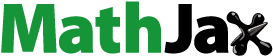
Abstract
Factor mismatch has seriously restricted the high-quality development of China’s economy. In order to solve the problem of unreasonable factor allocation in the Chinese economy, this paper takes the innovative pilot cities implemented by the Chinese government as a quasi-natural experiment to study its impact on innovation factor mismatch. The results show that: (1) the implementation of innovation-driven policies significantly improves the mismatch of innovation factors, which still holds after a series of robustness tests and endogeneity tests; (2) For non-state-owned enterprises, non-labor-intensive enterprises and the eastern region, the implementation of innovation-driven policies can better improve the mismatch of innovation factors. Furthermore, this paper finds that the implementation of innovation-driven policies can improve the mismatch index of high-skilled R&D personnel and the capital mismatch index of basic research and applied research. (3) Innovation-driven policies improve the mismatch of innovation factors by strengthening the flow of factors and promoting the upgrading of industrial structure.
1. Introduction
Since the reform and opening up, my country’s economy has developed rapidly by relying on the "extensive growth" model. However, this extensive growth model ignores the rational allocation of factors required for high-quality economic development, resulting in low efficiency of factor allocation in the process of economic development, and increasingly serious factor mismatches between regions, industries and departments. To this end, the 18th National Congress of the Communist Party of China proposed to take innovation as the basis for development, optimize the allocation of factors such as land, labor, and technology, and then correct the mismatch of factors. As the country’s top-level design reflects the innovative development concept, the innovative pilot city policy must be an innovation-driven model that shifts the direction of development to high-quality development, and then optimizes the allocation of innovation resources. However, this article cannot help but wonder, with the implementation of innovative urban policies for so long, how effective are innovative urban policies, and whether they can improve the mismatch of innovative elements? If the mismatch of innovation elements can be improved, what is the path to achieve it? Answering the above questions is of great significance to improving the high-quality development of my country’s economy under the conditions of the new era.
Scholars have studied the policy effects of innovative pilot city policies from different perspectives, mainly involving the study of innovation systems. For example, Li et al. (Citation2022) found that innovative city policy implementation significantly improves urban green innovation capacity. Zhang et al. (Citation2022) found that innovative cities can improve urban green progress by enhancing innovation investment and factor aggregation. Gao and Yuan (Citation2022) found that innovative city construction has a long-term positive effect on innovation performance in the surrounding area, and only a spillover effect exists. Zhang and Wang (Citation2022) found that innovative cities improve the flow of knowledge between industry, academia, and research in cities mainly through innovation funding support and the strengthening of innovation cooperation.
The existing literature on innovation factor mismatch focuses on the following four characteristics: The first is a distorted labor market (Micco & Repetto, Citation2012). The second is a distorted financial market: Financial friction can lead to distorted capital allocation among existing business units, and both episodic mismatch and endogenous mismatch are impactful (Moll, Citation2014). The third characteristic comprises irrational policies and institutional factors, as most studies on resource mismatch focus on specific policies or institutions that cause resource mismatch such as taxation, credit constraints, industrial policies, and so on, and in such studies, institutional and policy arrangements regarding the government are the main cause of resource mismatch (Brandt et al., Citation2013). The final focus is on the existence of information asymmetries related to industry productivity levels as well as distribution and related factors (David et al., Citation2016; Fuchs et al., Citation2016) that require potential heterogeneous firms to collect and prepay their fees before entering the market; the expenditure of such fees becomes a sunk cost that must be considered when firms need to make business strategy adjustments at a later stage, which will affect their entry and exit decisions, hinder the efficient flow of resources, and generate resource mismatch.
At present, the research on innovation-driven policies mainly focuses on the innovation system, but does not study the impact on factor mismatch. As for the research on innovation factor mismatch, although some scholars have studied its impact from the perspective of relevant policies (tax policy, industrial policy, credit policy, etc.), they have not studied the impact of innovation-driven policies on innovation factor mismatch. Based on the lack of existing literature and from the perspective of innovation-driven policies, this paper studies the net effect of innovation-driven policies on the mismatch of innovation factors using the method of difference and difference.
Therefore, the marginal contributions of this study are as follows. First, this study is the first to study the impact of innovation factor mismatch using the pilot policy of establishing innovative pilot cities, which provides evidence for promoting the construction of innovative pilot cities and enriches the literature related to innovative pilot cities and innovation factor mismatch. Second, based on a double-difference estimation model, this study obtains the net effect of innovative pilot city establishment by comparing the differences in innovation factor mismatch between firms in cities that implemented innovative pilot policies first, firms in cities that implemented innovative pilot policies later, and firms in non-pilot cities, with the help of differences in innovative pilot city policy across firms and different pilot times. Using this method, the endogeneity problem arising from omitted variables can be effectively avoided. Simultaneously, this study explores the impact mechanism of the establishment of innovative pilot cities on the allocation of innovation factors from several perspectives.
2. Theoretical mechanism and hypothesis formulation
According to the Ministry of Science and Technology issued by the "about to further advance the innovation of the pilot cities guidelines" (hereinafter referred to as "opinions"), an innovative pilot cities to speed up the construction of innovation system and improve the innovation environment, further increase investment in science and technology talent and technology, improve the research and development into a share of GDP, play the capital to reduce the unreasonable allocation of factors in the production of resource waste (Qin et al., Citation2022), then realize the reasonable allocation of resources. On the one hand, according to the general requirements of the Opinions, innovative cities should guide innovation factors to reasonably gather with enterprises. In this process, due to the entry of new technology or new factors, it will inevitably lead to the withdrawal of a number of factors that can not adapt to the development of enterprises, and this process will inevitably lead to the reasonable allocation of factors; On the other hand, innovative pilot cities should explore innovative development modes according to their own resource endowments and location factors, promote the interaction of innovation subjects and the flow of factors, and strengthen the radiation effect on other regions. Due to the interrelation and interdependence between the economic subjects in the urban system, industrial network layout is formed. The industrial network layout will further strengthen the sharing, learning and matching mechanism of the city (Duranton & Puga,Citation2004), and further promote the reasonable arrangement of innovation elements among different regions. Therefore, Hypothesis 1 was proposed:
H1: The establishment of innovative pilot cities has improved the mismatch of innovation factors
H2: Innovative pilot cities improve innovation factor mismatch by enhancing factor mobility
H3: Innovative pilot cities are established to improve the innovation factor mismatch through industrial structure upgrading.
3. Study design
3.1. Data sources and processing
Since the implementation of innovative pilot cities was in 2008, to ensure at least four years before the implementation of the policy, this study selected the sample data of listed companies in Shanghai and Shenzhen A-shares during 2002–2017 to study the impact of the establishment of innovative pilot cities on the allocation of innovation factors, and the required data came from the Guotaian, Wind, and China City Statistical Yearbooks. The data were processed through the following steps: (1) excluding listed companies in finance and insurance and those marked as ST-listed companies; (2) excluding listed companies with more missing data; and (3) excluding listed companies during the period of 2002–2017. Ultimately, 18,327 observations were obtained. In addition, all continuous variables in this study were deflated to the 1% level using the WINSORIZE method.
3.2. Variable selection
3.2.1. Explained variables
This study draws on Hsieh and Klenow (Citation2009) study and uses the R&D capital mismatch indexπki and R&D personnel mismatch indexπri to indicate the degree of innovation factor mismatch, which are calculated as follows:
(1)
(1)
In Equationequation (1)(1)
(1) , the πki and πri are the absolute price distortion factors for R&D, which are generally replaced by relative price distortion factors.
(2)
(2)
In Equationequation (2)(2)
(2) , the si is the innovation output share of region i, theβki andβLi are the output elasticities of the R&D capital and R&D personnel of region i, respectively. EquationEq. (2)
(2)
(2) reflects the ratio of the actual allocation of innovation factors in the region to the ideal situation, that is, the degree of mismatch; if the value is greater than 1, it indicates that the region is over-allocated with innovation factors and vice versa. To avoid the influence of inconsistent sign direction on the regression results, the larger the absolute value, the greater the degree of mismatch between innovation factors. Subsequently, to estimate the output elasticity β, this study assumes that the innovation production function is a C-D production function with constant returns to scale:
(3)
(3)
In Equationequation (3)(3)
(3) , Y is the innovation output, represented by the number of patents; Kit is the R&D capital output; and Lit is the R&D personnel input, represented by the full-time equivalent of R&D personnel.
3.2.2. Explanatory variables
The explanatory variables include the time dummy variable (post), grouping dummy variable (treat), and interaction term (post*treat). The time dummy variable (post) indicates the policy implementation period around 2008; the grouping dummy variable (treat) indicates whether the prefecture-level cities where the A-share listed enterprises in Shanghai and Shenzhen are located are the policy pilot areas; and the interaction term (post*treat) is the core explanatory variable of this study, which indicates the policy impact of the establishment of innovative pilot cities on innovation factor allocation.
3.2.3. Control variables
To avoid bias caused by omitted variables, other variables are also controlled for in this study: firm age (Age), expressed as the number of years since the firm went public; firm size (Size), expressed as the natural logarithm of total assets at the end of the current year; gearing ratio (Asro), expressed as the ratio of total liabilities to total assets; Tobin’s Q (Tb_Q), expressed as the ratio of firm market value to total assets (Tb_Q), expressed as the ratio of market capitalization to total assets; asset structure (Ajg), expressed as the ratio of net fixed assets to total assets; and growth capacity (Grow), expressed as the growth rate of the company’s operating income in the current year over the previous year.
3.3. Measurement model setting
where i denotes industry, t denotes time, CXYSCPit denotes the allocation of innovation factors, and treatit is the innovation pilot city dummy variable. When the listed company belongs to the 2008 innovation pilot city, treatit takes 1, and when it does not, it takes 0. Postit is the time dummy variable, which takes 0 before 2008 and 1 otherwise; and the interaction term treatit*postit. The coefficient of β3 reflects changes in the allocation of innovation factors of the enterprises before and after the implementation of the innovation pilot city policy in the pilot enterprises relative to the non-pilot enterprises. Xit denotes a series of control variables such as firm age, firm size, gearing ratio, Tobin’s Q, asset structure, and growth capacity. By adding individual firm-fixed effects to the double-difference model μi, the impact of firm-level unobservable factors that do not change over time in innovation pilot cities, and innovation factor allocation is controlled for by adding firm-level fixed effects to the double-difference model. By controlling for time-fixed effects, θt controls for the differences in innovation factor allocation due to time trends before and after the implementation of the 2008 innovation pilot city policy. εit is a random error term.
4. Analysis of the empirical results
4.1. Baseline regression results
Columns (1) and (2) of show the estimated results without adding control variables, and columns (3) and (4) show the treatment effects of policy implementation on the R&D capital mismatch index and R&D personnel mismatch index after adding control variables. From the coefficients and significance levels of the treatment variable Treat*post, it is clear that the establishment of innovative pilot cities significantly improves the R&D capital mismatch index and R&D personnel mismatch index, indicating that the establishment of innovative pilot cities significantly improves the innovation factor mismatch phenomenon. From columns (3) and (4), where both control variables are included and fixed effects are considered, it can be seen that the coefficients of Treat*post are 0.154 and 0.1657, respectively, and significant at the 1% level, indicating that after the implementation of innovative pilot cities, pilot companies’ R&D capital mismatch improved by 15.4% and R&D personnel mismatch improved by 16.57%. The above results verify hypothesis 1, that the establishment of innovative pilot cities improves the mismatch of innovation factors in enterprises.
Table 1. Policy treatment effect of innovation pilot city establishment on innovation factor mismatch.
4.2. Robustness test
In order to ensure the stability of the regression results, the following tests were also done in this paper.
4.2.1. Parallel trend test
The results in show that establishing innovative pilot cities improves the level of innovation factor mismatch. However, the prerequisite for using the double-difference model is that the experimental and control groups must have the same trend of change in innovation factor allocation before the implementation of the innovative pilot city policy; that is, the double-difference model is tested for parallel trends. To this end, drawing on the approach of Xuesong et al. (Citation2018), this study constructs the regression model of Equationequation (5)(5)
(5) .
(5)
(5)
Among them, treated*post2005, treated*post2004, and treated*post2003 denote the interaction terms in the three years before the implementation of the policy. Post2005 indicates that the value is 1 in 2005 and 0 in the other years; where post2004 indicates a value of 1 in 2004 and 0 in the other years. Post2003. If the coefficients α2,α3, and α4 are not significant, it means that they pass the parallel trend hypothesis test. The results in show that the treated*post2005, treated*post2004, and treated*post2003 coefficients are not significant, so it can be concluded that the mismatch of innovation factors in the experimental and control groups have the same change trends before the implementation of the innovative pilot city policy, thus satisfying the parallel trend hypothesis test.
Table 2. Parallel trend test.
4.2.2. Counterfactual test
In order to exclude other events before the implementation of the policy that led to the implementation of the innovative pilot city policy causing the mismatch of innovation factors to be improved. In this study, the implementation time of the innovation pilot cities was advanced by three years. If the coefficient of the interaction term treat*post is still significant, the improvement in innovation mismatch was not caused by the implementation of the innovation pilot city policy but by other events. Columns (1) and (2) of the results in show that the coefficient of the interaction term treat*post is 0.0924 and insignificant when the implementation of the policy is advanced to 2003, indicating that the improvement of the innovation factor mismatch was not influenced by other policies or events before the base year 2005, and the implementation of the innovation pilot city policy has no significant effect on the innovation factor mismatch in the experimental and control groups. There is no significant effect of the implementation of the innovation pilot city policy on the innovation factor mismatch in the experimental or control groups, which means that the improvement in the innovation factor mismatch is caused by the actual policy pilot year, and the previous empirical results are strongly robust.
Table 3. Counterfactual test.
4.2.3. Improving sample selection bias
In order to make the selective errors in the trends of pilot and non-pilot cities consistent and reduce the estimation bias of the double-difference method, this study further tests the data with the PSM-DID method. The specific steps are as follows: the dummy variable of whether it is a policy pilot city is logit regressed on the innovation factor mismatch indicator to obtain the PSM value, and the city with the closest PSM value can be used as the control group. In this paper, we adopt the “k-nearest neighbor matching (k = 4) method” based on Abadie et al. (Citation2004), and choose firm age (Age), firm size (Size), gearing (Asro), Tobin’s Q (Tb_Q), asset structure (Ajg), and growth capacity (Grow) as matched characteristic variables, resulting in a sample size of 10,656, including 1,747 in the experimental group and 8,909 in the control group. presents the regression results obtained using the double-difference method after the sample matching. The results show that the coefficient of the interaction term treated*post is significantly positive at the 1% level, which verifies that the implementation of the innovation pilot city policy has significantly improved the level of innovation factor mismatch in the pilot urban area; thus, the conclusions of this study are still robust.
Table 4. Changes to the sample selection bias.
4.2.4. Panel interaction fixed effects and urban fixed effects
Considering that the mismatch of innovation factors may be influenced by the characteristics of the city where a firm is located, to better reflect the improvement effect of innovation pilot city policy on the mismatch of innovation factors, this study introduces city characteristics factors for further robustness testing. This study introduces city-fixed effects based on model (1) for testing. The results are presented in . Column (1) of shows the R&D capital mismatch index, which controls for time and city-fixed effects, while column (2) of further controls for industry fixed effects. Both the interaction term Treat* post coefficient estimates in columns (1) and (2) of are significantly positive, indicating that the introduction of city-specific factors does not affect the regression results. Because some city characteristic factors may change with time trends, cross-fixed effects of time-fixed effects and city-fixed effects are introduced to control for city characteristic factors that change with time trends, and individual firm-fixed effects are introduced to control for the characteristic factors of the firm’s city. The results are shown in column (3) of , indicating that the interaction term Treat*post coefficient is positive but insignificant after controlling for time-varying city characteristic factors, verifying that the regression results of this study are robust.
Table 5. Robustness tests considering panel interaction-fixed effects and city-fixed effects.
4.2.5. Instrumental variables
In the above analysis, although we control the city fixed effect and time fixed effect to reduce the bias of the benchmark regression results, the problem of missing variables still exists, which causes the endogeneity problem of innovative pilot cities. For this reason, this paper draws on the practice of Zeng Jingjing and Zhou Danping (Citation2019), and uses the historical capital as an instrumental variable for regression. The construction of an innovative city emphasizes that urban development and cultural construction must be compatible with each other. The strong cultural heritage of the historical capital has an important impact on the cultural development of an innovative city, so the correlation hypothesis of the instrumental variable is satisfied. In addition, historical antiquity is determined by historical conditions, thus satisfying the exogenous assumption of instrumental variables. Therefore, the product of the historical ancient capital data and the time dummy variable is used as the instrumental variable of the innovative pilot city, and the least squares method is used for re-regression. The specific regression results are shown in . The results in show that the impact of innovative pilot cities on innovation factors is still statistically significant and positive, indicating that the regression results in this paper are robust.
Table 6. Instrumental variables.
4.2.6. Robustness test considering multi-time-point DID heterogeneity treatment effects
Baker et al. (Citation2022) found that when using a multi-time-point DID model for empirical analysis, there may be significant estimation bias due to the existence of "heterogeneous treatment effects". To this end, this paper draws on the practice of De Chaisemartin et al. (Citation2020) and uses the twowayfeweights command in the STATA software to test the possible "heterogeneity" of the model. If the result is closer to 1, it indicates that the heterogeneity test result is more robust, otherwise it is less robust. The results show that the result of the heterogeneity treatment effect is 1.32, which is relatively close to 1, thus indicating that the underlying regression results in this paper are robust.
4.2.7. Replace the explained variable
In the previous analysis, this paper uses the number of patent applications to represent innovation output. Since the number of invention patent applications can better reflect the innovation output, the number of invention patent applications is used to represent the innovation output. On the other hand, since the innovation output time requires a certain period of time, this paper adopts a one-period lag treatment for R&D personnel and R&D capital investment. The specific regression results are shown in , which further shows that the results are robust.
Table 7. Replace the explained variable.
4.3. Analysis of impact mechanism
4.3.1. Analysis of factor flow mechanism
Drawing on Anderson and Van Wincoop (Citation2003), a gravity model is used to measure regional innovation factor flows, where the expression for R&D capital flows is:
(6)
(6)
In the above equation, cflij denotes the amount of R&D capital flow from region i to region j. Ki and Kj are the R&D capital stock in regions i and j, respectively, and Rij is the geographical distance between regions i and j, which is obtained based on the latitude and longitude measurements of the two regional centers. Thus, the expression for the total amount of R&D capital in region i is
(7)
(7)
Unlike capital mobility, R&D personnel mobility is more likely to be influenced by economic, employment, and other social environment factors. Therefore, this study selected GDP per capita, average wage of employed urban workers per unit, average sales price of commodity houses, and number of R&D institutions to represent the above factors to reflect regional attractiveness based on the gravity model. Therefore, the representation of R&D personnel mobility is.
(8)
(8)
In the above equation, pflij denotes the flow of R&D personnel from region i to region j, Ni is the R&D personnel in region i, Gj is the GDP per capita of region j, Sj is the average wage in region j, Pj is the average house price in region j, and Ij is the number of R&D institutions in region j. Rij is the geographic distance between regions i and j, which is also measured based on the latitude and longitude of the two regional centers. Thus, the total R&D personnel flow in region I is expressed as
(9)
(9)
This study then draws on Wang et al. (Citation2023) to test, in the first step, whether the implementation of strategic policies in innovative pilot cities promotes the generation of matching opportunities; in the second step, it tests whether innovative pilot cities can promote the allocation of innovation factors in firms; and in the third step, matching opportunities are put into control variables and the interaction term treated*post is regressed for analysis. Therefore, the following model is constructed:
(10)
(10)
(11)
(11)
(12)
(12)
If the coefficient of Equationequation (10)(10)
(10) is significant, it indicates that the innovative pilot city policy has an impact on matching opportunities. If the coefficient of Equationequation (11)
(11)
(11) is significant, it indicates that the innovative pilot city strategy has an impact on the allocation of innovation factors of enterprises. If the interaction term in EquationEq. (12)
(12)
(12) is not significant, but the coefficient decreases, it indicates that the innovative pilot city strategy promotes enterprise innovation through the innovation environment. The regression results are presented in .
Table 8. Analysis of the mediating effects of factor flows.
Columns (1) to (3) and (4) to (6) of show the analysis of the mediating effects of the R&D capital mismatch index and R&D personnel mismatch index with factor mobility, respectively. The coefficient value of Treat*post in column (1) of is 0.5884, and both are significant, indicating that the strategic policy of innovative pilot cities promotes factor mobility. The regression results in column (2) show that the coefficient values of Treat*post are both 0.6207, indicating that the innovative pilot city strategy significantly improves the R&D capital mismatch phenomenon. The regression results in column (3) show that by placing factor mobility into the control variables, the core explanatory variable is significantly lower and the absolute value of the coefficient becomes smaller. This surface innovation pilot city improves the level of R&D capital mismatch among enterprises by enhancing factor mobility. For the same reason, columns (4) to (6) show that innovative pilot cities improve the level of R&D mismatch through factor mobility. This is mainly because the implementation of the policy will have a siphon effect, which will lead to the accumulation of innovative elements in the pilot cities, thus strengthening the flow of elements, and the flow of elements can enhance the exchange of information, and enterprises can learn from each other, strengthen communication, reduce the cost of enterprise search, and improve capital. mismatch level.
4.3.2. Analysis of industrial structure upgrading mechanism
Most of the current quantification of industrial structure upgrading has been conducted in terms of industrial structure rationalization and industrial structure advancement. To this end, this study draws on the study of Zhang et al. (Citation2020) to measure industrial structure rationalization using the Thiel index with the following formula:
(13)
(13)
where Yimt/Yit denotes the share of industry m in region i in the local GDP and Limt/Lit denotes the proportion of employed persons in industry m in region i to the total number of employed persons. The larger the value, the more irrational the industrial structure. The advanced industrial structure is expressed as the ratio of the output value of the tertiary industry to that of the secondary industry. The larger the ratio, the more advanced the industrial structure. The calculation results are listed in .
Table 9. Analysis of the mediating effect of the R&D capital mismatch index.
The coefficient values of Treat*post in column (1) of are 4.1e + 03 and significant, indicating that the strategic policy of innovative trial cities plays a facilitating role in the rationalization of industrial structure. The regression results in column (2) show that the coefficient values of Treat*post are both 0.1657, indicating that the innovative pilot city strategy significantly improves R&D capital mismatch. The regression results in column (3) surface show that the core explanatory variable was significantly lower, and the absolute value of the coefficient became smaller when industrial organization rationalization was included in the control variables. This surface innovation pilot city improves the current situation of R&D capital mismatch among enterprises by strengthening the rationalization of industrial structure. The results in columns (4), (5), and (6) of also indicate that the innovative pilot cities are improving the current situation of innovation R&D mismatch through an advanced industrial structure. Therefore, the results in suggest that innovative pilot cities improve the level of R&D capital mismatch through industrial structure upgrades. For the same reason, the regression results in show that innovative pilot cities improve the level of R&D staff mismatch by upgrading their industrial structure. Combining the results of and , we can see that innovative pilot cities improve the level of innovation factor mismatch by upgrading the industrial structure. So hypothesis 3 is proved.
Table 10. Analysis of the mediating effects of the R&D staff mismatch index.
4.4. Heterogeneity analysis
Based on this, to further investigate the mechanism of the operation of innovation pilot cities on innovation factor mismatch, this study further investigates whether there are differences in the implementation of innovation pilot cities on innovation factor mismatch in terms of firm ownership and industry factor intensity using a group regression method, starting from the perspective of firm ownership and industry factor intensity.
4.4.1. Heterogeneity analysis of business ownership
The results for the heterogeneity of the enterprise ownership regressions are presented in . The results in show that for SOEs, the coefficients of Treat*post for the R&D capital mismatch index and R&D personnel mismatch index are less than 0. This indicates that the implementation of the innovative pilot city policy does not improve the R&D capital mismatch index or the R&D personnel mismatch index of SOEs; in contrast, for non-SOEs, the coefficients of the R&D capital mismatch index and R&D personnel mismatch index are 0.2046 and 0.1491, respectively, and the coefficient values and significance levels are higher than those of SOEs, indicating that the implementation of the innovation pilot city policy has a greater impact on non-SOEs.
Table 11. Analysis of heterogeneity of enterprise ownership.
Specifically, for SOEs, because the actual controller is the government, the government can directly intervene in enterprise innovation factor allocation activities and decisions, and such behavior may lead to the mismatch phenomenon of the SOE innovation factors that cannot be improved but may intensify its mismatch phenomenon; for non-SOEs, the government can only indirectly regulate their allocation behavior through economic policies or the market; regulating factor allocation through market behavior is conducive to the reasonable allocation of innovation resources.
4.4.2. Heterogeneity analysis of industry factor intensity
Enterprises with different factor intensities have different R&D investment efforts, and the improvement in innovation factor mismatch is bound to differ. To this end, the sample of listed companies studied in this paper is divided into three categories according to the relative intensity of production factors: labor-, capital-, and technology-intensive firms.
As can be seen from , it can be seen that the regression coefficients of Treat*post for capital-intensive enterprises and technology-intensive enterprises are greater than 0, and both are significant at the 1% level, but the coefficients for labor-intensive enterprises are less than 0. This indicates that the establishment of innovative pilot cities does not improve the innovation resource mismatch phenomenon for labor-intensive enterprises, while the mismatch of resources between capital- and technology-intensive firms is significantly improved. This may be due to the fact that labor-intensive enterprises do not need innovation factors such as R&D capital and R&D personnel much, so such policy dividends do not flow to labor-intensive enterprises or have little impact on them after the national policy of innovative pilot cities is implemented, which is why the innovation factor mismatch phenomenon of labor-intensive enterprises is not improved.
Table 12. Heterogeneity analysis of industry factor intensity.
4.4.3. Regional heterogeneity analysis
As most innovation resources are concentrated in the eastern coastal region, it is necessary to consider whether the implementation of the innovative pilot city policy plays an ameliorating role in the regional innovation factor mismatch. To this end, 251 sample cities were divided into three major zones, namely, eastern, central, and western, according to the regional division criteria of the National Planning Commission, and regressions were conducted. The results in show that the establishment of innovative pilot cities has significantly improved the mismatch of innovation factors in the eastern region, while the mismatch of innovation factors in the central and western regions has not improved. This may be because in the eastern regions with a high degree of marketization, the market mechanism plays an absolute role in the process of resource allocation, mobilizes the enthusiasm of market transaction participants, promotes the flow of a variety of innovative resources, and prevents low-productivity enterprises from occupying more factors of production, thus contributing to the improvement of factor mismatch. In contrast, the central and western regions with a lower degree of marketization are unable to produce at the optimal factor scale, thus exacerbating the inhibiting effect of factor market distortion on resource allocation, which does not improve the innovation factor mismatch phenomenon and eventually leads to the above results.
Table 13. Regional heterogeneity analysis.
4.4.4. Analysis of mismatch types of innovation elements
In order to further examine the impact of innovative urban policies on the mismatch of different types of innovation factors, this paper divides the investment of R&D personnel into low-skilled (below undergraduate), medium-skilled (undergraduate and master) and high-skilled (doctoral) according to the educational level. R&D capital is divided into basic research, applied research and experimental development capital according to the type of input activities. The results in show that, on the one hand, the implementation of innovative city policies can significantly improve the mismatch index of high-skilled R&D personnel, mainly because high-skilled R&D personnel have strong innovation ability and high innovation success rate, and provide more benefits to high-skilled R&D personnel, will increase its enthusiasm, and high-skilled R&D personnel can better return the R&D investment. On the other hand, it can be found that the implementation of innovative urban policies can significantly improve the capital mismatch index of basic research and applied research.
Table 14. Analysis of mismatch types of innovation elements.
5. Conclusion and policy implications
This paper takes the innovation-oriented city policy implemented in China in 2008 as the natural experiment to test the influence of the establishment of innovation-oriented city on the mismatch of innovation factors. The results are as follows: the implementation of the innovation pilot city policy significantly improved the innovation factor resource mismatch phenomenon, and these results still hold after a series of robustness tests. The mechanism test indicates that innovative pilot cities improve the innovation factor mismatch phenomenon mainly through factor mobility and industrial structure upgrading. The heterogeneity results show that the establishment of innovative pilot cities significantly improves the mismatch of innovation factors for non-SOEs, but not for SOEs; the establishment of innovative pilot cities significantly improves the mismatch of innovation factors for capital-intensive and technology-intensive enterprises, but not for labor-intensive enterprises; the establishment of innovative pilot cities significantly improves the mismatch of innovation factors for in the eastern region, but not in the central and western regions;Innovative pilot cities are better able to improve the mismatch level of high-skilled R&D personnel and the level of innovation in basic and applied research capital.
The findings of this study provide the following policy insights for effectively promoting innovative pilot city policies and improving the mismatch between innovation factors.
First, innovative pilot city policies can significantly improve the innovation factor mismatch phenomenon, and this proves the appropriateness of the country in improving the innovation factor mismatch phenomenon with the help of the innovative pilot city policy. The implementation of this policy can achieve a win-win situation of high-quality economic development and high-end matching of factors. Therefore, the government should implement an innovative pilot city policy in a systematic manner and provide certain financial subsidies and tax benefits to regions with better implementation results, which can be used to motivate other regions to better realize the demonstrated effect of the policy.
Second, more emphasis should be placed on supporting non-state, capital and technology-intensive enterprises. Non-state enterprises are at a disadvantage compared to SOEs in terms of national “invisible” policies, which puts them under greater pressure of innovation costs and may divert too many of the resources originally used to improve the mismatch of innovation factors to other activities, which will inevitably affect the normal business activities of enterprises due to the excessive dispersion of resources. Therefore, the state can set up a special financial department that is mainly responsible for the approval of non-state enterprises to improve the mismatch of innovation factors resources. In addition, local governments should clarify the vein and structure of the central government’s innovation city policy implementation in detail, they should provide multiple support policies such as financial assistance and financial subsidies to capital and technology-intensive enterprises with a willingness to improve the mismatch of innovation factors.
Third, considering that factor flow and industrial structure upgrading are important ways for innovative pilot cities to improve the innovation factor mismatch, the path of innovative pilot cities should be optimized from the perspective of accelerating factor flow and promoting industrial structure upgrades to mobilize enterprises to improve innovation factor allocation. Enterprises should speed up the formation of innovation teams, introduce high-end innovation talents and increase innovation factor investment, and always adhere to the innovation-driven development strategy; in addition, they should also strengthen inter-regional exchanges and cooperation to promote the coordinated development of innovation factors.
Additional information
Funding
References
- Abadie, A., Drukker, D., Herr, J. L., & Imbens, G. W. (2004). Implementing matching estimators for average treatment effects in Stata. The Stata Journal: Promoting Communications on Statistics and Stata, 4(3), 290–311. https://doi.org/10.1177/1536867X0400400307
- Anderson, J. E., & Van Wincoop, E. (2003). Gravity with gravitas: A solution to the border puzzle. American Economic Review, 93(1), 170–192. https://doi.org/10.1257/000282803321455214
- Baker, A., Larcker, D. F., & Wang, C. C. Y. (2022). How much should we trust staggered difference-in-differences estimates. Journal of Financial Economics, 144(2), 370–395.
- Brandt, L., Tombe, T., & Zhu, X. (2013). Factor market distortions across time, space and sectors in China. Review of Economic Dynamics, 16(1), 39–58. https://doi.org/10.1016/j.red.2012.10.002
- Camagni, R., Capello, R., & Caragliu, A. (2017). Static vs. dynamic agglomeration economies: Spatial context and structural evolution behind urban growth. In Seminal studies in regional and urban economics (pp. 227–259). Springer.
- David, J. M., Hopenhayn, H. A., & Venkateswaran, V. (2016). Information, misallocation, and aggregate productivity. The Quarterly Journal of Economics, 131(2), 943–1005. https://doi.org/10.1093/qje/qjw006
- De Chaisemartin, C., & D'Haultfoeuille, X. (2020). Two-way fixed effects estimators with heterogeneous treatment effects. American Economic Review, 110(9), 2964–2996.
- Duranton, G., & Puga, D. (2004). Chapter 48 Micro-foundations of urban agglomeration economies. In Handbook of regional and urban economics (vol. 4, pp. 2063–2117). Elsevier. https://doi.org/10.1016/S1574-0080(04)80005-1
- Fuchs, W., Green, B., & Papanikolaou, D. (2016). Adverse selection, slow-moving capital, and misallocation. Journal of Financial Economics, 120(2), 286–308. https://doi.org/10.1016/j.jfineco.2016.01.001
- Gao, K., & Yuan, Y. (2022). Government intervention, spillover effect and urban innovation performance: Empirical evidence from national innovative city pilot policy in China. Technology in Society, 70, 102035. https://doi.org/10.1016/j.techsoc.2022.102035
- Hsieh, C. T., & Klenow, P. J. (2009). Misallocation and manufacturing TFP in China and India. Quarterly Journal of Economics, 124(4), 1403–1448. https://doi.org/10.1162/qjec.2009.124.4.1403
- Jingjing, Z., & Danping, Z. (2019). Regional characteristics, industrial structure and urban innovation performance: A quasi-natural experiment based on innovative city pilot. Review of Public Administration, 1(3), 66–97.
- Li, L., Li, M., Ma, S., Zheng, Y., & Pan, C. (2022). Does the construction of innovative cities promote urban green innovation? Journal of Environmental Management, 318, 115605.https://doi.org/10.1016/j.jenvman.2022.115605
- Li, J., & Yu, N. (2019). Decision-making under human capital mismatch: prioritizing innovation drive or industrial upgrading? Economic Research, 54(8), 152–166.
- Micco, A., & Repetto, A. (2012). Productivity, misallocation and the labor market. Documentos de Trabajo.
- Moll, B. (2014). Productivity losses from financial frictions: Can self-financing undo capital misallocation? American Economic Review, 104(10), 3186–3221. https://doi.org/10.1257/aer.104.10.3186
- Qin, M., Su, C.-W., Zhong, Y., Song, Y., & Lobonț, O.-R. (2022). Sustainable finance and renewable energy: Promoters of carbon neutrality in the United States[J]. Journal of Environmental Management, 324, 116390. https://doi.org/10.1016/j.jenvman.2022.116390
- Stone, C. J. (1985). Additive regression and other nonparametric models. The Annals of Statistics, 13(2), 689–705. https://doi.org/10.1214/aos/1176349548
- Su, C.-W., Pang, L.-D., Tao, R., Shao, X., & Umar, M. (2022). Renewable energy and technological innovation: Which one is the winner in promoting net-zero emissions?[J]. Technological Forecasting and Social Change, 182, 121798. https://doi.org/10.1016/j.techfore.2022.121798
- Sun, D., & Yijun, Y. (2019). Collaborative innovation and regional industrial upgrading from the perspective of spatial spillover. Statistical Research, 36(10), 100–114.
- Wang, M., Huang, Y., An, Z., et al. (2023). Reforming the world’s largest heating system: Quasi-experimental evidence from China. Energy Economics, 117, 106417.
- Xuesong, Q., Jin, K., Yinglun, T., & Xiaping, C. (2018). Industrial policy, capital allocation efficiency and total factor productivity of enterprises-an empirical study based on the natural experiment of China’s 2009 top ten industry revitalization plan. China Industrial Economics, 8, 42–59.
- Yigitcanlar, T., & Kamruzzaman, M. (2018). Does smart city policy lead to sustainability of cities? Land Use Policy, 73, 49–58. https://doi.org/10.1016/j.landusepol.2018.01.034
- Zhang, M., Hong, Y., & Zhu, B. (2022). Does national innovative city pilot policy promote green technology progress? Evidence from China. Journal of Cleaner Production, 363, 132461. https://doi.org/10.1016/j.jclepro.2022.132461
- Zhang, M., Sun, X., & Wang, W. (2020). Study on the effect of environmental regulations and industrial structure on haze pollution in China from the dual perspective The effect of environmental regulations and industrial structure on haze pollution in China from the dual perspective. Journal of Cleaner Production, 256, 120748. https://doi.org/10.1016/j.jclepro.2020.120748
- Zhang, S., & Wang, X. (2022). Does innovative city construction improve the industry-university-research knowledge flow in urban China? Technological Forecasting and Social Change, 174, 121200. https://doi.org/10.1016/j.techfore.2021.121200