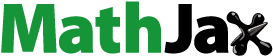
Abstract
The study investigates the time-varying correlation between El Niño phenomenon and agricultural commodity markets by employing the bootstrap full- and sub-sample rolling-window Granger causality tests. We find that the El Niño index (ENI) which measures the intensity of El Niño phenomenon, has both positive and negative impacts on agricultural commodity prices (ACP). The positive impact indicates that El Niño phenomenon may reduce the output of agriculture commodities, resulting in supply shortages and price increases. However, the negative impact suggests that affected by external uncertainties, ENI does not always cause ACP to change in the same direction. Conversely, we find ACP has negative impact on ENI, showing that agricultural commodity markets can reflect the extreme climate conditions globally in advance. The analyses can assist investors to adjust investment decisions and optimize asset allocation. Additionally, countries can improve the abnormal climate monitoring system to mitigate losses, and consider the impact of external uncertainties on agricultural commodity markets and ensure food security.
1. Introduction
The aim of the study is to analyze whether the El Niño phenomenon is really related to the agricultural commodity market. El Niño is a natural phenomenon, referring to the abnormal warming of the sea surface temperature in the central and eastern equatorial Pacific Ocean (Hove & Kambanje, Citation2019). It has a significant impact on regional climates across the globe, influencing seasonal temperatures and precipitation in many areas (Peri, Citation2017). While El Niño phenomenon brings heavy rains to south-eastern South America, western North America and eastern Africa, it creates droughts in Australia, and other Southeast Asian (Lefkovitz, Citation2013; Gutierrez, Citation2017). The global climate condition has changed due to the occurrence of El Niño, which significantly affects the agricultural commodity market with related effects on prices (Peri, Citation2015; Ubilava, Citation2018). This shows that agricultural commodity prices (ACP) will necessarily be sensitive to extreme weather shocks (Ubilava & Holt, Citation2013). On the one hand, the El Niño phenomenon adversely affects agricultural production, leading to a reduction in the supply of agricultural commodities, then driving up ACP (Marpaunga et al., Citation2019). More precisely, the El Niño phenomenon is usually linked with lower agricultural production growth rates and higher ACP (Salcedo et al., Citation2019). The drought-induced by El Niño phenomenon is also responsible for the majority of losses in agriculture production (Dawe et al., Citation2009; Gelcer et al., Citation2013), and it puts upward on ACP through supply shocks (Fajri et al., Citation2019). Besides, El Niño phenomenon brings other climatic conditions, including floods or hurricanes and tsunamis, which adversely influence agricultural production, resulting in a rise in ACP (Rojas et al., Citation2014; Abdolrahimi, Citation2016). On the other hand, the El Niño phenomenon maybe increase the supply of agricultural commodities and reduce its prices. For instance, rice production in most of the countries (comprising 38% of global production) is positively affected by the El Niño phenomenon (Villoria & Ubilava, Citation2012). The production of maize and sorghum has also increased during El Niño episodes in Pampas, Argentine (Podestá et al., Citation1999). Additionally, the El Niño phenomenon may increase the average global soybean production by 2.1-5.4% (Iizumi et al., Citation2014). We can conclude that the potential effect of climatic conditions on agricultural commodity markets is typically achieved through production shocks, which is a crucial link connecting El Niño phenomenon to ACP (Ubilava, Citation2018). Consequently, it can be expected that there will be different degrees and directions of correlation from the El Niño phenomenon to ACP. In turn, agricultural activities are directly or indirectly related to the occurrence of the El Niño phenomenon. Agriculture is a major source of greenhouse gas (GHG) emissions globally (Reay et al., Citation2012; Vetter et al., Citation2017; Tongwane & Moeletsi, Citation2018), which causes rising average sea level and increasing extreme climate events (Zou et al., Citation2015). With the increasing emissions of GHG, the occurrences of extreme climate, including drought, flood, heat wave and cold injury, are significantly frequent (Qin et al., Citation2023). Carbon emissions are slowing down, but the decarbonization process remains sluggish (Škare & Porada-Rochoń, Citation2023). Specifically, globalization has led to a constant evolution of the skills required for personal and professional development (Škare et al., Citation2022). The overall increase in global food demand, intensification of agricultural production with additions of more inputs of synthetic fertilizer, and expanding agricultural lands are the major factors causing GHG emissions (Tongwane & Moeletsi, Citation2018). Besides, these gas emissions may occur indirectly during the transportation of agricultural commodities (Wood & Cowie, Citation2004). The burning of fossil fuels emits GHG that contribute to climate change and global warming (Su et al., Citation2023a). Oil is a very important input in the transportation and production process of agricultural commodities (Zhang & Qu, Citation2015; Su et al., Citation2019b), but the consumption of oil can increase GHG emissions, resulting in global warming (Qin et al., Citation2020). Affected by these factors, the greenhouse effect can accelerate the rise in sea surface temperatures, leading to a significant increase in the frequency of El Niño phenomenon (Collins et al., Citation2010; Cai et al., Citation2018). Thus, there is a correlation between the El Niño phenomenon and agricultural commodity markets.
We apply the monthly data, covering the period from January 1997 to October 2022, to explore the interrelationship between the El Niño phenomenon and agricultural commodity markets by performing the full- and sub-sample tests. We find that the El Niño index (ENI), which measures the intensity of El Niño phenomenon, can significantly and positively affect ACP, this shows the El Niño phenomenon typically has an adverse impact on the output of agriculture commodities, thus resulting in a shortage of supply and price increases. However, this view can not be held by a negative impact from ENI to ACP. Affected by other external uncertainties, ENI does not always cause ACP to change in the same direction. In turn, ACP has a negative effect on ENI, suggesting that the role of agricultural markets in reflecting extreme global weather events cannot be ignored. The empirical results do not always support the Inter-temporal Capital Asset Pricing Model, which indicates that a positive influence from the El Niño phenomenon to ACP. In addition, these analyses provide revelations for the investor, they can grasp ACP changes in advance according to the El Niño phenomenon to conduct trading activities and avoid investment losses. The agricultural-related sector can understand the inter-linkages between the El Niño phenomenon and agricultural commodity markets, thereby reducing pollutant emissions while ensuring agricultural production. Also, our results offer implications for countries (especially for developing countries) and help them improve preventive climate risk management policies to cope with El Niño shocks to agricultural production and strengthen food security.
This paper makes several contributions. To begin with, the extant literature mostly investigates the positive impact of the El Niño phenomenon on ACP. The empirical evidence shows that El Niño phenomenon tends to reduce the production of agricultural commodities, and thus pushing up ACP. However, the El Niño phenomenon may also lead to an oversupply of agricultural production, which causes ACP to decline. Thus, there may be a causal relationship of different directions from El Niño phenomenon to ACP. This paper expands the literature by exploring the negative impact of the El Niño phenomenon on ACP. Secondly, the previous studies focus on a unidirectional causality between the El Niño phenomenon and ACP, but explorations of the mutual influence are relatively limited. On the contrary, this paper tries to analyze the inter-linkages between the two variables. Moreover, this study attempts to explore whether the El Niño phenomenon has an impact on the broader ACP from a global perspective, which distinguishes our analysis from previous studies. Thirdly, the causality between the El Niño phenomenon and ACP could be non-constant. The previous literature has not examined if a structural change could affect this causality. This paper identifies the non-constant interaction between the El Niño phenomenon and agricultural commodity markets by performing a sub-sample rolling-window causality test.
The paper has been arranged as follows:First, section 2 gives an overview of the relevant studies. We illustrate the Inter-temporal Capital Asset Pricing Model in section 3, and then reveal the causality test method in section 4. Next, section 5 presents the data used in the study. Section 6 explains our results. To conclude, section 7 provides conclusive remarks.
2. Literature review
There is substantial evidence of causal linkages among El Niño, agricultural production and commodity prices. Many studies support the positive effect of the El Niño phenomenon on ACP. Brunner (Citation2002) shows that global ACP is strongly affected by the El Niño phenomenon, with prices for food commodities and agricultural raw materials being influenced more than any other commodity. Ubilava (Citation2012) reveals that the El Niño phenomenon has a short-term positive impact on coffee prices. Ubilava and Holt (Citation2013) prove that the El Niño phenomenon leads to price increases in palm oil, soy oil and other vegetable oils. Rahman et al. (Citation2013) argue that during El Niño episodes, the supply of palm oil has reduced, causing its price to be on an upward trend. Iizumi et al. (Citation2014) show a significant adverse impact of El Niño phenomenon on the wheat yields in parts of China, the U.S., Australia, Mexico and parts of Europe, leading to an increase in wheat prices. Peri (Citation2015) highlights that corn and soybeans prices are affected by extreme climate events, and tend to increase with El Niño phenomenon. Regan (Citation2019) denotes that there is a positive significant relationship between the El Niño phenomenon and crop yield as well as its price. Cao et al. (Citation2023) show that El Niño reduced global-mean crop yield by 1.32%, 1.33%, and 0.37% for wheat, rice, and maize, respectively, but increased it for soybean by 1.9%. Cheng et al. (Citation2023) argue that food markets are more strongly affected in the case of extreme temperature anomalies. In contrast, several studies have confirmed that ACP is negatively affected by the El Niño phenomenon. Liao et al. (Citation2010) indicate that the El Niño phenomenon has a negative effect on soybean prices. Ubilava (Citation2017) asserts that wheat prices show a downward trend during the El Niño period. Bastianin et al. (Citation2018) hold that El Niño phenomenon may be conducive to production and reduce coffee prices. However, the causal link between El Niño phenomenon and ACP is not always supported. Letson and Mccullough (Citation2001) examine the relationship between soybean prices and the El Niño phenomenon, but no meaningful connection is found. Laosuthi and Selover (Citation2007) state that there is no causal relationship between the El Niño phenomenon and coffee prices. Oostdam (Citation2017) suggests that the prices of selected agricultural commodities (e.g. cocoa, coffee, rice, and soybeans) are not affected by the El Niño phenomenon.
Conversely, the causal effect of agricultural commodity markets on the El Niño phenomenon have expounded by certain studies. GHG is considered one of the primary sources of the rise in global warming, carbon dioxide (CO2) emissions constitute a large proportion of the GHG (Su et al., Citation2023b). The production and transportation of agricultural commodities contribute to GHG emissions, which can increase the sea surface temperature, and then trigger the El Niño phenomenon. Tilman et al. (Citation2011) show that agricultural activities, such as land clearing, soil cultivation, and the use of nitrogen fertilizer all emit GHG. Reay et al. (Citation2012) illustrate that agriculture has caused a huge perturbation to the global nitrogen cycle, and significantly increased nitrous oxide emissions. Nwani (Citation2017) points out oil consumption during the transportation of agricultural commodities also generates carbon dioxide, which contributes to GHG emissions. More importantly, the global climate has undergone significant changes due to the emission of GHG into the atmosphere (Tongwane et al., Citation2016). Collins et al. (Citation2010) highlight that the frequency of El Niño phenomenon changes with the acceleration of global warming. Subsequently, Cai et al. (Citation2014) indicate that affected by global warming, the El Niño phenomenon is likely to increase frequently. Salcedo et al. (Citation2019) denote that in recent years, strong El Niño phenomenon has occurred in the global warming context.
The impact of the El Niño phenomenon on ACP has been studied in the existing literature. However, they focus on a small number of agricultural commodities and certain areas thought to be significantly affected by the El Niño phenomenon. There is no specific time-series analysis exploring the clear causal impact of El Niño events on agricultural commodity markets from a global perceptive. Thus, we cannot obtain from these studies whether El Niño phenomenon has impacts for broader ACP. The study tries to fill this gap by exploring the impact of the El Niño phenomenon on global ACP. Furthermore, prior studies have mainly investigated the positive causal effect of the El Niño phenomenon on ACP, but the negative impact has been largely ignored. In general, El Niño phenomenon induced weather conditions can have different magnitudes of impact on regions across the globe. El Niño phenomenon can adversely affect agricultural production and result in an increase in ACP. Similarly, it may also lead to an oversupply of agricultural production and cause ACP to decline. There may be correlations of different directions from El Niño phenomenon to ACP. Besides, the existing literature may not consider structural mutations and ignore unstable parameters in Granger causality test, which is not possible to analyze time-varying interaction between El Niño phenomenon and ACP. Therefore, we apply the bootstrap sub-sample rolling-window test to improve the accuracy of the outcomes and obtain the interrelationship between the two variables.
3. The inter-temporal capital asset pricing model
Cifarelli and Paladino (Citation2010) investigate the effect of systemic risks on asset prices by constructing the Inter-temporal Capital Asset Pricing Model (ICAPM). In the study, we use the El Niño index (ENI) and ACP as surrogate variables, respectively. Firstly, we believe that agricultural commodity markets have two types of investment groups: informed traders (they usually conduct trading activities by measuring systematic risks and returns), and feedback traders (they take ACP in the previous period into account to invest). Secondly, we assume that the diversified investment in the agricultural commodity market cannot eliminate systemic risks, which can be represented by ENI that measures abnormal weather phenomenon. Informed traders tend to make reasonable predictions about ACP based on ENI, and will judge the number of agricultural commodities to invest. Thus, the demand for agricultural commodities of this group is expressed as follows:
(1)
(1)
where
denotes the percentage of agricultural commodities held by all informed traders during the time period of t.
represents that the value is positive, and
means that the rise in ENI will lead to an increase in
denotes the price of agricultural commodities when there is no El Niño. Besides, in the time period of t-1, the conditional expected value of ACP is expressed as
represents the ex-post ACP in t period. When
it indicates that there are only informed traders in the agricultural commodity market. Subsequently, we convert EquationEquation (1)
(1)
(1) into EquationEquation (2)
(2)
(2) , and find that a higher ENI will lead to an upward trend in ACP. This shows that the El Niño phenomenon really matters for the agricultural commodity market.
(2)
(2)
In addition, there is another type of investors in the agricultural commodity market who make current investment decisions according to the previous ACP. The percentage of these feedback traders’ demand () for agricultural commodities is represented by EquationEquation (3)
(3)
(3) :
(3)
(3)
where
states that when the change in previous ACP is positive (or negative), these traders may tend to increase (or decrease) the current investment in agricultural commodities. We observe that if there are two types of investors (informed traders and feedback traders) in the agricultural commodity market, then
Therefore, EquationEquation (1)
(1)
(1) will ultimately be expressed by EquationEquation (4)
(4)
(4) :
(4)
(4)
Compared to EquationEquation (2)(2)
(2) ,
is a new term, this shows that the agricultural commodity market is affected to a certain extent by the investment behaviour of feedback traders. The total coefficient of
is
and its value is positive due to
Thus, the ICAPM has proved that ENI can positively affect ACP. In the case of an increase in ENI, ACP will show an upward trend in order to hedge the systemic risk of El Niño phenomenon.
4. Methodology
4.1. Bootstrap full-sample causality test
In conventional vector autoregression (VAR) models, the statistic commonly used to test Granger causality, which can not follow the standard asymptotic distribution. In this condition, Shukur and Mantalos (Citation1997) indicate that the residual-based bootstrap (RB) method can improve the critical values, and thus, enhancing the accuracy of causality analysis. Additionally, they state that the RB technique is more suitable for testing the standard asymptotic distribution, even in a small sample. In particular, they have proved that small sample corrected likelihood ratio (LR) tests present relatively better power and size properties (Shukur & Mantalos, Citation2000). Thus, this study examines the causal link between ENI and ACP by adopting the RB based corrected-LR statistic. We consider the the following bivariate VAR (p) system in EquationEquation (5)(5)
(5) :
(5)
(5)
where p represents the known lag order, it can be determined according to the Schwarz Information Criterion (SIC). We divide Z into two sub-vectors (ENI and ACP), which can be expressed as
Besides, the El Niño phenomenon and ACP may have certain relationships with crude oil prices (COP), thus affecting the inter-linkages between these two variables. COP can influence the fluctuations of ACP through the input channel (Paris, Citation2018), and the continued rise in oil demand will cause the El Niño phenomenon (Qin et al., Citation2020). Thus, COP is selected as the control variable, and we rewrite EquationEquation (5)
(5)
(5) as follows:
(6)
(6)
where
is a white-noise process.
i = 1, 2, and j = 1, 2, 3, where L denotes the lag operator (
).
Accordingly, under the restriction of (k = 1, 2, ……, p), we can test the null hypothesis that ENI is not the Granger cause of ACP. Likewise, we also can examine the null hypothesis that ACP is not the Granger cause of ENI, in the constraint of
(k = 1, 2, ……, p). Based on this, if the first hypothesis can be rejected, it shows that ENI has significant causal impacts on ACP. Conversely, there is a significant causal influence of ACP on ENI when the second hypothesis is rejected.
4.2. Parameter stability test
One of the assumptions for the full-sample test in VAR models is that the parameters are constant, which is usually unrealistic. If the parameters change over time, this means that the full-sample test is unreliable. Thus, in order to overcome this problem, we examine parameter stability by using the Sup-F, Mean-F, and Exp-F tests (Andrews, Citation1993; Andrews & Ploberger, Citation1994). In the short term, the Sup-F test is able to check structural mutations in parameters, and the Mean-F and Exp-F tests are used to prove whether parameters change gradually over time. Additionally, we also apply the Lc statistics test, developed by Nyblom (Citation1989) and Hansen (Citation1992), which can test the parameters in the VAR models follow a random walk process or not. According to these stability tests, the interaction between ENI and ACP changes over time can be evidenced when the parameters are unstable. Therefore, we will employ the sub-sample test to explore the causal link between the two variables.
4.3. Sub-sample rolling-window causality test
This method can separate the full-time series into small samples according to the width of the rolling window (Balcilar et al., Citation2010). Choosing the appropriate width is complicated, and if it is small, the robustness of the results may not be guaranteed. A large one improves the accuracy of the results but reduces the number of scrolling. Therefore, when the parameter is not constant, it means that the selected width cannot be less than 20 (Pesaran & Timmermann, Citation2005). Then, we show the specific way: Let the range of the whole sample be H, and the width of the rolling window be i. The endpoint of each sub-sample is i, i + 1, ……, H, thereby getting the H-i + 1 sub-samples. Next, we obtain the sub-sample causal relationship test results. The causal influence from ENI to ACP can be presented by with Nb representing the frequency of bootstrap repetition. Likewise, the causal influence from ACP to ENI is measured by
Both
and
are bootstrap estimates from EquationEquation (6)
(6)
(6) . The 90% confidence intervals are applied in this paper, in which the lower and upper bound are the same as the 5th and 95th quantiles of
and
respectively (Balcilar et al., Citation2010).
5. Data
This paper is undertaken to examine the influence of El Niño phenomenon on agricultural commodity markets, selecting the monthly data from January 1997 to October 2022. The strong El Niño of 1997-1998 is dubbed ‘the climate event of the Twentieth Century’ (Changnon & Bell, Citation2000; Cai et al., Citation2014). This extreme El Niño phenomenon starts in April-May-June 1997 and lasts 12 months, with sea surface temperatures exceeding 28 °C, which disrupts the normal patterns of precipitation and atmospheric circulation. Due to the abnormal changes in the ocean-atmosphere circulation, extreme climatic conditions have emerged around the globe. The 1997–1998 El Niño brings torrential rainfalls and flooding to parts of California, the southeastern US, equatorial East Africa and Chile. It is also responsible for severe droughts in Papua New Guinea, Indonesia, Central America, and northeastern Brazil (McPhaden, Citation2001). Because of these droughts and floods, roughly 4% of the agricultural production area globally has been affected (Rojas et al., Citation2019), many agricultural commodities have experienced a greater poor harvest. The 1997-1998 El Niño has deteriorated agricultural production, which results in a shortage of supply, and thus increases ACP. Thus, we can conclude that there may be a significant impact of the El Niño phenomenon on the agricultural commodity market. We select the ENIFootnote1 to reflect the intensity of the El Niño phenomenon. A higher ENI indicates that a strong El Niño has occurred, and vice versa. And then, we choose agriculture price indexFootnote2 established by the International Monetary Fund (IMF) as the measurement of ACP, to reflect conditions in the global agricultural commodity market. This index uses 2016 as the base-year (average of 2016 = 100) and is a weighted average of prices for 20 primary commodities grouped into three main classes: food, beverages and agriculture raw materials. Besides, global COP has plummeted in 1997 due to insufficient demand. Since crude oil is a very important input in the transportation and production process of agricultural commodities, ACP is affected by COP fluctuations (Zhang & Qu, Citation2015). Therefore, we choose a COPFootnote3 to reflect the supply and demand situation of the oil market.
highlights the trends of ENI and ACP. It can be observed that ACP does not increase in all periods with high ENI. On average, the El Niño phenomenon will occur every 2-7 years and lasts 9-12 months each time (Shultz et al., Citation2014). The 1997-1998 El Niño adversely affects agricultural production, which has caused a reduction in the area of food crops harvested by about −6.4% (Irawan, Citation2002). Affected by a supply shortage, ACP presents an upward trend during this period. Similarly, the 2002-2003 El Niño induced drought has reduced agricultural production in the U.S., India, China and Australia, resulting in a slight rise in ACP. Furthermore, the occurrence of the El Niño phenomenon may have a continuously positive impact on ACP in a long-term process, which is reflected in the El Niño periods of 2006-2007 and 2009-2010. The abnormal climate caused by EI Niño in 2006-2007 leads to the global food crisis. The prices of major agricultural commodities (e.g. wheat, soybeans and corn) have surged unprecedentedly from 2007 to mid-2008, followed by a sharp decline due to insufficient demand during the global financial crisis (Su et al., Citation2019b). Subsequently, the moderate-intensity El Niño phenomenon has formed from late 2009 through early 2010. The grain-producing regions of the Midwestern U.S. have experienced high temperatures, resulting in a decrease in the production of corn, soybeans and other grains. The shortage of supply and the growing demand for agricultural commodities has caused ACP to rise rapidly from the second half of 2010 to April 2011. During 2015-2016, in the wake of one of the strongest El Niño phenomenon in recent history (Ubilava, Citation2018). However, ACP shows a downward trend, mainly due to the continued decline in global oil prices during this period. COP can influence the fluctuations in ACP (Mitchell, Citation2008) because crude oil is an important cost input in the production of agricultural commodities (Kumar, Citation2017). The moderate-intensity El Niño phenomenon occurs from September 2018 to July 2019 and severely affects rainfall and temperature patterns in many regions globally. Nevertheless, ACP has experienced a downward trend during this period. This is mainly because the global trade war launched by the U.S. has a significant negative impact on economic growth, thus reducing the demand for agricultural commodities, and ultimately leading to a decline in ACP. In 2020, a sudden coronavirus disease (COVID-19) pandemic unprecedentedly impacted the global economy. The most severe public health disaster in the past 100 years is rapidly evolving into a worldwide economic crisis (Su et al., Citation2022c). Meanwhile, ACP has risen sharply during this period. Therefore, we can conclude that the El Niño phenomenon does not always cause ACP to change in the same direction under the external shocks. Also, the interrelationship between ENI and ACP is complex and changes over time.
Figure 1. The trends of ENI and ACP.
Notes: We simply label certain important uncertainty events, due to space constraints.
Source: Authors’ Calculations.

The results of the descriptive statistics are presented in . The means of ENI, ACP and COP indicate that they are concentrated at the levels of −0.002, 90.616 and 55.954, respectively. Besides, the skewness of these three variables is positive. ENI has a kurtosis greater than 3, which means the sequence satisfies the leptokurtic distribution. Meanwhile, since the kurtosis is less than 3, ACP and COP satisfy the platykurtic distribution. Additionally, the Jarque-Bera index indicates that ENI, ACP and COP are non-normally distributed at the significance level of 1%. Thereby, it is not suitable to apply the conventional Granger causality test. Then, the RB method is used to solve the problem of the non-normal distribution of these three variables. The study also adopts the rolling-window method to analyze the causal inter-linkages between ENI and ACP.
Table 1. Descriptive statistics.
6. Empirical results
In the first stage, in order to determine whether the time series are stationary or not, we use the Augmented Dickey-Fuller (ADF) test (Dickey & Fuller, Citation1981), the Phillips-Perron (PP) test (Phillips & Perron, Citation1988) and the Kwiatkowski-Phillips-Schmidt-Shin (KPSS) test (Kwiatkowski et al., Citation1992). The corresponding results in clearly show that the ENI is stationary in level, while ACP and COP are I (1). Therefore, this paper adopts the original ENI, and the first-order difference of ACP and COP to establish the VAR model, and conducts Granger causality test accordingly.
Table 2. Unit root tests.
Based on EquationEquation (6)(6)
(6) , the bivariate VAR system with ENI and ACP is applied to test the full-sample causal relationship. According to SIC, the optimal lag order is chosen to be 3. Through the p-values in , we can conclude that there is no correlation between these two variables, which means that ENI is not affected by ACP, and vice versa. Besides, the test results are not supported by the extant literature (Peri, Citation2017; Ubilava, Citation2018; Salcedo et al., Citation2019), and are inconsistent with the ICAPM, which states that ENI positively affects ACP.
Table 3. Full-sample bootstrap Granger causality tests.
We perform a full-sample estimate in the bivariate VAR model, where assumptions are that the parameters are stable. Nevertheless, due to structural mutations in the VAR model, the parameters may not be constant, that is, the causal link between ENI and ACP can exhibit the time-variations characteristic. Then, following Andrews (Citation1993) and Andrews and Ploberger (Citation1994), the Sup-F, Mean-F and Exp-F tests are applied in this study to examine the stability of short-run parameters. Besides, the Lc statistics test (Nyblom, Citation1989; Hansen, Citation1992) is employed as well to make Granger causality tests more reliable. reports the corresponding results.
Table 4. Parameter stability tests.
ENI, ACP and the VAR system have structural mutations at the 1% significance level, which can be obtained from the Sup-F tests. We also observe from the Mean-F and Exp-F tests that parameters in ENI, ACP and the VAR system will gradually change over time at a significance level of 1%. Additionally, the Lc statistics test shows that the parameters in the VAR system cannot follow the random walk process at the level of 10%, which further proves that the parameters are non-constancy. Based on these stability tests, we find that due to structural mutations, the parameters exhibit unstable properties. Thus, the result of the causality analysis between ENI and ACP is inaccurate, that means that the bootstrap full-sample method does not apply to the study. Then, in order to identify the time-variations interrelationship between these two variables, we conduct the bootstrap sub-sample rolling-window causality test. In particular, 24-monthsFootnote4 is selected as the width of the rolling-window, which can make the results of the causal analysis more accurate. Meanwhile, we also obtain the direction of the impact of ENI on ACP (or the impact of ACP on ENI).
According to the p-value in , we can identify the degree and direction of the impact of ENI on ACP presented in . ENI does Granger cause ACP in these periods (January 2001-December 2001, June 2011-March 2012, November 2014 -September 2015 and October 2016-June 2017) at the significance level of 10%. The positive effect (January 2001-December 2001) and negative impacts (June 2011-March 2012, November 2014 -September 2015 and October 2016-June 2017) exist from ENI to ACP. ENI is at a relatively low level during the period of January 2001-December 2001, mitigating the adverse impact of abnormal climatic conditions on the global agricultural commodity market. ACP shows a downward trend under the influence of the weak El Niño phenomenon. In fact, the favourable weather condition has significantly increased the output or supply of some agricultural commodities. For instance, Brazil, the global largest producer, achieves a 10.7% rise in coffee production, which causes its price to fall to the lowest level in the past 8 years (Guo, Citation2002). From January to November 2001, the global output of tea has increased by 5.1% compared with the same period of the previous year, resulting in a significant price drop. However, because of the global economic slowdown and recession, especially the U.S. market demand is continuously in depression, causing the demand for agricultural commodities and its price to decline. Besides, the sluggish demand and abundant supply have increased competition in agricultural commodity markets. Due to the global increase in tea production in 2001, competition becomes more and more severe among India, Kenya, Sri Lanka, and Bangladesh. Also, Brazil, Argentina and the U.S. are increasingly competitive in soybean markets. All these factors have aggravated the decline in ACP to a varying degree. Therefore, we can prove the positive effect of ENI on ACP.
Figure 2. The bootstrap p-value of rolling test statistic that ENI is not the Granger cause of ACP.
Notes: The vertical axis represents the bootstrap p-value. The ‘dashed line’ legend indicates that the bootstrap p-value is equal to 0.1.
Source: Authors’ Calculations.

Figure 3. Bootstrap estimation of the sum of rolling coefficients of the influence from ENI to ACP.
Notes: The shaded areas indicate that ENI Granger causes ACP at the 10% significance level. We can identify the direction of the impact of ENI on ACP through the average bound for sum of coefficients. If the average bound is greater than 0, ENI has a positive effect on ACP and vice versa.
Source: Authors’ Calculations.

However, the view can not always be supported, which can be seen in the negative effect from ENI to ACP. El Niño phenomenon is relatively weak during the period of June 2011-March 2012, but it has caused severe droughts in important agricultural production areas (e.g. Southeast Asia, Eastern Europe, South Africa and Russia). The reduction in agricultural output is a direct factor leading to an upward trend in ACP. Meanwhile, affected by the acceleration of global biofuel production, the demand for agricultural commodities has risen sharply (Lucotte, Citation2016). The usage of biofuels not only pushes up the prices of agricultural raw materials used in bioenergy production but also puts upward pressure on the prices of other agricultural commodities (Su et al., Citation2019b). Accordingly, the increase in biofuel demand is one of the strong explanations for the rise in ACP (Czech, Citation2013). Over the same period, the rising COP accounts for another explanation for increasing ACP. The geopolitical events linked to oil-producing countries like Libya, Yemen again push the COP above $100 per barrel in 2011 and 2012 (Kumar, Citation2017). In general, higher oil prices push up the cost of fuel, fertilizers and other chemicals used in agricultural production (Gardebroek & Hernandez, Citation2013), thereby leading to an increase in ACP. Besides, with the easing of the European debt crisis in the second half of 2011, the global economy has gradually recovered. The growing demand for agricultural commodities in developing countries (e.g. China and India) has also led to an increase in ACP. These factors ultimately have caused ACP to be at a relatively high level. And then, the negative influence of ENI to ACP can be evidenced.
The sea surface temperature in the central-eastern equatorial Pacific is anomalously higher than normal from 2014 to 2015, triggering El Niño phenomenon (Li et al., Citation2015; Shao et al., Citation2016). Affected by the anomalous atmospheric circulation and the strong El Niño phenomenon, extreme climate conditions have occurred around the globe. Obviously, ENI exhibits an upward trend during the period of November 2014-September 2015, but there is a decline in ACP. The high temperature caused by the El Niño phenomenon may lead to a decline in demand for energy products (e.g. natural gas and crude oil), which are typically used as inputs for agricultural production (Ubilava, Citation2013). Over the same period, the global economic slowdown and excess oil production have contributed to a downward trend in COP (Su et al., Citation2019a). As a result, decreased input prices have inevitably led to a decline in ACP. In recent years, the ability to forecast the occurrence of El Niño phenomenon has improved, which is conducive to increasing the supply of agricultural commodities. Achieving social objectives requires public authorities’ efforts, involving a complex and pragmatic decision-making process (Dima et al., Citation2016). Furthermore, agricultural commodities are generally traded in U.S. dollars, and their appreciation contributes to the decrease in ACP (Czech, Citation2013). Since 2014, the Federal Reserve has continuously raised interest rates, and capital starts returning to the U.S. from emerging markets, leading to the continued strengthening of the dollar. Accordingly, ACP decreases during the period of high ENI, the negative impact can be proved.
Affected by the strong El Niño phenomenon of 2015-2016, Australia, Southeast Asia and Southern Africa have experienced high temperatures and severe droughts (Hu & Fedorov, Citation2019). The year 2016 is the warmest year on record (Salcedo et al., Citation2019), the temperatures of land and ocean are very high. Although El Niño phenomenon may adversely affect agricultural production in certain regions, global agricultural commodity supplies are generally abundant, and ACP exhibits a downward trend during the period of October 2016-June 2017. For instance, the price of palm oil has plummeted due to the surge in production in Malaysia and Indonesia, and Brazil has experienced a bumper harvest of sugar raw materials, which has caused sugar price to fall. The Russian Federation, Ukraine and Kazakhstan (RUK) regions are one of the important sources of global agricultural commodity supply, such as wheat and barley have accounted for 70% and 20% of grain exports in 2016, respectively. More importantly, the El Niño phenomenon does not adversely affect agricultural production in the RUK area (Rojas et al., Citation2019). Besides, the decrease in COP makes the cost of agricultural commodity production and transportation lower, and also suppresses the demand for biofuels in the market, thus reducing ACP (Burakov, Citation2016). Because biofuels are considered as alternatives to conventional fuels, and the low oil price has created downward pressure on the demand for biofuels (Su et al., Citation2019b). More importantly, the decline in biofuel production has reduced the demand and prices of agricultural commodities such as maize and soybeans. At the same time, the global economic downturn has led to a decline in demand for agricultural commodities. In particular, China’s supply-side structural reforms and the slowdown in economic growth have reduced the import demand for agricultural commodities, which has further caused ACP to drop. With the proposal of supply-side structural reform, the strategy of expanding domestic demand is crucial to economic development (Su et al., Citation2022a). Therefore, under the situation of weak aggregate demand, the oversupply of the global agricultural commodity market and the decline of COP, ACP shows a downward trend during the period of high ENI. These results do not always support the ICAPM, which states that ENI can positively affect ACP.
According to the p-value in , we can obtain the degree and direction of the impact of ACP on ENI shown in . ACP does Granger cause in these periods (November 2002-June 2003, October 2009-August 2010, June 2012-December 2013 and January 2019-June 2019) at the significance level of 10%. There are negative impacts of ACP on ENI during these periods. The growing global population has put pressure on agricultural production systems (Vetter et al., Citation2017). And then, the conversion of other land uses has increased agricultural land to meet the needs of more agricultural production globally (Tongwane et al., Citation2016). The output of agricultural commodities has increased due to the expansion of production, thereby causing ACP to decline during the period of November 2002-June 2003. However, agriculture activities are the major source of GHG emissions globally. For instance, nitrous oxide is an important anthropogenic GHG and agriculture represents its largest source (Reay et al., Citation2012). Changes in land use, irrigation and fertilizer application can lead to dynamic variations in soil carbon and nitrogen, and thus, increasing GHG emissions (Ogle et al., Citation2013). Global warming-induced by the GHG emission, which causes rising average sea level and increasing extreme climate events (Zou et al., Citation2015). Over the same period, this El Niño phenomenon begins in June 2002, lasts ten months and then gradually disappears in May 2003. The El Niño during 2002-2003 is classified as moderate in intensity, which means that ENI is at a relatively high level. Thus, we can prove the negative effect of ACP on ENI.
Figure 4. The bootstrap p-value of rolling test statistic that ACP is not the Granger cause of ENI.
Notes: The vertical axis represents the bootstrap p-value. The ‘dashed line’ legend indicates that the bootstrap p-value is equal to 0.1.
Source: Authors’ Calculations.

Figure 5. Bootstrap estimation of the sum of rolling coefficients of the influence from ACP to ENI.
Notes: The shaded areas indicate that ACP Granger causes ENI at the 10% significance level. We can identify the direction of the impact of ACP on ENI through the average bound for sum of coefficients. If the average bound is greater than 0, ACP has a positive effect on ENI and vice versa.
Source: Authors’ Calculations.

Affected by the outbreak and spread of the financial crisis, market demand is continuously in depression, and the global economy experiences a recession during the period of October 2009-August 2010. Agricultural commodities are closely related to the macro-economic situation because they are crucial inputs in the production process of many goods (Tule et al., Citation2019). The market demand for agricultural commodities has declined due to the global economic recession, which in turn causes ACP to fall. Meanwhile, under the shock of the global financial crisis, the price of oil has fallen sharply. Energy revolution, climate policies and significant events will enhance the linkage between renewable energy and others (Su et al., Citation2022b). There are mutual substitution effects between fossil fuels and clean energy, the decline in COP has reduced the demand for biofuels, resulting in lower demand and prices for agricultural commodities. The use of renewable resources is a good example of sustainable development (Chang & Fang, Citation2023). However, the reduction in the usage of bio-energy and the combustion of fossil energy produces more GHG emissions, which has accelerated the occurrence of the 2009-2010 El Niño. During this period, except for the substantial increase in temperature, the El Niño also causes other severe weather conditions including abnormal droughts in Asia and Africa (Qin et al., Citation2020). Thereby, we can evidence that ENI is at a higher level during the lower ACP.
From June to September 2012, the grain-producing regions of the U.S. have suffered the most severe drought since 1956, leading to a decline in the production of agricultural commodities such as soybeans, wheat, and corn. As the U.S. supplies nearly half the global corn and most of its soybean and wheat exports, the shortage of supply for agricultural commodities pushes ACP to a record high. Besides, the investor trading behaviour is a key determinant of various asset prices, including ACP (Zhou et al., Citation2019). Financial deepness enhances growth by improving the efficiency of capital (Gu et al., Citation2021). Financial speculative capital flows into agricultural commodity markets to gain benefits, which increases the demand for agricultural commodities and leads to a rise in ACP during the period of June 2012-December 2013. At the same time, geopolitical conflicts among oil-producing countries have drastically pushed up COP (Su et al., Citation2019b). Rising oil prices have increased the demand for biofuels, which has also caused the demand and prices for agricultural commodities to rise (Nemati, Citation2017). Nevertheless, ENI is at a relatively low level, which means that the El Niño phenomenon is weaker during this period. There are two ways to explain the low level of ENI. First, the increase in biofuel consumption has contributed to reducing GHG emissions. This is because the usage of clean energy can reduce environmental pollution, and slow down the process of global warming (Obama, Citation2017). Second, in recent years, sustainable agricultural development has been regarded as an important measure to mitigate the adverse impact of agricultural activities on climate change (Tongwane & Moeletsi, Citation2018). For instance, water used during agricultural irrigation can influence nitrogen dynamics in the soil and ultimately GHG emissions. At the global level, the balanced exploitation of agricultural water resources, and the coordinated use of surface and groundwater have played an important role in reducing GHG emissions and mitigating global warming (Zou et al., Citation2015). These factors may reduce the possibility of the occurrence of the El Niño phenomenon, and then make ENI lower. Accordingly, the negative impact of ACP on ENI can be proved.
The improvement of agricultural production efficiency has led to a significant increase in global agricultural commodities output, which in turn provides a relatively sufficient supply. During the period of January 2019-June 2019, the Sino-U.S. trade friction has further escalated, resulting in a substantial reduction in China’s demand for U.S. agricultural commodities, thus amplifying downside risk for global ACP. Besides, the trade disputes between the U.S. Trump administration and other countries (e.g. the European Union, Japan, and Mexico) have a significant negative impact on the global economy, which has led major international organizations to lower economic growth expectations. In the context of global trade disputes, an oversupply of agricultural commodities and slow economic growth have caused ACP to decline. However, the United Nations’ World Meteorological Organization (WMO) announces that a moderate-intensity El Niño has occurred from September 2018 to July 2019. This means that there is an increase in ENI, we can explain it from two sides. First, on average, the El Niño phenomenon will occur every 2-7 years and lasts 9-12 months each time (Shultz et al., Citation2014). The periodic anomalous change in atmospheric circulation is the direct factor for this 2018-2019 El Niño phenomenon, which has significantly affect rainfall and temperature patterns in many regions globally. Second, emissions of GHG can occur either directly during agricultural activities (eg., cultivation and harvesting), or indirectly during the production and transport of required inputs (e.g. herbicides, pesticides and fertilizers). These factors may be combined with long-term global warming to boost the sea surface temperature, which leads to the occurrence of the El Niño phenomenon, and ultimately makes the ENI higher during this period. Therefore, we can evidence the negative impact of ACP to ENI.
To summarize, the empirical results of this study prove that there is a two-way causal effect between the El Niño phenomenon and agricultural commodity markets. ENI can significantly and positively affect ACP, this shows the El Niño phenomenon usually has an adverse impact on the output of agriculture commodities, thus, resulting in a shortage of supply and price increases. However, the negative effect of ENI on ACP does not support this view in certain periods. This is because the technology to predict the occurrence of the El Niño phenomenon has been continuously improved, reducing the risk of extreme weather conditions on agricultural production. In addition, the high temperature-induced by El Niño phenomenon may reduce the demand and price of energy products, such as crude oil, which are used as inputs for agricultural production and transport. Accordingly, the decline in input prices has caused ACP to fall. These empirical results do not always support the ICAPM, which shows that ENI has positive effects on ACP. Conversely, ACP negatively affects ENI, suggesting that an agricultural commodity market is an effective tool that can reflect global extreme climate events in advance.
7. Conclusion
This study explores whether the El Niño phenomenon is really related to the agricultural commodity market, and analyzes the time-varying interrelationship between ENI and ACP by employing the sub-sample causality test. As a consequence, we have proved that there are bidirectional causality between ENI and ACP. The positive impact of ENI on ACP states that El Niño phenomenon may become strong sentiment issues, supporting ACP in an upward trend. Because traders believe that when El Niño occurs, the output of agricultural commodities is expected to decline and this, in turn, will increase ACP. However, this view can not be held by a negative impact, which can be explained in two ways. First, advanced global periodic climate forecasts have improved the ability of agricultural producers to respond to the El Niño phenomenon. They can be based on climate risk management to reduce the influence of adverse weather conditions, and take advantage of the favourable climate to expand agricultural production. Thus, the prediction of the El Niño phenomenon is conducive to stabilizing the global ACP. Second, combining ACP with other factors (e.g. oil prices, global economic situation and biofuel production), can provide a more comprehensive analysis of fluctuations in the agricultural commodity market. Affected by these external uncertainties, ENI does not always cause ACP to change in the same direction. These empirical results cannot always be supported by the ICAPM, which states that ENI can positively affect ACP. In turn, we find the negative impacts of ACP on INF, showing that the agricultural commodity market plays an important role in reflecting the global climate change (especially extreme weather conditions). Through the mutual influences between ENI and ACP, we conclude that the El Niño phenomenon can easily cause fluctuations in the agricultural commodity market, but it does not always cause ACP to rise. The agricultural commodity market is also related to other external factors, which together have impacts on ACP. Additionally, as a useful tool reflecting the occurrence of the El Niño phenomenon in advance, the role of the agricultural commodity market cannot be ignored.
Understanding the interaction between ENI and ACP and the role of El Niño phenomenon in agricultural commodity markets can offer implications for investors, agricultural-related sectors and governments. First, high ENI tends to increase ACP. Investors can grasp ACP changes in advance based on ENI, subsequently, they need to decide whether to invest in agricultural commodities, thereby achieving the purpose of reducing risk and maintaining wealth. Agricultural-related sectors need to mitigate the adverse effects of climate anomalies on crop production by strengthening food security management. In order to avoid a sharp rise in ACP caused by the El Niño phenomenon, governments must improve the environmental monitoring and forecasting system to effectively prevent agricultural production reduction and ensure an adequate supply. Second, under the external shocks, high ENI does not always cause ACP to rise. Both investors and governments need to understand the external uncertainties that are likely to affect agricultural commodity markets. El Niño phenomenon usually combines with other sides (e.g. oil prices, dollar value, global economic situation, and biofuel production) to have a negative impact on ACP. Thus, investors should optimize asset allocation to mitigate investment risks, and governments need to comprehensively consider these factors to maintain the stability of ACP. Third, ACP can negatively affect ENI. Due to the increase in population and the demand for food production, GHG emissions from agricultural activities continue to increase. This highlights the need for agricultural-related sectors to reduce GHG emission through sustainable development. For instance, the more efficient use of scarce water resources to reduce the damages to the ecological environment, and investment in improved varieties that can dramatically increase agricultural output. Additionally, the government should consider the fluctuation of ACP as a useful tool for climate risk management system, in order to provide more effective early warning for the occurrence of El Niño phenomenon. In the future study, we intend to explore which kind of agricultural price index (food, beverages and agriculture raw materials) is the most relevant to the El Niño phenomenon by applying the wavelet analysis.
Acknowledgment
This paper is funded by the China Scholarship Council.
Disclosure statement
No potential conflict of interest was reported by the authors.
Notes
1 The El Niño index can be taken from the U.S. National Weather Service.
2 The price of agricultural commodity is collected from IMF Financial Statistics.
3 The price of WTI crude oil is obtained from the U.S. Energy Information Administration.
4 Besides, we change the width to 20-, 28-, and 32- months, respectively, and conduct the robustness tests accordingly. These results are similar to the empirical analysis that rolling-window width is 24.
References
- Abdolrahimi, M. (2016). The effect of El Niño Southern Oscillation (ENSO) on world cereal production. Working paper. The University of Sydney.
- Andrews, D. W. K. (1993). Tests for parameter instability and structural Change with unknown change point. Econometrica, 61(4), 821–856. https://doi.org/10.2307/2951764
- Andrews, D. W. K., & Ploberger, W. (1994). Optimal tests when a nuisance parameter is present only under the alternative. Econometrica, 62(6), 1383–1414. https://doi.org/10.2307/2951753
- Balcilar, M., Ozdemir, Z. A., & Arslanturk, Y. (2010). Economic growth and energy consumption causal nexus viewed through a bootstrap rolling window. Energy Economics, 32(6), 1398–1410. https://doi.org/10.1016/j.eneco.2010.05.015
- Bastianin, A., Lanza, A., & Manera, M. (2018). Economic impacts of El Niño Southern Oscillation: Evidence from the Colombian coffee market. Agricultural Economics, 49(5), 623–633. https://doi.org/10.1111/agec.12447
- Brunner, A. D. (2002). El Niño and world primary commodity prices: Warm water or hot air? Review of Economics and Statistics, 84(1), 176–183. https://doi.org/10.1162/003465302317332008
- Burakov, D. (2016). Oil prices, exchange rate and prices for agricultural commodities: Empirical evidence from Russia. Agris on-Line Papers in Economics and Informatics, 08(02), 33–47. https://doi.org/10.7160/aol.2016.080203
- Cai, W., Borlace, S., Lengaigne, M., van Rensch, P., Collins, M., Vecchi, G., Timmermann, A., Santoso, A., McPhaden, M. J., Wu, L., England, M. H., Wang, G., Guilyardi, E., & Jin, F.-F. (2014). Increasing frequency of extreme El Niño events due to greenhouse warming. Nature Climate Change, 4(2), 111–116. https://doi.org/10.1038/nclimate2100
- Cai, W., Wang, G., Dewitte, B., Wu, L., Santoso, A., Takahashi, K., Yang, Y., Carréric, A., & McPhaden, M. J. (2018). Increased variability of eastern Pacific El Niño under greenhouse warming. Nature, 564(7735), 201–206. https://doi.org/10.1038/s41586-018-0776-9
- Cao, J., Zhang, Z., Tao, F., Chen, Y., Luo, X., & Xie, J. (2023). Forecasting global crop yields based on El Niño Southern Oscillation early signals. Agricultural Systems, 205, 103564. https://doi.org/10.1016/j.agsy.2022.103564
- Chang, C. L., & Fang, M. (2023). Impact of a sharing economy and green energy on achieving sustainable economic development: Evidence from a novel NARDL model. Journal of Innovation & Knowledge, 8(1), 100297. https://doi.org/10.1016/j.jik.2022.100297
- Changnon, S. A., & Bell, G. D. (2000). El Niño 1997-1998: The climate event of the century. Oxford University Press.
- Cheng, S., Li, X., & Cao, Y. (2023). Global evidence of the exposure-lag-response associations between temperature anomalies and food markets. Journal of Environmental Management, 325(Pt B), 116592. https://doi.org/10.1016/j.jenvman.2022.116592
- Cifarelli, G., & Paladino, G. (2010). Oil price dynamics and speculation: A multivariate financial approach. Energy Economics, 32(2), 363–372. https://doi.org/10.1016/j.eneco.2009.08.014
- Collins, M., An, S.-I., Cai, W., Ganachaud, A., Guilyardi, E., Jin, F.-F., Jochum, M., Lengaigne, M., Power, S., Timmermann, A., Vecchi, G., & Wittenberg, A. (2010). The impact of global warming on the tropical Pacific Ocean and El Niño. Nature Geoscience, 3(6), 391–397. https://doi.org/10.1038/ngeo868
- Czech, K. (2013). Speculation in the agricultural commodity market. Problems of World Agriculture, 13, 10–16.
- Dawe, D., Moya, P., & Valencia, S. (2009). Institutional, policy and farmer responses to drought: El Niño events and rice in the Philippines. Disasters, 33(2), 291–307. https://doi.org/10.1111/j.1467-7717.2008.01075.x
- Dickey, D. A., & Fuller, W. A. (1981). Likelihood ratio statistics for autoregressive time series with a unit root. Econometrica, 49(4), 1057–1072. https://doi.org/10.2307/1912517
- Dima, B., Lobonţ, O.-R., & Nicoleta-Claudia, N. (2016). Does the quality of public policies and institutions matter for entrepreneurial activity? Evidences from the European Union’s Member States. Panoeconomicus, 63(4), 425–439. https://doi.org/10.2298/PAN1604425D
- Fajri, H. C., Siregar, H., & Sahara, S. (2019). Impact of climate change on food price in the affected provinces of El Niño and La Niña phenomenon: Case of Indonesia. International Journal of Food and Agricultural Economics, 7(4), 329–339.
- Gardebroek, C., & Hernandez, M. A. (2013). Do energy prices stimulate food price volatility? Examining volatility transmission between US oil, ethanol and corn markets. Energy Economics, 40, 119–129. https://doi.org/10.1016/j.eneco.2013.06.013
- Gelcer, E., Fraisse, C. W., Dzotsi, K. A., Hu, Z., Mendes, R., & Zotarelli, L. (2013). Effects of El Niño Southern Oscillation on the space-time variability of Agricultural Reference Index for Drought in midlatitudes. Agricultural and Forest Meteorology, 174–175, 110–128. https://doi.org/10.1016/j.agrformet.2013.02.006
- Gu, J., Gouliamos, K., Lobonţ, O.-R., & Nicoleta-Claudia, M. (2021). Is the fourth industrial revolution transforming the relationship between financial development and its determinants in emerging economies? Technological Forecasting and Social Change, 165, 120563. https://doi.org/10.1016/j.techfore.2020.120563
- Guo, P. X. (2002). Review of the global agricultural commodities market in 2001 and prospect in 2002. International Agricultural Trade, 1, 8–11.
- Gutierrez, L. (2017). Impacts of El Niño Southern Oscillation on the wheat market: A global dynamic analysis. Plos One, 12(6), e0179086. https://doi.org/10.1371/journal.pone.0179086
- Hansen, B. E. (1992). Tests for parameter instability in regressions with I (1) processes. Journal of Business & Economic Statistics, 10(3), 321–336. https://doi.org/10.2307/1391545
- Hove, L., & Kambanje, C. (2019). Lessons from the El Niño-induced 2015/16 drought in the Southern Africa region. Current Directions in Water Scarcity Research, 2, 33–54.
- Hu, S., & Fedorov, A. V. (2019). The extreme El Niño of 2015-2016: The Role of westerly and easterly wind bursts, and preconditioning by the failed 2014 event. Climate Dynamics, 52(12), 7339–7357. https://doi.org/10.1007/s00382-017-3531-2
- Iizumi, T., Luo, J.-J., Challinor, A. J., Sakurai, G., Yokozawa, M., Sakuma, H., Brown, M. E., & Yamagata, T. (2014). Impacts of El Niño Southern Oscillation on the global yields of major crops. Nature Communications, 5(1), 1–7. https://doi.org/10.1038/ncomms4712
- Irawan, B. (2002). Stabilization of upland agriculture under El Niño-induced climatic risk: Impact assessment and mitigation measures in Indonesia. Working paper. The CGPRT Centre.
- Kumar, D. (2017). On volatility transmission from crude oil to agricultural commodities. Theoretical Economics Letters, 07(02), 87–101. https://doi.org/10.4236/tel.2017.72009
- Kwiatkowski, D., Phillips, P. C. B., Schmidt, P., & Shin, Y. (1992). Testing the null hypothesis of stationarity against the alternative of a unit root: How sure are we that economic time series have a unit root. Journal of Econometrics, 54(1–3), 159–178. https://doi.org/10.1016/0304-4076(92)90104-Y
- Laosuthi, T., & Selover, D. D. (2007). Does El Niño affect business cycles. Eastern Economic Journal, 33(1), 21–42. https://doi.org/10.1057/eej.2007.2
- Lefkovitz, Y. (2013). Climate and commodity prices: An analysis of the role of ENSO forecasts in agricultural commodity markets. Working paper. New York University.
- Letson, D., & Mccullough, B. D. (2001). ENSO and soybean prices: Correlation without causality. Journal of Agricultural and Applied Economics, 33(3), 513–521. https://doi.org/10.1017/S1074070800020976
- Li, Q. Q., Wang, A. Q., Zhou, B., & Wang, P. L. (2015). Global major weather and climate events in 2014 and the possible causes. Meteorological Monthly, 41(4), 497–507.
- Liao, S. Y., Chen, S. T., & Chen, C. C. (2010). Modelling the ENSO forecast on soybean spot and future prices. Journal of Agricultural & Fore, 59(2), 91–103.
- Lucotte, Y. (2016). Co-movements between crude oil and food prices: A post-commodity boom perspective. Economics Letters, 147, 142–147. https://doi.org/10.1016/j.econlet.2016.08.032
- Marpaunga, B., Siregarb, H., & Anggraeni, L. (2019). Analysis of El Niño impact and the price of food commodities on inflation. Journal Economize Indonesia, 8(1), 21–35.
- McPhaden, M. J. (2001). El Niño and La Niña: Causes and global consequences. The Earth System: Physical and Chemical Dimensions of Global Environmental Change, 1, 353–370.
- Mitchell, D. (2008). A note on rising food prices. Policy Research Working Paper No. 4682. World Bank.
- Nemati, M. (2017). Relationship among energy, bio-energy, and agricultural commodity prices: Re-considering structural changes. International Journal of Food and Agricultural Economics, 5(3), 1–8.
- Newey, W. K., & West, K. D. (1987). A simple, positive semi-definite, heteroskedasticity: An autocorrelation consistent covariance matrix. Econometrica, 55(3), 703–708. https://doi.org/10.2307/1913610
- Nwani, C. (2017). Causal relationship between crude oil price, energy consumption and carbon dioxide (CO2) emissions in Ecuador. OPEC Energy Review, 41(3), 201–225. https://doi.org/10.1111/opec.12102
- Nyblom, J. (1989). Testing for the constancy of parameters over time. Journal of the American Statistical Association, 84(405), 223–230. https://doi.org/10.1080/01621459.1989.10478759
- Obama, B. (2017). The irreversible momentum of clean energy. Science (New York, N.Y.), 355(6321), 126–129. https://doi.org/10.1126/science.aam6284
- Ogle, S. M., Buendia, L., Butterbach-Bahl, K., Breidt, F. J., Hartman, M., Yagi, K., Nayamuth, R., Spencer, S., Wirth, T., & Smith, P. (2013). Advancing national greenhouse gas inventories for agriculture in developing countries: Improving activity data, emission factors and software technology. Environmental Research Letters, 8(1), 015030. https://doi.org/10.1088/1748-9326/8/1/015030
- Oostdam, S. (2017). The influence of the El Niño proxy-the ‘Southern Oscillation Index’-on food commodity prices. Working paper. Erasmus University Rotterdam.
- Paris, A. (2018). On the link between oil and agricultural commodity prices: Do biofuels matter? International Economics, 155, 48–60. https://doi.org/10.1016/j.inteco.2017.12.003
- Peri, M. (2017). Climate variability and the volatility of global maize and soybean prices. Food Security, 9(4), 673–683. https://doi.org/10.1007/s12571-017-0702-2
- Peri, M. (2015). Climate variability and agricultural price volatility: The case of corn and soybeans [Paper presentation]. Conference Paper, 29th International Conference of Agricultural Economists, Milan, Italy.
- Pesaran, M. H., & Timmermann, A. (2005). Small sample properties of forecasts from autoregressive models under structural breaks. Journal of Econometrics, 129(1–2), 183–217. https://doi.org/10.1016/j.jeconom.2004.09.007
- Phillips, P. C. B., & Perron, P. (1988). Testing for a unit root in time series regression. Biometrika, 75(2), 335–346. https://doi.org/10.1093/biomet/75.2.335
- Podestá, G. P., Messina, C. D., Grondona, M. O., & Magrin, G. O. (1999). Associations between grain crop yields in central-eastern Argentina and El Niño-Southern Oscillation. Journal of Applied Meteorology, 38(10), 1488–1498. https://doi.org/10.1175/1520-0450(1999)038<1488:ABGCYI>2.0.CO;2
- Qin, M., Qiu, L. H., Tao, R., Umar, M., Su, C. W., & Jiao, W. (2020). The inevitable role of El Niño: A fresh insight into the oil market. Economic Research-Ekonomska Istraživanja, 33(1), 1943–1962. https://doi.org/10.1080/1331677X.2020.1768428
- Qin, M., Su, C. W., Umar, M., Lobonţ, O. R., & Manta, A. G. (2023). Are climate and geopolitics the challenges to sustainable development? Novel evidence from the global supply chain. Economic Analysis and Policy, 77, 748–763. https://doi.org/10.1016/j.eap.2023.01.002
- Rahman, A. K. A., Abdullah, R., Nambiappan, B., & Shariff, F. M. (2013). The impact of La Niña and El Niño events on crude palm oil prices: An econometric analysis. Oil Palm Industry Economic Journal, 13(2), 38–52.
- Reay, D., Davidson, E. A., Smith, K. A., Smith, P., Melillo, J. M., Dentener, F., & Crutzen, P. J. (2012). Global agriculture and nitrous oxide emissions. Nature Climate Change, 2(6), 410–416. https://doi.org/10.1038/nclimate1458
- Regan, S. (2019). A look at the relationship between El Niño-southern oscillation and agricultural commodities in the United States. Working paper. Utica College.
- Rojas, O., Li, Y., & Cumani, R. (2014). Understanding the drought impact of El Niño on the global agricultural areas: An assessment using FAO’s Agricultural Stress Index (ASI). Food and Agriculture Organization of the United Nations.
- Rojas, O., Piersante, A., Cumani, M., & Li, Y. (2019). Understanding the drought impact of El Niño/La Niña in the grain production areas in Eastern Europe and Central Asia. World Bank Publications.
- Salcedo, D. S. A., Melo-Velandia, L. F., & Parra-Amado, D. (2019). Nonlinear relationship between the weather phenomenon El Niño and Colombian food prices. Working Paper, Borradores de Economia 1085.
- Schwarz, G. (1978). Estimating the dimension of a model. Annuals of Statistics, 6, 461–464.
- Shao, X., Liao, Y. M., Liu, Y. J., & Nie, Y. (2016). Global major weather and climate events in 2015 and the possible causes. Meteorological Monthly, 42(4), 489–495.
- Shukur, G., & Mantalos, P. (1997). Size and power of the RESET test as applied to systems of equations: A bootstrap approach. Working Paper. Department of Statistics, University of Lund.
- Shukur, G., & Mantalos, P. (2000). A simple investigation of the Granger-causality test in integrated-cointegrated VAR systems. Journal of Applied Statistics, 27(8), 1021–1031. https://doi.org/10.1080/02664760050173346
- Shultz, J. M., Shepherd, J. M., Bagrodia, R., & Espinel, Z. (2014). Tropical cyclones in a year of rising global temperatures and a strengthening El Niño. Disaster Health, 2(3–4), 151–162. https://doi.org/10.1080/21665044.2014.1111722
- Škare, M., & Porada-Rochoń, M. (2023). Are we making progress on decarbonization? A panel heterogeneous study of the long-run relationship in selected economies. Technological Forecasting and Social Change, 188, 122279. https://doi.org/10.1016/j.techfore.2022.122279
- Škare, M., Blanco-Gonzalez-Tejero, C., Crecente, F., & del Val, M. T. (2022). Scientometric analysis on entrepreneurial skills-creativity, communication, leadership: How strong is the association? Technological Forecasting and Social Change, 182, 121851. https://doi.org/10.1016/j.techfore.2022.121851
- Su, C. W., Chen, Y., Hu, J., Chang, T., & Umar, M. (2023a). Can the green bond market enter a new era under the fluctuation of oil price? Economic Research-Ekonomska Istraživanja, 36(1), 536–561. https://doi.org/10.1080/1331677X.2022.2077794
- Su, C. W., Liu, F., Qin, M., & Chnag, T. (2022a). Is a consumer loan a catalyst for confidence? Economic Research-Ekonomska Istraživanja, 35, 1–22. https://doi.org/10.1080/1331677X.2022.2142260
- Su, C. W., Qin, M., Tao, R., & Moldovan, N. (2019a). Is oil political? From the perspective of geopolitical risk. Defence and Peace Economics, 32(4), 451–467. https://doi.org/10.1080/10242694.2019.1708562
- Su, C. W., Umar, M., Kirikkaleli, D., Awosusi, A. A., & Altuntaş, M. (2023b). Testing the asymmetric effect of financial stability towards carbon neutrality target: The case of Iceland and global comparison. Gondwana Research, 116, 125–135. https://doi.org/10.1016/j.gr.2022.12.014
- Su, C. W., Wang, X. Q., Tao, R., & Oana-Ramona, L. (2019b). Do oil prices drive agricultural commodity prices? Further evidence in a global bio-energy context. Energy, 172, 691–701. https://doi.org/10.1016/j.energy.2019.02.028
- Su, C. W., Yuan, X., Tao, R., & Shao, X. (2022b). Time and frequency domain connectedness analysis of the energy transformation under climate policy. Technological Forecasting and Social Change, 184, 121978. https://doi.org/10.1016/j.techfore.2022.121978
- Su, C. W., Yuan, X., Umar, M., & Chang, T. (2022c). Is presidential popularity a threat or encouragement for investors? Economic Research-Ekonomska Istraživanja, 35, 1–24. https://doi.org/10.1080/1331677X.2022.2129409
- Tilman, D., Balzer, C., Hill, J., & Befort, B. L. (2011). Global food demand and the sustainable intensification of agriculture. Proceedings of the National Academy of Sciences of the United States of America, 108(50), 20260–20264. https://doi.org/10.1073/pnas.1116437108
- Tongwane, M. I., & Moeletsi, M. E. (2018). A review of greenhouse gas emissions from the agriculture sector in Africa. Agricultural Systems, 166, 124–134. https://doi.org/10.1016/j.agsy.2018.08.011
- Tongwane, M. I., Mdlambuzi, T., Moeletsi, M. E., Tsubo, M., Mliswa, V., & Grootboom, L. (2016). Greenhouse gas emissions from different crop production and management practices in South Africa. Environmental Development, 19, 23–35. https://doi.org/10.1016/j.envdev.2016.06.004
- Tule, M. K., Salisu, A. A., & Chiemeke, C. C. (2019). Can agricultural commodity prices predict Nigeria’s inflation? Journal of Commodity Markets, 16, 100087. https://doi.org/10.1016/j.jcomm.2019.02.002
- Ubilava, D. (2012). El Niño, La Niña, and world coffee price dynamics. Agricultural Economics, 43(1), 17–26. https://doi.org/10.1111/j.1574-0862.2011.00562.x
- Ubilava, D. (2013). El Niño Southern Oscillation and primary agricultural commodity prices: Causal inferences from smooth transition models [Paper presentation]. Conference Paper, 57th AARES Annual Conference, Sydney, Australia.
- Ubilava, D. (2017). The ENSO effect and asymmetries in wheat price dynamics. World Development, 96, 490–502. https://doi.org/10.1016/j.worlddev.2017.03.031
- Ubilava, D. (2018). The role of El Niño Southern Oscillation in commodity price movement and predictability. American Journal of Agricultural Economics, 100(1), 239–263. https://doi.org/10.1093/ajae/aax060
- Ubilava, D., & Holt, M. (2013). El Niño Southern Oscillation and its effects on world vegetable oil prices: Assessing asymmetries using smooth transition models. Australian Journal of Agricultural and Resource Economics, 57(2), 273–297. https://doi.org/10.1111/j.1467-8489.2012.00616.x
- Vetter, S. H., Sapkota, T. B., Hillier, J., Stirling, C. M., Macdiarmid, J. I., Aleksandrowicz, L., Green, R., Joy, E. J. M., Dangour, A. D., & Smith, P. (2017). Greenhouse gas emissions from agricultural food production to supply Indian diets: Implications for climate change mitigation. Agriculture, Ecosystems & Environment, 237, 234–241. https://doi.org/10.1016/j.agee.2016.12.024
- Villoria, N., & Ubilava, D. (2012). Effects of El Niño Southern Oscillation on world cereal production [Paper presentation]. Conference Paper, the Agricultural & Applied Economics Associations Annual Meeting, Seattle, WA.
- Wood, S., & Cowie, A. (2004). A review of greenhouse gas emission factors for fertiliser production. Working paper. Cooperative Research Centre for Greenhouse Accounting.
- Zhang, C., & Qu, X. (2015). The effect of global oil price shocks on China’s agricultural commodities. Energy Economics, 51, 354–364. https://doi.org/10.1016/j.eneco.2015.07.012
- Zhou, L., Zhang, R., & Huang, J. (2019). Investor trading behavior on agricultural future prices. The North American Journal of Economics and Finance, 47(1), 365–379. https://doi.org/10.1016/j.najef.2018.05.004
- Zou, X., Li, Y., Li, K., Cremades, R., Gao, Q., Wan, Y., & Qin, X. (2015). Greenhouse gas emissions from agricultural irrigation in China. Mitigation and Adaptation Strategies for Global Change, 20(2), 295–315. https://doi.org/10.1007/s11027-013-9492-9