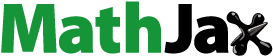
Abstract
Reducing urban-rural income inequality (URII) is a prerequisite in promoting people’s welfare and realizing sustainable development for many developing countries. However, virtually little is understood about how place-based policies affect URII. To fill this void, we utilize China’s Old Revolutionary Development Program (ORDP)—a large-scale and novel type of place-based policy targeted at undeveloped regions, as a natural experiment and use the time-varying difference-in-differences (DID) model to evaluate the effect of ORDP on URII and further investigate its potential mechanisms and heterogeneity effects. The panel data covering 613 Chinese counties from 2010 to 2019 are used. Our results show that ORDP can lead to an average of 11.2% decrease in URII, but this effect takes a period to emerge. Mechanism analysis presents that ORDP mitigates URII chiefly through government intervention and financial development. Further heterogeneity analysis casts light on the fact that ORDP exerts a greater role in alleviating URII in old revolutionary counties that are located in western and central China compared to those located in eastern China. Additionally, the effect of ORDP on URII is greater in undeveloped counties compared with developed counties. Our findings provide enlightenment for the government in China and other developing countries on how to mitigate URII through well-designed place-based policies.
1. Introduction
Urban-rural income inequality (URII) in China—the greatest developing nation in the world, has attracted much attention. It is reported that China’s URII ranks as one of the largest worldwide (Wang et al., Citation2019). As shown in , the ratio of per capita disposable income (PCDI) of China’s urban residents versus rural residents is 1.86 in 1985, while this number increased to 2.56 in 2020, which is a 37.63% growth. The tenth goal in the 2030 Sustainable Development Goals (SDGs) is ‘Reduce inequality within and among countries’, and the widening disparity between urban and rural income is detrimental to economic development and poses a threat to social stability and people’s welfare, which is a major threat to the accomplishment of 2030 SDGs (Tang & Sun, Citation2022; Yao & Jiang, Citation2021; Zhang et al., Citation2021). Therefore, a better understanding of how to reduce URII is a prerequisite in promoting people’s welfare and realizing sustainable development in China.
Figure 1. Changes in income inequality between urban and rural residents in China, 1978–2020.
Source: Author's Source.

Since the 1980s, URII has become a worldwide research topic. Numerous studies have investigated the determinants of URII. Existing related literature has confirmed that urbanization degree (Yao & Jiang, Citation2021; Yuan et al., Citation2020), financial development (Jung & Cha, Citation2021; Su et al., Citation2019; Zhang & Chen, Citation2015), industrial structure upgrades (Hong & Zhang, Citation2021; Zhou & Li, Citation2021), and fiscal incentives (Tang & Sun, Citation2022) are factors that have impacts on URII. However, research examining how URII is impacted by the place-based policy is relatively scarce.
In reality, place-based policies, namely spatially targeted development programs, are considered one of the most effective measures to mitigate URII in theory. For example, Yang et al. (Citation2022) claimed that place-based policies are designed to facilitate economic development in lagging regions and mitigate spatial inequality. Kline and Moretti (Citation2014) pointed out that place-based policies exert an influence on economic activity, income, and employment, as well as the industry structure of targeted regions, which is conducive to alleviating URII. However, previous studies on place-based policies mainly focused on investigating their impact on regional prosperity (such as economic growth) and paid little attention to human welfare (such as income inequality), which contradicts the requirements of high-quality development and the people-oriented development concept in China. Therefore, it is critical to carry on quantitative analysis to empirically examine whether place-based policies can reduce URII and thus achieve the people-based goal of improving people’s well-being (Zhang, Citation2020).
To fill the above research gap, we take advantage of China’s Old Revolutionary Development Program policy (ORDP)— a large-scale and novel type of place-based policy, and employ the time-varying difference-in-differences (DID) model, to empirically examine whether place-based policy can reduce URII. Moreover, we also seek to uncover the veil of the mechanism of place-based policy on URII, which can make us more informed about the potential operating mechanism of place-based policy accordingly. ORDP involves a number of assistance measures in finance, land, talent, and industry, aiming to support the development of old revolutionary base areas. Our study is primarily concerned with the following: (1) estimating the average and dynamic treatment effectiveness of ORDP on URII; (2) investigating the potential mechanism of ORDP on URII; (3) inspecting the heterogeneous treatment effects of ORDP in different old revolutionary base areas and regions with different economic levels. We found that ORDP can lead to an average of 11.2% decrease in URII, but this effect takes a period to emerge. Mechanism analysis presents that ORDP can reduce URII chiefly through government intervention and financial development. Heterogeneity analysis demonstrates that ORDP has a greater influence on URII in old revolutionary counties that are located in western and central China and in counties with low economic levels.
Our research enriches the relevant literature in four aspects. First, to our knowledge, this paper is the first to provide a rigorous quantitative estimation of the social welfare effectiveness of place-based policies. Place-based policies exert a vital influence on assisting state governments to provide a high-quality life and well-being for their people by means of sustainable economic, social, and environmental development. Most existing empirical studies on place-based policies have mainly concentrated on their economic effects. For example, Jia et al. (Citation2020) documented that the Great Western Development Program—a remarkable place-based policy in China, has increased the yearly GDP growth rate by 1.6 percent in the targeted areas. Lu et al. (Citation2019) concluded that China’s Economic Zone Program poses a positive effect on production in the targeted areas. Barbieri et al. (Citation2020) found that the first special economic zone boosted industrial output and economic development in China. Nonetheless, little literature casts light on the welfare effects (such as URII) of place-based policies, particularly in developing countries, which prevents us from gaining a comprehensive understanding of place-based policies. Therefore, our research bridges knowledge gaps by evaluating the impact of place-based policies on URII from the perspective of welfare effects, which is important to further understand place-based policies and enhance the well-being of residents.
Second, we use ORDP as a quasi-natural experiment of place-based policy. Research efforts on place-based policies in China have received extensive attention, but most of the papers lay more emphasis on the impact of special economic zones (SEZ) place-based policy. For example, Lu et al. (Citation2019) proposed that SEZ has a positive influence on industry output and stimulates firm entry. Barbieri et al. (Citation2020) found that SEZ can promote local industrial development. Different from the SEZ program that addresses developed areas, the ORDP program analyzed in this paper specifically targets economically impoverished and geographically disadvantaged areas. In other words, place-based policies have positive policy effects in developed areas does not mean that they will be the same in less developed areas due to disparities in economic basis and resource endowments in different regions. Thus, using ORDP as a quasi-natural experiment provides a comprehensive understanding of the policy effects of place-based policies in less developed areas. Additionally, as noted by Jia et al. (Citation2020), place-based policies in underdeveloped regions are aimed less at addressing market failures and more at providing government intervention. Therefore, ORDP can provide us a valuable opportunity to cast light on how government intervention mitigates URII and promotes social equity.
Third, the existing research is deficient due to the limitations of scale: most studies regarding URII and place-based policies have been conducted at the provincial or prefectural level, with rare studies conducted at the county level. For instance, most studies on the determinants of URII are supported by data from 31 Chinese provinces (Hong & Zhang, Citation2021; Su et al., Citation2019; Yao & Jiang, Citation2021; Zhou & Li, Citation2021), and the existing research on the effectiveness of place-based policies is also mostly based on prefectural, provincial or even national data (Barbieri et al., Citation2020; Jia et al., Citation2020; Keller & Virág, Citation2022; Lu et al., Citation2022). However, some scholars pointed out that the effect of place-based policies may be more likely to occur at the micro-scale (Lu et al., Citation2022). To test the feasibility, we use county-level data to investigate the effect of place-based policies. The county is the third level of China’s four-level local administrations, following the provincial and prefectural city levels. Moreover, the official list of old revolutionary areas in the ORDP is at the county level, and the county government is a more fundamental executor of ORDP than the provincial and municipal government. Therefore, the merits of employing county-level data not only lie in the fact that sufficient observations are tracked and the dependent variables are more accurate compared with those at the provincial or prefectural level, which enables us to obtain more reliable results, but also provide more feasible policy suggestions concerning reducing URII for county governments.
Fourth, we develop a theoretical framework and conduct an empirical analysis of the mechanisms through which the effectiveness of place-based policies on URII happens. Previous research on place-based policies attaches more importance to their effectiveness but the mechanism has not been examined. For example, Barbieri et al. (Citation2020) found that place-based policies can contribute to increased industrial output, but fail to explore the mechanism in depth. We are devoted to uncovering the veil of the impact mechanism of place-based policies on URII. Specifically, we demonstrate that government intervention and financial development are two main mechanisms. Our findings are conducive to enriching existing research on place-based policies and will inspire Chinese authorities to optimize place-based policies in other fields.
Our paper’ framework is structurally arranged as follows. Section 2 introduces the policy background of ORDP. Section 3 constructs the theoretical framework. Section 4 documents the data and model. Section 5 reports the results of empirical estimation, involving the baseline results, a number of robustness tests, the mechanisms of ORDP on URII, and the heterogeneity analysis of ORDP on URII. Section 6 concludes and proposes corresponding policy recommendations.
2. Literature review and policy background
2.1. Literature review
With the rapid growth of the economy, the problem of increasing URII is difficult to alleviate and has attracted much attention (Zhang et al., Citation2021). Therefore, the driving factors of URII have become a classical topic that society, academia, and governments are constantly concerned about. Most studies have investigated the determinants of URII in terms of urbanization degree, financial development, industrial structure upgrades, and fiscal incentives. As for urbanization degree, Yao and Jiang (Citation2021) observed that the higher the degree of urbanization, the wider the URII. Yuan et al. (Citation2020) employed a stepwise meta-regression method and conclude that urbanization causes an increase in URII. Concerning financial development, Jung and Cha (Citation2021) found that financial development may lead to a rise in URII. Huang and Zhang (Citation2020) argued that financial development aggravates URII in the short term, while alleviating URII in the long term. As to industrial structure upgrades, Hong and Zhang (Citation2021) and Zhou and Li (Citation2021) held the view that industrial structure upgrades exert a great impact on reducing URII. Regarding fiscal incentives, Tang and Sun (Citation2022) proposed that fiscal incentives can mitigate URII by means of three approaches, namely labor shifts, financial accessibility, and human capital.
However, apart from the above determinants of URII, place-based policies are also effective measures to narrow URII in theory, yet there exists finite comprehension of the causality between place-based policies and URII. Previous research relating to place-based policies has mostly concentrated on their impact on regional prosperity (e.g., economic growth). Some scholars hold the opinion that place-based policies can promote economic performance. For instance, Wang et al. (Citation2022) demonstrated that Chinese National Economic and Technological Development Zones can advance urban economic development. Ren et al. (Citation2020) denoted that the Northeast China Revitalization Strategy’s implementation has resulted in a 25.7% rise in regional economic development. But others hold contradictory views. For example, Yang et al. (2021) claimed that the economic performance of central and eastern China has even deteriorated under Regional Development Plans. Falck et al. (Citation2019) cast doubt on whether the German Innovative Regional Growth Cores Program, one of the largest place-based innovation policies in Germany, facilitates regional economic growth.
In summary, prior research on place-based policies usually examines their effectiveness on regional prosperity (such as economic growth) and lays less emphasis on welfare effectiveness (such as URII), which deviates from the principle of high-quality development and people-oriented development concept in China. Consequently, our paper attempts to bridge knowledge gaps by evaluating the impact of place-based policies on URII from the perspective of welfare effects, which is conducive to having a supplementary understanding of place-based policies and enhancing human well-being.
2.2. Policy background
Old revolutionary regions, or the old base areas of the Chinese Revolution, refer to the revolutionary base areas created under the direction of the Chinese Communist Party during the period of the Agrarian Revolutionary War and the Anti-Japanese War. Compared with other regions in China, old revolutionary base areas are in a relatively lagging stage of development. Therefore, the Chinese government has given high priority to supporting the revitalization and development of old revolutionary regions. Among them, ORDP is one of the most massive supportive programs.
ORDP has been introduced in five inter-provincial key old revolutionary regions covering a total of 283 counties since 2012. More specifically, as shown in , ORDP was gradually implemented in 2012, 2015, and 2016. In 2012, the Jiangxi-Fujian-Guangdong ORDP was implemented, encompassing 99 county-level old revolutionary areas, 205,894 square kilometers of territory, and 47 million people. In the same year, the Shanxi-Gansu-Ningxia ORDP was implemented, encompassing 24 county-level old revolutionary areas, 80,025 square kilometers of territory, and about 7 million people. In 2015, the Dabie Mountain ORDP was implemented, encompassing 46 county-level old revolutionary areas, 92,196 square kilometers of territory, and about 44 million people. Also in 2015, the Left and Right River ORDP was implemented, encompassing 59 county-level old revolutionary areas, 170,394 square kilometers of territory, and about 23 million people. In 2016, the Sichuan-Shanxi ORDP was implemented, encompassing 55 county-level old revolutionary areas, 141,201 square kilometers of territory, and about 29 million people. reports the distribution of the ORDP counties.
ORDP boasts as one of China’s most significant place-based policies from the perspective of scale, duration, and government investment (Zhang & Zeng, Citation2020). In total, ORDP covers about 10 percent of China’s county-level administrative regions and 11 percent of its total population. ORDP provides a series of financial, fiscal, industrial, and talent policies implemented to promote the prosperity and happiness of the people in the old revolutionary regions. During the 13th Five-Year Plan period, China’s National Development and Reform Commission has arranged more than 300 billion yuan of central budget investment in the ORDP program, accounting for about 1/8 of the total size of central budget investment during the same period.
3. Theoretical framework
3.1. The impact of ORDP on URII
Based on the specific policies implemented in ORDP, we put forward our Hypothesis 1 that ORDP can reduce URII. The reasons are as follows.
First, ORDP can increase rural productivity and the income level of rural residents, which reduces URII. For example, most measures in ORDP program aim to enhance rural productivity and agricultural output by increasing the effective arable land area and promoting agricultural mechanization, which contributes to raising rural residents’ income and reducing URII (Yao & Jiang, Citation2021). Moreover, the rise in rural productivity brought by ORDP can create a rural labor surplus, encouraging rural residents to seek out higher-paying jobs in cities, which can also reduce URII (Wang et al., Citation2019).
Second, ORDP can increase the human capital stock of rural residents and reduce URII. For instance, most of the policies implemented in ORDP program are designed to improve the health, education, and employment levels of rural residents to achieve social equity and sustainable development. These policies help to improve the human capital stock, such as the physical health, education, and labor skills of rural residents (Bai et al., Citation2021). The increase in human capital stock makes the rise of rural income possible and reducing URII since rural laborers with higher human capital stock will transfer to the cities to earn higher income (Hu, Citation2021). At the same time, with more rural residents migrated to cities, the population engaging in agricultural production will decease and the agricultural income of rural residents will increase, thus can reduce URII (Cheng & Ma, Citation2022).
However, we also propose that the effect of ORDP on URII will take a period to emerge, which is our Hypothesis 2. The reasons for this Hypothesis are as follows. First, the policies implemented in ORDP needs to be given a certain amount of time for the local government and enterprises to respond and thus it takes a period for the effect of ORDP to emerge. Second, ORDP realizes its policy effect primary through massive financial investment in infrastructure construction and physical input in rural areas, it also requires to be given a certain amount of time for the infrastructure or other physical capital to be constructed and has a positive effect in reducing URII (Lin, Citation2019).
3.2. The mechanisms of ORDP on URII
In the above subsections 3.1, we analyzed theoretically the role of ORDP on URII, but what are the mechanistic pathways of ORDP leading to the reduction of URII? Clarifying this issue has significant implications in promoting people’s welfare and realizing sustainable development in China. Following the prior research, we propose our Hypothesis 3 that the effect of ORDP on URII is mainly caused by government intervention and financial development. The specific explanations are as follows.
First, ORDP will increase government intervention in the ORDP areas, resulting in a reduction in URII. Previous studies have verified that government intervention can reduce URII (Jia et al., Citation2020; Kline & Moretti, Citation2014). As we stated in introduction, ORDP attaches more importance to providing government intervention instead of addressing market failures. ORDP issues a series of policies to provide government intervention in the old revolutionary regions, which is beneficial to reducing URII. On the one hand, government intervention, such as transfer payments, tax benefits and fee reduction in ORDP regions, is targeted at rural areas (Su et al., Citation2022a, Citation2022b), which makes the rise of rural income possible and can mitigate URII in ORDP regions (Sun et al., Citation2021). On the other hand, another common government intervention implemented in ORDP is government investment in livelihood and welfare projects, such as infrastructure construction (Li et al., Citation2022). This kind of government investment is mainly distributed in rural areas where basic public services are not equal and transportation conditions are more backward, which can also reduce URII (Zhang & Zhang, Citation2021; Zolfaghari et al., Citation2020).
Second, ORDP alleviates URII by boosting financial development. The high level of financial development in ORDP areas broadens the financing channels for rural residents and enables rural households to increase their income more easily, which will in turn mitigate URII significantly (Lassoued, Citation2021; Su et al., Citation2019). On the one hand, financial policies implemented in ORDP, such as encouraging and guiding financial institutions to provide credit support for construction projects in rural regions through financial discounts and fee subsidies, standardizing and developing rural microfinance companies, and raising credit support for small and medium-sized firms, can more effectively distribute financial resources to rural activity and promotes the financial development in rural areas, and thus improve rural residents’ income (Yuan et al., Citation2020). On the other hand, reforms of the financial systems included in ORDP, such as directed credit and strict banking supervision, improving the basic financial guarantee mechanism at the county level, simplifying the administration and decentralization of financial market to enhance the resource allocation efficiency of the capital market, can boost financial development in ORDP regions to some extent and has a positive impact on rural residents’ income (Jha, Citation2019, Citation2020; Lassoued, Citation2021; Qin et al., Citation2022).
4. Data and model
4.1. Data
To evaluate the relationship between ORDP and URII, we manually collect data of URII, ORDP, and other variables affecting URII. We construct our sample relying on the panel data of 613 Chinese counties from 2010 to 2019. And we choose the period between 2010 and 2019 as our sample because of the following factors. First, we need a suitable period (e.g., two years) to conduct the DID estimation before 2012—the first year of the implementation of ORDP. Second, some variables at the county level before 2010 and after 2019 are inaccessible because of data availability. For example, the PCDI of urban residents is only available after 2010, and the rural employed population is only available before 2019.
4.1.1. URII data
With reference to Wang et al. (Citation2019), we use the ratio of PCDI between urban and rural residents to measure URII. To eliminate inflation effect, consumer price indexes of each province based on the price level of 2010 are used to calculate the real income. It is worth noting that since 2014, China’s statistics on the PCDI of rural residents have shifted (Zhang et al., Citation2021). That is, the income of rural residents from 2010 to 2013 was the per capita net income while from 2014 to 2019 was the PCDI. However, since Chinese rural residents have limited social security, their disposable income is equal to their net income.
4.1.2. ORDP data
ORDP, the primary explanatory variable in this study, reveals whether a county has adopted ORDP in a specific year. Specifically, ORDP is assigned a value of 1 if ORDP has been implemented in a certain year in a certain region; otherwise, it is assigned a value of 0. Therefore, the differences in URII before and after the ORDP project between the ORDP county and non-ORDP county are divided to test the effect of ORDP on URII.
4.1.3. Control variables
To describe the impact of ORDP on narrowing URII more accurately and simultaneously minimize estimation errors resulting from colinearity, we control four factors that may affect URII based on previous studies: economic development level, population density, rural employment, and agricultural mechanization. The specifics are as follows.
Economic development level. It is defined as the logarithm of real gross domestic product (GDP). According to the Kuznets theory, URII and economic growth will have an inverted U relation (Wang et al., Citation2019).
Population density. We use the ratio of the total population to the administrative area to measure this variable. The impact of population density on URII is ambiguous. On the one hand, it promotes rural surplus labor to shift from the agricultural sector which has lower income, to the urban industrial sector which has higher income, and thus can reduce URII (Zhang et al., Citation2021). On the other hand, with the increase of population density, the degree of industrialization and urbanization becomes higher and thus will boost the income growth of urban residents and increases URII (Hao et al., Citation2016; Yao & Jiang, Citation2021).
Rural employment. We use the value of rural employed population to measure this variable. For rural residents, employment is a crucial source of income (Zhang, Citation2021). The increase in rural employment opportunities can enhance the income of rural households (Su et al., Citation2019), which may mitigate URII to some extent (Zhou & Li, Citation2021).
Agricultural mechanization. We adopt the total power of agricultural machinery to measure this variable. Agricultural mechanization can influence URII by enhancing the production efficiency of farmers. Large-scale mechanization of agriculture is conducive to the formation of an orderly agricultural production system, saving farmers’ time cost and capital cost, bringing about the improvement of production efficiency and the expansion of production scale. Therefore, agricultural mechanization can increase farmers’ income and reduce URII (Xue & Pan, Citation2021).
4.2. Descriptive statistics
The data of variables stated above were obtained from the China County Statistical Yearbook, the China City Statistical Yearbook, the China Province Statistical Yearbook, and the Statistical Bulletin of Economic and Social Development of each county from 2010 to 2019. The average data in some years was used to remedy the missing data. The descriptive statistics for these variables are shown in .
Table 1. Definition and description of variables.
4.3. Model: time-varying DID method
Due to the different ORDP implementation times, a time-varying DID model is used to evaluate the effectiveness of ORDP on URII. The fundamental tenet of DID method is to choose a pilot group that carries out ORDP and a control group that does not. Subsequently, the effects of ORDP on URII are evaluated by comparing the differences between the dependent variables of the ORDP group and the non-ORDP group before and after the implementation of ORDP. Therefore, the DID model can avoid the endogeneity problem resulting from ORDP implementation and control for the impact of unobservable individual characteristics on URII (Pan & Tang, Citation2021).
First, we use the following time-varying DID model, which is similar to Beck et al. (Citation2010), to evaluate the average treatment effectiveness of ORDP on URII.
(1)
(1)
Where is the URII in county
at year
is a dummy variable that is assigned a value of 1 in the years after county
has implemented ORDP and 0 otherwise. Accordingly, the coefficient of interest
refers to the estimator of
which quantifies the net effects of ORDP on URII. If
is negative and significant, then ORDP narrows URII; if
is positive and significant, then ORDP increases URII; and if
is non-significant, then ORDP does not exert a significant effect on URII.
represents the relevant control variables.
and
indicate county fixed effects and time fixed effects, respectively, and
represents the random error term.
Next, referring to Pan et al. (Citation2022), a dynamic DID model is constructed to examine the dynamic treatment effect of ORDP, which can be defined as:
(2)
(2)
Where denotes the post-ORDP county, otherwise
is the coefficient of
representing the effect of ORDP after the implementation of ORDP in year
. The other variables are in line with EquationEquation (1)
(1)
(1) .
5. Empirical estimation results
In this part, we first evaluate the average treatment effect of ORDP on URII on the basis of the time-varying DID model; second, we assess the dynamic treatment effect of ORDP on URII; third, we conduct a number of robustness tests to improve the reliability of our findings. And then, we validate the underlying mechanisms of ORDP on URII. Finally, we evaluate the heterogeneous effects of ORDP on URII.
5.1. The average treatment effect of ORDP on URII
Estimated from EquationEquation (1)(1)
(1) , the average treatment effect of ORDP on URII is reported in . Stepwise regression was put into effect by sequentially increasing control variables and the outcomes are presented in Columns (1)-(5). It can be seen that the coefficients of
are significantly negative with or without control variables. Specifically, the result from Column (5) shows that ORDP has significantly narrowed URII by 11.2% at the 1% significance level. Therefore, we conclude that ORDP is conducive to mitigating URII in China, which validates our Hypothesis 1. The likely reasons for this baseline result are as follows. On the one hand, ORDP can boost rural productivity and rural residents’ income level, thus reducing URII (Yao & Jiang, Citation2021). On the other hand, ORDP can improve rural residents’ human capital stock, such as physical health level, education, and labor skills, thus can induce rural laborers that have transferred to cities to earn a higher income, which also can reduce URII (Bai et al., Citation2021; Hu, Citation2021).
Table 2. The average treatment effect of ORDP on URII.
5.2. The dynamic treatment effect of ORDP on URII
The outcomes in only illustrate the average treatment effect of ORDP on URII. However, reducing URII is a long-term challenge for China and we should attach great importance to the dynamic treatment effect of ORDP on URII. Based on EquationEquation (2)(2)
(2) , we investigate the dynamic treatment effects of ORDP on URII, and shows the results.
Table 3. The dynamic treatment effect of ORDP on URII.
We can discover that the effect of ORDP on URII takes a period to emerge, which validates our Hypothesis 2. Specifically, the coefficients of and
are not significant, with or without including control variables, suggesting that the effectiveness of ORDP on URII is not significant in the current and first years when ORDP was implemented. However, the coefficients of
and
are significant and show a slight upward trend, indicating that the impact of ORDP on URII becomes more significant in the second and third years of ORDP implementation. The result presented above can be explained by the following reasons. On the other hand, the local government and businesses need time to react to the policies enacted in the ORDP, therefore it takes time for the program’s effects to become significant. On the other hand, ORDP achieves its policy effects mainly through massive fiscal investment in the construction of infrastructure or other physical capital in rural areas. These investments should also take time to play a positive role in reducing URII (Lin, Citation2019).
5.3. Robustness test
To enhance the accuracy of baseline results, we also conducted a number of robustness tests. Specifically, these involve a common trend test, a placebo test, a difference-in-difference method of propensity score matching (PSM-DID) estimation, excluding other policies’ impact, lagging control variables for one phase, replacing control variables, adjusting the sample time bandwidth, and winsorizing extreme values.
5.3.1. Common trend test
A precondition for applying the DID model to calculate the net effect of ORDP on URII is that URII in the control groups and experimental groups show a parallel trend before the ORDP implementation. Therefore, with reference to Beck et al. (Citation2010), we utilize the event method to examine whether the research samples satisfied the common trend. The equation is shown below:
(3)
(3)
Where denotes the time span before and after ORDP implementation.
represents the prior-ORDP county.
denotes the post- ORDP county. If the coefficients of
does not pass the significance test, which suggests the common trend hypothesis is fulfilled. The other variables are identical to EquationEquation (2)
(2)
(2) . We assume the sixth year before policy implementation (
) is the base period and we drop it to avoid interference from multicollinearity.
shows the test coefficients of parallel trends, while the vertical line means the 95% confidence interval. It reveals that the coefficients before the implementation of ORDP are not significant, which demonstrates that the pilot group and control group before ORDP are comparable and satisfy the common trend assumption.
5.3.2. Placebo test
To examine whether random probability will bias our baseline results, a placebo test was conducted by randomly sampling ORDP counties as the pseudo-treatment group based on the method by Pan et al. (Citation2022). More specifically, 250 counties selected randomly from the 613 counties were treated as the pseudo-ORDP counties, and simultaneously the remaining counties formed the control group. Subsequently, we regress the baseline DID model using the pseudo-sample and repeated the above generation process 1,000 times.
depicts the estimated coefficients for the pseudo-ORDP counties as well as the corresponding p-values. The results demonstrate that the regression coefficients are mostly adjacent to 0 and most p values were higher than 0.1, which is obviously different from the baseline estimated coefficient in . Therefore, we can conclude that the reduction of URII is indeed brought by ORDP.
5.3.3. PSM-DID estimation
To reduce the sample selection bias resulting from the non-randomness of the implementation of ORDP, the PSM-DID was employed for further robustness estimation referring to Qi et al. (Citation2021). Specifically, the first step of PSM-DID is using a logit regression method to calculate the propensity score for each county based on the four control variables stated in as covariates. Second, several methods, such as radius matching, kernel matching, and nearest-neighbor matching, were utilized to pair ORDP counties with non-ORDP counties. Eventually, the impacts of ORDP on URII are re-identified based on the matched sample using EquationEquation (1)(1)
(1) .
shows the PSM-DID estimation results acquired from different matching methods. We can find that the coefficients of ORDP under different matching methods are all significantly negative, which coordinates with the baseline results. The results indicate that ORDP is conducive to narrowing URII, which supports the robustness of our baseline findings.
Table 4. Results of the PSM-DID method.
5.3.4. Eliminating the impact of other policies on URII
Apart from ORDP, there may be other policies implemented during our study periods, such as Targeted Poverty Alleviation Policy (TPA) and National Rural E-commerce Comprehensive Demonstration Project (NRECDP). These policies will also affect URII and will skew our evaluation results. The reasons are as follows. First, the TPA policy can greatly enhance the income level of rural residents, which is conducive to narrowing URII to some extent (Li & Li, Citation2021; Wang et al., Citation2019). The year 2013 and 2020 witnessed the proposal of the TPA policy and the achievement of the TPA goals, respectively. Not only the implementation time of the TPA policy is close to ORDP but the pilot counties in TPA mostly coincide with the sample counties in ORDP. Second, the NRECDP can promote rural income and reduce URII. This is because NRECDP increases internet facilities and human capital for rural E-commerce by introducing E-commerce technologies into rural China (Li & Li, Citation2021; Peng et al., Citation2021). The NRECDP was gradually carried out nationwide from 2014 and its implementation time and pilot counties are overlapped with those of ORDP.
To enhance the accuracy of the estimated results, it is necessary to eliminate the interference of TPA and NRECDP policies. Following Zheng et al. (Citation2021), we construct two new models by adding these policies as dummy variables to the benchmark DID model:
Where is a dummy variable that is assigned a value of 1 in the years after county
has implemented the TPA policy and 0 otherwise.
is a dummy variable that is assigned a value of 1 in the years after the county
has carried out NRECDP policies and 0 otherwise. The remaining variables are identical to those in the benchmark model.
The results are displayed in Columns (1)-(2) of . We can discover that the coefficients of after the inclusion of the dummy variables of TPA and NRECDP policy are in line with the baseline model results, suggesting that the implementation of TPA and NRECDP policies does not interfere with the impact of ORDP, which further proves the reliability of our estimates.
Table 5. The results of several other robustness tests.
5.3.5. Lagging control variables for one phase
Considering that there may exist a reverse effect between the control variables and the establishment of ORDP, all control variables delayed for one period were induced to the regression to reduce the potential endogenous problems. The empirical results are displayed in Column (3) of . We can find that ’ coefficient equals −0.132 and remains significant, which verifies the robustness of our conclusion again.
5.3.6. Replacing control variables
Since ORDP was set up in batches, this paper replaced control variables with the interaction terms between control variables and years to take the potential trend of variables into account and enhance the persuasion of our previous conclusion. The results are presented in Column (4) of , which demonstrate that the effectiveness of ORDP on URII stays significant.
5.3.7. Adjusting the sample time-bandwidth
To eliminate the impact of the sample time span on baseline results, we modify the sample time bandwidth in accordance with Yang et al. (Citation2019). Considering the COVID-19 pandemic in 2019 may influence URII, we select 2010-2018 as a new time bandwidth. The results are presented in Column (5) of , which demonstrate that the coefficient of is still significantly negative in the new time interval, providing that ORDP effects are independent of the sample time bandwidth and confirming the validity of our baseline results.
5.3.8. Winsorizing extreme values
To ensure that policy effects are not affected by extreme values, referring to Pan et al. (Citation2022), we deleted the up and bottom 1% of the sample. The results in Column (6) of further prove the robustness of the previous conclusion because the regression results are consistent with the baseline model after eliminating the outliers.
5.4. Mechanism analysis
The previous empirical results have unveiled that the implementation of ORDP can significantly narrow URII. In this section, we turn to investigate the potential mechanisms of ORDP on URII. According to the analytical framework in Section 3, financial development and government intervention are two possible mechanisms. Referring to Baron and Kenny (Citation1986), we employ a three-step method to verify these two mechanisms. The specific model is as follows.
(6)
(6)
(7)
(7)
(8)
(8)
Where represents the two mediation variables: financial development and government intervention. Based on previous studies (Hong & Zhang, Citation2021), we use the ratio of balance of loans from financial institutions at the end of the year to GDP to calculate financial development and the natural logarithm of government fiscal expenditure to represent government intervention.
presents the total effect of ORDP on URII.
denotes the direct effect of ORDP on URII;
is an indirect effect of ORDP on URII. The following are the precise test procedures. First, we examine the significance of
and
If they are both significant and
has the same sign as
then it proves that the mediating effect test is passed. Second, if
or
is not significant, the Bootstrap test will be used to check if
If the result is significant and the sign of
coincides with the sign of
then the mediating effect also exists.
Column (1) in shows the total effect of ORDP on URII, and Columns (2)-(3) show the mechanism test results of government intervention. We can find that the coefficient of is 0.018 at the 5% level of significance, representing that ORDP has indeed increased government intervention. The coefficient of
is −0.044, which is also significant. The sign of
is negative, which coincides with
(-0.117). The above results demonstrate that government intervention exerts a mediating effect in the course of ORDP implementation. Secondly, the results of the financial development are shown in Columns (4)-(5). It can be found that the coefficient of financial development
is −0.084 at a 5% significance level. However, the coefficient of ORDP
(-0.009) is not significant. Thus, we further explore whether the mediating effect exists by applying the Bootstrap test. As displayed in , the indirect effect equals − 0.004 at a 1% significance level, verifying that financial development is also a mechanism.
Table 6. The mechanism analysis of ORDP on URII.
Table 7. The Bootstrap test for financial development on URII.
5.5. Heterogeneity analysis
In the above section, we have revealed the fact that ORDP exerts a significant influence on narrowing URII. In this part, we further investigate the possible heterogeneity effectiveness of ORDP on URII across counties located in different old revolutionary regions and economic development levels.
5.5.1. Heterogeneity effects across counties in different old revolutionary areas
ORDP covers 5 old revolutionary areas with more than 1,500 counties in 15 provinces across China. The economic basis and resource endowment of each old revolutionary area vary greatly, and there are significant differences in the formulation and implementation of specific support policies in each old revolutionary area, which may lead to regional heterogeneity in the implementation effect of ORDP on URII. Therefore, this paper further explores the regional heterogeneity effects of ORDP on URII. Investigating the heterogeneity effects of ORDP in different old revolutionary areas is helpful to distinguish between old revolutionary areas that facilitate the reduction of URII and others that do not, which can inform policy customization for different old revolutionary areas.
The results in demonstrate that the effects of ORDP on URII are significantly regionally heterogeneous. Specifically, the coefficients of ORDP in Shanxi-Gansu and Ningxia, Dabie Mountain, Left and Right Rivers, and Sichuan-Shanxi old revolutionary regions are significantly negative, indicating that ORDP decreases URII in these four old revolutionary regions located in western and central China, which is identical to the baseline results. However, the coefficients of ORDP in Jiangxi-Fujian and Guangdong old revolutionary region are significantly positive, revealing that ORDP increases URII in this old revolutionary region mainly located in the eastern China.
Table 8. Heterogeneity effects across different old revolutionary areas.
The possible reason for this result is that compared with the other four old revolutionary regions, Jiangxi-Fujian and Guangdong old revolutionary region allocated more public resources towards urban regions than rural regions, which may lead to an increase in urban income as well as a decrease in rural income and thus an increase in URII (Lu et al., Citation2022). We manually conducted a textual analysis of ORDP plans to verify this reason. We find that in Jiangxi-Fujian and Guangdong old revolutionary region, terms related to ‘rural’ such as ‘rural areas’, ‘agriculture’ and ‘rural residents’, appear 69 times, while terms related to ‘urban’ such as ‘city’, ‘urban areas’ and ‘urban residents’, appear 83 times, which is 1.20 times more than the former. In contrast, the frequency ratios of terms related to ‘urban’ to terms related to ‘rural’ in the other four old revolutionary regions are all less than 1, with an average of 0.66. That means the other four old revolutionary regions lay more emphasis on rural regions than urban regions. To test the validity of this explanation, we regress the natural logarithm of urban PCDI (lnurban) on ORDP and the natural logarithm of rural PCDI (lnrural) on ORDP for counties located in Jiangxi-Fujian and Guangdong old revolutionary region. The results in show that ORDP in Jiangxi-Fujian and Guangdong old revolutionary region indeed increases urban income, but decreases rural income, thus leading to an increase in URII, providing support for the above explanation.
Table 9. The effects of Jiangxi-Fujian-Guangdong ORDP on urban and rural income.
5.5.2. Heterogeneity effects across counties in different economic development levels
Chen and Lin (Citation2014) pointed out that China’s URII conforms to a U-curve trend in the process of economic growth, indicating that URII first declines and then rises with economic growth. Consequently, it makes sense to analyze the heterogeneity effects of ORDP on URII across different economic development. Specifically, we divide the sample counties into undeveloped counties and developed counties according to the median GDP. And then, we use the grouping regression method to investigate the influence of ORDP on URII under different economic development level samples. Simultaneously, we also use the difference-in-differences-in-differences (DDD) method by including the dummy variable of different economic development level multiplied by
to examine the heterogeneity effects again.
The results of Columns (1)-(2) in show that ORDP only exerts a significant effect in undeveloped counties. Moreover, the coefficient of is significantly negative (-0.205), similarly indicating that ORDP has a greater negative effect on URII in undeveloped counties compared with developed counties. The possible explanation for this result is ORDP specifically targets economically impoverished and geographically disadvantaged areas and thus has a greater impact on URII in undeveloped counties. For example, most financial investment in ORDP program are distributed in transportation improvement, infrastructure construction and industrial development in the undeveloped ORDP areas, providing important financial guarantees for the reduction of URII in the undeveloped ORDP areas (Tang & Sun, Citation2022). Moreover, financial investment in these undeveloped ORDP regions is especially crucial for rural residents to raise their income and thus decreasing URII, because it provide rural residents with more access to local and regional job markets (Lu et al., Citation2022).
Table 10. Heterogeneity effects across different economic development levels.
6. Conclusions and policy suggestions
To accurately identify the effectiveness of place-based policies on URII, we take advantage of ORDP as a natural experiment of place-based policies and apply a time-varying DID method to evaluate the effect of ORDP on URII. We also explore the potential mechanisms and heterogeneity effects. We find that: first, ORDP can result in an average of 11.2% decrease in URII, but this effect takes a period to emerge. A series of robustness tests prove that our results are trustworthy. Second, mechanism research reveals that ORDP decreases URII chiefly through government intervention and financial development. Third, heterogeneity analysis indicates that ORDP leads to a greater reduction in URII in mid-western regions and undeveloped counties compared with eastern regions and developed counties.
According to the above study, we offer relevant policy suggestions. First, the central government should vigorously support the implementation of ORDP to promote the reduction of URII and improve the well-being of residents in the old revolutionary regions. Second, the government should attach more importance to improving the income growth of rural residents, alleviating URII, and boosting human welfare when formulating specific measures in ORDP, rather than concentrating solely on fostering economic development in the revolutionary old regions. Our empirical outcomes show that ORDP causes a decline in URII, which is beneficial to high-quality development and enhances human welfare. Therefore, the local government in the old revolutionary regions should incorporate URII decline into their development priorities and give greater emphasis to human welfare advancement, thus achieving a ‘win-win’ outcome between URII reduction and economic growth. Third, governments should consider strengthening government intervention and promoting financial development as important measures to reduce URII in old revolutionary areas. Our mechanism analysis presents that ORDP mitigates URII chiefly through government intervention and financial development. Consequently, the governments in the old revolutionary areas should consider the following measures: increasing fiscal and financial support for the economy of counties in old revolutionary areas, supporting capital investment such as infrastructure construction in the targeted areas, expanding the breadth and depth of financial transfer payments, cultivating multi-level financial organizations and institutions in rural areas, developing rural businesses and rural industries, and strengthening rural labor training to enhance rural residents’ human capital. Forth, local authorities should implement ORDP persistently and simultaneously adjust some measures in the current ORDP in old revolutionary counties located in western and central China, which complies with our results in heterogeneity analysis. For example, for the governments in Jiangxi-Fujian-Guangdong’s old revolutionary areas, they should modify ORDP to skew more towards rural areas than urban areas since ORDP in these areas has significantly increased urban income, but reduced rural income in this area.
Disclosure statement
No conflict of interest exists in the submission of this manuscript. We declare that we do not have any commercial or associative interest that represents a conflict of interest in connection with the manuscript submitted.
Additional information
Funding
References
- Bai, Y., Zhang, L., Sun, M., & Xu, X. (2021). Status and path of intergenerational transmission of poverty in rural China: A human capital investment perspective. Journal of Integrative Agriculture, 20(4), 1080–1091. https://doi.org/10.1016/S2095-3119(20)63373-1
- Barbieri, E., Pollio, C., & Prota, F. (2020). The impacts of spatially targeted programmes: Evidence from Guangdong. Regional Studies, 54(3), 415–428. https://doi.org/10.1080/00343404.2019.1635688
- Baron, R. M., & Kenny, D. A. (1986). The moderator-mediator variable distinction in social psychological research: Conceptual, strategic, and statistical considerations. Journal of Personality and Social Psychology, 51(6), 1173–1182. https://doi.org/10.1037/0022-3514.51.6.1173
- Beck, T., Levine, R., & Levkov, A. (2010). Big bad banks? The winners and losers from bank deregulation in the United States. The Journal of Finance, 5, 1637–1667. https://doi.org/10.1111/j.1540-6261.2010.01589.x
- Chen, B., & Lin, Y. (2014). Development strategy, urbanization and the urban-rural income gap in China. Social Sciences in China, 35, 5–20. https://doi.org/10.1080/02529203.2013.875651
- Cheng, R., & Ma, L. (2022). On the urban-rural income gap from the perspective of human capital structure optimization: Based on provincial-level empirical evidence. Journal of Beijing Technology and Business University, 37, 113–126. ( In Chinese ). https://kns.cnki.net/kcms/detail/detail.aspx?FileName=BJSB202203010&DbName=CJFQ2022
- Falck, O., Koenen, J., & Lohse, T. (2019). Evaluating a place-based innovation policy: Evidence from the innovative Regional Growth Cores Program in East Germany. Regional Science and Urban Economics, 79, 103480. https://doi.org/10.1016/j.regsciurbeco.2019.103480
- Hao, Y., Chen, H., & Zhang, Q. (2016). Will income inequality affect environmental quality? Analysis based on China’s provincial panel data. Ecological Indicators, 67, 533–542. https://doi.org/10.1016/j.ecolind.2016.03.025
- Hong, M., & Zhang, W. (2021). Industrial structure upgrading, urbanization and urban-rural income disparity: Evidence from China. Applied Economics Letters, 28(15), 1321–1326. https://doi.org/10.1080/13504851.2020.1813244
- Hu, Z. (2021). The effect of income inequality on human capital inequality: Evidence from China. Structural Change and Economic Dynamics, 58, 471–489. https://doi.org/10.1016/j.strueco.2021.06.015
- Huang, Y., & Zhang, Y. (2020). Financial inclusion and urban–rural income inequality: Long-run and short-run relationships. Emerging Markets Finance and Trade, 56(2), 457–471. https://doi.org/10.1080/1540496X.2018.1562896
- Jha, C. K. (2019). Financial reforms and corruption: Evidence using GMM estimation. International Review of Economics & Finance, 62, 66–78. https://doi.org/10.1016/j.iref.2019.03.003
- Jha, C. K. (2020). Financial reforms and corruption: Which dimensions matter? International Review of Finance, 20, 515–527. https://doi.org/10.1111/irfi.12210
- Jia, J., Ma, G., Qin, C., & Wang, L. (2020). Place-based policies, state-led industrialisation, and regional development: Evidence from China’s Great Western Development Programme. European Economic Review, 123, 103398. https://doi.org/10.1016/j.euroecorev.2020.103398
- Jung, S. M., & Cha, H. E. (2021). Financial development and income inequality: Evidence from China. Journal of the Asia Pacific Economy, 26(1), 73–95. https://doi.org/10.1080/13547860.2020.1717301
- Keller, J., & Virág, T. (2022). A drop in the sea or catalyst for change: Diverse effects of the place-based approach in Europe. European Planning Studies, 30(5), 860–878. https://doi.org/10.1080/09654313.2021.1928047
- Kline, P., & Moretti, E. (2014). People, places, and public policy: Some simple welfare economics of local economic development programs. National Bureau of Economic Research, 6, 629–662. https://doi.org/10.3386/w19659
- Lassoued, M. (2021). Control of corruption, microfinance, and income inequality in MENA countries: Evidence from panel data. SN Business & Economics, 1(7), 1–19. https://doi.org/10.1007/s43546-021-00099-w
- Li, C., Lan, G., Zhang, G., Cheng, P., Shi, Y., & Gao, Y. (2022). Policy uncertainty, social responsibility and corporate M&A. Journal of Business & Industrial Marketing. https://doi.org/10.1108/JBIM-08-2021-0405
- Li, X., & Li, L. (2021). Evaluation of China’s targeted poverty alleviation policies: A decomposition analysis based on the poverty reduction effects. Sustainability, 13(21), 11691. https://doi.org/10.3390/su132111691
- Lin, S. C. (2019). Transport infrastructure and income inequality: Evidence from China’s province data. Mendeley Data.
- Lu, H., Liu, M., & Song, W. (2022). Place-based policies, government intervention, and regional innovation: Evidence from China’s Resource-Exhausted City program. Resources Policy, 75, 102438. https://doi.org/10.1016/j.resourpol.2021.102438
- Lu, H., Zhao, P., Hu, H., Zeng, L., Wu, K. S., & Lv, D. (2022). Transport infrastructure and urban-rural income disparity: A municipal-level analysis in China. Journal of Transport Geography, 99, 103292. https://doi.org/10.1016/j.jtrangeo.2022.103292
- Lu, Y., Wang, J., & Zhu, L. (2019). Place-based policies, creation, and agglomeration economies: Evidence from China’s economic zone program. American Economic Journal: Economic Policy, 11(3), 325–360. https://doi.org/10.1257/pol.20160272
- Pan, D., Hong, W., & He, M. (2022). Can campaign-style enforcement facilitate water pollution control? Learning from China’s Environmental Protection Interview. Journal of Environmental Management, 301, 113910. https://doi.org/10.1016/j.jenvman.2021.113910
- Pan, D., & Tang, J. (2021). The effects of heterogeneous environmental regulations on water pollution control: Quasi-natural experimental evidence from China. The Science of the Total Environment, 751, 141550. https://doi.org/10.1016/j.scitotenv.2020.141550
- Peng, C., Ma, B., & Zhang, C. (2021). Poverty alleviation through e-commerce: Village involvement and demonstration policies in rural China. Journal of Integrative Agriculture, 20(4), 998–1011. https://doi.org/10.1016/S2095-3119(20)63422-0
- Qi, S., Cheng, S., & Cui, J. (2021). Environmental and economic effects of China’s carbon market pilots: Empirical evidence based on a DID model. Journal of Cleaner Production, 279, 123720. https://doi.org/10.1016/j.jclepro.2020.123720
- Qin, M., Su, C., Zhong, Y., Song, Y., & Oana-Ramona, L. Ţ. (2022). Sustainable finance and renewable energy: Promoters of carbon neutrality in the United States. Journal of Environmental Management, 324, 116390. https://doi.org/10.1016/j.jenvman.2022.116390
- Ren, W., Xue, B., Yang, J., & Lu, C. (2020). Effects of the Northeast China revitalization strategy on regional economic growth and social development. Chinese Geographical Science, 30(5), 791–809. https://doi.org/10.1007/s11769-020-1149-5
- Su, C., Meng, X., Tao, R., & Umar, M. (2022a). Chinese consumer confidence: A catalyst for the outbound tourism expenditure? Tourism Economics, 0(0), 135481662110652. https://doi.org/10.1177/13548166211065250
- Su, C., Meng, X., Tao, R., & Umar, M. (2022b). Policy turmoil in China: A barrier for FDI flows? International Journal of Emerging Markets, 17(7), 1617–1634. https://doi.org/10.1108/IJOEM-03-2021-0314
- Su, C., Song, Y., Ma, Y., & Tao, R. (2019). Is financial development narrowing the urban-rural income gap? A cross-regional study of China. Papers in Regional Science, 98(4), 1779–1800. https://doi.org/10.1111/pirs.12428
- Sun, Z., Zhao, L., Wang, S., Zhang, H., Wang, X., & Wan, Z. (2021). Targeted poverty alleviation and households’ livelihood strategy in a relation-based society: Evidence from Northeast China. International Journal of Environmental Research and Public Health, 18(4), 1747. https://doi.org/10.3390/ijerph18041747
- Tang, L., & Sun, S. (2022). Fiscal incentives, financial support for agriculture, and urban-rural inequality. International Review of Financial Analysis, 80, 102057. https://doi.org/10.1016/j.irfa.2022.102057
- Wang, M., Yang, Y., Zhang, B., Liu, M., & Liu, Q. (2019). How does targeted poverty alleviation policy influence residents’ perceptions of rural living conditions? A study of 16 villages in Gansu Province, Northwest China. Sustainability, 11(24), 6944. https://doi.org/10.3390/su11246944
- Wang, S., Tan, S., Yang, S., Lin, Q., & Zhang, L. (2019). Urban-biased land development policy and the urban-rural income gap: Evidence from Hubei Province, China. Land Use Policy, 87, 104066. https://doi.org/10.1016/j.landusepol.2019.104066
- Wang, X., Shao, S., & Li, L. (2019). Agricultural inputs, urbanization, and urban-rural income disparity: Evidence from China. China Economic Review, 55, 67–84. https://doi.org/10.1016/j.chieco.2019.03.009
- Wang, Z., Wang, S., Wang, J., & Wang, Y. (2022). Development zones and urban economic performance in China: Direct impact and channel effects. Growth and Change, 53(4), 1762–1782. https://doi.org/10.1111/grow.12621
- Xue, Y., & Pan, X. (2021). The impact of agricultural mechanization on urban-rural income gap in China: An empirical analysis based on comprehensive FGLS estimation. Scientific and Social Research, 3(5), 7–11. https://doi.org/10.36922/ssr.v3i5.1201
- Yang, X., Lin, S., Li, Y., & He, M. (2019). Can high-speed rail reduce environmental pollution? Evidence from China. Journal of Cleaner Production, 239, 118135. https://doi.org/10.1016/j.jclepro.2019.118135
- Yang, Z., Shao, S., Xu, L., & Yang, L. (2022). Can regional development plans promote economic growth? City-level evidence from China. Socio-Economic Planning Sciences, 83, 101212. https://doi.org/10.1016/j.seps.2021.101212
- Yao, Y., & Jiang, L. (2021). Urbanization forces driving rural urban income disparity: Evidence from metropolitan areas in China. Journal of Cleaner Production, 312, 127748. https://doi.org/10.1016/j.jclepro.2021.127748
- Yuan, Y., Wang, M., Zhu, Y., Huang, X., & Xiong, X. (2020). Urbanization’s effects on the urban-rural income gap in China: A meta-regression analysis. Land Use Policy, 99, 104995. https://doi.org/10.1016/j.landusepol.2020.104995
- Zhang, H. (2020). Does the western development strategy reduce the rural-urban income disparity?—Evidence from regression discontinuity design. China Journal of Economics, 7, 162–193. https://kns.cnki.net/kcms/detail/detail.aspx?dbcode=CJFD&dbname=CJFDLAST2020&filename=TSJJ202002007&uniplatform=NZKPT&v=GfuQB4Kbrf5keXsLETjsf5RnRX178FMEEn-9jq8rh-M5ltQp1ymb58Akuw6pSiTL
- Zhang, J. (2021). Tourism and rural income inequality: Empirical evidence for China. Current Issues in Tourism, 12(14), 1–18. https://doi.org/10.1080/13683500.2021.2010674
- Zhang, J., & Zhang, Y. (2021). The relationship between China’s income inequality and transport infrastructure, economic growth, and carbon emissions. Growth and Change, 52(1), 243–264. https://doi.org/10.1111/grow.12472
- Zhang, M., & Zeng, L. (2020). Effect review and governance enlightenment of the state preference policy to the old revolutionary areas. Chinese Public Administration, 06, 92–96. In Chinese https://kns.cnki.net/kcms/detail/detail.aspx?FileName=ZXGL202006017&DbName=CJFQ2020
- Zhang, M., Zhang, G., & Liu, H. (2021). Analysis of the impact of tourism development on the urban-rural income gap: Evidence from 248 prefecture-level cities in China. Asia Pacific Journal of Tourism Research, 26(6), 614–625. https://doi.org/10.1080/10941665.2021.1886132
- Zhang, P., Li, W., Zhao, K., & Zhao, S. (2021). Spatial pattern and driving mechanism of urban–rural income gap in Gansu Province of China. Land, 10(10), 1002. https://doi.org/10.3390/land10101002
- Zhang, Q., & Chen, R. (2015). Financial development and income inequality in China: An application of SVAR approach. Procedia Computer Science, 55, 774–781. https://doi.org/10.1016/j.procs.2015.07.159
- Zheng, Q., Wan, L., Wang, S., Wang, C., & Fang, W. (2021). Does ecological compensation have a spillover effect on industrial structure upgrading? Evidence from China based on a multi-stage dynamic DID approach. Journal of Environmental Management, 294, 112934. https://doi.org/10.1016/j.jenvman.2021.112934
- Zhou, Q., & Li, Z. (2021). The impact of industrial structure upgrades on the urban–rural income gap: An empirical study based on China’s provincial panel data. Growth and Change, 52(3), 1761–1782. https://doi.org/10.1111/grow.12502
- Zolfaghari, M., Kabiri, M., & Saadatmanesh, H. (2020). Impact of socio-economic infrastructure investments on income inequality in Iran. Journal of Policy Modeling, 42(5), 1146–1168. https://doi.org/10.1016/j.jpolmod.2020.02.004