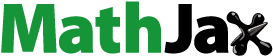
Abstract
COVID-19 adversely affected all market mechanisms, shocked economies, halted business operations, and disrupted supply chains. This study examines macroeconomic instability by assessing the commodities market in highly traded regimes using descriptive statistical analysis, the Economic Policy Uncertainty (EPU) Index, and Markov Regime Swapping (MRS) for data covering February 26 to November 30, 2020. The findings indicate that most commodities respond to stock values in both high and low-volatility regimes of supply and demand. Additionally, commodity prices are still in a low volatility regime due to COVID-19-related market uncertainty. The returns for oil, corn, gold, copper, natural gas, silver, and soybeans adapt to low macroeconomic uncertainty and variable rankings for COVID-19. In contrast, changes in COVID-19 deaths had no impact on natural gas or oil under any regime. As agricultural commodities and valuable metals have less negative feedback to exogenous variables than any other commodities, this model is useful for regulators and stockholders. The results suggest that in a global pandemic, portfolio managers and other stakeholders should use priceless metals like gold as a short-term hedge against systemic market risks to achieve macroeconomic stability and facilitate a rapid recovery in the green economy.
JEL CODES:
1. Introduction
Commodities markets play a crucial role in the world economy, which in turn is sensitive to developments in markets. An economic downturn can affect commodity prices significantly (Sun et al., Citation2021). Clarifying the nature of commodity price movements and their causes may require clarity on the factors that affect product demand and supply. Understanding these factors would therefore make it easier to evaluate how changes in the marketplace, such as disruptions to the export oil market, impact countries that export and import commodities. Formulating policies that support the economic objectives of sustainable development, stable inflation, poverty alleviation, food security, and climate change mitigation depends on this type of research. Since the start of the present decade, several significant events highlighted the intricate and unstable connection between commodities markets and overall economic growth. The COVID-19 pandemic affected energy prices. The collapse of transportation and travel due to the pandemic shutdowns imposed by the US and other nations weakened global demand (X. Gao et al., Citation2022; Y. Huang et al., Citation2020).
The World Health Organization (WHO) declared the COVID-19 pandemic a global emergency in February 2020. It affected 111 countries, with 118,500 cases reported globally (Cao, Citation2020; Ielasi et al., Citation2018). Global growth was expected to increase from 2.4% in 2019 to 2.5% in 2020 (Alacevich, Citation2020). Since the pandemic outbreak, the world economy and commodity markets had a sharp downturn greater than that of the 2008 global financial crisis (Li et al., Citation2022; Su et al., Citation2022; Yakubu & Sarkodie, 2021). Since the first COVID-19-infected case on 26 February 2020, the US recorded the highest single-day fatality rate that exceeded Italy (Reang et al., Citation2021). This halted government processes and forced the implementation of strict lockdowns nationwide, including travel limitations, social distancing, stay-at-home instructions, and other safety measures (Sarkodie & Owusu, Citation2020), which significantly affected other powerful economies like China, Germany, Japan, Canada, France, and the UK (Baloch et al., Citation2021). Before the COVID-19 outbreak in 2020, a 2.5% rise in global growth was expected after the 2.4% in 2019 (Yakubu & Sarkodie, 2021). The COVID-19 pandemic had a more significant impact on the financial market than any other global health emergency, such as the Spanish flu (Baker et al., Citation2020). In contrast, under this economic uncertainty, a comprehensive policy can be effective in the US and other countries facing fiscal difficulties (Adedoyin et al., Citation2020; J. Wang et al., Citation2021). Consequently, the COVID-19 pandemic revealed the vulnerability of the international economic and commodity markets to global crises. Moreover, COVID-19’s influence on commodity markets interrupted both supply and demand. depicts the variable effects on commodities. The first wave of the COVID-19 pandemic increased commodity return volatility considerably (Y. Huang et al., Citation2020). Additionally, investor uncertainty caused by the pandemic resulted in higher commodity prices (Salisu & Sikiru, Citation2020).
This study investigates macroeconomic instability by analysing the commodities market in highly traded regimes. The dynamic nature of the Markov Regime Swapping (MRS) enables us to achieve the research objectives. The findings provide a unique perspective and policy directions for market sustainability and recovery of the green economy. The empirical results offer evidence that helps decision-makers understand the economic effects of COVID-19 on commodities under different regimes, especially during unpredictable times. Regulators, managers, and investors may find it advantageous to hedge against short-term risks in their assets and portfolio.
Our findings may also help regulators and investors identify and predict potential transition points for commodities returns across volatility zones. We evaluated the effects of COVID-19 and the economic uncertainty policy’s regime-switching characteristics using a dynamic MRS model. We applied this model to define the transitions between various commodity price regimes, particularly those caused by structural breaks and the price of crude oil. We included a lagged dependent variable in the MRS model to correct for omitted-variable bias, which added to model parameter estimation bias. The MRS approach can describe temporary volatilities at high speeds (Hamilton, Citation1989). Additional studies demonstrate that the MRS model is capable of accurately forecasting the potential transitions between low and high growth regimes, identifying the mean change in the US GDP with oil price, and examining the volatility in the future value chain of oil-based products (Fong & See, Citation2002; Raymond & Rich, Citation1997).
2. Literature review
2.1. Covid-19 pandemic adverse shocks on product market prices
Several studies provided evidence of gold’s hedging capability as a safe-haven asset during the COVID-19 pandemic (Corbet et al., Citation2020). COVID-19’s effects on financial markets, commodity markets, and the worldwide economy can never be exaggerated. The function of gold as a haven inspired many stockholders and governments (Y. Huang et al., Citation2020). The COVID-19 pandemic affected the streel market in Australia and Brazil, influenced gold mining, and limited the labour movement, especially in Mexico, Peru, and South Africa, hence allowing prices to rise in 2020 (Klees et al., Citation2012). According to Cincinelli and Piatti (Citation2021) and J. J. Wang (Citation2020), deteriorating credit increases the likelihood of default and reduces capital sufficiency. Warning signs across credit exposures, institutional sizes, and nations can help policymakers determine the best course of action. The Islamic stock funds also function as safe havens that can help investors trying to reduce their exposure to pandemic threats and shocks (J. Gao et al., Citation2021; Su et al., Citation2022; Umar et al., Citation2021). Clean energy is a factor with a significant positive spillover impact, whereas bilateral trade and economic growth have an adverse influence on carbon emissions (Guo et al., Citation2022; Liang et al., Citation2022; Wahab et al., Citation2022).
Moreover, China’s industrial base, which represents 50% of the use of copper, plummeted in the first quarter due to COVID-19 mitigation measures (Y. Huang et al., Citation2020). The pandemic generated demand and supply shocks simultaneously that disrupted international supply chains and global trade (Baldwin & Tomiura, Citation2020). Similar to the 2008 global financial crisis, COVID-19 had significant consequences on global economic fragility (Corbet et al., Citation2020). Furthermore, COVID-19 mitigation measures imposed worldwide had a dramatic economic impact worldwide. A recent study identified an important link between the commodity market and other markets (Zhang & Broadstock, Citation2020). Since the pandemic, most commodity values have been volatile. Commodity price dependency increased from 14.8% to 47.9% after the 2008 global financial crisis (L. Wang et al., Citation2022; Zhang & Broadstock, Citation2020). Due to constraints in worldwide economic activities, the COVID-19 pandemic caused a severe drop in the demand and cost of crude oil (G. T. Reddy et al., Citation2020).
2.2. Macroeconomic volatility and commodities markets
Macroeconomic volatility and stockholder sentiment are important market signals that drive commodities prices (Sarkodie & Owusu, Citation2020). Before investing in a commodities market, policymakers and investors often monitor these elements closely (Ahmed, Citation2019). Macroeconomic uncertainty negatively affects stock market revenues, subsequently contributing to higher stock market volatility (Cheng et al., Citation2017). In the three phases of the COVID-19 outbreak, G. Wang and Hagigi (Citation2019) and Yarovaya et al. (Citation2021) examined the effect of human capital efficiency (HCE) on the profitability of equity investments, reporting that firms with higher HCE rankings beat their rivals during the COVID-19 epidemic. The industries most at risk of declining enterprise value and net sales are mining, manufacturing, and retail. Some policy changes may help maintain solvency at the pre- COVID-19 levels. Mirza et al. (Citation2020) found that hybrid support through debt and equity should be offered to counteract worsening business disruptions to prevent a breakdown.
Although some research investigates the impact of COVID-19 on the commodities markets, most of these studies have a narrow focus. Additionally, they include unique items from agriculture, metals, and components reliant on a lag but do not adjust for omitted variable bias, potentially creating an estimation bias that affects the conclusions drawn from the results. Two dangers to the commodities markets are the subject of this paper: (1) COVID-19 and (2) the effects of macroeconomic ambiguity. By analysing the impact of COVID-19 on human well-being as well as the EPU in the US on various commodities, including natural gas, oil, soybeans, corn, gold, silver, steel, and copper, we contribute to the global discussion on the pandemic’s long-term impact on financial growth.
2.3. Link between green finance and the COVID-19 pandemic
Adjacent regions influence the carbon footprint and thus affect spatial variability substantially. In the near term, fiscal decentralization also increases carbon pollution, though it reduces carbon emissions over time and is necessary for meeting the goal of net-zero carbon emissions (Safi et al., Citation2022). A comparative analysis demonstrates that Chinese green funds outperformed their equivalents given the numerous environmentally friendly economic initiatives China implemented (Ji et al., Citation2021). Investments in renewable energy underperformed investments in conventional energy. Additionally, conventional fund managers show market volatility timing. The performance of renewable funds also declined during COVID-19, leading to another investment drag (Naqvi et al., Citation2021).
There was a positive association between short- and long-term investments to address industrial pollution and regional economic growth. However, the adverse impact of urban salaries and overseas investment on projects completed to address contamination is having the opposite effect on regional green development. Policymakers in industrially polluting countries can decrease pollution and promote a green economy in their countries by adopting the Chinese model of green growth in the economy (Imran et al., Citation2022). However, in terms of both size and investment portfolio, fund managers are shifting away from high-risk choices towards low-risk (Rizvi et al., Citation2020). The effectiveness of socially conscious exchange-traded funds (ETFs) is generally comparable to that of traditional ETFs. This is essential for investors who care about the environment as it demonstrates that the added dimension of filtering for socially responsible ETFs does not substantially decrease performance (Lobato et al., Citation2021; Wahab et al., Citation2022) supported the cointegration connections between expenditure, carbon emissions, imports, exports, energy, gross domestic product, and technological innovation and structural breaks (such as the mild 2001 recession; 2008 global financial crisis; 2011 share price decline; and 2014 export decline in France, the UK, Italy, and Japan). For the pre-COVID period through the epidemic, the impacts of human capital adequacy grew much more prominent, one study evaluated outperformance using the adjusted Sharpe and Sortino ratios, Jensen’s alpha, stochastic dominance, market, and variance duration remained constant (Hasnaoui et al., Citation2021).
Funds that significantly outperform their peers that score lower on human capital efficiency have greater human capital adequacy. Equities can increase the effectiveness of human capital to maintain resilience during macroeconomic shocks. Mirza et al. (Citation2020) and Rizvi et al. (Citation2020) analysed the large reduction in valuations across all industries due to the potential drop in sales and rising cost of equity, reporting that the pandemic was the primary cause of the collapse in shareholder value. In rare circumstances, typical businesses in particular industries could lose up to 60% of their intrinsic worth in a single year, The outcomes were constant when the assessment was based on cash inflows or capital gains ().
3. Research methods
3.1. Data sources
This study investigates how daily COVID-19 instances affect commodity prices. We predict that the COVID-19 pandemic had a significant impact on the commodity and financial markets, which will have long-term ramifications for long-term economic growth (Yakubu & Sarkodie, 2021). We analysed the effects for the US using data on COVID-19 outcomes from the John Hopkins University of Medicine database. We collected incidence data for COVID-19 daily between 26 February and 30 November 2020. Given community diffusion, COVID-19 cases were first reported in the US.
Therefore, we consider the daily COVID-19 situation (recovered, confirmed, and death cases) as well as the macroeconomic volatility in the US.
We obtained the macroeconomic uncertainty indicator from the Federal Reserve Bank, and additional computational data for the EPU index based on American newspapers from the New York Fed’s economic data book (Baker et al., Citation2020). We employed the Nasdaq indexes for steel, copper, gold, silver, and other metals as endogenous proxy variables representing commodity prices. We included the Henry Hub soybean, corn, and natural gas prices as additional autonomous variables along with the commercial price of West Texas Intermediate crude oil, which is a common benchmark for crude oil commodities (Scarcioffolo & Etienne, Citation2021). We measured COVID-19 outcomes using the log of daily cases. We computed commodity prices and US macroeconomic uncertainty using the initial disparities of regular commodity market prices (Salisu & Sikiru, Citation2020). provides a summary of COVID-19 cases reported in the US, with a maximum of 13,541,186 instances. depicts the US EPU index and contrasts the price trajectories of the two energy commodities. Copper has recorded the highest coefficient value of 0.0002 and the lowest value count of −0.079, while steel has the highest coefficient value of 0.068, which had a more serious effect on the commodities markets (P11 and P22).
3.2. Model
The MRS gained popularity in financial modelling (Bollain-Parra et al., Citation2021) after its initial application in modelling recessions and business cycle growth, which reflect a gradual long-term pattern (Hamilton, Citation1989). The MRS is a swapping prototype covering price changes, novel energetic pricing, and basic elements that last several periods following a change. The technique became popular in financial modelling. The MRS is defined as
(1)
(1)
The horizontal (x) and vertical (y) axes represent time and frequency, respectively, where a lower frequency signifies a larger size or scope. Time-frequency synchronization may be used to locate regions in the night before going to bed d wherein two sequences co-vary. However, regime model flipping can affect the entire distribution; it is more limited in its impact on the model’s intercept autocorrelation
and volatility
(Bollain-Parra et al., Citation2021). Our framework considers that an exogenous factor shifts between two regimes based on the MRS posterior probability from state (
) to state (
):
(2)
(2)
The chance of a regime shift for any two-chain state is determined by the amount of time in the regime time-varying model using Markov transition possibilities, where where the MRS framework
is equal to
The longer time spent in regime
results in a greater parameter
Meanwhile,
is an exogenous variable in regimes
and
(Hamilton, Citation1989). For convenience, we specify EquationEquation (1)
(1)
(1) as
(3)
(3)
The assessed parameters contain the lagged values of natural gas oil
soybeans
gold
copper
silver
and steel
(corn: CO; oil: OIL; soybeans: SB; natural gas: NGAS; gold: GD; silver: SIL; steel: STL; copper: COP).
4. Results and discussion
4.1. Descriptive statistical analysis
summarises the descriptive statistical analysis for the data, including the standard deviation, mean, skewness, kurtosis, correlations, and Jarque-Bera test.
Table 1. Descriptive statistical analysis.
In 's findings, compared to CO, COP, GD, and the macroeconomic uncertainty index, SB reached its peak aggregate market price in 195 days. The three variables of CON, REC, and DD had an average of 5,318,815, 1,520,024, and 133,416 cases each in the 195 days they were recorded. In addition, the Jarque-Bera results for all series, except GD, reject the null hypothesis (H0) of a normal distribution, implying that all variables save GD deviate from the assumption of normality.
4.2. Unit root test results
In this section, we used the Philip-Perron (PP) and Augmented Dicky-Fuller (ADF) tests to examine the stationarity qualities of the variables. This helps to eliminate spurious regressions, resulting in more reliable estimates (Perron, Citation1990). The ADF and PP unit root results are shown in . At a 1% significance level, both the PP and ADF tests confirmed that all log factors refute the null hypothesis (H0) of a unit root test. Thus, all sampled variables have the value I (0), suggesting that the process is stationary.
Table 2. Unit root test results at two levels.
4.3. Energy commodity markets after COVID-19
provides the MRS model’s constants for natural gas and oil commodities under both regimes. For these commodities, we find two volatility regime types: high volatility and low volatility. and demonstrate that for every 1% increase in daily confirmed COVID-19 incidents, oil returns experience a 0.013% rise under high volatility but a 0.007% decrease under low volatility. The COVID-19 treatment instances
Table 3. Results of variables of regime 1 to 2.
Table 4. Markov regime swapping outcomes: soybean and corn prices.
show positive constant daily consumption-based carbon emissions in both low and high volatility regimes. The high volatility regime, an upsurge in day-to-day COVID-19 recovery cases, raised crude oil returns to values about 0.036% at a p-value of 0.1. In contrast, the low volatility regimes cases rose to 0.027% at a p-value >0.05. In both cases, shows an unfavourable and positive coefficient for EPU, demonstrating that a 1 increase results in a 2.4.
In , the results indicate a 1 to 2% increase in oil revenues in the high volatility zone at a 0.05 p-value and a loss of 0.35% to 36% in oil value revenues. The EPU index is created using the volatility of financial operations. The lagged oil price shows positive and negative coefficients in regimes of high and low volatility at a 0.05 p-value, respectively. This implies that a 1 percentage increase in historical oil prices increases oil returns by 21% in under high volatility while decreasing them by 72% under low volatility.
The worldwide lockdowns and emergencies due to the rise in COVID-19 cases hampered travel and transportation. This condition uses up 67% of oil reserves, mostly in the US, which explains why the low volatility regime has a negative coefficient (Huang et al., Citation2020). A decline in oil earnings increases the possibility that a depression will experience a high volatility regime, according to the significant coefficients between the high volatility zone and the region’s lower prices. Thus, investors are more likely to purchase more oil when the price is low during a pandemic and they are under a lot of stress. In both regimes, the daily COVID-19 deaths and previous oil prices show a constructive relationship with an insignificant effect on oil.
The rise in daily recovered cases of COVID-19 is projected to diminish global lockdown measures and travel restrictions, resulting in increased fuel use for production and transportation as economic activities recover (Y. Huang et al., Citation2020; G. T. Reddy et al., Citation2020). As shows, COVID-19 instances affected gas price recovery. In this regard, adding 1% of COVID-19 cases every day boosts gas output by 0.88% under high-volatility, but prompts a 0.018% decline under low-volatility. COVID-19 lockdown measures implemented in the US forced people to stay indoors and work from home. Hence, the high and positive coefficient of the volatility regime is statistically significant, which can explain the increase in gas-driven power demand. As of 2019, gas contributed 43% of electricity generation in the US (Fraillon et al., Citation2020). In both regimes, daily COVID-19 deaths and delayed natural gas rates show negligible favourable and unfavourable correlations. In the low volatility regime, the coefficient linked with the daily COVID-19 recoveries is undesirable at no expressive level but notably constructive in the high volatility regime. In theory, if the number of daily COVID-19 recoveries increases, then lockdown measures loosen and fewer people stay indoors, thus lowering electricity usage. The lagged natural gas values show statistically significant positive coefficients in both regimes, though they are negative in the high volatility regime. The low volatility region transition possibility is 98% while the high volatility regime transition possibility is insignificant. In the previous regime, a 1% increase in EPU resulted in a 1.1% surge and 0.13% drop in natural gas returns. Our results are consistent with the literature suggesting that during the COVID-19 pandemic, energy commodity prices changed more than metal commodity prices.
4.3. Agriculture commodities
The COVID-19 epidemic had a mixed impact on agricultural commodities and disrupted national and international supply chains, limited exports, and led to stockpiling, which raised concerns about food security. presents the MRS results for soybean and corn. However, the values were 0.018%, 0.016%, and 0.004% less in the low volatility regime. This demonstrates the recovery in the high-volatility regime, which may affect the cost of biofuel crops like corn and soybeans (C. V. Reddy et al., Citation2020).
The results could imply that most agricultural commodities are essential for worldwide food security. illustrates the substantial adverse effect of EPU on corn returns in low volatility, while the economy is characterized by uncertainty under high volatility. Additionally, the low and high volatility regimes have a transitory possibility of 27% and 4.3%, respectively. In , under the high volatility regime, the EPU index as well as the COVID-19 deaths confirmed cases, and recoveries are related to soybean returns, with a significant negative relationship under the low volatility system. Agricultural products had a steady rate throughout the pandemic that resulted in normal economic conditions with low volatility. Moreover, the EPU index and COVID-19 recoveries, deaths, and confirmed cases did not affect soybean returns. It is plausible that agricultural commodities’ poor sensitivity to market shocks is unimportant. Furthermore, the decline in natural gas and oil production, which impacts consumer hoarding behaviour and use of biofuel from soybeans during the initial stages of the lockdown, might be ascribed to the positive high volatility coefficient. In low-volatility regimes, the transition is probably greater than in high-volatility regions (Baffes et al., Citation2020; Khan et al., Citation2021).
4.4. Metals
shows that both the high and low volatility regimes have a significant positive and negative coefficient. An increase of 1% in the COVID deaths and confirmed cases and the EPU index will result in a rise of 0.033%, 0.026%, and 0.06% at a p-value of 0.01 in silver returns, and a decline of 0.37% under high volatility. Nevertheless, the coefficients of COVID-19 deaths, confirmed cases, and recoveries and the EPU index in the regime are 0.62%, 0.29%, 0.62%, and 0.028%, respectively. Silver returns are receptive to previous prices in both regimes and should affect future prices. Under low volatility, the transitional probability is higher than that under high volatility. Hence, the MRS results for silver returns indicate a halt in the first regime rather than the second. Silver returns had a much stronger negative association with COVID-19 deaths and registered cases and the EPU index than did gold returns. This shows that despite their relatively stable pricing relative to other metals, gold and silver in low volatility regimes did not react well to the COVID-19 situation and the EPU indicator (Y. Huang et al., Citation2020). Increased COVID-19 confirmed cases and deaths led the US to enact stricter travel restrictions and lockdowns, which lowered the silver price in the market. In comparison, a surge in COVID-19 recoveries led the US to adopt more flexible lockdown policies, which benefitted silver prices. Furthermore, the MRS model for silver is unlikely to transition under a high-volatility region, implying that silver can work as a haven during an international epidemic, although it is weaker than gold. Due to the lower rate of silver relative to gold throughout the pandemic, investors flocked to silver-backed ETFs, nearly exceeding the prior high established during the 2008 global financial crisis (Huang et al., Citation2020).
Table 5. MRS results: gold and silver prices.
The MRS returns significant positive coefficients in the high volatility zone in . The percentage of COVID-19 deaths (1%), confirmed cases, and recoveries, and the EPU index have a negligible impact on the high volatility regime adjustments, affecting them by 0.276, 0.282, 0.16, and 6.035%, respectively. While COVID-19 recoveries and deaths, and the EPU index do not have statistically significant negative coefficients, confirmed COVID-19 cases do. Gold returns typically increased under both regimes, suggesting that the price of gold varies according to historical supply and demand dynamics of high and low volatility. Gold’s safe-haven properties under high volatility are apparent; we find a 1% fluctuation of the EPU index and confirmed cases, 10% for recoveries, and 5% for deaths. Other research investigating the safe-haven feature of silver and gold indicates that silver is a superior safe-haven in a stock market collapse, which refutes our finding (Tunio et al., Citation2021). The low volatility regime’s results could be related to COVID-19 confirmed cases, which resulted in stringent lockdown regulations that harmed the economy, subsequently affecting the price of gold in the commodity market. According to the MRS results, the first volatility regime is more likely to hold gold returns than the second volatility region. Gold is an excellent hedging commodity even in an international economic emergency, mainly when the gold market is doing well and trading at a superior position. An increase in the gold price highlights indecision in the stock market (Baker et al., Citation2020). Our findings are in line with other research reporting that crises have a negative effect on gold prices, but to a smaller extent than other safe-haven metals (D. Huang & Kilic, Citation2019). These conclusions support the use of gold as a hedge during pandemics to provide short-term protection and market risk diversification.
Furthermore, gold’s value as a hedge varies over time. Our results support earlier findings that gold is an effective hedge against macroeconomic variables (Y. Huang et al., Citation2020). Our results align with existing findings of a correlation between uncertainty and the price volatility of precious metals (Scarcioffolo & Etienne, Citation2021). However, our results point to gold’s inability to defend critical products such as oil, contrary to market shockwaves and the pandemic.
4.5. Common metal commodities
The MRS results for copper returns in show that under low volatility, confirmed COVID-19 deaths and recoveries and the EPU indicator have no statistically significant effects. In contrast, it has a statistically significant reaction under high volatility; a 1% increase in COVID-19 deaths, confirmed cases, and recoveries and the EPU index raises copper returns by 0.41%, 0.53%, 0.82%, and 1.77%, respectively. Unlike silver and gold, which have a substantial sensitivity to external variables in low volatility regimes, copper returns have no such response. Copper prices increased in the third quarter of 2020 by 22%, the highest increase since the second quarter of 2019. Though all external variables had a less substantial beneficial impact on copper returns, copper is unlikely to function as a safe-haven for stockholders. Under high and low volatility, we find a significant positive and negative correlation between staggered copper returns and copper returns, respectively. Hence, copper prices respond to historical events such as supply bottlenecks caused by the increase in COVID-19 cases. The MRS model shows that the residual probability of the low volatility area is higher than that of the high volatility area, consistent with the results above. reports the MRS results for steel, where under low volatility, steel returns will increase by 0.048%, 0.052%, and 0.12%. A 1% fluctuation in COVID-19 recoveries and verified cases and the EPU index will affect steel returns. In contrast, steel returns under high volatility did not significantly correlate with COVID-19 confirmed cases or recoveries, or the EPU index.
Table 6. MRS results: steel and copper.
This suggests that past supply and demand influence steel returns. Moreover, production at Vale in Brazil was disrupted. According to the MRS results, steel is more likely to remain in a low-volatility region than in a high-volatility region. Amidst the COVID-19 pandemic, our findings are consistent with existing reports that gold’s cumulative impulse response is steadier than those of other metals like copper, aluminium, and silver (Bakas & Triantafyllou, Citation2020).
4.6. Model testing
To address potential omitted-variable bias, we employed lagged-dependent variables to check our results. Additionally, we performed extensive diagnostic tests to confirm the remaining independence of the proposed model. summarises the results of these additional tests.
Table 7. Model testing using the diagnostic test of regimes 1 and 2.
reports the results of the CUSUM stability test. (A) Models 4 (B), 8 (C), 12 (D), and 16 (E) are all available (E) Models 20 (F), 24 (G), and 29 are all available (H). Model 32 is a well-liked.
We check the outcomes using the Durbin Watson test, Breusch-Godfrey test, skewness, heteroskedasticity, and kurtosis, where we find no suggestion of sequential correlation, autocorrelation, or heteroskedasticity in most of the estimated models shown here. However, a few models exhibit heteroskedasticity though they do not affect the model’s residual structural stability. The overall result of the square plots is based on the CUSUM square test regression with 95% matching confidence zones, where any measure outside the 95% confidence zone indicates that the model is structurally unstable. Our CUSUM results indicate that plots are inside the 95% confidence zone, demonstrating the long-term stability of our model.
5. Limitations
This study may be limited by the size and accuracy of the sample, as it analyses only a selection of commodities markets and with one frequency, though yearly or monthly may be more appropriate. Future work could include other relevant variables or parameters to extend our results. To the best of our knowledge, we employed appropriate data, theoretical grounding, and econometric approaches to achieve the research objectives. Other theoretical underpinnings and econometric methods might be applicable to this kind of research inquiry.
6. Policy suggestions and future implications
This research provides insights on gold and silver’s hedging potential during pandemics, though their hedging potential changes between Markov regimes since it is time- and regime-dependent. In the short run, investors can effectively hedge against systematic risks in their portfolio investments. Our empirical research may apply to future outbreaks of similar nature and offer guidance for investors. As new rules trigger new economic changes, our data can also help regulators assess the possibility of a continuation of a particular regime in each market. Oil-exporting countries can use the data from oil and natural gas returns to formalize efforts in battling global diseases that affect global energy commodity prices. As the recent market instability affected most commodities, entities such as OPEC may reduce supply to boost demand. Therefore, advanced models such as dynamic autoregressive distributed lag can be used in future studies to examine the consequences of counterfactual shocks across commodities markets.
7. Conclusion
We studied the dynamic relationship between COVID-19 epidemic outcomes, commodity prices, and the EPU index in the US under low and high volatility regimes using the MRS model. The COVID-19 epidemic is creating instability in global commodity markets. In this study, we employed the EPU, together with natural gas, soybeans, oil, corn, gold, silver, copper, and steel, as exogenous variables. All the models in this study include a connection between the variables, indicating regime dependency. Relative to high volatility zones, the anticipated probability transition in low volatility regions is noticeably larger and the prices of many of these commodities should remain stable for longer under low volatility. Hence, low volatility regimes are more likely than high volatility regimes are to sustain economic growth during a pandemic. Therefore, portfolio managers, investors, and policymakers ought to be aware of these facts. High confirmed COVID-19 cases are problematic for oil price commodities in our oil-based Markov framework because they can affect natural gas consumption and pricing. Restrictions related to the COVID-19 pandemic significantly reduced transportation and travel, leading to a 67% demand for oil in low volatility regions. Moreover, we find a positive relationship between the returns on corn and soybeans to verified COVID-19 cases, deaths, and recoveries, and the EPU under high volatility. Corn returns have no significant relationship under low volatility given rural commodities’ low susceptibility to exogenous shocks. We find that soybean returns in both regimes react to their historical supply and demand growth. Further, we identify a positive and significant relationship between gold and silver returns, COVID-19 scenarios, and the EPU index under high volatility. Both high and low goods respond to past market changes and are likely to predict future market developments, according to evidence from the steel and copper MRS models. Steel and copper prices surged by 25% and 22%, respectively, in the third quarter of 2020 due to rising demand and increased imports in China. The positive significant response of copper to the EPU index and COVID-19 outcomes in the high volatility regime may be attributed to rising copper prices and a decline in market supply. To enhance the green economy and improve national development, this special contribution is a milestone in achieving market sustainability, unfavourable shock-mitigation, and macroeconomic stability.
Disclosure statement
No potential conflict of interest was reported by the author(s).
References
- Adedoyin, F. F., Alola, A. A., & Bekun, F. V. (2020). An assessment of environmental sustainability corridor: The role of economic expansion and research and development in EU countries. The Science of the Total Environment, 713, 136726. https://doi.org/10.1016/j.scitotenv.2020.136726
- Ahmed, W. M. (2019). Asymmetric impact of exchange rate changes on stock returns: Evidence of two de facto regimes. Review of Accounting and Finance, 19(2), 147–173. https://doi.org/10.1108/RAF-02-2019-0039
- Ahmed, M. Y., & Sarkodie, S. A. (2021). How COVID-19 pandemic may hamper sustainable economic development. Journal of public affairs, 21(4), e2675. https://doi.org/10.1002/pa.267
- Alacevich, M. (2020). The political economy of the World Bank. Stanford University Press.
- Baffes, J., Kabundi, A. N., & Nagle, P. S. O. (2020). The role of income and substitution in commodity demand. World Bank Policy Research Working Paper, 9122.
- Bakas, D., & Triantafyllou, A. (2020). Commodity price volatility and the economic uncertainty of pandemics. Economics Letters, 193, 109283. https://doi.org/10.1016/j.econlet.2020.109283
- Baker, S. R., Bloom, N., Davis, S. J., Kost, K., Sammon, M., & Viratyosin, T. (2020). The unprecedented stock market reaction to COVID-19. The Review of Asset Pricing Studies, 10(4), 742–758. https://doi.org/10.1093/rapstu/raaa008
- Baker, S. R., Farrokhnia, R. A., Meyer, S., Pagel, M., & Yannelis, C. (2020). How does household spending respond to an epidemic? Consumption during the 2020 COVID-19 pandemic. The Review of Asset Pricing Studies, 10(4), 834–862. https://doi.org/10.1093/rapstu/raaa009
- Baldwin, R., & Tomiura, E. (2020). Thinking ahead about the trade impact of COVID-19. Economics in the Time of COVID-19, 59.
- Baloch, Z. A., Tan, Q., Khan, M. Z., Alfakhri, Y., & Raza, H. (2021). Assessing energy efficiency in the Asia-Pacific region and the mediating role of environmental pollution: evidence from a super-efficiency model with a weighting preference scheme. Environmental science and pollution research international, 28(35), 48581–48594. https://doi.org/10.1007/s11356-021-13663-6
- Bollain-Parra, L., Oscar, V., Aguilasocho-Montoya, D., del Río, M., & de la, C. (2021). Pandemic (COVID-19) news sentiment, economic policy uncertainty and volatility spillover in global leisure and recreation stocks. In Seabra, C., Paiva, O., Silva, C. and Abrantes, J .L. (Eds.), Pandemics and Travel (Tourism Security-Safety and Post Conflict Destinations) (pp. 141–156). Bingley: Emerald Publishing Limited. https://doi.org/10.1108/978-1-80071-070-220211009
- Cao, X. (2020). COVID-19: Immunopathology and its implications for therapy. Nature Reviews. Immunology, 20(5), 269–270. https://doi.org/10.1038/s41577-020-0308-3
- Cheng, Z., Li, L., & Liu, J. (2017). The emissions reduction effect and technical progress effect of environmental regulation policy tools. Journal of Cleaner Production, 149, 191–205. https://doi.org/10.1016/j.jclepro.2017.02.105
- Cincinelli, P., & Piatti, D. (2021). How inefficient is an inefficient credit process? An analysis of the Italian banking system. The Journal of Risk Finance, 22(3/4), 209–239. https://doi.org/10.1108/JRF-08-2020-0184
- Corbet, S., Larkin, C., & Lucey, B. (2020). The contagion effects of the COVID-19 pandemic: Evidence from gold and cryptocurrencies. Finance Research Letters, 35, 101554. https://doi.org/10.1016/j.frl.2020.101554
- Fong, W. M., & See, K. H. (2002). A Markov switching model of the conditional volatility of crude oil futures prices. Energy Economics, 24(1), 71–95. https://doi.org/10.1016/S0140-9883(01)00087-1
- Fraillon, J., Ainley, J., Schulz, W., Friedman, T., & Duckworth, D. (2020). Preparing for life in a digital world: IEA International computer and information literacy study 2018 international report. Springer Nature.
- Gao, J., O’Sullivan, N., & Sherman, M. (2021). Chinese securities investment funds: The role of luck in performance. Review of Accounting and Finance, 20(5), 271–297. https://doi.org/10.1108/RAF-07-2020-0182
- Gao, X., Ren, Y., & Umar, M. (2022). To what extent does COVID-19 drive stock market volatility? A comparison between the US and China. Economic Research-Ekonomska Istraživanja, 35(1), 1686–1706. https://doi.org/10.1080/1331677X.2021.1906730
- Guo, X., Liang, C., Umar, M., & Mirza, N. (2022). The impact of fossil fuel divestments and energy transitions on mutual funds performance. Technological Forecasting and Social Change, 176, 121429. https://doi.org/10.1016/j.techfore.2021.121429
- Hamilton, J. D. (1989). A new approach to the economic analysis of nonstationary time series and the business cycle. Econometrica, 57(2), 357–384. https://doi.org/10.2307/1912559
- Hasnaoui, J. A., Rizvi, S. K. A., Reddy, K., Mirza, N., & Naqvi, B. (2021). Human capital efficiency, performance, market, and volatility timing of Asian equity funds during COVID-19 outbreak. Journal of Asset Management, 22(5), 360–375. https://doi.org/10.1057/s41260-021-00228-y
- Huang, D., & Kilic, M. (2019). Gold, platinum, and expected stock returns. Journal of Financial Economics, 132(3), 50–75. https://doi.org/10.1016/j.jfineco.2018.11.004
- Huang, Y., Yang, H., Xiong, T., Adekoya, D., Qiu, W., Wang, Z., Zhang, S., & Balogun, M.-S. (2020). Adsorption energy engineering of nickel oxide hybrid nanosheets for high areal capacity flexible lithium-ion batteries. Energy Storage Materials, 25, 41–51. https://doi.org/10.1016/j.ensm.2019.11.001
- Ielasi, F., Rossolini, M., & Limberti, S. (2018). Sustainability-themed mutual funds: An empirical examination of risk and performance. The Journal of Risk Finance, 19(3), 247–261. Article 3. https://doi.org/10.1108/JRF-12-2016-0159
- Imran, M., Hayat, N., Saeed, M. A., Sattar, A., & Wahab, S. (2022). Spatial green growth in China: Exploring the positive role of investment in the treatment of industrial pollution. Environmental Science and Pollution Research, 1–14.
- Ji, X., Zhang, Y., Mirza, N., Umar, M., & Rizvi, S. K. A. (2021). The impact of carbon neutrality on the investment performance: Evidence from the equity mutual funds in BRICS. Journal of Environmental Management, 297, 113228.
- Khan, Z., Ali, S., Dong, K., & Li, R. Y. M. (2021). How does fiscal decentralization affect CO2 emissions? The roles of institutions and human capital. Energy Economics, 94, 105060. https://doi.org/10.1016/j.eneco.2020.105060
- Klees, S. J., Samoff, J., & Stromquist, N. P. (2012). The World Bank and education: Critiques and alternatives. (Vol. 14) Springer Science & Business Media.
- Li, X., Su, C.-W., Li, Z., & Umar, M. (2022). What threatens stock market returns under the COVID-19 crisis in China: The pandemic itself or the media hype around it? Economic Research-Ekonomska Istraživanja, 1–22.
- Liang, C., Umar, M., Ma, F., & Huynh, T. L. (2022). Climate policy uncertainty and world renewable energy index volatility forecasting. Technological Forecasting and Social Change, 182, 121810. https://doi.org/10.1016/j.techfore.2022.121810
- Lobato, M., Rodríguez, J., & Romero, H. (2021). A volatility-match approach to measure performance: The case of socially responsible exchange traded funds (ETFs). The Journal of Risk Finance, 22(1), 34–43. https://doi.org/10.1108/JRF-04-2020-0066
- Mirza, N., Hasnaoui, J. A., Naqvi, B., & Rizvi, S. K. A. (2020). The impact of human capital efficiency on Latin American mutual funds during Covid-19 outbreak. Swiss Journal of Economics and Statistics, 156(1), 1–7. https://doi.org/10.1186/s41937-020-00066-6
- Naqvi, B., Mirza, N., Rizvi, S. K. A., Porada-Rochoń, M., & Itani, R. (2021). Is there a green fund premium? Evidence from twenty seven emerging markets. Global Finance Journal, 50, 100656. https://doi.org/10.1016/j.gfj.2021.100656
- Perron, P. (1990). Testing for a unit root in a time series with a changing mean. Journal of Business & Economic Statistics, 8(2), 153–162.
- Raymond, J. E., & Rich, R. W. (1997). Oil and the macroeconomy: A Markov state-switching approach. Journal of Money, Credit, and Banking, 29(2), 193–213. https://doi.org/10.2307/2953675
- Reang, D., Hazarika, A., Sileshi, G. W., Pandey, R., Das, A. K., & Nath, A. J. (2021). Assessing tree diversity and carbon storage during land use transitioning from shifting cultivation to indigenous agroforestry systems: Implications for REDD + initiatives. Journal of Environmental Management, 298, 113470. https://doi.org/10.1016/j.jenvman.2021.113470
- Reddy, C. V., Reddy, I. N., Ravindranadh, K., Reddy, K. R., Shetti, N. P., Kim, D., Shim, J., & Aminabhavi, T. M. (2020). Copper-doped ZrO2 nanoparticles as high-performance catalysts for efficient removal of toxic organic pollutants and stable solar water oxidation. Journal of Environmental Management, 260, 110088. https://doi.org/10.1016/j.jenvman.2020.110088
- Reddy, G. T., Reddy, M. P. K., Lakshmanna, K., Kaluri, R., Rajput, D. S., Srivastava, G., & Baker, T. (2020). Analysis of dimensionality reduction techniques on big data. IEEE Access. 8, 54776–54788. https://doi.org/10.1109/ACCESS.2020.2980942
- Rizvi, S. K. A., Mirza, N., Naqvi, B., & Rahat, B. (2020). Covid-19 and asset management in EU: A preliminary assessment of performance and investment styles. Journal of Asset Management, 21(4), 281–291. https://doi.org/10.1057/s41260-020-00172-3
- Safi, A., Wang, Q.-S., & Wahab, S. (2022). Revisiting the nexus between fiscal decentralization and environment: Evidence from fiscally decentralized economies. Environmental Science and Pollution Research, 29, 58053–58064. https://doi.org/10.1007/s11356-022-19860-1
- Salisu, A., & A., Sikiru, A., A. (2020). Pandemics and the Asia-Pacific islamic stocks. Asian Economics Letters, 1(1), 17413. https://doi.org/10.46557/001c.17413
- Sarkodie, S. A., & Owusu, P. A. (2020). Impact of meteorological factors on COVID-19 pandemic: Evidence from top 20 countries with confirmed cases. Environmental Research, 191, 110101. https://doi.org/10.1016/j.envres.2020.110101
- Scarcioffolo, A. R., & Etienne, X. L. (2021). Regime-switching energy price volatility: The role of economic policy uncertainty. International Review of Economics & Finance, 76, 336–356. https://doi.org/10.1016/j.iref.2021.05.012
- Su, C.-W., Rizvi, S. K. A., Naqvi, B., Mirza, N., & Umar, M. (2022). COVID19: A blessing in disguise for European stock markets? Finance Research Letters, 49, 103135. https://doi.org/10.1016/j.frl.2022.103135
- Su, C.-W., Xi, Y., Tao, R., & Umar, M. (2022). Can bitcoin be a safe haven in fear sentiment? Technological and Economic Development of Economy, 28(2), 268–289. https://doi.org/10.3846/tede.2022.15502
- Sun, T.-T., Su, C.-W., Mirza, N., & Umar, M. (2021). How does trade policy uncertainty affect agriculture commodity prices? Pacific-Basin Finance Journal, 66, 101514. https://doi.org/10.1016/j.pacfin.2021.101514
- Tunio, R. A., Jamali, R. H., Mirani, A. A., Das, G., Laghari, M. A., & Xiao, J. (2021). The relationship between corporate social responsibility disclosures and financial performance: A mediating role of employee productivity. Environmental Science and Pollution Research International, 28(9), 10661–10677. https://doi.org/10.1007/s11356-020-11247-4
- Umar, M., Su, C.-W., Rizvi, S. K. A., & Shao, X.-F. (2021). Bitcoin: A safe haven asset and a winner amid political and economic uncertainties in the US?. Technological Forecasting and Social Change, 167, 120680.
- Wahab, S., Imran, M., Safi, A., Wahab, Z., & Kirikkaleli, D. (2022). Role of financial stability, technological innovation, and renewable energy in achieving sustainable development goals in BRICS countries. Environmental science and pollution research international, 29(32), 48827–48838. https://doi.org/10.1007/s11356-022-18810-1
- Wang, G., & Hagigi, M. (2019). The effect of the need for subsequent seasoned equity offerings on earnings management motivation. Review of Accounting and Finance, 18(1), 25–52. https://doi.org/10.1108/RAF-01-2018-0019
- Wang, J. J. (2020). Does analyst forecast dispersion represent investors’ perceived uncertainty toward earnings? Review of Accounting and Finance, 19(3), 289–312. https://doi.org/10.1108/RAF-10-2018-0224
- Wang, J., Umar, M., Afshan, S., & Haouas, I. (2021). Examining the nexus between oil price, COVID-19, uncertainty index, and stock price of electronic sports: Fresh insights from the nonlinear approach. Economic Research-Ekonomska Istrazivanja, 35(1), 2217–2233.
- Wang, L., Ahmad, F., Luo, G., Umar, M., & Kirikkaleli, D. (2022). Portfolio optimization of financial commodities with energy futures. Annals of Operations Research, 313(1), 401–439. https://doi.org/10.1007/s10479-021-04283-x
- Yarovaya, L., Mirza, N., Abaidi, J., & Hasnaoui, A. (2021). Human capital efficiency and equity funds’ performance during the COVID-19 pandemic. International Review of Economics & Finance, 71, 584–591. https://doi.org/10.1016/j.iref.2020.09.017
- Zhang, D., & Broadstock, D. C. (2020). Global financial crisis and rising connectedness in the international commodity markets. International Review of Financial Analysis, 68, 101239. https://doi.org/10.1016/j.irfa.2018.08.003