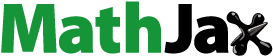
Abstract
The growing environmental degradation and climate change issue require serious attention since they harm human health and adversely affect sustainable development. The current study examines the association of government institutional trust with the environmental quality of the BRICS economies during 1990–2020. Also, this study considers economic growth and technological innovation as control variables. The empirical findings validate the sloe heterogeneity and panel cross-section dependence. Therefore, this study uses the second-generation estimators, including the Mean Group and Augmented Mean Group approaches. The empirical results asserted that governmental, institutional trust, and technological innovation are the drivers of a sustainable environment as they significantly reduce the region’s carbon emissions level. On the contrary, economic growth is a substantial factor in increased environmental degradation in the region. The estimated results are found robust by the fully modified ordinary least square and dynamic ordinary least square approaches. Also, a bidirectional causal association exists between government institutional trust – emissions, technological innovation – emissions, and economic growth – emissions. Based on the results, this study suggested strengthening institutional quality, increasing investment in research and development, education, and adopting environmentally friendly energy resources.
1. Introduction
In the absence of legislative, incentive-based regulations which impose restrictions on polluting or contaminating activities and promote sustainable behavior, it is very unlikely that issues exacerbated by rising climate change and carbon emissions will ever be resolved. Nevertheless, application in the real world frequently demonstrates environmental legislation’s success, including carbon taxes or renewables subsidies (Hughes & Podolefsky, Citation2015; Murray & Rivers, Citation2015). Yet, environmental policies aiming at climate change mitigation entirely depend on popular support, and climate measures such as carbon taxes are often met with substantial public resistance (Berger, Citation2022; Rhodes et al., Citation2017; Umar et al., Citation2021).
Numerous studies have linked public support for the environment and other climate policies to trust in government institutions, demonstrating that individuals often support a broad range of environmental policy initiatives if they trust different governmental institutions as well as actors and/or reside in areas with higher rates of trust and confidence (Fairbrother et al., Citation2019; Harring & Jagers, Citation2013; Hmaittane et al., Citation2019). In light of the fact that confidence in lawmakers and the political structure is weak throughout various regions (Dorfleitner & Grebler, Citation2022; Newton et al., Citation2018), mistrust in government may pose a significant barrier to implementing necessary climate measures. However, most previous research has either mixed up trust in partial government institutions and actors who implement policies and laws (including politicians, political parties, and parliament) or confidence in unbiased public officials and government institutions that incorporate and impose policies and laws. This study contends this is difficult for a number of factors. For instance, a well-functioning democratic system does not always ensure a strong and corruption-free judicial system (Huang, Citation2019; Kaiser & Welters, Citation2019; Rothstein & Teorell, Citation2008). Consequently, public confidence in these institutions is not always correlated. Large-scale research surveys support this disparity, demonstrating that although politicians are among the least trustworthy groups in society (Newton et al., Citation2018).
Nonetheless, the issue of government institutional trust is prevailing in most parts of the world, where emerging economies are still developing at a rapid phase and struggling to achieve higher economic growth and performance. However, the governments and lawmakers of these economies are imposing various environmental policies to deal with the issue of climate change and excessive carbon emissions in time. Still, the literature remained silent in exploring the relationship between government institutional trust and environmental quality. This motivates the current study to empirically investigate the issue for viable and timely policy measures to address the rapidly growing environmental concerns.
Apart from the government institutional trust, several factors and indicators could influence the environmental quality of the country or region, where economic growth and technological innovation got more importance. However, the existing literature fails to provide a thorough picture of the influence exhibited by these variables on the environment and on carbon emissions in particular. Specifically, there are two concepts: firstly, the authors claimed that an increased level of economic growth and technological innovation adversely affects the environmental quality (Adeel-Farooq et al., Citation2020; Erdogan, Citation2021; Murshed & Dao, Citation2020; Wang & Dong, Citation2022;). This is due to higher investment in environmentally friendly energy resources, energy efficiency, and carbon capture technologies (Gao et al., Citation2021; Su et al., Citation2022). Secondly, a group of scholars also claimed that increased economic and technological innovation only focuses on the development of industrial structure and increased productivity while using traditional non-renewable energy resources (Kongkuah et al., Citation2022; Leitão et al., Citation2022; Mughal et al., Citation2022; Rahman et al., Citation2022). Such contradictions in the empirical results could lead to mis specified policy implications, which could adversely affect the country’s sustainable development. This motivates the current study regarding the need to reinvestigate the said nexus in emerging economies.
Following the backdrop, the prime objective of this study is to empirically analyze the influence of government institutional trust on environmental quality. As discussed earlier, government institutional trust is vital in environmental policy-making. Whereas the literature completely ignored such important elements of economic and environmental quality. Therefore, it is a novel contribution of the study, which open the doors for policy-makers and scholars to analyze each region’s situation empirically. The second objective of this study is to analyze whether economic growth has any role in environmental quality? Unlike other studies that provide contradictory results for various regions at different times, this study empirically analyzes the said nexus in the case of the BRICS economies, which is a novel contribution to the contradictory literature using advanced and appropriate second-generation econometric approaches. Lastly, this study aims to reinvestigate the association between technological innovation and environmental quality. However, numerous studies have already explored this nexus. Still, the picture is not crystal clear as the existing literature provides asymmetric results, which could harm the region’s future policies. Therefore, it needs time to reinvestigate this nexus more clearly. By doing so, this study will help the existing literature to provide the actual long-run influence of technological innovation on the environmental quality of emerging economies.
The following is the organization of the study: Section-2 presents a review of relevant literature; Section-3 provides the theoretical framework and methodological setup for empirical estimations; Section-4 indicates empirical results and their discussion, and Section-5 concludes the paper along with the policy implications.
2. Literature review
This section reviews the existing literature concerning the relationship between carbon emissions and economic and non-economic variables, including economic growth, technological innovation, and government institutional trust.
Concerning the carbon emissions and economic growth nexus, the literature is extensive—covering a number of countries and regions across different time periods. For instance, the recent study of (Zafar et al., Citation2022) analyzed 22 top remittance receiving economies from 1986 to 2017. The study found that economic growth is detrimental to environmental quality as it encourages the level of CO2 emissions in the region. However, the increased inflow of remittances increased export diversification, and using renewable energy could help the environmental recovery. Similarly, (Leitão et al., Citation2022) claimed that economic growth is the primary driver of environmental degradation and increased carbon emissions in a panel of 40 countries. Despite several initiatives for environmental recovery, the issue of carbon emissions is still at an increasing level across the world. Where (Kongkuah et al., Citation2022) and (Ahmed & Ahmed, Citation2018) claimed that the increased economic growth, trade, and energy consumption significantly degrades environmental quality due to excessive carbon emissions in the environment in China. However, the study further argued that increased urbanization and stringent environmental policies could adversely impact the emissions level due to increased skill, education level, awareness, and efficient resource utilization. Also, the recent development in the industrial sector, i.e. industrial 4.0, has capabilities of reducing excessive energy consumption, promoting energy efficiency, and reduce negative externalities related to the environment (Jiang et al., Citation2022). It must be noted that economic growth contributes to CO2 due to increased investment in the traditional fossil fuel energy sector and its consumption (Ferrat et al., Citation2021). However, the increased energy consumption further enhances industrial production and other economic activities, which on the one hand, increases the pollution (CO2 emissions) level and economic growth on the other hand (Abbasi et al., Citation2021; Shahbaz et al., Citation2021). However, the latter further argued that volatility or fluctuation in oil prices have an adverse impact on CO2 emissions, which leads to environmental sustainability (Lobato et al., Citation2021). Although most of the studies mentioned above have provided empirical evidence regarding the positive and significant influence of economic growth on environmental degradation and CO2 emissions in particular. Still, several scholars believe that several remedial measures could help reduce carbon emissions and promote environmental sustainability. For instance, (Wang & Zhang, Citation2021) argued that economic growth, although have an environmentally destructive impact: still, renewable energy, trade openness, and higher oil prices could be used as substantial measures to decouple economic growth from CO2 emissions. In the same vein, (Kirikkaleli et al., Citation2022; Salari et al., Citation2021; Yang et al., Citation2021;) illustrate that production efficiency, energy-saving technical development, renewable energy consumption, and financial development could play a substantial role in decoupling economic growth from the CO2 emissions as well as reducing environmental degradation.
Apart from economic growth, there are several factors and indicators of carbon emissions, which scholars and policy-makers have explored in various countries and regions. Specifically, the recent literature empirically argued that technological innovation could play a substantial role in environmental quality. However, the literature regarding the nexus of technological innovation and carbon emissions could be classified into three groups. Firstly, one group of scholars, including (Adebayo et al., Citation2022; Mughal et al., Citation2022; Rahman et al., Citation2022), among others, provide empirical evidence that technological innovation enhances the industrial output, which only contributes to economic growth with increased utilization of fossil fuels: thus, increases the level of CO2 emissions and promote environmental degradation. On the other hand, several studies such as (Abid et al., Citation2022; Bibi et al., Citation2021; Cheng et al., Citation2021; Ji et al., Citation2021; Muhammad et al., Citation2022; Su et al., Citation2022; Umar et al., Citation2022; Wang & Dong, Citation2022; Yang & Umar, Citation2022; Yuan et al., Citation2022;) provides evidence that technological innovation not only contributes to economic growth, but it also enhances energy efficiency and reduces the fossil fuel consumption. Therefore, technological innovation plays a positive role in environmental sustainability. Besides, several recent studies have emphasized that, unlike traditional technological innovation, green technological innovation could negatively influence the increased emissions level (Lin & Ma, Citation2022; Obobisa et al., Citation2022; Razzaq et al., Citation2021; Zeng et al., Citation2022). Thirdly, the existing literature also explores technological innovation’s asymmetric and heterogenous impact on CO2 emissions. Specifically, the recent studies of (Cheng et al., Citation2021; Su et al., Citation2021; Wang et al., Citation2022; Zhao et al., Citation2021;), reveal that the influence of technological innovation on CO2 emissions varies across quantiles, or regions. Apart from the specific influence of technological innovation on emissions, (Abid et al., Citation2022) explored that there exists a bidirectional causal association between economic growth, technological innovation, and CO2 emissions.
Concerning government institutional trust, the existing literature is scant compared to the empirical evidence available for the nexus of economic growth, technological innovation, and CO2 emissions. In the existing literature, studies have empirically analyzed the influence of political instability and stability (Galinato & Galinato, Citation2012; Ulllah et al., Citation2022), political risk (Khan et al., Citation2022), political and social factors (Benlemlih et al., Citation2022), Composite risk index (Hassan et al., Citation2022), economic policy uncertainty (Nakhli et al., Citation2022), governance quality (Omri et al., Citation2021), are few to mention. Specifically, (Zhang et al., Citation2022) analyzed the nexus of institutional factors and environmental quality in BRICS economies from 1996 to 2019. Using the non-linear ARDL approach, the study found that negative law & order and corruption shocks significantly increase environmental degradation. Whereas negative shocks in political stability and government institutional trust substantially promote environmental sustainability via reducing the CO2 emissions level. In addition, studies asserted that increased political stability tends to reduce the positive growing relationship between income and CO2 emissions (Galinato & Galinato, Citation2012). Similarly, (Adebayo et al., Citation2022; Sohail et al., Citation2022), reveals that increased political instability reduces green energy utilization and promotes environmental degradation in Pakistan. On the contrary, the recent study of (Hassan et al., Citation2022) empirically argued that lower political risk significantly reduces the CO2 emissions level, while lower economic, financial, and composite risks help promote CO2 emissions. Moreover, there is bidirectional causality between economic policy uncertainty and carbon emissions (Nakhli et al., Citation2022).
3. Data and methodology
3.1. Theoretical framework and model construction
This study is based on the STIRPAT framework (Dietz et al., Citation2007; York et al., Citation2003). Dietz and Rosa’s STIRPAT concept is among the most well-known in the field of environmental economics. Ehrlich and Holden proposed a quantitative version of the standard IPAT (impact = population + affluence + technology) paradigm. The STIRPAT model is an improvement over the IPAT concept since it tackles the limitations of IAPT's theoretical model, which assumes several causes that impact the environment in equal proportions (Shahbaz et al., Citation2016). The STIRPAT model estimates non-monotonic and non-proportional functional interactions between ecosystem-influencing elements (Tan et al., Citation2016). The STIRPAT model is used as a stochastic model for two main reasons. Firstly, to anticipate ecological implications based on main driving factors, and secondly, to estimate causal effects represented by coefficients in the STIRPAT model.
STIRPAT's primary purpose is to quantify the effects of driving factors on the environment. We consequently choose logarithmic expression for the variable. In log form, the coefficient of STIRPAT's driving force reflects the driving force’s elasticity. In accordance with York et al. (Citation2003), the parameter is known as ‘ecological elasticity’ (ECEL). If STIRPAT's definition and estimate are accurate, the ECEL may be interpreted as the marginal environmental consequences of the associated driving factors. (York et al., Citation2003) also contend that the error component of the STIRPAT approach developed by (Dietz et al., Citation2007) is technology (T) since the white noise component comprises all variables other than population (P) and affluence (A). Additionally, technology (T) may be directly decomposed by introducing other elements that seem to have a potential impact on per unit output into the STIRPAT model. In this case study, carbon emissions are employed to represent environmental quality, which I in the model specification capture. The other three effect elements are population, technology, and affluence (Dietz & Rosa, Citation1997), given as:
Where the general model could be written in the non-logarithmic form below:
(1)
(1)
Where represents cross-sections, while
is the time period. Besides,
indicates the country effect,
reports the present change of the three impact factors, i.e. T, P, and A, on the environment, respectively, while
is the model’s random error.
The logarithmic () version of EquationEquation (1)
(1)
(1) could be expressed as follows:
(2)
(2)
Following the same procedure, this study uses carbon dioxide (CO2: measured kt) emissions as a proxy for environmental quality, whereas the population is proxied by government institutional trust (represented as government stability: GS), affluence is indicated by gross domestic production product (GDP: measured as constant US$2015), which is a general term for economic growth, and the technology is indicated by technological innovation (TI: measured as the number of patents by residents and non-residents. The regression equation for the variables adopted is given as:
Except for the GS, which is extracted from the PRS GroupFootnote1, data for all the variables is extracted from the World Development Indicators of the World BankFootnote2. The data covers BRICS economies, including Brazil, Russia, India, China, and South Africa, over the period of the last three decades, i.e. 1990–2020.
3.2. Estimation strategy
This research analyses the descriptive statistics for variables under investigation to describe the panel data comprehensively. Particularly, descriptive analytics encompasses the mean, median, and range values, the latter comprising the lowest and highest values of observation. This research also investigates the variable’s standard deviation, which indicates the spread of data from the overall mean and demonstrates the volatility of the temporal variable. In addition, two normality metrics are employed to investigate the distributional feature of the data. Especially, skewness and Kurtosis have been used to verify whether a variable’s distribution meets the normalcy criterion. Skewness and Kurtosis nonetheless provide empirical evidence regarding the dispersion of the variable. However, this paper investigates the normality issue with more precision. Specifically, this study used the (Jarque & Bera, Citation1987) normality test, which evaluates skewness and excess Kurtosis and maintains their values at zero—suggesting the null hypothesis of a normally distributed for variable. Following is Jarque-Bera’s empirical equation for normality statistics:
(3)
(3)
Seeing as this research focuses on panel data, it is appropriate to employ panel data techniques. The first phase of this panel inquiry is to evaluate the Slope heterogeneity and Cross-section Dependence of the selected Panel data. Countries on the panel may exhibit resemblance in some areas and variances in others. However, the homogenous characteristics of countries may lead to biased forecasts in econometric analysis, particularly in panel estimates (Çoban & Topcu, Citation2013; Wei et al., Citation2022). Therefore, assessing the homogenous or heterogeneous characteristics of the BRICS economies is necessary. In this instance, we used the slope coefficient homogeneity (SCH) test devised by (Pesaran & Yamagata, Citation2008) to examine coefficients identical to the assumptions: slope coefficients are homogeneous. The fundamental formulas for the preceding specification are provided below:
(4)
(4)
(5)
(5)
Where denotes the slope coefficient homogeneity (SCH) and
denotes the SCH that has been modified.
Numerous factors may increase a nation’s dependence on the rest of the world in today’s globalized environment, in which a change in a particular variable in one economy may have repercussions on the variable in another country or area. However, disregarding cross-sectional dependence might produce in results that are unreliable and inaccurate (Wei et al., Citation2022). Therefore, we used the cross-section dependency (CD) test created by (Pesaran, Citation2004) to assess cross-section dependence across the BRICS countries. The following is a broad description of the aforementioned test, which presupposes the independence of cross-sections:
(6)
(6)
Since panel data difficulties, i.e. SCH and CD, are prevalent in the data, this research used an adequate unit root estimation method to address the issue. This research used the cross-sectional IPS (i.e. CIPS) test designed by (Pesaran, Citation2006, Citation2007) originally presented a factor model to investigate unexplainable cross-sectional means for cross-sectional dependence. Employing the same approaches, (Pesaran, Citation2007) integrates mean and first differential cross-section lags to the Augmented Dickey-Fuller (ADF) regression model. This method allows for cross-sectional dependency even if the panel is imbalanced (N < T or N > T). The simple formula for the ADF's cross-section is:
(7)
(7)
In EquationEquation (5)(7)
(7) ,
denotes the mean number of observations. To avoid autocorrelation problem, it is possible to put the first differenced lags of
and
into the above equation, as shown below:
(8)
(8)
Each component of cross-sectional CIPS data is averaged (CADFi). The main CIPS model is expressed as below, which assumes the presence of unit root in the data as a null hypothesis:
(9)
(9)
For a panel data analysis, it is important to use stationary data, following the backdrop that reveals that all the variables are stationary. This allows for identifying the long-run equilibrium relationship between the variables. In this regard, this study uses two cointegration approaches. Firstly, the Kao residual cointegration test proposed by (Kao, Citation1999) assumes no cointegration between the variables as null hypothesis. Secondly, as a result of the diagnostic tests revealing heterogeneous slope coefficients and validating cross-section dependency. Therefore, this research employs a suitable empirical method that accounts for the aforementioned difficulties. Specifically, (Westerlund, Citation2007) error correction approach is implemented. This test posits that the error correction term has a zero—null hypothesis value. In addition, this assessment is effective in terms of considering both the group mean statistics, and the panel statistics, which could be obtained via using the following equation:
(10)
(10)
(11)
(11)
Where both EquationEquations (8)(10)
(10) and Equation(9)
(11)
(11) evaluate the group mean statistics.
(12)
(12)
(13)
(13)
EquationEquations (10)(12)
(12) and (11) evaluate the panel statistics.
This study confirms the existence of cointegration between CO2 emissions, economic growth, technological innovation, and government institutional trust. Therefore, it is best of the research interest to explore the long-run elasticities for each variable under-taken. This study observed that in the existing research work, most of the studies had used the FMOLS, DOLS, and CCR approaches, which provide long-run coefficients. Still, these approaches are limited in terms of tackling various panel data concerns like slope heterogeneity, cross-section dependence, and endogeneity, while ignoring such issues could provide biased estimates (Danish & Ahmad, Citation2018). Since this study validates the persistence of slope heterogeneity and dependence of cross-section, therefore, this study uses appropriate long-run estimators such as the mean group (MG) and augmented mean group (AMG), which account for cross-section dependence, endogeneity, and slope heterogeneity (Danish & Ahmad, Citation2018).
This study follows (Khan et al., Citation2019) by adopting the dynamic ordinary least square (DOLS) and fully modified ordinary least square (FMOLS) methodologies, which are the parametric (DOLS) and non-parametric (FMOLS) approaches, respectively, to provide the findings in a robust and unbiased manner: and dependable estimators by addressing the challenges of endogeneity and serial correlation. The DOLS method is deemed effective since it addresses the problem of non-stationarity in time series data. As a result of the orthogonal error term cointegration equation, the DOLS method contains the augmentation of cointegration regression with both leads and lags.
In addition to the robustness test, this research aims to investigate the causative relationship among carbon emissions and the regressors since previous estimators failed to demonstrate a causal link between the study variables. In this regard, this research employs the panel Granger causality heterogeneous test developed by (Dumitrescu & Hurlin, Citation2012), which effectively addresses the panel data concerns as described.
4. Results and discussion
This section presents the empirical outcomes obtained via the previously mentioned specifications and discussion. Before moving to the analysis of long-run elasticities, this study evaluates the descriptive statistics for each variable under consideration—reported in . Specifically, the mean, median, and range, including the maximum and minimum values, are positive. This indicates the progressiveness of variables such as CO2, GDP, TI, and GS. This study noted that all variables’ maximum and minimum values have substantial variations. Therefore, this study evaluates the standard deviation of all the variables, reflecting volatility or fluctuation in the time series variables. The results show that technological innovation is the most volatile variable, followed by CO2, GDP and GS, with the standard deviation of 0.599, 0.478, 0.445, and 0.126446, respectively. In addition to the descriptive statistics, this study also analyzed the normality of each variable. In this sense, the skewness and Kurtosis are evaluated, indicating statistical values other than 1 and 3, respectively. However, this study uses the Jarque and Bera normality (1987) test for comprehensive normality analysis. The test’s empirical findings provide significant statistical estimates for CO2, TI, and GS at 5% and 1% levels. However, the results are found insignificant for GDP, which validates the normal distribution of the variables. Hence, in the case of the former three variables, the null hypothesis could be rejected, and it is concluded that CO2, TI, and GS follow the asymmetric distribution.
Table 1. Descriptive and normality stats.
After the descriptive and normality statistics, this study employed the panel diagnostic test, including slope heterogeneity and cross-section dependence. Empirical results for these tests are provided in . Since these are the primary issues in the panel data and overlooking these issues could lead to biased and inefficient estimates. From the results, both the SCH () and ASCH (
) are found significant at a 1% level. The significant results reject the null hypothesis of homogenous slope coefficients. Instead, the slopes are heterogeneous. In addition, the cross-section dependence test provides statistically significant statistics for each variable under consideration. Therefore, the null hypothesis of cross-section independence could be rejected, and it is concluded that the variables are cross-sectionally dependent. The cross-sectional dependence illustrates that any type of shock in a particular variable and a specific country could have a spillover influence on the variable in another region.
Table 2. Diagnostic tests.
Since the diagnostic tests validate the slope coefficient heterogeneity and cross-section dependence, utilizing a first-generation unit root test could provide misleading results as such approaches cannot tackle the slope heterogeneity and cross-section dependence issues. In this regard, the current study utilizes the second-generation CIPS unit root test developed by (Pesaran, Citation2007), which is more powerful in dealing with the said issue. The estimated results for the said test are provided in . Only GS is found statistically significant at I(0), while CO2, GDP, and TI are non-stationary. Since it is pertinent that the variables must be stationary for identification of the long-run elasticities. Therefore, the unit root of these variables is also analyzed on the first difference. This time, these variables provide statistically significant estimates at a 1% level to reject the null hypothesis of unit root presence. Thus, all the variables are found stationary despite the fact that not all the variables are I(1) but follow mixed order of integration.
Table 3. Unit root.
Since the stationarity of all the variables under consideration is confirmed, it is essential to examine whether the long-run association exists between the variables. Concerning, this study employed two cointegration tests, i.e. Kao residual cointegration test and the (Westerlund, Citation2007) cointegration test. The empirical outcomes of these tests are provided in . Regarding the former test, the results provide significant results at 5% to reject the null hypothesis of no cointegration between the variables. Similarly, the (Westerlund, Citation2007) ECM test also provides statistically significant estimates for
and
Therefore, the null hypothesis of no cointegration between the variables could be rejected as the error correction is not zero. It is concluded that CO2, GDP, TI, and GS are cointegrated, and a long-run equilibrium relationship exists between them.
Table 4. Cointegration results.
After validating the long-run equilibrium relationship between the variables, this study uses the second-generation long-run estimators that could allow for slope heterogeneity and cross-section dependence of the panel. Specifically, two estimators are used: the mean group and augmented mean group estimators. The empirical results for these estimators are provided in . The results show that economic growth positively and significantly causes an increase in the CO2 emissions in the BRICS economies. More specifically, an increase of one percent in the GDP enhances CO2 emissions by 0.5278% (MG) and 0.5221% (AMG). There are several channels through which economic growth enhances environmental degradation. For instance, Increased industrial activity in economies with a low degree of economic development results in a rise in energy-intensive output and pollution emissions. However, the increased emissions level is directly linked to fossil fuel energy, which is detrimental to environmental sustainability(Mughal et al., Citation2022; Rahman et al., Citation2022). Also, the industrial sector tends to increase productivity for profit generation, whereas fossil fuels are a cost-effective measure to enhance profitability. Therefore, the industrial sector’s extreme reliance on fossil fuels leads not only to natural resource depletion but also causes excessive carbon emissions, which is disastrous for environmental quality. The empirical findings of this study are in line with the empirical results of (Ahmed & Ahmed, Citation2018; Leitão et al., Citation2022; Shahbaz et al., Citation2021; Zafar et al., Citation2022), which also validate the harmful impact of economic growth on environmental quality in different regions.
Table 5. Long-run estimates.
On the contrary, technological innovation adversely affects CO2 emissions in the BRICS economies during the selected time period. An increase of one percent in the TI reduces carbon emissions by 0.1491% (MG) and 0.1218% (AMG). These results are statistically significant at 1% and 5%, respectively. In contrast to the industrial revolution, technological improvements are no longer predominantly driven by coal, iron, and steam. Rather, new technology has resulted in more sustainable practices, improved management of the available natural resources, and the use of solar and other renewable energy sources. It has been shown in the literature that they have a very favorable effect on the environment. Specifically, the recent technological innovation is more concerned about environmental recovery rather than just increasing industrial output. For instance, the recent development in technological innovation in the shape of renewable energy, nuclear power, carbon capture and storage, improved fuel efficiency, electric vehicles, fuel blending, advanced diesels and hybrids, and hydrogen fuel cells, to name a few, are considered as innovative and efficient in terms of environmental sustainability. The empirical results of this study is consistent with the empirical results of (Cheng et al., Citation2021; Erdogan, Citation2021; Godil et al., Citation2021; Wang & Dong, Citation2022;), which also provide evidence that technological innovation enhances energy efficiency, renewable’s consumption, and reduces environmental degradation. In addition, this found the negative impact of government institutional trust on environmental degradation. A one percent increase in the GS causes a significant decrease in CO2 emissions by 0.0463% (MG) and 0.0622% (AMG), with a one percent significance level. There is a prominent channel through which government institutional trust promotes environmental sustainability. Specifically, the more the government is stabilized, the more the authorities pay attention towards environmental recovery and policies regarding pollution emissions in the industrial sector. Unlike political instability, political stability reduces corruption by strengthening economic and financial institutes, which further help regulate the sustainable extraction of natural resources. Moreover, government institutional trust helps construct and implement stringent environmental policies, reducing pollution-intensive industrial production. Hence, political stability is a vital factor in environmental sustainability. The empirical findings are in line with the earlier studies of (Adebayo, Citation2022; Galinato & Galinato, Citation2012; Sohail et al., Citation2022), which empirically validate the positive nexus of government and political stability with environmental quality improvement. ()
Table 6. Robustness.
After identifying the long-run elasticities for each variable, this study employed the FMOLS and DOLS specifications as robustness tools to validate the empirical findings of MG and AMG. The empirical outcome of the FMOLS and DOLS is provided in , where the influence of economic growth is found to be positive on environmental degradation. Despite a small difference in the magnitude values, the influence remained the same, which is consistent with the existing studies of (Abbasi et al., Citation2021; Leitão et al., Citation2022). Similarly, the empirical outcomes suggest the negative impact of TI and GS on CO2 emissions, which is consistent with the estimate of earlier techniques. Thus, the estimated results validate the empirical results and are reliable and statistically significant at 1%, 5%, and 10% levels.
Once the long-run coefficients are obtained and validated by parametric and non-parametric approaches, this study tends to analyze the causal association between the variables under consideration, as the earlier specifications are limited in terms of displaying the causal nexus between the variables. In this regard, the current study employed the Dumitrescu-Hurlin heterogenous granger causality test, which also tackles the panel data issue of slope heterogeneity and cross-section dependence. The empirical results of the test are provided in . The empirical results show that there is a two-way causal association between the variables under consideration. That is, the GDP, TI, and GS significantly cause CO2 emissions, while the feedback effect is present, where the CO2 emissions also cause these variables. The significance level for the bidirectional causal association is significant at a 1% level for all the variables. The existence of a causal association between these variables reveals that any policy level changes in each variable could have a spillover effect on the CO2 emissions. Therefore, economic growth, technological innovation, and government institutional trust could be used as a policy to curb carbon emissions in the BRICS economies.
Table 7. Dumitrescu-Hurlin causality test.
5. Conclusion and policy implications
The issue of climate change, global warming, and environmental degradation are considered a threat to human health and sustainable development. Nonetheless, countries across the globe are taking various measures to tackle this rapidly growing issue. Still, the emerging economies are paying more attention towards economic expansion and development. Simultaneously, the emerging economies (BRICS in particular) are also evolving technologically. Therefore, it is important to empirically investigate these economies to explore the recent trends and dynamics of economic growth, technological innovation, and government stabilization on environmental quality in the last three decades. Using advanced and appropriate econometric approaches, these empirical results reveal that the BRICS economies are cross-sectionally dependent, where any shock in a particular variable has a spillover effect on the variable in another country. Besides, all the mentioned variables are cointegrated, allowing the second-generation long-run estimators to be used. Particularly, the mean group and augmented mean group estimators asserted that economic growth is a viable factor in increased CO2 emissions in the region. Since the BRICS economies are paying more attention to developing the industrial sector. Therefore, the energy demand in this region is rapidly increasing, which is a primary reason for increased environmental degradation. Similarly, the higher demand for non-renewable energy resources further motivates the investors in the BRICS region to invest in natural resources, which boosts the depletion of natural resources, consequently leading to higher emissions in the region. On the contrary, technological innovation is found on the rise in these economies, which not only stimulates productivity and industrial efficiency but also increases fuel efficiency and renewable energy production and consumption, due to which the CO2 emissions in the BRICS region decline. Moreover, government institutional trust is also an important factor for the BRICS environmental sustainability as it reduces corruption, motivates investors to invest in environmentally friendly resources, enhances institutional quality, and reduces CO2 emissions.
Based on the empirical results, this study suggests policies for the environmental sustainability of BRICS economies. Firstly, economic growth is positively associated with pollution emissions due to the excessive use of non-renewable natural resources. Therefore, policies that could divert economic growth from investment and subsidizing the fossil fuel industry towards environmentally friendly resources are required. Further, higher economic growth could be used as a policy tool for environmental recovery. Secondly, technological innovation is found to be harmful to CO2 emissions: therefore, the BRICS economies are suggested to increase investment in research and development, advanced technologies, and education to promote the culture and awareness about environmental recovery. Besides, this study recommends subsidizing the firms actively involved in environmentally friendly technologies and promoting energy efficiency and renewable energy resources. Lastly, government institutional trust is a substantial factor in environmental sustainability. Therefore, the government should take serious steps in maintaining stability in the institutional quality, regulatory quality, political system, etc., to appropriately consider environmental concerns and sustainable exploitation of natural resources.
Disclosure statement
No potential conflict of interest was reported by the authors.
Notes
1 For data, visit: https://www.prsgroup.com/
2 For data, visit: https://databank.worldbank.org/source/world-development-indicators
References
- Abbasi, K. R., Shahbaz, M., Jiao, Z., & Tufail, M. (2021). How energy consumption, industrial growth, urbanization, and CO2 emissions affect economic growth in Pakistan? A novel dynamic ARDL simulations approach. Energy, 221, 119793. https://doi.org/10.1016/j.energy.2021.119793
- Abid, A., Mehmood, U., Tariq, S., & Haq, Z. U. (2022). The effect of technological innovation, FDI, and financial development on CO2 emission: Evidence from the G8 countries. Environmental Science and Pollution Research International, 29(8), 11654–11662. https://doi.org/10.1007/s11356-021-15993-x
- Adebayo, T. S. (2022). Renewable energy consumption and environmental sustainability in Canada: Does political stability make a difference? Environmental Science and Pollution Research, 29(40), 61307–61322. https://doi.org/10.1007/s11356-022-20008-4
- Adebayo, T. S., Oladipupo, S. D., Adeshola, I., & Rjoub, H. (2022). Wavelet analysis of impact of renewable energy consumption and technological innovation on CO2 emissions: Evidence from Portugal. Environmental Science and Pollution Research, 29(16), 23887–23904. https://doi.org/10.1007/s11356-021-17708-8
- Adeel-Farooq, R. M., Raji, J. O., & Adeleye, B. N. (2020). Economic growth and methane emission: Testing the EKC hypothesis in ASEAN economies. Management of Environmental Quality: An International Journal, 32(2), 277–289. https://doi.org/10.1108/MEQ-07-2020-0149
- Ahmed, K., & Ahmed, S. (2018). A predictive analysis of CO2 emissions, environmental policy stringency, and economic growth in China. Environmental Science and Pollution Research International, 25(16), 16091–16100.
- Benlemlih, M., Assaf, C., & El Ouadghiri, I. (2022). Do political and social factors affect carbon emissions? Evidence from international data. Applied Economics, 54(52), 1–14.
- Berger, D. (2022). Investor sentiment: A retail trader activity approach. Review of Accounting and Finance, 21(2), 61–82. https://doi.org/10.1108/RAF-06-2021-0152
- Bibi, A., Zhang, X., & Umar, M. (2021). The imperativeness of biomass energy consumption to the environmental sustainability of the United States revisited. Environmental and Ecological Statistics, 28(4), 821–841. https://doi.org/10.1007/s10651-021-00500-9
- Cheng, Y., Awan, U., Ahmad, S., & Tan, Z. (2021). How do technological innovation and fiscal decentralization affect the environment? A story of the fourth industrial revolution and sustainable growth. Technological Forecasting and Social Change, 162, 120398. https://doi.org/10.1016/j.techfore.2020.120398
- Cheng, C., Ren, X., Dong, K., Dong, X., & Wang, Z. (2021). How does technological innovation mitigate CO2 emissions in OECD countries? Heterogeneous analysis using panel quantile regression. Journal of Environmental Management, 280, 111818.
- Çoban, S., & Topcu, M. (2013). The nexus between financial development and energy consumption in the EU: A dynamic panel data analysis. Energy Economics, 39, 81–88. https://doi.org/10.1016/j.eneco.2013.04.001
- Danish, M., & Ahmad, T. (2018). A review on utilization of wood biomass as a sustainable precursor for activated carbon production and application. Renewable and Sustainable Energy Reviews, 87, 1–21. https://doi.org/10.1016/j.rser.2018.02.003
- Dietz, T., & Rosa, E. A. (1997). Environmental impacts of population and consumption. In Environmentally significant consumption: Research directions (pp. 92–99). National Academy of Sciences. https://www.researchgate.net/profile/James-Sweeney-12/publication/265153679_Environmentally_Significant_Consumption_Research_Directions/links/55b7799408aec0e5f4381e63/Environmentally-Significant-Consumption-Research-Directions.pdf#page=101
- Dietz, T., Rosa, E. A., & York, R. (2007). Driving the human ecological footprint. Frontiers in Ecology and the Environment, 5(1), 13–18. https://doi.org/10.1890/1540-9295(2007)5[13:DTHEF2.0.CO;2]
- Dorfleitner, G., & Grebler, J. (2022). Corporate social responsibility and systematic risk: International evidence. The Journal of Risk Finance, 23(1), 85–120. https://doi.org/10.1108/JRF-07-2020-0162
- Dumitrescu, E.-I., & Hurlin, C. (2012). Testing for Granger non-causality in heterogeneous panels. Economic Modelling, 29(4), 1450–1460. https://doi.org/10.1016/j.econmod.2012.02.014
- Erdogan, S. (2021). Dynamic nexus between technological innovation and building sector carbon emissions in the BRICS countries. Journal of Environmental Management, 293, 112780. https://doi.org/10.1016/j.jenvman.2021.112780
- Fairbrother, M., Sevä, I. J., & Kulin, J. (2019). Political trust and the relationship between climate change beliefs and support for fossil fuel taxes: Evidence from a survey of 23 European countries. Global Environmental Change, 59, 102003. https://doi.org/10.1016/j.gloenvcha.2019.102003
- Ferrat, Y., Daty, F., & Burlacu, R. (2021). Short- and long-term effects of responsible investment growth on equity returns. The Journal of Risk Finance, 23(1), 1–13. https://doi.org/10.1108/JRF-07-2021-0107
- Galinato, G. I., & Galinato, S. P. (2012). The effects of corruption control, political stability and economic growth on deforestation-induced carbon dioxide emissions. Environment and Development Economics, 17(1), 67–90. https://doi.org/10.1017/S1355770X11000222
- Gao, J., O’Sullivan, N., & Sherman, M. (2021). Chinese securities investment funds: The role of luck in performance. Review of Accounting and Finance, 20(5), 271–297. https://doi.org/10.1108/RAF-07-2020-0182
- Godil, D. I., Yu, Z., Sharif, A., Usman, R., & Khan, S. A. R. (2021). Investigate the role of technology innovation and renewable energy in reducing transport sector CO2 emission in China: A path toward sustainable development. Sustainable Development, 29(4), 694–707. https://doi.org/10.1002/sd.2167
- Harring, N., & Jagers, S. C. (2013). Should we trust in values? Explaining public support for pro-environmental taxes. Sustainability, 5(1), 210–227. https://doi.org/10.3390/su5010210
- Hassan, T., Song, H., & Kirikkaleli, D. (2022). International trade and consumption-based carbon emissions: Evaluating the role of composite risk for RCEP economies. Environmental Science and Pollution Research International, 29(3), 3417–3437. https://doi.org/10.1007/s11356-021-15617-4
- Hmaittane, A., Bouslah, K., & M’Zali, B. (2019). Does corporate social responsibility affect the cost of equity in controversial industry sectors? Review of Accounting and Finance, 18(4), 635–662. https://doi.org/10.1108/RAF-09-2018-0184
- Huang, F. (2019). The impact of downside risk on UK stock returns. Review of Accounting and Finance, 18(1), 53–70. https://doi.org/10.1108/RAF-07-2017-0139
- Hughes, J. E., & Podolefsky, M. (2015). Getting green with solar subsidies: Evidence from the California solar initiative. Journal of the Association of Environmental and Resource Economists, 2(2), 235–275. https://doi.org/10.1086/681131
- Jarque, C. M., & Bera, A. K. (1987). A test for normality of observations and regression residuals. International Statistical Review/Revue Internationale de Statistique, 55(2), 163–172.
- Ji, X., Zhang, Y., Mirza, N., Umar, M., & Rizvi, S. K. A. (2021). The impact of carbon neutrality on the investment performance: Evidence from the equity mutual funds in BRICS. Journal of Environmental Management, 297, 113228. https://doi.org/10.1016/j.jenvman.2021.113228
- Jiang, L., Sakhare, S. R., & Kaur, M. (2022). Impact of industrial 4.0 on environment along with correlation between economic growth and carbon emissions. International Journal of System Assurance Engineering and Management, 13(1), 415–423.
- Kaiser, L., & Welters, J. (2019). Risk-mitigating effect of ESG on momentum portfolios. The Journal of Risk Finance, 20(5), 542–555. https://doi.org/10.1108/JRF-05-2019-0075
- Kao, C. (1999). Spurious regression and residual-based tests for cointegration in panel data. Journal of Econometrics, 90(1), 1–44. https://doi.org/10.1016/S0304-4076(98)00023-2
- Khan, Y., Oubaih, H., & Elgourrami, F. Z. (2022). The role of private investment in ICT on carbon dioxide emissions (CO2) mitigation: Do renewable energy and political risk matter in Morocco? Environmental Science and Pollution Research, 29, 52885–52899.
- Khan, S. A. R., Sharif, A., Golpîra, H., & Kumar, A. (2019). A green ideology in Asian emerging economies: From environmental policy and sustainable development. Sustainable Development, 27(6), 1063–1075. https://doi.org/10.1002/sd.1958
- Kirikkaleli, D., Güngör, H., & Adebayo, T. S. (2022). Consumption‐based carbon emissions, renewable energy consumption, financial development and economic growth in Chile. Business Strategy and the Environment, 31(3), 1123–1137. https://doi.org/10.1002/bse.2945
- Kongkuah, M., Yao, H., & Yilanci, V. (2022). The relationship between energy consumption, economic growth, and CO2 emissions in China: The role of urbanisation and international trade. Environment, Development and Sustainability, 24(4), 4684–4708. https://doi.org/10.1007/s10668-021-01628-1
- Leitão, J., Ferreira, J., & Santibanez-González, E. (2022). New insights into decoupling economic growth, technological progress and carbon dioxide emissions: Evidence from 40 countries. Technological Forecasting and Social Change, 174, 121250. https://doi.org/10.1016/j.techfore.2021.121250
- Lin, B., & Ma, R. (2022). Green technology innovations, urban innovation environment and CO2 emission reduction in China: Fresh evidence from a partially linear functional-coefficient panel model. Technological Forecasting and Social Change, 176, 121434. https://doi.org/10.1016/j.techfore.2021.121434
- Lobato, M., Rodríguez, J., & Romero, H. (2021). A volatility-match approach to measure performance: The case of socially responsible exchange traded funds (ETFs). The Journal of Risk Finance, 22(1), 34–43. https://doi.org/10.1108/JRF-04-2020-0066
- Mughal, N., Arif, A., Jain, V., Chupradit, S., Shabbir, M. S., Ramos-Meza, C. S., & Zhanbayev, R. (2022). The role of technological innovation in environmental pollution, energy consumption and sustainable economic growth: Evidence from South Asian economies. Energy Strategy Reviews, 39, 100745. https://doi.org/10.1016/j.esr.2021.100745
- Muhammad, S., Pan, Y., Agha, M. H., Umar, M., & Chen, S. (2022). Industrial structure, energy intensity and environmental efficiency across developed and developing economies: The intermediary role of primary, secondary and tertiary industry. Energy, 247, 123576. https://doi.org/10.1016/j.energy.2022.123576
- Murray, B., & Rivers, N. (2015). British Columbia’s revenue-neutral carbon tax: A review of the latest “grand experiment” in environmental policy. Energy Policy, 86, 674–683. https://doi.org/10.1016/j.enpol.2015.08.011
- Murshed, M., & Dao, N.T.T. (2022). Revisiting the CO2 emission-induced EKC hypothesis in South Asia: the role of Export Quality Improvement. GeoJournal, 87, 535–563. https://doi.org/10.1007/s10708-020-10270-9
- Nakhli, M. S., Shahbaz, M., Jebli, M. B., & Wang, S. (2022). Nexus between economic policy uncertainty, renewable & non-renewable energy and carbon emissions: Contextual evidence in carbon neutrality dream of USA. Renewable Energy, 185, 75–85. https://doi.org/10.1016/j.renene.2021.12.046
- Newton, K., Stolle, D., & Zmerli, S. (2018). Social and political trust. The Oxford Handbook of Social and Political Trust, 37, 961–976.
- Obobisa, E. S., Chen, H., & Mensah, I. A. (2022). The impact of green technological innovation and institutional quality on CO2 emissions in African countries. Technological Forecasting and Social Change, 180, 121670. https://doi.org/10.1016/j.techfore.2022.121670
- Omri, A., Kahia, M., & Kahouli, B. (2021). Does good governance moderate the financial development-CO2 emissions relationship? Environmental Science and Pollution Research International, 28(34), 47503–47516. https://doi.org/10.1007/s11356-021-14014-1
- Pesaran, M. H. (2006). Estimation and inference in large heterogeneous panels with a multifactor error structure. Econometrica, 74(4), 967–1012. https://doi.org/10.1111/j.1468-0262.2006.00692.x
- Pesaran, M. H. (2007). A simple panel unit root test in the presence of cross‐section dependence. Journal of Applied Econometrics, 22(2), 265–312. https://doi.org/10.1002/jae.951
- Pesaran, M. H., & Yamagata, T. (2008). Testing slope homogeneity in large panels. Journal of Econometrics, 142(1), 50–93. https://doi.org/10.1016/j.jeconom.2007.05.010
- Pesaran, M. H. (2004). General diagnostic tests for cross section dependence in panels (IZA Discussion Paper No. 1240). Institute for the Study of Labor (IZA).
- Rahman, M. M., Alam, K., & Velayutham, E. (2022). Reduction of CO2 emissions: The role of renewable energy, technological innovation and export quality. Energy Reports, 8, 2793–2805. https://doi.org/10.1016/j.egyr.2022.01.200
- Razzaq, A., Sharif, A., Ahmad, P., & Jermsittiparsert, K. (2021). Asymmetric role of tourism development and technology innovation on carbon dioxide emission reduction in the Chinese economy: Fresh insights from QARDL approach. Sustainable Development, 29(1), 176–193. https://doi.org/10.1002/sd.2139
- Rhodes, E., Axsen, J., & Jaccard, M. (2017). Exploring citizen support for different types of climate policy. Ecological Economics, 137, 56–69. https://doi.org/10.1016/j.ecolecon.2017.02.027
- Rothstein, B., & Teorell, J. (2008). What is quality of government? A theory of impartiality in the exercise of political power. Governance, 21(2), 165–190. https://doi.org/10.1111/j.1468-0491.2008.00391.x
- Salari, M., Javid, R. J., & Noghanibehambari, H. (2021). The nexus between CO2 emissions, energy consumption, and economic growth in the US. Economic Analysis and Policy, 69, 182–194. https://doi.org/10.1016/j.eap.2020.12.007
- Shahbaz, M., Shahzad, S. J. H., Ahmad, N., & Alam, S. (2016). Financial development and environmental quality: The way forward. Energy Policy, 98, 353–364. https://doi.org/10.1016/j.enpol.2016.09.002
- Shahbaz, M., Sharma, R., Sinha, A., & Jiao, Z. (2021). Analyzing nonlinear impact of economic growth drivers on CO2 emissions: Designing an SDG framework for India. Energy Policy, 148, 111965. https://doi.org/10.1016/j.enpol.2020.111965
- Sohail, M. T., Majeed, M. T., Shaikh, P. A., & Andlib, Z. (2022). Environmental costs of political instability in Pakistan: Policy options for clean energy consumption and environment. Environmental Science and Pollution Research International, 29(17), 25184–25193. https://doi.org/10.1007/s11356-021-17646-5
- Su, C.-W., Mirza, N., Umar, M., Chang, T., & Albu, L. L. (2022). Resource extraction, greenhouse emissions, and banking performance. Resources Policy, 79, 103122. https://doi.org/10.1016/j.resourpol.2022.103122
- Su, C.-W., Umar, M., & Gao, R. (2022). Save the environment, get financing! How China is protecting the environment with green credit policies? Journal of Environmental Management, 323, 116178. https://doi.org/10.1016/j.jenvman.2022.116178
- Su, C.-W., Xie, Y., Shahab, S., Faisal, C. M. N., Hafeez, M., & Qamri, G. M. (2021). Towards achieving sustainable development: Role of technology innovation, technology adoption and CO2 emission for BRICS. International Journal of Environmental Research and Public Health, 18(1), 277. https://doi.org/10.3390/ijerph18010277
- Tan, X., Dong, L., Chen, D., Gu, B., & Zeng, Y. (2016). China’s regional CO2 emissions reduction potential: A study of Chongqing city. Applied Energy, 162, 1345–1354. https://doi.org/10.1016/j.apenergy.2015.06.071
- Ulllah, Z., Fen, T. X., Tunio, F. H., & Ullah, I. (2022). Impact of CO2 emissions, exchange rate regimes, and political stability on currency crises: Evidence from South Asian countries. The Journal of Asian Finance, Economics and Business, 9(2), 29–36.
- Umar, M., Ji, X., Kirikkaleli, D., & Alola, A. A. (2021). The imperativeness of environmental quality in the United States transportation sector amidst biomass-fossil energy consumption and growth. Journal of Cleaner Production, 285, 124863. https://doi.org/10.1016/j.jclepro.2020.124863
- Umar, M., Mirza, N., Hasnaoui, J. A., & Rochoń, M. P. (2022). The nexus of carbon emissions, oil price volatility, and human capital efficiency. Resources Policy, 78, 102876. https://doi.org/10.1016/j.resourpol.2022.102876
- Wang, Q., & Dong, Z. (2022). Technological innovation and renewable energy consumption: A middle path for trading off financial risk and carbon emissions. Environmental Science and Pollution Research International, 29(22), 33046–33062.
- Wang, Z., Gao, L., Wei, Z., Majeed, A., & Alam, I. (2022). How FDI and technology innovation mitigate CO2 emissions in high-tech industries: Evidence from province-level data of. Environmental Science and Pollution Research International, 29(3), 4641–4653.
- Wang, Q., & Zhang, F. (2021). The effects of trade openness on decoupling carbon emissions from economic growth–evidence from 182 countries. Journal of Cleaner Production, 279, 123838. https://doi.org/10.1016/j.jclepro.2020.123838
- Wei, J., Rahim, S., & Wang, S. (2022). Role of environmental degradation, institutional quality, and government health expenditures for human health: Evidence from emerging seven countries. Frontiers in Public Health, 10, 870767. https://doi.org/10.3389/fpubh.2022.870767
- Westerlund, J. (2007). Testing for error correction in panel data. Oxford Bulletin of Economics and Statistics, 69(6), 709–748. https://doi.org/10.1111/j.1468-0084.2007.00477.x
- Yang, J., Hao, Y., & Feng, C. (2021). A race between economic growth and carbon emissions: What play important roles towards global low-carbon development? Energy Economics, 100, 105327. https://doi.org/10.1016/j.eneco.2021.105327
- Yang, S., & Umar, M. (2022). How globalization is reshaping the environmental quality in G7 economies in the presence of renewable energy initiatives? Renewable Energy, 193, 128–135. https://doi.org/10.1016/j.renene.2022.05.006
- York, R., Rosa, E. A., & Dietz, T. (2003). STIRPAT, IPAT and ImPACT: Analytic tools for unpacking the driving forces of environmental impacts. Ecological Economics, 46(3), 351–365. https://doi.org/10.1016/S0921-8009(03)00188-5
- Yuan, X., Su, C.-W., Umar, M., Shao, X., & Lobonţ, O.-R. (2022). The race to zero emissions: Can renewable energy be the path to carbon neutrality? Journal of Environmental Management, 308, 114648. https://doi.org/10.1016/j.jenvman.2022.114648
- Zafar, M. W., Saleem, M. M., Destek, M. A., & Caglar, A. E. (2022). The dynamic linkage between remittances, export diversification, education, renewable energy consumption, economic growth, and CO2 emissions in top remittance‐receiving countries. Sustainable Development, 30(1), 165–175. https://doi.org/10.1002/sd.2236
- Zeng, S., Li, G., Wu, S., & Dong, Z. (2022). The impact of green technology innovation on carbon emissions in the context of carbon neutrality in China: Evidence from spatial spillover and nonlinear effect analysis. International Journal of Environmental Research and Public Health, 19(2), 730. https://doi.org/10.3390/ijerph19020730
- Zhang, D., Ozturk, I., & Ullah, S. (2022). Institutional factors-environmental quality nexus in BRICS: A strategic pillar of governmental performance. Economic Research-Ekonomska Istraživanja, 35(1), 5777–5789. https://doi.org/10.1080/1331677X.2022.2037446
- Zhao, J., Shahbaz, M., Dong, X., & Dong, K. (2021). How does financial risk affect global CO2 emissions? The role of technological innovation. Technological Forecasting and Social Change, 168, 120751. https://doi.org/10.1016/j.techfore.2021.120751