of Chicago.
- Song, X. (2017). Empirical analysis of digital inclusive finance bridging the urban–rural residents’ income gap. Finance & Economics, 6, 14–25.
- Tian, J., Tan, Q., & Jin, J. (2021). Can digital finance improve misallocation of resources? Collected Essays on Finance and Economics, 4, 49–60.
- Wang, L., Wang, Y. A., Sun, Y. X., Han, K. J., & Chen, Y. T. (2022). Financial inclusion and green economic efficiency: evidence from China. Journal of Environmental Planning and Management, 65(2), 240–271. https://doi.org/10.1080/09640568.2021.1881459
- Wuttke, J. (2017). The dark side of China’s economic rise. Global Policy, 8, 62–70. https://doi.org/10.1111/1758-5899.12439
- Yang, X. (2001). Economics: New classical versus neoclassical frameworks. Blackwell.
- Yang, X., & Ng, Y.-K. (1993). Specialization and economic organization: A new economic framework. North-Holland.
- Ye, D., Huang, A., & Zeng, F. (2021). Does structural matching between finance and the real economy promote economic growth? International Review of Economics & Finance, 73, 11–29. https://doi.org/10.1016/j.iref.2020.12.037
- Yi, X. J., & Zhou, L. (2018). Does digital inclusive finance significantly influence household consumption? Evidence from household survey data in China. Journal of Financial Research, 11, 47–67.
- Yu, G. H., & Lu, Z. (2021). Rural credit input, labor transfer and urban-rural income gap: evidence from China. China Agricultural Economic Review, 13(4), 872–893. https://doi.org/10.1108/CAER-09-2020-0229
- Yu, N., & Wang, Y. (2021). Can digital inclusive finance narrow the Chinese urban–rural income gap? The perspective of the regional urban–rural income structure. Sustainability, 13(11), 6427. https://doi.org/10.3390/su13116427
- Zhang, H. W. (2019). Effect of low carbon economy on enterprise competitiveness: A multiple mediation model. Applied Ecology and Environmental Research, 17(4), 8793–8803. https://doi.org/10.15666/aeer/1704_87938803
- Zhang, X., Wan, G. H., & Zhang, J. J. (2019). Digital economy, financial inclusion, and inclusive growth. Economic Research Journal, 54(8), 71–86.
- Zhao, X., Lynch, J. G., Jr., & Chen, Q. (2010). Reconsidering Baron and Kenny: Myths and truths about mediation analysis. Journal of Consumer Research, 37(2), 197–206. https://doi.org/10.1086/651257
- Zheng, L. (2014). China’s internet finance: Mode, influence, nature, and risk. International Economic Review, 5, 103–118.
- Zhu, L., Yang, C., Zhang, Y., & Xue, Y. (2022). Using marginal land resources to solve the shortage of rural entrepreneurial land in China. LAND, 11(7), 1035. https://doi.org/10.3390/land11071035
- Zhu, S., Yu, C., & He, C. (2020). Export structures, income inequality and urban-rural divide in China. Applied Geography, 115, 102150. https://doi.org/10.1016/j.apgeog.2020.102150.
Appendix
Table A1. Panel unit-root test.
Table A2. Panel co-integration test.
Table A3. Robustness test with additionally policy factor.
Table A4. Robustness test with different time periods.