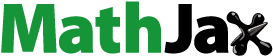
Abstract
Based on provincial-level agricultural input and output data in China from 2000 to 2019, this study estimates the Luenberger-Hicks-Moorsteen index using the nonparametric method of the directional distance function to obtain agricultural green total factor productivity (AGTFP). The results show that AGTFP in China has increased significantly over the past 20 years, with significant differences between provinces and among the eastern, central, and western regions. Land market distortion has had a significant negative impact on AGTFP. The results suggest that the government needs to promote the market-oriented reform of land factors, solve the price distortion caused by land finance, promote the intensification and modernization of agricultural management, and realize the potential of green agricultural production.
1. Introduction
Food security remains a long-standing and challenging issue for many developing countries. Among them, China has more difficult problems of feeding 22% of the global population while having only 7% of its arable land. Threatened by the COVID-19 pandemic, global uncertainty, and climate change, China’s food security has become a more pressing issue. China’s population is estimated to reach 1.5 billion by 2030, necessitating the production of an additional 100 million tons of food annually.Footnote1 In the Global Food Security Index for 2021, out of 113 countries, China is 34th (Kate, Citation2022). In the face of enormous population pressure and external environmental uncertainty, China must make every effort to increase agricultural productivity (Wang et al., Citation2020). Therefore, research on agricultural production in China is theoretically and practically significant.
Green total factor productivity (GTFP) is an essential indicator of China’s agricultural economic growth and supply-side structural transformation. The concept not only relates to the pace of technological advancement, sometimes referred to as total factor productivity (TFP), but is also associated with environmental protection (Su et al., Citation2022a; Shen et al., Citation2022). In agricultural production, the output includes both desired and undesired polluting output. China has serious environmental pollution problems in agricultural production (Shen et al., Citation2022). For instance, China is the world’s largest user of chemical fertilizers and pesticides. Wang et al. (Citation2010) posit that over 15% of the total greenhouse gas emissions, around 90% of nitrous oxide, and 60% methane emissions are from agriculture in China. Since the Chinese government announced the goals of carbon peaking and carbon neutrality, GTFP has become a more scientific indicator of capturing the extent of green development in agriculture (Deng et al., Citation2022). Despite the annual increase in carbon dioxide emissions from agriculture, the trend toward reaching the peak has declined in recent years (Jin et al., Citation2021; Su et al., Citation2022b). As noted by the 14th Five-Year Plan, the country is considering setting a new cut-off point for agricultural GTFP (AGTFP) improvement and further improving agricultural development while increasing the carbon constraint target.
Moreover, distortion issues exist in the agricultural land market. Land is an essential and unique production input factor in agricultural production. Problems of poor quality of inputs and inefficient allocation of land factors persist in China (Pingali, Citation2012). More prominently, in terms of transfer and scale effect, land allocation issues in agricultural production have hampered agricultural growth (Ye, Citation2015). Under the current land allocation system, the land scale does not correlate with its production efficiency, which means that the allocation of land resources in China might be distorted (Gai et al., Citation2017). The allocation of land resources affects not only technological progress but also the capitalization and mechanization of agricultural production. Under such circumstances, it is challenging to intensify and increase the scale of farming operations. The agrarian production efficiency per unit of land area is also reduced, and pesticides, chemical fertilizers, and plastic films are used excessively. This then has a significant impact on the polluting output of agricultural production. Thus, reversing regional land resource misallocation and improving land resource utilization efficiency through land market reform is one of the ways to enhance GTFP in agriculture.
Previous literature focuses on the relationship between the distortion of the factor market and AGTFP rather than on the land itself (Yang et al., Citation2022). Several studies concentrate on the relationship between land market distortions and TFP but do not focus on agriculture or consider the pollution caused by agricultural production (Lu et al., Citation2020; Yang et al., Citation2022). Thus, to address this, this study emphasizes the role of land market distortions on AGTFP. We utilize input-output data on Chinese agriculture from 2000 to 2019 to estimate the GTFP using the Luenberger-Hicks-Moorsteen total factor productivity (LHMTFP) index and investigate the impact of distorted land on GTFP in China.
This study makes the following contributions. First, the LHMTFP indicator is introduced to capture the changes in AGTFP in China over the past two decades. The By-Production technique complies with the requirements for material balance, while the LHM indicator considers variations in input and output over time and non-desired outcomes. The methodology of this study provides new insights. Second, we analyze the spatial differences and time variation of AGTFP at a macro level and capture the impact of land market distortions on AGTFP. This allows policymakers to recognize the importance of land market distortions and improves agricultural green products from the perspective of land factor marketization.
2. Literature review
2.1. AGTFP
One approach to measuring GTFP in agriculture is to consider pollution-causing inputs. For instance, Wang et al. (Citation2012) utilized nitrogen and phosphorus loss in agricultural output as a factor input to calculate the GTFP change index in Chinese agriculture from 1992 to 2010 through a stochastic frontier production function approach. Another method considers undesirable outcomes, such as pollutant emissions and agricultural surface contamination. Xu et al. (Citation2019) quantified agricultural carbon emissions in five ways – namely, agrarian materials, rice cultivation, soil, livestock and poultry rearing, and straw burning – to capture China’s AGTFP from 1998 to 2016 by directional distance function (DDF). Xu et al. (Citation2020) included soil nitrogen dioxide emissions to estimate GTFP in agriculture. They found that the productivity with soil nitrogen dioxide emissions is much lower than that without these emissions. Chen et al. (Citation2022) utilized a three-stage data envelopment analysis technique paired with a slack-based metric model to estimate the AGTFP by taking carbon emissions and agricultural surface source pollution as undesirable outputs.
Existing literature indicates that AGTFP in China presents an upward trend with significant regional differences. Wu and Song (Citation2018), for instance, showed that the AGTFP in the Yangtze River Economic Zone has increased from 1997 to 2015 but remains lower than the average at the national level. Liu and Feng (Citation2019) found that China’s AGTFP follows a U-shaped trend, with the turning point occurring in 2010, and that the eastern region had the greatest AGTFP, while the western area had the highest AGTFP growth rate. Ji and Xia (Citation2020) revealed a fluctuating increase in China’s AGTFP from 2011 to 2016 with a significant absolute β-convergence trend. Liu et al. (Citation2021) reported that China’s AGTFP showed an increasing trend with inter-provincial differences.
The determinants of AGTFP include technological progress (Liu & Feng, Citation2019), foreign direct investment (Wang et al., Citation2019), crop insurance (Fang et al., Citation2021), and agricultural factor endowment and regional characteristics (Liu et al., Citation2021). Liu and Lv (Citation2021) indicated that rural human capital and GTFP in China have a non-linear connection. Wu and Yao (Citation2021) used a spatial Durbin model to investigate the spatial impact of AGTFP under factor market distortions and showed that factor market distortions inhibit GTFP growth in agriculture. Yin et al. (Citation2022) found that agro-industrial agglomeration has a significant negative effect on AGTFP.
2.2. Land market distortion
A body of studies has addressed the vital role of land distortions in firm investment, industry productivity, and the urban-rural income gap. For instance, Huang et al. (Citation2015) found that industrial land price distortions significantly promote firm overinvestment with different behavior across ownership attributes and industry attributes. Wang et al. (Citation2021) concluded that government intervention in industrial land concessions causes price distortions of land, further leading to lower productivity of industrial firms. Zhang and Ge (Citation2021) found that land market distortions significantly increase the wealth disparity between cities and rural areas.
A large amount of literature also indicates the significant effect of land misallocation on agricultural production. For example, Chamberlin and Ricker-Gilbert (Citation2016) found that the land lease market has promoted the transfer of land from small-scale farmers with weak abilities to small-scale farmers with strong abilities, which improves agricultural productivity in Malawi and Zambia. Chen (Citation2017) showed that agricultural productivity is lower in economies with a higher proportion of untitled land. Chen et al. (Citation2018) used the price-to-value ratio as an indicator of land distortion. They found that housing activities stimulate farmland price value distortion but do not increase farmland value. Le (Citation2020) concluded that removing all land-use restrictions results in a rise of 8.03% in the GDP per capita. Chen et al. (Citation2022) found that land redistribution increases agricultural productivity at the regional level by an average of 43%. Britos et al. (Citation2022) investigated the influence of land market flaws on agricultural output. They noted that the total output of maize, bean, and coffee is lower than the effective level due to land market imperfections. Our study explored the effect of land distortions on AGTFP from 2000 to 2019 in China.
3. Model
3.1. Environmental production technology
We introduced a set of environmental production options using economic axioms according to sub-production technology (Murty & Russell, 2002; Murty et al., Citation2012). First, we start with a theoretical definition of the By-Production technique. and
represent the number of pollution-free inputs and pollution-producing inputs, respectively. The output vectors y and b indicate desired and unwanted outcomes in the production process, respectively. We can then establish the anticipated production sub-technology
using production economics concepts. The technology describes converting all inputs into desired outputs, which relates to typical production technologies. Here, we ignore the process of production externalities. The definition also has an unintended contamination production sub-technology
In this case, only the polluting inputs and undesirable outcomes are considered. This technique considers the externalities associated with the production process, thus distinguishing it from the study of traditional efficiency. The intersection of sub-technologies
and
is termed as BP technology, which considers both productivity and environmental efficiency (Shen et al., Citation2022). In general, after considering the inputs and outputs, the BP technique (
) can be expressed as follows:
3.2. Directional distance function
A distance function can be used to characterize the BP approach discussed in the previous section. The performance gaps of the examined decision units can be identified using the DDF technique. As environmental production techniques include both desired and undesired outputs, the DDF may account for the distinct optimization orientations of the two types of outputs. Desired outputs boost social welfare and should be improved, whereas non-desired outputs cause negative externalities and should be decreased. Input resources are similarly limited and should be minimized to the greatest extent feasible. This environmental production technique can be expressed in the DDF (Chung et al., Citation1997; Färe et al., Citation2005). The generalized DDF describes the rise in desirable output and the decrease of the unwanted output and input at the technology level in periods and
It can be defined as follows:
is the directional vector of inputs, desired outputs, and undesired outputs.
denotes a mixed periodic DDF.
3.3. LHM indicator and decomposition in the environment
Briec and Kerstens (Citation2004) developed the LHM productivity meter, which may be regarded as an extra full TFP metric (O’Donnell, Citation2012). The major purpose of our study is to broaden the scope of the LHM indicator by considering non-desired outcomes. We may then use this information to examine the environmentally adjusted TFP indicator (Shen et al., Citation2019).
Non-desired outputs can be incorporated into TFP measurements in various ways (Dakpo et al., Citation2016; Ancev et al., Citation2017; Dakpo & Ang, Citation2019). Unwanted outputs can be treated as inputs and lowered at the same time. They can also be used as weakly disposable outputs in the model. The former approach is problematic, so we adopted the latter and simultaneously increased desired outputs and decreased non-desired outputs in the optimization process. The environmental LHM indicator measures the change in GTFP by considering the distance between the boundary and observation points within the observation period and
This is done along the direction of (desired and undesired) outputs, keeping the input level constant and the output level stable along the path of inputs (Baležentis et al., Citation2021). To avoid arbitrariness in the choice of the baseline cycle, we implemented the two cycles sequentially as the baseline cycle. The environmental LHM measure for the base period is defined as
(1)
(1)
The first two terms in parentheses denote the gap between the intended and undesirable outputs along the direction of cycle ‘s border. The final two terms show the distance between the inputs and the boundary. We see a GTFP gain when the value of this indicator is greater (lesser) than 0. Similarly, the LHM measure for cycle
is defined as:
(2)
(2)
To produce an indicator of the variation in LHM production between periods and
we use the average score of indicators in (1) and (2) (Baležentis et al., Citation2022):
(3)
(3)
3.4. Strategy for estimation
Parametric and nonparametric approaches can be used to estimate the DDF. We opted for the nonparametric method, which allows the estimation of production constraints without defining any particular operational form and places a priori assumptions on the technique, such as monotonicity and convexity. Murty et al. (Citation2012) proposed an improved sub-production model that keeps the production possibility set convex and returns to the scale variable. We assumed reduced convexity and employed a free disposal hull model.
A series of linear programs must be solved in computing the LHM indicators and their components in expressions (1) and (2). Only two uncommon situations are presented here. This study compared the input and output vectors of cycle with the cycle
The relevant output or input DDF is defined in our technique. We assumed decision-making units
where
Next, we constructed an empirical bound using these units’ input and output vectors. Specifically, we derived the output DDF
by solving the linear algorithm shown below (LP1).
(LP1)
(LP1)
and
are vectors of intensity variables.
shows the maximum increase in desired output and maximum decrease in undesired output. The input DDF
could be acquired by solving the linear algorithm below (LP2).
(LP2)
(LP2)
and
are vectors of intensity variables.
represents the input value in DDF.
denotes the maximum reduction in the direction of the input defined in period
3.5. Data
To estimate the AGTFP, we employed input and output data from 30 provinces in mainland China (excluding Tibet). The inputs were labor, land, and capital, among which capital inputs included mechanical power, fertilizer use, pesticide use, and agricultural film use. We measured desired output using gross agricultural output. To reduce the impact of pricing factors, we used prices in the year 2000 as the benchmark and corrected the data of gross agrarian output for all years. Agrarian carbon emissions, including the carbon emissions of fertilizer, pesticide, agricultural film, diesel, and irrigation combined, measured undesired output. Data were obtained from the China Stock Market & Accounting Research Database, the National Bureau of Statistics, and the China Rural Statistical Yearbook (2000–2019). and give specific indicator descriptions and descriptive statistics.
Table 1. Description of variables.
Table 2. Statistical descriptions.
3.6. Specification of the econometric model
Land distortion refers to factor market prices differing from their potential costs owing to imperfect markets or government regulation, resulting in the non-optimal distribution in production. China’s land market reform has been slow and characterized by the eastern region reforming at a significantly faster pace than the western region in recent years. As essential inputs for agricultural production, land scale, and quality profoundly impact agricultural productivity. Resource allocation and market-based transactions in the land market significantly impact farming efficiency and agricultural green development. Therefore, one of the primary purposes of this study is to capture the effect of land distortion on agricultural productivity in China. This study uses a multiple linear regression model, and the econometric model is constructed as follows:
where
is agricultural productivity in province
at year
The main explanatory variable is land factor market distortion, denoted by
Local governments expropriate land from farmers and strategically transfer it to supply land resources between industrial land and commercial and residential land. In this process, the government has a two-way monopoly on the non-agricultural land market and the primary market for urban land. This ultimately manifests in large land transfer fees being dominated by local governments (Sun et al., Citation2014; Wu et al., Citation2015). Therefore, we used the share of land concessions in regional fiscal revenue as a proxy variable to measure land market distortions (Xie, Citation2017). The data are obtained from the CSMAR database and the China Land and Resources Statistical Yearbook (2000–2019).
This study selected control variables based on previous research and data availability (Liang & Long, Citation2015; Wu & Yao, Citation2021). We used the following variables as control variables: agricultural development level, agricultural structure, rural human capital, degree of agricultural capitalization, level of industrialization, level of urbanization, agriculture-related financial expenditure, agricultural tax burden, and agricultural terms of trade. lists the definitions of the specific variables. Among all variables, agricultural tax refers to several agriculture-related taxes in general. Specifically, it is the sum of slaughter, farming, special agricultural production, deed, pastoral, and cropland occupation taxes before 2007 and the sum of the deed, tobacco leaf, and cropland occupation taxes since 2007. Due to data limitations, we obtained the data from 2003 to 2019 for 30 provinces in mainland China excluding Tibet. The data are obtained from the dataset of the National Bureau of Statistics and CSMAR. The descriptive results of all variables are shown in .
Table 3. Definition of variables.
Table 4. Descriptive statistics.
4. Results
4.1. Agricultural green productivity changes in China during 2000–2019
shows the national cumulative AGTFP in China. In general, the level of AGTFP has risen steadily. Against the background of resources and the environment increasingly becoming a hard constraint for economic growth, technological progress in agriculture is an essential factor for agricultural development. Amid increasing ecological and environmental pressure, the key to achieving agricultural carbon emission reduction lies in technical efficiency.
presents the average AGTFP of each province from 2000 to 2019. The AGTFP varies widely between provinces. From the numerical point of view, the average AGTFP of the 30 provinces in the past 20 years reaches a maximum of 0.096 and a minimum of −0.131. From the perspective of the provinces, economic belts such as Beijing-Tianjin-Hebei and Jiangsu-Zhejiang-Shanghai have higher AGTFP values. In contrast, Hainan, Gansu, Qingdao, and other underdeveloped inland provinces have lower AGTFP values. The results show that Hainan, Gansu, and Qinghai have a negative AGTFP. In the case of Hainan, this might be due to limited land resources, tight arable land resources, small-farmer and low-efficiency agricultural operations, and extensive environmental losses in agricultural development (Song et al., Citation2020). Gansu, located in northwestern China, has insufficient water resources, a weak ecological environment, and low environmental capacity, which limit the development of agriculture and technical efficiency improvement. Qinghai, in the northeastern region of the Qinghai-Tibet Plateau, has a high altitude, cold climate, and fragile ecological environment. Only a small number of cold-tolerant crops are suitable for growth there. Furthermore, agricultural research and technology are underdeveloped in this area. All of these factors caused slow development and expansion of the agricultural economy.
AGTFP is higher in Beijing, Tianjin, and Hebei, with Beijing having the highest AGTFP. Agricultural green technological progress is the driving force of AGTFP growth in Beijing, Tianjin, and Hebei. The latest data from the National Bureau of Statistics show that agriculture in Beijing contributes to innovative and green development, owing to the enormous contribution (72%) of agriculture science and technological progress that exceeds the national average by 14 percentage points (Li & Lu, Citation2019). Moreover, fertilizer and pesticide applications decreased by 46% and 30%, respectively, from 2013 to 2018 (National Bureau of Statistics of China Citation2019). Another finding is the significant variation of AGTFP between regions.
shows the average AGTFP in different regions from 2000 to 2019. The results show that the eastern region has the highest AGTFP and the fastest growth. The central region has the second highest AGTFP, followed by the northeast region. The western region has the lowest AGTFP. We also observe a significant spatial divergence in productivity growth, with the overall distribution trend rising from west to east. Meanwhile, it rises and then falls from south to north, corresponding with previous results (Yang et al., Citation2019).
4.2. Impact of land distortion on agricultural green productivity
The impact of land distortion on green productivity in China’s agriculture is shown in . The Hausman test disqualifies the models with random effects. The results of White’s test indicate a heteroskedasticity problem, so we also regress the equation with clustering standard errors. With and without adding other control variables, the results show that land market distortion negatively and significantly impacts agricultural green productivity. Moreover, the impact is still significant with clustering standard errors. To enhance the credibility of the regression model, we run the regressions with a quality bilateral tail shrinkage of 1% and quality bilateral truncation of 1%. The regression results still show a significant negative impact of land market distortion on green productivity in agriculture.
Table 5. Results of impact of land distortion on agricultural green productivity.
The distortion of the land market marks the deviation of land price from opportunity cost, leading to the misallocation or suboptimal allocation of land, which then harms the improvement of AGTFP. The results of this study indicate that if land can be effectively allocated and if the distortion of the land market can be improved by 1%, China’s AGTFP will increase by 37.5%. This finding is generally consistent with previous research, but there are subtle differences. This study measures the impact of land distortion alone, whereas previous literature measured the combined effects of three types of distortions: land, capital, and labor. Using provincial data from 1997 to 2018, Wu and Yao (Citation2021) concluded that a 1% reduction in factor market distortion, which is the average of capital market distortion, labor market distortion, and land market distortion, would lead to an increase in about 19.7% in AGTFP. Similarly, Yang et al. (Citation2022) calculated a 15.7% improvement using provincial data from 1997 to 2020. Changes in the land factor market alone can lead to more significant green productivity gains. This also illustrates the importance of considering land market distortions. Britos et al. (Citation2022) found that due to land market distortions, the total production of corn and beans was 19% lower than their efficient levels, and coffee was 31% lower.
The importance of land distortion in influencing green productivity is that distortion of land resource prices is related to economic and social development, such as land finance, local government investment attraction, and urban construction. This study takes the share of land concessions in regional fiscal revenue as a proxy variable for land market distortion. When the number of agricultural lands is reduced due to land finance and land transfer, village communal land is more likely to be confiscated in large quantities for economic development. This might result in extensive land use and a waste of land resources. It is not easy to form large-scale and intensive management. Using pesticides, fertilizers, and agricultural films leads to a wide range of pollution, high carbon emission levels, and difficulty in improving agricultural green productivity. The main reasons for this are the existence of land financial problems and the virtual land ownership caused by the unclear subject of land property rights in rural collective-owned land and the existing fiscal and tax system ().
Table 6. Results of impact of land distortion on agricultural green productivity.
For control variables, the results indicate that agricultural development level, agricultural structure, rural human capital, degree of agricultural capitalization, and industrialization also significantly affect AGTFP. The level of local agricultural growth reflected in the agricultural base has a positive impact. Provinces with higher levels of agricultural development are more likely to achieve increased green agriculture output. Similarly, rural human capital has a positive influence on GTFP. This is because an increase in the average years of schooling enhances rural human capital, which leads to the modernization of agricultural production and operation and improves agricultural production efficiency.
Moreover, the agricultural structure negatively impacts AGTFP. The higher the ratio of food to cash crops, the lower the efficiency of land cultivation tends to be. With the same degree of carbon emission, the higher the share of grain sowing, the lower the GTFP. Likewise, the degree of agricultural capitalization negatively affects AGTFP. The higher the proportion of total agricultural machinery power and the greater the pollution-causing inputs, the lower the level of AGTFP. The level of regional industrialization also has a negative effect on AGTFP. During the growth of secondary industry-oriented economies, the government tends to supply industrial production factors at low prices, hindering the development of AGTFP. The negative impact of agricultural taxation on GTFP in agriculture is not significant. An agricultural tax burden reduces farmers’ burdens and promotes agricultural technological progress and production efficiency to a certain extent. However, since 2006, agricultural taxes have been discontinued, and other related taxes have been drastically reduced, making the effect of the agricultural tax burden on green agricultural productivity insignificant.
5. Conclusion
This study combines the environmental DDF and nonparametric method to estimate the AGTFP at the provincial level from 2000 to 2019. It then further examines the impact of land market distortion on AGTFP.
The findings indicate that China’s AGTFP steadily increased during 2000–2019. Although there are fluctuations, the overall increase is considerable. Taking 2000 as the benchmark, it rose to 0.49 in 2019. The absolute and change values of AGTFP vary greatly among Chinese provinces and regions. The eastern regions have the highest AGTFP and the fastest growth. The central region has the second highest AGTFP, followed by the northeast area. The western region has the lowest AGTFP. Productivity growth differs significantly among regions.
We also confirm the negative influence of land price distortion on GTFP in agriculture. The distortion of the land market leads to the failure of optimal allocation of land resources, which hinders the improvement of AGTFP. Moreover, we also identify the significant positive impact of the agricultural base and rural human capital on the AGTFP level. Agricultural structure, degree of agricultural capitalization, and industrialization have a markedly detrimental effect on the AGTFP level.
Therefore, we formulate the ensuing policy suggestions. First, the government should build a perfect institutional system for market-oriented land factor allocation and promote market-oriented land factor reform. The specific power of land acquisition and management should be decentralized. The management of the balance between occupancy and compensation in urban and rural regions, as well as the growth and decline of productive land use, should be put into place by the authorities. Second, the government should clarify the main body and the fundamental principles of land ownership. The ambiguous right of land ownership is the root cause of the direct distortion of land resource prices and various problems related to land transactions. Legal solutions must be applied. Third, the government should adjust the central-to-local revenue distribution ratio to solve the price distortion caused by land finance. Adjusting the fiscal and taxation system can solve the mismatch between financial and service powers faced by Chinese local governments, help avoid over-reliance on land finance, and change the asymmetry between land supply and demand such that prices conform to the equilibrium level of the market.
This article is not without limitations. There is no subdivision of various industries within agriculture, and high-pollution agriculture and low-pollution agriculture are indistinguishable.
Disclosure statement
No potential conflict of interest was reported by the authors.
Notes
1 Dipanjan Roy Chaudhury. 2020. ‘With rising population and declining arable land, China may be staring at a major food crisis', The Economic Times. <https://economictimes.indiatimes.com/news/international/world-news/with-rising-population-and-declining-arable-land-china-may-be-staring-at-a-major-food-crisis/articleshow/77942570.cms?from=mdr>
References
- Ancev, T., Azad, M. S., & Akter, M. (2017). Environmentally adjusted productivity and efficiency: A review of concepts, methods and empirical work. In New directions in productivity measurement and efficiency analysis (pp. 9–58). Edward Elgar Publishing.
- Baležentis, T., Blancard, S., Shen, Z., & Štreimikienė, D. (2021). Analysis of environmental total factor productivity evolution in European agricultural sector. Decision Sciences, 52(2), 483–511. https://doi.org/10.1111/deci.12421
- Baležentis, T., Kerstens, K., & Shen, Z. (2022). Economic and environmental decomposition of Luenberger-Hicks-Moorsteen total factor productivity indicator: Empirical analysis of Chinese textile firms with a focus on reporting infeasibilities and questioning convexity. IEEE Transactions on Engineering Management, 1–14. https://doi.org/10.1109/TEM.2022.3195568
- Briec, W., & Kerstens, K. (2004). A Luenberger-Hicks-Moorsteen productivity indicator: Its relation to the Hicks-Moorsteen productivity index and the Luenberger productivity indicator. Economic Theory, 23(4), 925–939. https://doi.org/10.1007/s00199-003-0403-2
- Britos, B., Hernandez, M. A., Robles, M., & Trupkin, D. R. (2022). Land market distortions and aggregate agricultural productivity: Evidence from Guatemala. Journal of Development Economics, 155, 102787. https://doi.org/10.1016/j.jdeveco.2021.102787
- Chamberlin, J., & Ricker-Gilbert, J. (2016). Participation in rural land rental markets in sub-Saharan Africa: Who benefits and by how much? Evidence from Malawi and Zambia. American Journal of Agricultural Economics, 98(5), 1507–1528. https://doi.org/10.1093/ajae/aaw021
- Chen, C. R. (2017). Untitled land, occupational choice, and agricultural productivity. American Economic Journal: Macroeconomics, 9(4), 91–121. https://doi.org/10.1257/mac.20140171
- Chen, Y.-H., Lee, C.-L., Chen, G.-R., Wang, C.-H., & Chen, Y.-H. (2018). Factors causing farmland price-value distortion and their implications for peri-urban growth management. Sustainability, 10(8), 2701. https://doi.org/10.3390/su10082701
- Chen, C., Restuccia, D., & Santaeulàlia-Llopis, R. (2022). The effects of land markets on resource allocation and agricultural productivity. Review of Economic Dynamics, 45, 41–54. https://doi.org/10.1016/j.red.2021.04.006
- Chung, Y. H., Färe, R., & Grosskopf, S. (1997). Productivity and undesirable outputs: A directional distance function approach. Journal of Environmental Management, 51(3), 229–240. https://doi.org/10.1006/jema.1997.0146
- Dakpo, K. H., & Ang, F. (2019). Modelling environmental adjustments of production technologies: A literature review. In T. Ten Raa and W. H. Greene (Eds.), The Palgrave Handbook of Economic Performance Analysis (pp. 601–657). Springer International Publishing.
- Dakpo, K. H., Jeanneaux, P., & Latruffe, L. (2016). Modelling pollution-generating technologies in performance benchmarking: Recent developments, limits and future prospects in the nonparametric framework. European Journal of Operational Research, 250(2), 347–359. https://doi.org/10.1016/j.ejor.2015.07.024
- Deng, H., Li, H., Pan, A., & Shen, Z. (2022). Decomposition of green agricultural productivity gain under a multiple-frontier framework. Journal of Global Information Management, 30(6), 1–22.
- Fang, L., Hu, R., Mao, H., & Chen, S. J. (2021). How crop insurance influences agricultural green total factor productivity: Evidence from Chinese farmers. Journal of Cleaner Production, 321, 128977. https://doi.org/10.1016/j.jclepro.2021.128977
- Färe, R., Grosskopf, S., Noh, D.-W., & Weber, W. (2005). Characteristics of a polluting technology: Theory and practice. Journal of Econometrics, 126(2), 469–492. https://doi.org/10.1016/j.jeconom.2004.05.010
- Gai, Q. E., Zhu, X., Cheng, M. W., & Shi, Q. H. (2017). Land misallocation and aggregate labor productivity. Economic Research Journal, 52(5), 117–130.
- Huang, J. B., Xu, Z., & Xu, S. (2015). Land price distortion, enterprises’ property and over-investment—An empirical research based on the data of Chinese industrial enterprises and land price of cities in China. China Industrial Economics, 3, 57–69.
- Ji, C. J., & Xia, H. M. (2020). Study on the impact of agricultural science and technology service on agricultural green total factor productivity in China. Chinese Journal of Agricultural Resources and Regional Planning, 41(12), 136–143.
- Jin, S. Q., Lin, Y., & Niu, K. Y. (2021). Driving green transformation of agriculture with low carbon: Characteristics of agricultural carbon emissions and its emission reduction path in China. Reform, 5, 29–37.
- Kate, W. (2022). Food Security: This is How China Plans to Feed Its 1.4 Billion People. World Economic Forum.
- Le, K. (2020). Land use restrictions, misallocation in agriculture, and aggregate productivity in Vietnam. Journal of Development Economics, 145, 102465. https://doi.org/10.1016/j.jdeveco.2020.102465
- Li, Q. G., & Lu, X. C. (2019). Beijing accelerates the innovation and development of agricultural science and technology parks. Beijing, China: Farmer's Daily. https://caijing.chinadaily.com.cn/a/201905/17/WS5cddefaba310e7f8b157d250.html.
- Liang, J., & Long, S. B. (2015). China’s agricultural green total factor productivity growth and its affecting factors. Journal of South China Agricultural University (Social Science Edition), 14(3), 1–12.
- Liu, Y., & Feng, C. (2019). What drives the fluctuations of "green" productivity in China’s agricultural sector? A weighted Russell directional distance approach. Resources Conservation and Recycling, 147, 201–213. https://doi.org/10.1016/j.resconrec.2019.04.013
- Liu, F., & Lv, N. (2021). The threshold effect test of human capital on the growth of agricultural green total factor productivity: Evidence from China. The International Journal of Electrical Engineering & Education, 002072092110032. https://doi.org/10.1177/00207209211003206
- Liu, D. D., Zhu, X. Y., & Wang, Y. F. (2021). China’s agricultural green total factor productivity based on carbon emission: An analysis of evolution trend and influencing factors. Journal of Cleaner Production, 278, 123692. https://doi.org/10.1016/j.jclepro.2020.123692
- Lu, X-h., Jiang, X., & Gong, M-q (2020). How land transfer marketization influence on green total factor productivity from the approach of industrial structure? Evidence from China. Land Use Policy, 95, 104610. https://doi.org/10.1016/j.landusepol.2020.104610
- Murty, S., Russell, R. R., & Levkoff, S. B. (2012). On modeling pollution-generating technologies. Journal of Environmental Economics and Management, 64(1), 117–135. https://doi.org/10.1016/j.jeem.2012.02.005
- National Bureau of Statistics of China. (2019). China Rural Statistical Yearbook. China Statistics Press.
- O’Donnell, C. J. (2012). An aggregate quantity framework for measuring and decomposing productivity change. Journal of Productivity Analysis, 38(3), 255–272. https://doi.org/10.1007/s11123-012-0275-1
- Pingali, P. L. (2012). Green revolution: Impacts, limits, and the path ahead. Proceedings of the National Academy of Sciences of the United States of America, 109(31), 12302–12308. https://doi.org/10.1073/pnas.0912953109
- Shen, Z., Baležentis, T., & Ferrier, G. D. (2019). Agricultural productivity evolution in China: A generalized decomposition of the Luenberger-Hicks-Moorsteen productivity indicator. China Economic Review, 57, 101315. https://doi.org/10.1016/j.chieco.2019.101315
- Shen, Z., Baležentis, T., & Vardanyan, M. (2022). Evaluating green productivity gains with the exponential by-production technology: An analysis of the Chinese industrial sector. Environmental Modeling and Assessment, 27(5), 759–770. https://doi.org/10.1007/s10666-022-09849-y
- Shen, Z., Wu, H., Bai, K., & Hao, Y. (2022). Integrating economic, environmental and societal performance within the productivity measurement. Technological Forecasting and Social Change, 176, 121463. https://doi.org/10.1016/j.techfore.2021.121463
- Song, C., Zhang, J., Liu, L., Ma, W., Ding, S., & Zhao, H. (2020). Spatial and temporal characteristics of agricultural green development indicators in Hainan Island. Chinese Journal of Eco-Agriculture, 28(8), 1156–1167.
- Sun, X. L., Zhou, F. Z., & Lin, W. P. (2014). Land finance and the tax-sharing system: An empirical interpretation. Social Sciences in China, 35(3), 47–64.
- Su, C., Pang, L., Tao, R., Shao, X., & Umar, M. (2022a). Renewable energy and technological innovation: Which one is the winner in promoting net-zero emissions? Technological Forecasting and Social Change, 182, 121798. https://doi.org/10.1016/j.techfore.2022.121798
- Su, C., Yuan, X., Umar, M., & Chang, T. (2022b). Dynamic price linkage of energies in transformation: Evidence from quantile connectedness. Resources Policy, 78, 102886. https://doi.org/10.1016/j.resourpol.2022.102886
- Wang, J., Huang, J., & Rozelle, S. (2010). Climate change and China’s agricultural sector: An overview of impacts, adaptation and mitigation.
- Wang, L., Su, C.-W., Ali, S., & Chang, H.-L. (2020). How China is fostering sustainable growth: The interplay of green investment and production-based emission. Environmental Science and Pollution Research International, 27(31), 39607–39618. https://doi.org/10.1007/s11356-020-09933-4
- Wang, Q., Wang, H., & Chen, H. A. I. D. A. N. (2012). A study on agricultural green TFP in China: 1992–2010. Economic Review, 5, 24–33.
- Wang, Y. F., Xie, L., Zhang, Y., Wang, C. Y., & Yu, K. (2019). Does FDI promote or inhibit the high-quality development of agriculture in China? An agricultural GTFP perspective. Sustainability, 11(17), 4620. https://doi.org/10.3390/su11174620
- Wang, B., Zhang, Y. Y., & Feng, S. Y. (2021). Local government intervention, land price distortion and industrial enterprise productivity. Economic Theory and Business Management, 41(7), 51–63.
- Wu, Q., Li, Y. L., & Yan, S. Q. (2015). The incentives of China’s urban land finance. Land Use Policy, 42, 432–442.
- Wu, C. Q., & Song, Z. Y. (2018). Study on the measurement and affecting factors of agricultural green total factor productivity in the Yangtze river economic belt. Science & Technology Progress and Policy, 35(17), 35–41.
- Wu, G. S., & Yao, S. (2021). Measurement and effect of agricultural green TFP under factor market distortion. Ecological Economy, 37(1), 96–102.
- Xie, D. S. (2017). Land market distortion and insufficient household consumption in China. Journal of Shanghai University of Finance and Economics, 19(3), 81–94.
- Xu, X. C., Huang, X. Q., Huang, J., Gao, X., & Chen, L. H. (2019). Spatial-temporal characteristics of agriculture green total factor productivity in China, 1998-2016: Based on more sophisticated calculations of carbon emissions. International Journal of Environmental Research and Public Health, 16(20), 3932. https://doi.org/10.3390/ijerph16203932
- Xu, X. C., Zhang, L., Chen, L. H., & Liu, C. J. (2020). The role of soil N2O emissions in agricultural green total factor productivity: An empirical study from China around 2006 when agricultural tax was abolished. Agriculture, 10(5), 150. https://doi.org/10.3390/agriculture10050150
- Yang, Y., Ma, H., & Wu, G. (2022). Agricultural green total factor productivity under the distortion of the factor market in China. Sustainability, 14(15), 9309. https://doi.org/10.3390/su14159309
- Yang, Q. J., Wang, C. L., & Liu, X. P. (2019). Spatial differentiation of agricultural green total factor productivity and its driving factor in China. The Journal of Quantitative & Technical Economics, 36(10), 21–37.
- Ye, J. Z. (2015). Land transfer and the pursuit of agricultural modernization in China. Journal of Agrarian Change, 15(3), 314–337. https://doi.org/10.1111/joac.12117
- Yin, X. Y., Jia, X. J., & Li, D. M. (2022). The Empact of agricultural industrial agglomeration on green total factor productivity: Based on the perspective of spatial spillover effect. Chinese Journal of Agricultural Resources and Regional Planning, 1–16. https://kns.cnki.net/kcms/detail/detail.aspx?FileName=ZGNZ20220128008&DbName=CAPJ2022
- Zhang, J. P., & Ge, Y. (2021). The influence of land market allocation on the rural-urban income distribution in China. A perspective of imbalance development of urbanization. Journal of Huazhong Agricultural University (Social Sciences Edition, 3, 172–181.