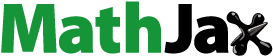
Abstract
Along with emission reduction targets, carbon abatement policies increasingly target the reduction of carbon intensity. In this context, uncovering factors that reduce carbon intensity is a timely research subject and carries significant policy implications. The goal of this study is to explore the dynamic relationships between carbon and energy intensity, renewable energy, economic development, structural transformation, and globalization in a global panel comprising 126 countries and two income-based subpanels. Robust System-GMM estimators indicate that increasing renewable sources in the energy mix can assist countries in mitigating the carbon intensity of electricity generation. Moreover, current results highlight that economic growth is the most effective mitigating factor of carbon intensity at the global level, revealing that on average, countries have managed to decouple economic and pollution (carbon intensity) growth. Results document these links both in the short-and, most importantly, the long-run setting. Other important results reveal that the mitigating effect of renewable energy is stronger with the increase of economic development, whereas structural transformation only decreases carbon intensity in low- and middle-income countries. Consequently, consistent long-term climate policies that promote these mitigating factors and decrease documented driving factors such as energy intensity could work synergistically across multiple SDGs of the 2030 Agenda.
JEL CODES:
1. Introduction
The process of industrialization, which drove global economic expansion over the last two centuries, has been closely linked to increased environmental pollution (Chen et al., Citation2021). Worldwide, ambient air pollution has emerged as a significant contributor to illness and mortality (Khomenko et al., Citation2021; Tudor, Citation2022), with the World Health Organization (WHO) labelling it as the greatest environmental health risk (World Health Organization (WHO), Citation2019). Furthermore, air pollution is one of the primary causes of lost labour productivity, cognitive deterioration, mental health issues, criminal behavior and decline in the average human expectancy (Yang & Tang, Citation2022; World Economic Forum, Citation2022). Moreover, the economic cost of air pollution is estimated at $2.9 trillion, or 3.3% of global GDP (World Economic Forum, Citation2020).
In this context, international efforts, such as the Paris Agreement (United Nations Framework Convention on Climate Change (UNFCCC),), Citation2021), aim to substantially reduce global greenhouse gas (GHG) emissions to mitigate the devastating consequences of climate change (Natural Resources Defense Council (NRDC),), Citation2021; Tudor & Sova, Citation2021). Moreover, significant concern is dedicated to preserving economic growth while reducing emissions—and particularly carbon emissions that accounted for 74% of GHG emissions in 2016 (OWID—Our World in Data). This process, called ‘decoupling’, represents the dissociation between economic growth and fossil fuels consumption, given that the latter is the main contributor to emissions (Ruffing, Citation2007; Le et al., Citation2021). Although in the years before the pandemic, a significant proportion of the global economy has experienced ‘absolute decoupling’, IEA documented in 2021 the strongest coupling of carbon emissions with GDP growth since 2010 (International Environment Agency (IEA),), Citation2022). This raises concerns over a temporary delay in the decarbonization process globally, particularly considering estimates that the war in Ukraine will affect the attainment pollution control and sustainable development goals (Rawtani et al., Citation2022).
Therefore, while quantifying total GHG or carbon emissions is essential, this approach does not address the efficiency of resource utilization, nor the link between emissions mitigation and economic growth (Fortune, Citation2021). In this vein, inspired by the DPSIR (Driving forces, Pressure, State, Impact, Response) typology of environmental indicators (Smeets & Weterings, Citation1999), we propose an analysis of emissions complemented by carbon intensity–based metrics that reveal the efficiency of resource use can identify potential decoupling of economic and emissions growth that is, in turn, a key factor for global decarbonization. reveals a strong positive relationship between carbon intensity (CI) and total GHG for top polluting countries (Climate Trade, Citation2021), particularly in the case of China and the United States, further attesting to the importance of mitigating carbon intensity in the global climate fight arena.
Figure 1. Carbon intensity of electricity production (CI) and total GHG emissions for the top polluting countries (last year of available data). Source: Authors’ representation based on World Bank Development Indicators (WDI) and the BP Statistical Review of World Energy and Ember.

Furthermore, a thorough analysis of CI cannot ignore technological changes that lead to increased shares of low-carbon and renewable sources in energy production and consumption (Geels, Citation2018). In this context, the mitigating effect of low-carbon and renewable (or sustainable) energy production and consumption on polluting emissions has long been recognized (Apergis et al., Citation2018; Wang et al., Citation2021). Our data confirm this link in the case of the top five global polluters—see . However, there is quite a high variation of the low-carbon energy share in electricity and energy sources across countries, which reflects countries’ endowments of fossil versus non-fossil fuels, development policies or commitments to reductions in GHG emissions (Cheng et al., Citation2016; Oshiro et al., Citation2018).
Figure 2. Share of primary energy consumption from renewable sources and total GHG emissions (GHG) for top polluting countries (last year of available data). Source: Authors’ representation based on World Bank Development Indicators (WDI) and the BP Statistical Review of World Energy and Ember.

Renewable sources have become a strategic decision for energy consumption with a prominent role in the long-term global energy mix (Rahman et al., Citation2022). However, the relationship between renewable energy consumption and CI remains under-investigated, which strongly motivates the current research. In this context, the present study assesses the role of renewable energy in carbon intensity for a large global panel of countries and on sub-panels delineated by countries’ development level. We thus shed light on the particularities of the renewable energy—carbon intensity link for economies that are located on different development phases, with implications for the formulation of decarbonization policies. Moreover, we support a better grasp of the uneven distribution of the exposure to the desired net-zero transition in the developed and developing world, with potential consequences on both development goals and environmental management.
Another critical relationship that needs to be properly understood refers to CI and energy intensity. Although both influence GHG emissions, their impact on emissions may be congruent or opposite (Le et al., Citation2019; Xia et al., Citation2021). Moreover, energy intensity is an important driving force of declining CI (Bhattacharyya & Matsumura, Citation2010; Ling et al., Citation2021), which associates well with the long-term trends in energy intensity at global level fuelled by increased energy efficiency and the economic shift towards services (Peters et al., Citation2017). Hence, we include energy intensity as a factor in our models to uncover interesting interaction patterns with CI, further leading to more nuanced policy approaches towards environmental challenges.
Furthermore, we include economic growth as an explanatory factor to determine whether countries are decoupling their economies to achieve decarbonization. We thereby contribute to the literature on decoupling drivers and, indirectly, to research on the Environmental Kuznets Curve (EKC), the conceptual cornerstone of the effects of economic growth on the environment (Grossman & Krueger, Citation1993).
In addition to the main variables of interest, the role of relevant covariates such as structural transformation (high-tech exports) and globalization (international trade) is also considered. These represent more recent additions to empirical research on environmental degradation, which capture the role of intensified global trade and investments as channels for economic development and for GHG emissions. We also test the relevance of economic/structural transformation as a distinguishing characteristic of growth accompanied by distributional changes in economic activities across the main sectors of the economy—agriculture, industry, and services (Herrendorf et al., Citation2014; Beylis et al., Citation2020). Therefore, we significantly enrich the research on CI and environmental issues by studying the joint influence of these developments on carbon emissions generated in the process of electricity production that accompanies economic growth.
The original contributions of this research are outlined below. First, we concentrate on carbon emissions from the energy system, or CI, which adds to a rather limited literature that has traditionally preferred CO2 or GHG emissions to capture environmental damage. Second, the System-GMM panel estimators we use concurrently with the delta method deal with endogeneity and cross-sectional dependence reveal both the short- and long-run dynamics between the variables of interest in our model, allowing for a more nuanced understanding of decarbonization and its drivers and, ultimately, better-fitting environmental policies. Third, in addition to the importance of renewable energy in achieving decarbonization, which is a traditional variable in environmental models, we examine the combined impact of structural transformations in economies around the world and globalization, as proxied by trade openness, on CI, demonstrating how their interaction may support decarbonization and ultimately producing evidence on synergistic effects across multiple SDGs of the 2030 Agenda. To the best of our knowledge, this is the first contribution that examines the relationship between CI, renewable energy, and energy intensity in relation to economic transformation and globalization. This provides significant insight into the policy tools required to stimulate decarbonization while maintaining economic growth. Finally, by categorizing the countries in our panel based on their development level, we reveal the potential differences that the demands of growth in the developing world impose on decarbonization drivers as well as the paths taken by developed countries toward less polluting economic activity.
The remainder of our paper is divided into four major sections. Section 2 presents the relevant theoretical and empirical approaches in the literature, Section 3 outlines the research methodology, Section 4 shows the main results and discusses them in relation to previous findings, and Section 5 concludes by highlighting the main avenues for decarbonization policies revealed by research, as well as future explorations of the topic.
2. Literature review
Urgent action is needed to combat the impact of climate change, and the international community has started taking steps in this respect as part of the Paris Agreement and COP26, which aim to maintain global warming to below 2 °C by 2100. To achieve this, several countries vowed to reduce their total GHG emissions by adhering to nationally determined contributions (NDCs) and net-zero targets.
However, it is increasingly important to make the distinction between absolute pollution, or total GHG emissions (IEA, Citation2017; Lin et al., Citation2011), and relative pollution, or carbon intensity (Wilson et al., Citation2018; Vuuren et al., Citation2018), which measures the volume of emissions per unit of GDP or per electricity produced or consumed. Jaller and Matthews (Citation2021) argue that measuring total emissions do not clearly explain how efficiently entities use their resources, and what kind of economic growth leads to changes in total emissions. The authors argue that whilst making intensity improvements do not guarantee short-term reductions in total emissions, the trajectory of whether an entity is emitting less for a unit of output is relevant. To this end, it is important to consider economic growth in the context of intensity-based measures to determine whether entities are decoupling their economies in their road to decarbonization. Otherwise, total carbon reduction targets could make it more difficult for entities to meet their climate goals and remain competitive by reducing their flexibility to make incremental improvements. Similarly, Khan (Citation2019) supports the use of CI with the aim of finding opportunities for emissions reductions in the electricity sector.
One of our key research questions is whether entities are decoupling their economies to eventually drive absolute decarbonization. To address this, we compare intensity-based measures (e.g., renewable energy consumption) with economic growth and structural transformation, in line with the use of GDP (for countries) or revenues (for firms) by groups such as S&P Dow Jones in their carbon intensity indices.
According to the Environmental Kuznets Curve (EKC) theory proposed by Kuznets (Citation1955), there is an ‘inverted U curve’ between environmental pollution and economic growth, which means that economic growth initially generates high levels of environmental deterioration relative to income, but the trend reverses as incomes rise (Uchiyama, Citation2016). Extant literature remains mixed regarding the validation of EKC (Sarkodie & Strezov, Citation2019), but we find interesting the results of Li et al. (Citation2019) that identified two high-order clusters during 1971–2010, namely higher and lower-income countries, which showcased distinct features in respect to their carbon intensities. In our paper, we similarly group countries using World Bank (Citation2022) income level country classification.
Scale, composition, and technique/technology are the main drivers of the economic growth impact on the environment, with different relative importance depending on the position on the curve (Grossman & Krueger, Citation1995; Nathaniel et al., Citation2021). Thus, the scaling-up of economic activities at the initial stages of growth, driven by increased demand for energy, results in high GHG emissions, particularly when the demand is satisfied mostly by fossil fuels (Baloch et al., Citation2019). As the economy continues to grow, the scale effect is replaced by a composition effect determined by a structural shift associated with the development and consolidation of industrialization (Hettige et al., Citation2000; Shahbaz & Sinha, Citation2019). However, in the advanced stages of growth, the technique/technological effect enters the picture forcefully, as economies begin investing in cleaner processes that mitigate more successfully the negative impact of development on the environment (Balsalobre-Lorente et al., Citation2018). Murshed et al. (Citation2022) drew attention on the threshold level of economic development level that leads to the EKC to bend and suggest that, typically, the scale and composition effects are prominent before the threshold is reached, while the technological effect is more powerful after the threshold level. Therefore, one should expect finding a more substantial significance of the first two effects for the relationship between economic growth and emissions for less developed economies and of the third effect for more developed countries.
The impact of economic structural transformations on environmental quality must thus not be overlooked. Scholars agree that large GHG emission reductions cannot be realized without transitioning to more sophisticated economic activities that enable technologically improved manufacturing that increases productivity and reduces emissions (Andreoni et al., Citation2021; Markaki et al., Citation2021). Scholars have tried many methods to incorporate economic developments into emissions or carbon intensity models. Zheng et al. (Citation2020) used the Logarithmic Mean Divisia Index (LDMI) decomposition to separate industry segments into emission- and non-emissions-dominating groups and examine CO2 emission reduction in many Chinese cities under different industrial transformation trajectories. Ma et al. (Citation2019) employed a similar technique. Dynamic spatial models that study industrial structure and technological growth under environmental constraints have also been suggested in the literature (Li et al., Citation2017; Usman & Hammar, Citation2021).
An emerging line of research, that we also embrace, included the level of structural transformation of economies in econometric models through high-tech exports (HTE), considered a good indicator of a country’s industrialization and economic transformation process (Freire, Citation2021). Beser and Soyyigit (Citation2019) discover that per capita income has a greater impact on CO2 emissions than HTE in G20 nations, even while developed countries grow their emissions through production and export. Tnani (Citation2018) found that HTE, R&D, innovation, and environmental levies can reduce emissions in leading innovative countries with the most patent applications. These findings encourage us to use HTE to represent structural transformation.
Urban and Nordensvärd (Citation2018) demonstrate that Nordic nations’ high low-carbon energy shares explain their decoupling of economic development from carbon emissions. Their findings suggest that adding low-carbon and renewable energy to the energy mix could boost economic growth and reduce pollution. According to Zafar et al. (Citation2019) and Nathaniel et al. (Citation2021), preferring low-carbon energy can alleviate environmental harm without affecting economic growth, allowing the economy to enter the descending phase of the EKC sooner and improving environmental conditions. In their study of low-carbon technologies’ direct and spillover effects on carbon emissions, Sun et al. (Citation2019) found that technological improvement can hasten the EKC's inflection point. Su et al. (Citation2021) and Pincheira and Zuniga (Citation2021) also concluded that technology positively impacts emissions and, further, EKC.
There are several ways to accomplish climate commitments without impeding economic growth. Horobet et al. (Citation2022) concluded that globalization measured by trade openness might reduce the CI of energy production, but biofuels could raise it. This suggests that switching to nuclear and renewable energy could assist countries achieve their sustainable development goals faster. York (Citation2016) shows that decarbonization may increase energy demand, thus authorities must ensure that green energy replaces carbon-based energy consumption. This is consistent with Zehner (Citation2012) and Molyneaux et al. (Citation2010) who found that reducing carbon emissions per unit of energy may ultimately increase energy demand.
Other factors may affect carbon emissions; however literature is inconsistent regarding their underlying relationship. Our study examines the dynamic links between carbon and energy intensity, renewable energy, economic development, structural transformation, and globalization in 126 countries with different economic development levels. Cheng et al. (Citation2019) explored how renewable energy supply, economic development, exports, foreign direct investment, and domestic lending to the private sector affected carbon emissions per capita for Brazil, Russia, India, Indonesia, China, and South Africa (BRIICS) between 2000 and 2013. The analysis reveals that while renewable energy, foreign direct investment, and domestic credit to the private sector might dramatically reduce carbon emissions per capita, GDP per capita and exports tend to raise them. However, Wu et al. (Citation2018) used the Gini coefficient to show that the CI gap between 30 Chinese provinces between 1997 and 2014 increased. Economic growth, foreign direct investment, and trade openness negatively affected CI, while urbanization was its main driver in China’s provinces.
Sarkodie and Strezov (Citation2019) observed that foreign direct investments targeting sustainable energy can help emerging economies meet their environmental targets. However, Zhu et al. (Citation2016) found a negative link between carbon emissions and foreign direct investment, with the result becoming significant at higher quantiles. The study also indicated that trade liberalization reduced carbon emissions. Hu et al. (Citation2018) agreed that emerging economies should expand commercial services trade for low-carbon economic growth.
Our contribution to the existing body of research is to observe the common effects of various evolutions on environmental challenges but provide a deeper understanding of the interactions between economic transformation, technology, trade and globalization, renewable energy, energy intensity, and carbon intensity.
3. Research methodology
3.1. Panel data set
This study uses a set of panel data for 126 countries from 2007 to 2021. To allow for the estimation of a dynamic model while maximizing the number of observations, the countries have been selected based on the criteria of at least two years of available data. Consequently, an unbalanced global panel has emerged.
The dependent variable in the empirical investigation is represented by the CI of electricity production, measured in grams of carbon emitted per kilowatt-hour. Based on the Kaya identity, Peters et al. (Citation2017) suggest using the energy CI (CO2/energy) to track Paris Agreement progress (Yamaji et al., Citation1993; Kaya & Yokobori, Citation1997). The latter is a mathematical identity that explains total GHG emissions as a product of population, GDP per capita, energy intensity (energy per unit of GDP), and CI, commonly used in environmental literature to examine the complex and multidirectional relationships between these elements (Calbick & Gunton, Citation2014; Tavakoli, Citation2017). Thus, this study’s goal is to examine the dynamic links between carbon intensity (CI), energy intensity (EI), renewable energy (REC), economic development (GDP), structural transformation (HTE), and globalization (GLOB)—see EquationEquation (1)(1)
(1) . Variables are based on carbon intensity and environmental anthropogenic literature.
(1)
(1)
reports the variables included in the dynamic model, including abbreviations, definitions, and sources.
Table 1. Variable description and data sources.
3.2. Method
We model the relationship between CI, energy intensity, and renewable energy using dynamic panel data regression, moderated by globalization, economic development, and structural transformation. Panel models are a robust methodology used in environmental economics because of their numerous advantages over traditional cross-sectional or time-series models, given the limitations of econometric analyses due to a lack of observations (Tugcu, Citation2018). Furthermore, due to the speed with which they adjust to changes in economic policies, panel data approaches are enticing for empirical analyses in energy economics (Baltagi, Citation2005). These methodologies are particularly appealing to our research topic because they allow us to investigate the short- and long-run dynamics between the variables of interest, allowing for a more nuanced understanding of decarbonization and climate change that may support better-adjusted energy and environmental policies. This represents, as outlined in the Introduction section, one of the main contributions this paper makes to the literature.
The generalized method of moments (GMM) that introduces the lagged dependent variable among regressors is employed in this study to explore the relationships of interest. The system-GMM (Sys-GMM) estimator proposed by Arellano and Bover (Citation1995) and Blundell and Bond (Citation1998) has been confirmed as a strong estimator (Canh et al., Citation2019) and is particularly suitable for wide short panels when N > T (Oseni, Citation2016; Wang et al., Citation2022). Moreover, as opposed to the difference-GMM estimator, due to the inclusion of the lagged dependent variable, Sys-GMM handles well omitted dynamics in static panel data models (Omri & Nguyen, Citation2014). Equally important, Sys-GMM is robust in the presence of potential endogeneity of regressors and/or heteroskedasticity and autocorrelation within individuals (Canh et al., Citation2019; Konstantakopoulou, Citation2022; Roodman, Citation2009). Consequently, as panel GMM techniques can effectively deal with endogeneity and cross-sectional dependence (Apergis et al., Citation2022; Fang et al., Citation2022), they are implemented in the empirical investigation to uncover the short-run dynamics in estimating the proposed carbon intensity model. Of note, both the Difference and System-GMM estimators are available, and both can be implemented in a one-step or two-step version. However, it has been acknowledged that the System-GMM (Sys-GMM) technique is more efficient than the difference-GMM, given its ability to improve precision and reduce finite sample bias (Baltagi, Citation2008; Oseni, Citation2016), which motivated its choice in this research.
The extended form of the empirical model is given by EquationEquation (2)(2)
(2) . Of note,
stand for fixed country effects,
stand for time-effects,
is the error term with zero-mean, whereas all other variables are presented in .
(2)
(2)
where i = 1,…,126 and t = 2007,…,2021.
We estimate this dynamic model with a one-stage and a two-stage system GMM estimator. Of note, in the framework of a dynamic panel model as per EquationEquation (2)(2)
(2) , all the estimated coefficients are interpreted as short-term effects of the driving factors. However, as the global fight against pollution is a long-term process, the assessment of the long-term effects of the explanatory variables is equally relevant. In this context, we additionally implement the procedure developed by Papke and Wooldridge (Citation2005) to reveal the long-run coefficients for the main variables. In particular, the procedure requires rescaling each short-term coefficient by a factor of (1-the coefficient of the lagged dependent variable)-1. Furthermore, the standard errors for the long-run coefficients are produced using the delta method, via the ‘deltaMethod’ function within R’s ‘car’ package (Fox & Weisberg, Citation2019), similar to Kripfganz and Schwarz (Citation2019) and Sakyi and Immurana (Citation2021). Additionally, the asymmetric effects of driving factors are also assessed though re-estimating the main model depicted in EquationEquation (2)
(2)
(2) on two different income-based subpanels of countries according to the World Bank classification.
Overall, this research employs several investigative techniques, as follows: (i) It performs an exploratory analysis of data to assess the main relationships and the heterogeneity across countries; (ii) It estimates System-GMM dynamic panel models in one-step and two-steps variant to assess the relationship of interest on the short-run; furthermore, it applies the Windmeijer (Citation2005) correction to produce robust covariance matrix within the two-step Sys-GMM estimation; (iii) It employs the Papke and Wooldridge (Citation2005) technique corroborated with the delta method to assess the relationship of interest on the long-run; (iv) It performs diagnostic tests to confirm the reliability of the results, including the J-test of over-identifying restrictions developed by Sargan (Citation1958) and Hansen (Citation1982) for the null hypothesis of instrument validity and the test for second-order serial correlations in the residuals proposed by the Arellano and Bond (Citation1991). Additionally, Wald tests for the coefficients and for time dummies are estimated and reported (Wooldridge, Citation2010). Also, time dummies included in estimations can also capture any potential structural break in the time series (Corbacho et al., Citation2010; Rahman et al., Citation2022); (v) It explores potential asymmetry in the impact of explanatory variables on carbon intensity, which concurrently contributes to assure the robustness of results, and (vi) It further checks the findings’ robustness by employing the Dumitrescu-Hurlin causality test (Dumitrescu & Hurlin, Citation2012) to confirm the bidirectional causal nexus between the proposed driving factors and carbon intensity at the world level.
All estimations are carried out in R software. Specifically, System-GMM models are estimated by calling the dedicated ‘pgmm’ function in the R ‘plm’ package developed by Croissant and Millo (Citation2008).
4. Results and discussion
This section of the study presents the main findings resulting from the Sys-GMM panel regressions, complemented with a Granger causality analysis to verify the robustness of outcomes. We first present an exploratory analysis of the underlying variables in the panel and then proceed to estimation results and discuss them against existing literature.
4.1. Exploratory data analysis
presents descriptive statistics. The global panel reported significant heterogeneity among countries for all variables, with notably high ranges in economic development and structural transformation, as evidenced by the share of high-technology exports in manufactured exports. The CI of electricity production ranges from zero to 819.44 grams of carbon emitted per kilowatt-hour, with a 381.44 mean. Energy intensity ranges from 0.23 to 6.30, averaging 1.31. As expected, the global energy mix varies widely, with fossil-fuel-reliant countries consuming zero renewable energy and decarbonized countries using 82.83 percent. The global panel averages 13.32 percent renewable energy. Globalization averages 88.57 and high-tech exports 12.44.
Table 2. Descriptive statistics.
shows the worldwide panel’s energy-carbon intensity relationship in each country’s most recent year of data. Even though most industrialized economies (excluding Singapore, Estonia, and the UAE) have both low energy intensity and high carbon intensity, preliminary data suggest a positive association between the two (bottom left quadrant of the graph). The same section of the graph shows other developing countries with low energy and carbon intensity, including Angola, Kenya, Colombia, and Nepal. This suggests that the relationship between the two variables is influenced by factors other than development, such as the share of renewable energy in total energy sources, which is linked to technological advancement and economic structural transformation.
Figure 3. The relationship between the Energy intensity (EI) and the Carbon intensity of electricity production (CI) at the world level; the last year of available data per country. Source: Authors’ representation with data from the BP Statistical Review of World Energy and Ember.

shows global renewable energy-carbon intensity correlation. Visual inspection suggests a substantial negative association between the two factors and significant diversity among countries. Like in , most wealthy countries are on the bottom left of the graph, indicating low CI but also low renewable energy shares, whereas numerous developing economies show similar or even lower CI with greater renewable energy shares (Kenya, Sierra Leone, Eswatini, Nepal, Paraguay, etc.). However, the latter countries are not major polluters and may have significant renewables shares due to natural endowments (mostly hydropower and biomass). At the other end, developed economies and many developing countries with easy access to fossil fuels, which have built their progress on fossil fuels and have low renewable energy shares in their primary energy consumption, have only started a sustained transition toward low-carbon energy sources in the last decade (Chapman & Okushima, Citation2019; Wang et al., Citation2022).
Figure 4. The relationship between the share of primary energy consumption that comes from renewable sources (REC) and the Carbon intensity of electricity production (CI) at the world level; last year of available data per country. Source: Authors’ representation with data from the BP Statistical Review of World Energy and Ember.

4.2. Estimation results and discussion
All empirical results, including the two-step Sys-GMM estimates with their long-run coefficients and the one-step estimates for the global panel of 126 countries, are summarized in and Appendix A, respectively. It should be mentioned that in the Sys-GMM framework, a two-step estimator is acknowledged as more effective than a one-step estimator (Biresselioglu et al., Citation2016). Thus, although the coefficients for both versions are estimated and reported, the discussion of findings will concentrate on the estimates produced by the two-step Sys-GMM version, included in .
Table 3. Two-step system GMM estimates for the carbon intensity model.
Firstly, complying with two key conditions is paramount to assure the consistency of System GMM estimations, as follows: (i) the lack of serial correlation in the error term; and (ii) the lack of correlation between the instruments and the error term. As mentioned, these conditions are tested with the AR(2) and the J-test of over-identifying restrictions, respectively, which are reported within the results tables. Test results consistently indicate that both the null hypothesis of no second-order serial correlation in the error term, as well as the null hypothesis of valid instrumentation, are accepted in all specifications. Consequently, all diagnostic tests support the model’s validity, which allows for the discussion of empirical findings.
The estimation results show that energy intensity affects carbon intensity positively and significantly, suggesting that if energy intensity increases by one unit, we expect the carbon intensity to increase by 88 units, on average. This finding is in line with the results obtained by Eluwole et al. (Citation2020) in the case of the top 10 polluting countries, Yu et al. (Citation2020) for Chinese provinces, and Rahman et al. (Citation2022) for large emerging economies. Considering the long-term declining trend in energy intensity (Peters et al., Citation2017), this result points towards expectations of reducing carbon intensity at global level. Moreover, lower emissions from manufacturing and transportation were the effect of lower energy intensity, along with changes in fuel mix towards increased low-carbon energy (Greening, Citation2004; Schipper et al., Citation2001).
Other findings indicate that renewable energy consumption negatively and significantly affects carbon intensity, suggesting that increasing renewable sources in the energy mix can help countries mitigate the carbon intensity of electricity generation and meet Paris Agreement NDC emissions reduction targets. This result agrees with Adams and Acheampong (Citation2019), Saidi and Omri (Citation2020a), Wang et al. (Citation2022), and Rahman et al. (Citation2022). Thus, we contribute to scholarly literature and international bodies’ research and guidance on renewable energy’s crucial role in pollution mitigation. Changing the fuel mix to low-carbon energy sources is difficult, but technology developments and the growing difficulty of fossil fuel exploitation have made renewable energy cheaper and more affordable (Bazilian et al., Citation2013; Kalair et al., Citation2021). As Fernandez et al. (Citation2016) shown for Spain, the more persistent the integration of renewables into the energy mix, the lower the power price since cheaper renewables would replace more expensive traditional producers.
Most importantly, for the entire world panel, increased GDP per capita offers the economic underpinning for carbon intensity reduction and decarbonization. Thus, economic growth emerges as the most effective global carbon intensity moderating factor, showing that, on average, countries have decoupled economic and pollution growth. These findings support Davidsdottir and Fisher (Citation2011), Cheng et al. (Citation2018), and Dogan and Seker (Citation2016), who discovered a link between economic growth and carbon intensity reduction in the US and EU-28 countries. However, current research shows a decoupling between economic development and carbon intensity, not only in developed economies like the US or EU, which may indicate that world economies have achieved a level of economic development that supports environmental degradation decrease.
In turn, the structural transformation factor coefficient is positive and statistically significant at 10%, indicating that progress towards structural transformation will increase electricity generation’s CI. This mirrors research that show early economic development falls due to excessive resource exploitation, fossil fuel focus, and unsustainable industrialization (Baloch et al., Citation2019; Copeland & Taylor, Citation2004; Nathaniel et al., Citation2021). Thus, structural transformation limits carbon intensity improvement globally and on average. We agree with Fankhauser et al. (Citation2013) and Golub and Toman (Citation2016) that structural transformation, including technological innovations, is one of the main inducers of emissions mitigation, along with intelligent carbon policies and renewable energy investments. We also concur with Andersson and Karpestam (Citation2013) that reconfiguring economic structures and instituting a worldwide carbon price will make green technology investments more profitable.
It should also be noted that current findings do not establish a link between globalization and the carbon intensity of electricity generation for the panel of 126 countries considered in this study. This contradicts the findings of Rafiq et al. (Citation2016) and Fang et al. (Citation2020), which demonstrate that trade openness reduces carbon intensity. However, both papers included urbanization as a regressor, which may have affected their conclusions compared to ours. However, Ezcurra (Citation2007) found no evidence of trade openness as a possible explanatory variable for the dispersion of carbon emissions per capita in a panel of 87 countries, while Wang and Zhang (Citation2021) reported a heterogeneous effect of trade openness on the decoupling of economic development and carbon emissions.
Notably, energy efficiency, renewable energy consumption, economic development, and structural transformation all have large implied long-run coefficients that match the short-term effects. presents estimates of the independent variables’ long-term effects based on Papke and Wooldridge (Citation2005) using the DeltaMethod() function in R's ‘car’ package. This finding informs policymakers that these CI determinants are crucial both over short- and long-term. Since renewable energy consumption and economic development have a bigger long-term mitigating effect, energy mix and income improvements have a higher marginal influence on long-term carbon intensity improvement. Thus, these results highlight the major implications of consistent long-term climate policies.
Table 4. The long-run impact of driving factors on carbon intensity.
Subsequently, we apply the Dumitrescu and Hurlin (Citation2012) Granger causality test to uncover causal relations among the variables of interest that might have escaped in the analysis of long-run coefficients. Additionally, the causal links among factors complement the long-run estimates in informing effective energy policies (Bui et al., Citation2021). Of note, Pesaran’s CD test (Pesaran, Citation2004, Citation2021) has been first applied to the lagged variables to evaluate potential cross-sectional dependence in our sample. Test results (available upon request) consistently confirm cross-sectional dependence, which in turn indicates that Pesaran’s CIPS test (Pesaran, Citation2007) is appropriate to assess data stationarity. Results (not reported) indicate that GLOB and HTE are I(0), whereas all other variables are I(1). However, it should be mentioned that results might be unreliable due to the unbalanced nature of the panel (i.e., countries with non-consecutive periods), which can also affect Granger estimations.
The results of the Dumitrescu-Hurlin panel causality test results are reported in . As usual, non-stationary variables were first transformed into stationary variables by taking the first difference. To estimate panel Granger (non)-causality tests, the global panel has been redesigned to include all countries with at least 8 yearly observations, meeting the minimal observations requirement for R's ‘plm package’ pgrangertest() function. Thus, Granger tests are estimated on a 57-country panel, making results unreliable for comparison. However, reverse causality can reveal endogeneity (Li et al., Citation2021).
Table 5. Results (Z-tilde) of Dumitrescu-Hurlin panel causality test.
Long-term estimates show that energy intensity causes carbon intensity, but not the inverse. This finding is consistent with research on energy consumption, income, and carbon emissions in the US (Soytas et al., Citation2007), Turkey (Shahbaz et al., Citation2013), and China (Zhang & Cheng, Citation2009). However, we do not support previous findings of bi-directional relationships between energy intensity or consumption and emissions—Sharif et al. (Citation2020) for Turkey, Asumadu-Sarkodie and Owusu (Citation2016) for Ghana. This may be due to the large global panel of countries addressed in this study, as opposed to single country estimates in previous studies.
Moreover, CI mitigates structural transformation (high-technology exports), suggesting dynamic endogeneity may cause estimation issues. Thus, dynamic endogeneity in the current setting biases conventional panel methods like fixed effects estimators, calling into question past studies using similar approaches (Li et al., Citation2021). System-GMM estimators can handle endogeneity produced by reverse causality and unobserved heterogeneity by ‘internally altering the data’ (Ullah et al., Citation2018; Leszczensky & Wolbring, Citation2022), boosting confidence in current findings.
In the final step, we evaluate the potential asymmetric effects of CI drivers due to countries’ economic development stages. This method also assesses the robustness of findings to economic progress (Chen et al., Citation2005). The entire sample is divided in two income-level sub-panels, according to the World Bank classification: (1) 44-country high-income (HI) sub-panel; (2) 34-country middle and low-income (MLI) sub-panel. This analysis covers all countries with at least three years of observations, creating two unbalanced income-based sub-panels. shows that renewable energy and high-technology export determinants differ across high-income and low- and middle-income countries. Thus, renewable energy reduces carbon intensity in high-income countries but has no statistically significant effect in low- and middle-income countries. These findings confirm Taconet et al. (Citation2020) and Nguyen and Kakinaka (Citation2019) findings that countries have different renewable energy potentials and that high-income countries have a negative association between renewable energy consumption and CO2 emissions. However, we cannot confirm the positive effect of renewables on emissions in low-income countries as advanced by the latter authors, but our findings support the ‘neutrality’ between the two variables in low-income countries, explained by the uneven and inadequate utilization of renewable energy sources (Saidi & Omri, Citation2020b).
Table 6. Robust two-step system GMM estimates for the carbon intensity model on income-based sub-panels.
On the contrary, the structural transformation factor, denoted by high-technology exports, has a negative and considerable influence on CI in low- and middle-income countries. In high-income countries, this effect is absent. Thus, as economic development increases, renewable energy impacts increase and high-technology export effects decrease. Moreover, this finding reinforces the need for lower-income countries to shift their economies toward less-polluting sectors and industries, profiting from natural endowments in non-fossil fuels and/or trade and foreign direct investments (Horobet et al., Citation2021). In a volatile global climate with unpredictable energy prices and political conflicts, this will also boost resilience, competitiveness, and minimize exposure to fossil fuel costs and carbon price risk (Bulai et al., Citation2021; Rodrik, Citation2009; Teignier, Citation2018).
5. Conclusions
Carbon intensity-based indicators that show resource efficiency and decoupling of economic growth and pollution are becoming more important in global climate combat. Thus, mitigating factors for CI are paramount for carbon abatement policies. In this context, this study is a valuable contribution in the area of carbon intensity research that provides complex insights into the interactions between carbon intensity, renewable energy, energy intensity, structural transformation, and globalization. Ultimately, the current research produces evidence on synergistic effects across multiple SDGs of the 2030 Agenda. System-GMM panel estimators were used with the delta approach to address endogeneity and cross-sectional dependence and highlight short- and long-term dynamics between variables. By estimating short- and long-term effects, analyzing feedback effects and endogeneity via panel Granger causality tests, and re-estimating the correlations of interest on two income-level subpanels, the findings were proved to be robust.
Estimation results identified economic growth as the most effective mitigating factor of carbon intensity globally, revealing that, on average, countries managed to decouple economic and carbon intensity growth. This also suggests that global climate change regulations and packages in recent decades have not hurt economic growth, suggesting that continuing this route is the best approach to manage environmental degradation. Furthermore, supporting low- and middle-income nations develop through energy system reform and energy efficiency will accelerate the world’s progress toward the 2050 carbon neutrality objective.
Renewable energy consumption can also decrease carbon intensity significantly at the world level. Moreover, the mitigating impact of renewable energy consumption and economic development is stronger in the long term, highlighting the significant implications of consistent long-term climate policies. However, a disaggregation occurs between high-income and low- and middle-income countries whereby renewable energy has a strong mitigating effect on carbon intensity in high-income countries, but not in low and middle-income countries. This finding supports international agendas to adopt renewable energy as an effective pollution mitigant and strengthens the need for lower-income countries to focus on growing less or non-polluting sectors, by taking advantage of natural endowments in non-fossil fuels and/or trade and foreign direct investments. In this regard, providing affordable financing to lower-income countries to scale up their renewable energy infrastructure and supporting private sector renewable energy investments through international institutions or private-public partnerships may be successful in utilizing many lower-income countries’ natural endowments (water, wind, solar, etc.) in renewable energy. Besides, elaborating and, more crucially, implementing national or regional plans to attract foreign direct investments in renewable energy and circular economy industries may help mitigate climate change, create jobs, promote domestic industries, and boost economic growth. Furthermore, economic structural transformations also affect environmental quality. Estimation results show heterogenic effects: the structural transformation factor, proxied by high-technology exports, significantly decreases carbon intensity in low and middle-income countries, has no significant effect in high-income countries, and increases carbon intensity for the global panel, implying that structural transformation progress leads to increased carbon intensity of electricity generation globally. These findings show that lower-income nations that escape commodity dependence and achieve structural change (World Economic Forum, Citation2022) can profit from lowered pollution. This objective complements the ‘twin transition’ goal of decarbonization and digitalization forged by ICT development in both the developed and developing worlds. As structural transformation continues, we advise countries to emphasize renewable energy sources and integrate technological development into energy systems’ reform.
The limitations of our research reside around data availability, which limited the options regarding the estimation methods. Also, several other variables may have been included in the model, but this might have altered the information to be extracted from the links we aimed at exploring. We intend to address these in future research, along with investigating the impact of globalization considering foreign investments (direct and portfolio) instead of trade, as well as the relevance of countries’ existing energy mix and environmental measures, including commitments to fight climate change, on the carbon—energy intensity relationship.
Disclosure statement
The authors report there are no competing interests to declare.
Data availability statement
All data used in this research are publicly available in the World Bank Database (https://databank.worldbank.org/) and the Our World in Data (https://ourworldindata.org/).
Additional information
Funding
References
- Adams, S., & Acheampong, A. O. (2019). Reducing carbon emissions: The role of renewable energy and democracy. Journal of Cleaner Production, 240, 118245. https://doi.org/10.1016/j.jclepro.2019.118245
- Andersson, F. N. G., & Karpestam, P. (2013). CO2 emissions and economic activity: Short- and long-run economic determinants of scale, energy intensity and carbon intensity. Energy Policy, 61, 1285–1294. https://doi.org/10.1016/j.enpol.2013.06.004
- Andreoni, A., Mondliwa, P., Roberts, S., & Tregenna, F. (2021). Structural transformation in South Africa: The challenges of inclusive industrial development in a middle-income country (p. 416) Oxford University Press.
- Apergis, N., Jebli, M. B., & Youssef, S. B. (2018). Does renewable energy consumption and health expenditures decrease carbon emissions? Evidence for Sub-Saharan Africa countries. Renewable Energy, 127, 1011–1016. https://doi.org/10.1016/j.renene.2018.05.043
- Apergis, N., Polemis, M., & Soursou, S., E. (2022). Energy poverty and education: Fresh evidence from a panel of developing countries. Energy Economics, 106, 105430. https://doi.org/10.1016/j.eneco.2021.105430
- Arellano, M., & Bond, S. (1991). Some tests of specification for panel data: Monte Carlo evidence and an application to employment equations. The Review of Economic Studies, 58(2), 277–297. https://doi.org/10.2307/2297968
- Arellano, M., & Bover, O. (1995). Another look at the instrumental variable estimation of error-components models. Journal of Econometrics, 68(1), 29–51. https://doi.org/10.1016/0304-4076(94)01642-D
- Asumadu-Sarkodie, S., & Owusu, P. A. (2016). Multivariate co-integration analysis of the Kaya factors in Ghana. Environmental Science and Pollution Research International, 23(10), 9934–9943. https://doi.org/10.1007/s11356-016-6245-9
- Baloch, M. A., Mahmood, N., Zhang, J. W., & Danish. (2019). Effect of natural resources, renewable energy and economic development on CO2 emissions in BRICS countries. The Science of the Total Environment, 678, 632–638. https://doi.org/10.1016/j.scitotenv.2019.05.028
- Balsalobre-Lorente, D., Shahbaz, M., Roubaud, D., & Farhani, S. (2018). How economic growth, renewable electricity and natural resources contribute to CO2 emissions? Energy Policy, 113, 356–367. https://doi.org/10.1016/j.enpol.2017.10.050
- Baltagi, B. (2005). Econometric analysis of panel data (3rd ed.). John Wiley & Sons.
- Baltagi, B. H. (2008). Econometric analysis of panel data (Vol. 4). John Wiley & Sons.
- Bazilian, M., Onyeji, I., Liebreich, M., MacGill, I., Chase, J., Shah, J., Gielen, D., Arent, D., Landfear, D., & Zhengrong, S. (2013). Re-considering the economics of photovoltaic power. Renewable Energy, 53, 329–338. https://doi.org/10.1016/j.renene.2012.11.029
- Beser, N. O., & Soyyigit, S. (2019). The effects of high technology export and per capita income on carbon emission: An investigation on G20 countries. Business and Economic Horizons, 15(4), 542–559.
- Beylis, G., Jaef, R. F., Sinha, R., & Morris, M. (2020). Going Viral: COVID-19 and the accelerated transformation of jobs in Latin America and the Caribbean. World Bank Publications.
- Bhattacharyya, S. C., & Matsumura, W. (2010). Changes in the GHG emission intensity in EU-15: Lessons from a decomposition analysis. Energy, 35(8), 3315–3322. https://doi.org/10.1016/j.energy.2010.04.017
- Biresselioglu, M. E., Kilinc, D., Onater-Isberk, E., & Yelkenci, T. (2016). Estimating the political, economic and environmental factors’ impact on the installed wind capacity development: A system GMM approach. Renewable Energy, 96, 636–644. https://doi.org/10.1016/j.renene.2016.05.034
- Blundell, R., & Bond, S. (1998). Initial conditions and moment restrictions in dynamic panel data models. Journal of Econometrics, 87(1), 115–143. https://doi.org/10.1016/S0304-4076(98)00009-8
- Bui, Q., Wang, Z., Zhang, B., Le, H. P., & Vu, K. D. (2021). Revisiting the biomass energy-economic growth linkage of BRICS countries: A panel quantile regression with fixed effects approach. Journal of Cleaner Production, 316, 128382. https://doi.org/10.1016/j.jclepro.2021.128382
- Bulai, V. C., Horobet, A., Popovici, O. C., Belascu, L., & Dumitrescu, S. A. (2021). A VaR-based methodology for assessing carbon price risk across European Union economic sectors. Energies, 14(24), 8424. https://doi.org/10.3390/en14248424
- Calbick, K. S., & Gunton, T. (2014). Differences among OECD countries’ GHG emissions: Causes and policy implications. Energy Policy, 67, 895–902. https://doi.org/10.1016/j.enpol.2013.12.030
- Canh, N. P., Schinckus, C., & Thanh, S. D. (2019). Do economic openness and institutional quality influence patents? Evidence from GMM systems estimates. International Economics, 157, 134–169. https://doi.org/10.1016/j.inteco.2018.10.002
- Chapman, A., & Okushima, S. (2019). Engendering an inclusive low-carbon energy transition in Japan: Considering the perspectives and awareness of the energy poor. Energy Policy, 135, 111017. https://doi.org/10.1016/j.enpol.2019.111017
- Chen, Y., Cheng, L., Lee, C. C., & Wang, C. S. (2021). The impact of regional banks on environmental pollution: Evidence from China’s city commercial banks. Energy Economics, 102, 105492. https://doi.org/10.1016/j.eneco.2021.105492
- Chen, C., Koveos, P., & Zhang, Y. (2005). Economic development under political and economic asymmetries: The case of Shanghai and Taiwan. The Journal of Economic Asymmetries, 2(1), 41–57. https://doi.org/10.1016/j.jeca.2005.01.003
- Cheng, B., Dai, H., Wang, P., Xie, Y., Chen, L., Zhao, D., & Masui, T. (2016). Impacts of low-carbon power policy on carbon mitigation in Guangdong Province, China. Energy Policy, 88, 515–527. https://doi.org/10.1016/j.enpol.2015.11.006
- Cheng, C., Ren, X., Wang, Z., & Shi, Y. (2018). The impacts of non-fossil energy, economic growth, energy consumption, and oil price on carbon intensity: Evidence from a panel quantile regression analysis of EU 28. Sustainability, 10(11), 4067. https://doi.org/10.3390/su10114067
- Cheng, C., Ren, X., Wang, Z., & Yan, C. (2019). Heterogeneous impacts of renewable energy and environmental patents on CO2 emission – Evidence from the BRIICS. The Science of the Total Environment, 668, 1328–1338.
- Climate Trade. (2021). Which countries are the world’s biggest carbon polluters?. https://climatetrade.com/which-countries-are-the-worlds-biggest-carbon-polluters/ .
- Copeland, B. R., & Taylor, M. S. (2004). Trade, growth, and the environment. Journal of Economic Literature, 42(1), 7–71. https://doi.org/10.1257/.42.1.7
- Corbacho, M. A., Medina, L., & Caceres, C. (2010). Structural breaks in fiscal performance: Did fiscal responsibility laws have anything to do with them?. International Monetary Fund.
- Croissant, Y., & Millo, G. (2008). Panel data econometrics in R: The plm package. Journal of Statistical Software, 27(2), 1–43. https://doi.org/10.18637/jss.v027.i02
- Davidsdottir, B., & Fisher, M. (2011). The odd couple: The relationship between state economic performance and carbon emissions economic intensity. Energy Policy, 39(8), 4551–4562. https://doi.org/10.1016/j.enpol.2011.04.030
- Dogan, E., & Seker, F. (2016). Determinants of CO2 emissions in the European Union: The role of renewable and non-renewable energy. Renewable Energy, 94, 429–439. https://doi.org/10.1016/j.renene.2016.03.078
- Dumitrescu, E., & Hurlin, C. (2012). Testing for granger non-causality in heterogeneous panels. Economic Modelling, 29(4), 1450–1460. https://doi.org/10.1016/j.econmod.2012.02.014
- Eluwole, K. K., Saint Akadiri, S., Alola, A. A., & Etokakpan, M. U. (2020). Does the interaction between growth determinants a drive for global environmental sustainability? Evidence from world top 10 pollutant emissions countries. The Science of the Total Environment, 705, 135972.
- Ezcurra, R. (2007). Is there cross-country convergence in carbon emissions? Energy Policy, 35(2), 1363–1372. https://doi.org/10.1016/j.enpol.2006.04.006
- Fang, J., Gozgor, G., Mahalik, M. K., Mallick, H., & Padhan, H. (2022). Does urbanisation induce renewable energy consumption in emerging economies? The role of education in energy switching policies. Energy Economics, 111, 106081. https://doi.org/10.1016/j.eneco.2022.106081
- Fang, Z., Gao, X., & Sun, C. (2020). Do financial development, urbanization and trade affect environmental quality? Evidence from China. Journal of Cleaner Production, 259, 120892. https://doi.org/10.1016/j.jclepro.2020.120892
- Fankhauser, S., Bowen, A., Calel, R., Dechezleprêtre, A., Grover, D., Rydge, J., & Sato, M. (2013). Who will win the green race? In search of environmental competitiveness and innovation. Global Environmental Change, 23(5), 902–913. https://doi.org/10.1016/j.gloenvcha.2013.05.007
- Fernandez, J. M. R., Payán, M. B., Santos, J. M. R., & García, Á. L. T. (2016). Renewable generation versus demand-side management. A Comparison for the Spanish Market. Energy Policy, 96, 458–470.
- Fortune. (2021). Reducing carbon emissions is important. But tackling carbon intensity might be even more. https://fortune.com/2021/06/25/carbon-intensity-emissions-climate-change-paris-agreement/.
- Fox, J., & Weisberg, S. (2019). TETO & sage., C. An {R} Companion to Applied Regression (3rd ed.). Sage.
- Freire, C. (2021). Technological transformation and innovation for economic diversification and structural transformation in CDDCs. United Nations conference on trade and development https://unctad.org/system/files/non-official-document/DITC_COM_2021_D_BN03_en. pdf.
- Geels, F. W. (2018). Disruption and low-carbon system transformation: Progress and new challenges in socio-technical transitions research and the multi-level perspective. Energy Research & Social Science, 37, 224–231.
- Golub, A., & Toman, M. (2016). Climate change, industrial transformation, and environmental growth traps. Environmental and Resource Economics, 63(2), 249–263. https://doi.org/10.1007/s10640-015-9949-2
- Greening, L. A. (2004). Effects of human behavior on aggregate carbon intensity of personal transportation: Comparison of 10 OECD countries for the period 1970–1993. Energy Economics, 26(1), 1–30. https://doi.org/10.1016/j.eneco.2003.05.001
- Grossman, G., & Krueger, A. (1993). Environmental impacts of the North American Free Trade Agreement. In: P. Garber (Ed.), The U.S. – Mexico Free Trade Agreement (pp. 13–56). MIT Press.
- Grossman, G. M., & Krueger, A. B. (1995). Economic growth and the environment. The Quarterly Journal of Economics, 110(2), 353–377. https://doi.org/10.2307/2118443
- Hansen, L. P. (1982). Large sample properties of generalized method of moments estimators. Econometrica, 50(4), 1029–1054. https://doi.org/10.2307/1912775
- Herrendorf, B., Rogerson, R., & Valentinyi, A. (2014). Growth and structural transformation. Handbook of Economic Growth, 2, 855–941.
- Hettige, H., Mani, M., & Wheeler, D. (2000). Industrial pollution in economic development: The environmental Kuznets curve revisited. Journal of Development Economics, 62(2), 445–476. https://doi.org/10.1016/S0304-3878(00)00092-4
- Horobet, A., Popovici, O. C., Zlatea, E., Belascu, L., Dumitrescu, D. G., & Curea, S. C. (2021). Long-run dynamics of gas emissions, economic growth, and low-carbon energy in the European Union: The fostering effect of FDI and trade. Energies, 14(10), 2858. https://doi.org/10.3390/en14102858
- Horobet, A., Tudor, C. D., Belascu, L., & Dumitrescu, D. G. (2022). The role of distinct electricity sources on pollution abatement: Evidence from a wide global panel. Frontiers in Environmental Science, 10, 996515. https://doi.org/10.3389/fenvs.2022.996515
- Hu, H., Xie, N., Fang, D., & Zhang, X. (2018). The role of renewable energy consumption and commercial services trade in carbon reduction: evidence from 25 developing countries. Applied Energy, 211, 1229–1244. https://doi.org/10.1016/j.apenergy.2017.12.019
- International Environment Agency (IEA). (2022). Global energy review: CO2 emissions in 2021. IEA. https://www.iea.org/reports/global-energy-review-co2-emissions-in-2021-2
- International Environment Agency (IEA). (2017). Electricity information. https://www.iea.org/data-and-statistics/data-product/electricity-information
- Jaller, M., & Matthews, S. (2021). Can carbon intensity metrics help achieve net carbon reductions?. https://faculty.engineering.ucdavis.edu/jaller/wp-content/uploads/sites/298/2021/06/92021-Jaller-and-Matthews-Can-Carbon-Intensity-Metrics-Help-Achieve-Net-Carbon-Reductions.pdf
- Kaya, Y., & Yokobori, K. (Eds.). (1997). Environment, energy, and economy: Strategies for sustainability (pp. 16–26). United Nations University Press.
- Kalair, A., Abas, N., Saleem, M. S., Kalair, A. R., & Khan, N. (2021). Role of energy storage systems in energy transition from fossil fuels to renewables. Energy Storage, 3(1), e135. https://doi.org/10.1002/est2.135
- Khan, I. (2019). Temporal carbon intensity analysis: Renewable versus fossil fuel dominated electricity systems. Energy Sources, Part A: Recovery, Utilization, and Environmental Effects, 41(3), 309–323.
- Khomenko, S., Cirach, M., Pereira-Barboza, E., Mueller, N., Barrera-Gómez, J., Rojas-Rueda, D., de Hoogh, K., Hoek, G., & Nieuwenhuijsen, M. (2021). Premature mortality due to air pollution in European cities: A health impact assessment. The Lancet Planetary Health, 5(3), e121–e134. https://doi.org/10.1016/S2542-5196(20)30272-2
- Konstantakopoulou, I. (2022). Does health quality affect tourism? Evidence from system GMM estimates. Economic Analysis and Policy, 73, 425–440. https://doi.org/10.1016/j.eap.2021.12.007
- Kripfganz, S., & Schwarz, C. (2019). Estimation of linear dynamic panel data models with time‐invariant regressors. Journal of Applied Econometrics, 34(4), 526–546. https://doi.org/10.1002/jae.2681
- Kuznets, S. (1955). Economic growth and income inequality. American Economic Review, 45, 1–28.
- Jaller, M., & Matthews, S. (2021). Can carbon intensity metrics help achieve net carbon reductions? White paper, UC Davis. https://faculty.engineering.ucdavis.edu/jaller/wp-content/uploads/sites/298/2021/06/92021-Jaller-and-Matthews-Can-Carbon-Intensity-Metrics-Help-Achieve-Net-Carbon-Reductions.pdf
- Le, Quéré, C., Korsbakken, J. I., Wilson, C., Tosun, J., Andrew, R., Andres, R. J., & van Vuuren, D. P. (2019). Drivers of declining CO2 emissions in 18 developed economies. Nature Climate Change, 9(3), 213–217.
- Le, Quéré, C., Peters, G. P., Friedlingstein, P., Andrew, R. M., Canadell, J. G., Davis, S. J., & Jones, M. W. (2021). Fossil CO2 emissions in the post-COVID-19 era. Nature Climate Change, 11(3), 197–199.
- Leszczensky, L., & Wolbring, T. (2022). How to deal with reverse causality using panel data? Recommendations for researchers based on a simulation study. Sociological Methods & Research, 51(2), 837–865. https://doi.org/10.1177/0049124119882473
- Li, J., Ding, H., Hu, Y., & Wan, G. (2021). Dealing with dynamic endogeneity in international business research. Journal of International Business Studies, 52(3), 339–362. https://doi.org/10.1057/s41267-020-00398-8
- Li, W., Wang, W., Wang, Y., & Qin, Y. (2017). Industrial structure, technological progress and CO2 emissions in China: Analysis based on the STIRPAT framework. Natural Hazards, 88(3), 1545–1564. https://doi.org/10.1007/s11069-017-2932-1
- Li, W., Yang, G., Li, X., Sun, T., & Wang, J. (2019). Cluster analysis of the relationship between carbon emissions and economic growth. Journal of Cleaner Production, 225, 459–471. https://doi.org/10.1016/j.jclepro.2019.03.220
- Lin, Q. G., Huang, G. H., & Bass, B. (2011). Impacts from climate change and adaptation responses on energy economy and GHG emissions in the Toronto-Niagara Region, Canada. Energy Sources, Part A: Recovery, Utilization and Environmental Effects, 33(17), 1581–1597. https://doi.org/10.1080/15567036.2011.554696
- Ling, Y., Xia, S., Cao, M., He, K., Lim, M. K., Sukumar, A., Yi, H., & Qian, X. (2021). Carbon emissions in China’s thermal electricity and heating industry: An input-output structural decomposition analysis. Journal of Cleaner Production, 329, 129608. https://doi.org/10.1016/j.jclepro.2021.129608
- Ma, X., Wang, C., Dong, B., Gu, G., Chen, R., Li, Y., Zou, H., Zhang, W., & Li, Q. (2019). Carbon emissions from energy consumption in China: Its measurement and driving factors. The Science of the Total Environment, 648, 1411–1420.
- Markaki, M., Papadakis, S., & Putnová, A. (2021). A modern industrial policy for the Czech Republic: Optimizing the structure of production. Mathematics, 9(23), 3095. https://doi.org/10.3390/math9233095
- Molyneaux, L., Foster, J., & Wagner, L. (2010). Is there a more effective way to reduce carbon emissions? Energy and management group. School of Economics, University of Queensland.
- Murshed, M., Haseeb, M., & Alam, M. (2022). The environmental Kuznets curve hypothesis for carbon and ecological footprints in South Asia: The role of renewable energy. GeoJournal, 87(3), 2345–2372. https://doi.org/10.1007/s10708-020-10370-6
- Nathaniel, S. P., Alam, M., Murshed, M., Mahmood, H., & Ahmad, P. (2021). The roles of nuclear energy, renewable energy, and economic growth in the abatement of carbon emissions in the G7 countries. Environmental Science and Pollution Research, 28(35), 47957–47972. https://doi.org/10.1007/s11356-021-13728-6
- Natural Resources Defense Council (NRDC). (2021). Paris Climate Agreement: Everything you need to know. https://www.nrdc.org/stories/paris-climate-agreement-everything-you-need-know,
- Nguyen, K. H., & Kakinaka, M. (2019). Renewable energy consumption, carbon emissions, and development stages: Some evidence from panel cointegration analysis. Renewable Energy, 132, 1049–1057. https://doi.org/10.1016/j.renene.2018.08.069
- Omri, A., & Nguyen, D. K. (2014). On the determinants of renewable energy consumption: International evidence. Energy, 72, 554–560. https://doi.org/10.1016/j.energy.2014.05.081
- Oseni, I. O. (2016). Exchange rate volatility and private consumption in Sub-Saharan African countries: A system-GMM dynamic panel analysis. Future Business Journal, 2(2), 103–115. https://doi.org/10.1016/j.fbj.2016.05.004
- Oshiro, K., Masui, T., & Kainuma, M. (2018). Transformation of Japan’s energy system to attain net-zero emission by 2050. Carbon Management, 9(5), 493–501. https://doi.org/10.1080/17583004.2017.1396842
- Papke, L. E., & Wooldridge, J. M. (2005). A computational trick for delta-method standard errors. Economics Letters, 86(3), 413–417. https://doi.org/10.1016/j.econlet.2004.07.022
- Pesaran, M. H. (2004). General diagnostic tests for cross section dependence in panels (IZA Discussion Paper No. 1240). Institute for the Study of Labor (IZA).
- Pesaran, M. H. (2007). A simple panel unit root test in the presence of cross‐section dependence. Journal of Applied Econometrics, 22(2), 265–312. https://doi.org/10.1002/jae.951
- Pesaran, M. H. (2021). General diagnostic tests for cross-sectional dependence in panels. Empirical Economics, 60(1), 13–50. https://doi.org/10.1007/s00181-020-01875-7
- Peters, G. P., Andrew, R. M., Canadell, J. G., Fuss, S., Jackson, R. B., Korsbakken, J. I., Le Quéré, C., & Nakicenovic, N. (2017). Key indicators to track current progress and future ambition of the Paris Agreement. Nature Climate Change, 7(2), 118–122. https://doi.org/10.1038/nclimate3202
- Pincheira, R., & Zuniga, F. (2021). Environmental Kuznets curve bibliographic map: A systematic literature review. Accounting & Finance, 61(S1), 1931–1956. https://doi.org/10.1111/acfi.12648
- Rafiq, S., Salim, R., & Nielsen, I. (2016). Urbanization, openness, emissions, and energy intensity: A study of increasingly urbanized emerging economies. Energy Economics, 56, 20–28. https://doi.org/10.1016/j.eneco.2016.02.007
- Rahman, M. M., Sultana, N., & Velayutham, E. (2022). Renewable energy, energy intensity and carbon reduction: Experience of large emerging economies. Renewable Energy, 184, 252–265. https://doi.org/10.1016/j.renene.2021.11.068
- Rawtani, D., Gupta, G., Khatri, N., Rao, P. K., & Hussain, C. M. (2022). Environmental damages due to war in Ukraine: A perspective. The Science of the Total Environment, 850, 157932. https://doi.org/10.1016/j.scitotenv.2022.157932
- Rodrik, D. (2009). Industrial policy: Don’t ask why, ask how. Middle East Development Journal, 1(1), 1–29. https://doi.org/10.1142/S1793812009000024
- Roodman, D. (2009). How to do xtabond2: An introduction to difference and system GMM in Stata. The Stata Journal: Promoting Communications on Statistics and Stata, 9(1), 86–136. https://doi.org/10.1177/1536867X0900900106
- Ruffing, K. (2007). Indicators to measure decoupling of environmental pressure from economic growth. Sustainability Indicators: A Scientific Assessment, 67, 211.
- Saidi, K., & Omri, A. (2020a). Reducing CO2 emissions in OECD countries: Do renewable and nuclear energy matter? Progress in Nuclear Energy, 126, 103425. https://doi.org/10.1016/j.pnucene.2020.103425
- Saidi, K., & Omri, A. (2020b). The impact of renewable energy on carbon emissions and economic growth in 15 major renewable energy-consuming countries. Environmental Research, 186, 109567. https://doi.org/10.1016/j.envres.2020.109567
- Sakyi, D., & Immurana, M. (2021). Seaport efficiency and the trade balance in Africa. Maritime Transport Research, 2, 100026. https://doi.org/10.1016/j.martra.2021.100026
- Sargan, J. D. (1958). The estimation of economic relationships using instrumental variables. Econometrica, 26(3), 393–415. https://doi.org/10.2307/1907619
- Sarkodie, S. A., & Strezov, V. (2019). Effect of foreign direct investments, economic development and energy consumption on GHG emissions in developing countries. Science of the Total Environment, 646, 862–871. https://doi.org/10.1016/j.scitotenv.2018.07.365
- Schipper, L., Murtishaw, S., Khrushch, M., Ting, M., Karbuz, S., & Unander, F. (2001). Carbon emissions from manufacturing energy use in 13 IEA countries: Long-term trends through 1995. Energy Policy, 29(9), 667–688. https://doi.org/10.1016/S0301-4215(00)00163-4
- Shahbaz, M., Ozturk, I., Afza, T., & Ali, A. (2013). Revisiting the environmental Kuznets curve in a global economy. Renewable and Sustainable Energy Reviews, 25, 494–502. https://doi.org/10.1016/j.rser.2013.05.021
- Shahbaz, M., & Sinha, A. (2019). Environmental Kuznets curve for CO2 emissions: A literature survey. Journal of Economic Studies, 46(1), 106–168. https://doi.org/10.1108/JES-09-2017-0249
- Sharif, A., Baris-Tuzemen, O., Uzuner, G., Ozturk, I., & Sinha, A. (2020). Revisiting the role of renewable and non-renewable energy consumption on Turkey’s ecological footprint: Evidence from Quantile ARDL approach. Sustainable Cities and Society, 57, 102138. https://doi.org/10.1016/j.scs.2020.102138
- Smeets, E., & Weterings, R. (1999). Environmental indicators: Typology and overview. European Environmental Agency.
- Soytas, U., Sari, R., & Ewing, B. T. (2007). Energy consumption, income, and carbon emissions in the United States. Ecological Economics, 62(3–4), 482–489. https://doi.org/10.1016/j.ecolecon.2006.07.009
- Su, C. W., Xie, Y., Shahab, S., Faisal, C. M. N., Hafeez, M., & Qamri, G. M. (2021). Towards achieving sustainable development: Role of technology innovation, technology adoption and CO2 emission for BRICS. International Journal of Environmental Research and Public Health, 18(1), 277. https://doi.org/10.3390/ijerph18010277
- Sun, H., Edziah, B. K., Sun, C., & Kporsu, A. K. (2019). Institutional quality, green innovation and energy efficiency. Energy Policy, 135, 111002. https://doi.org/10.1016/j.enpol.2019.111002
- Taconet, N., Méjean, A., & Guivarch, C. (2020). Influence of climate change impacts and mitigation costs on inequality between countries. Climatic Change, 160(1), 15–34. https://doi.org/10.1007/s10584-019-02637-w
- Tavakoli, A. (2017). How precisely "kaya identity" can estimate GHG emissions: A global review. Jordan Journal of Earth and Environmental Sciences, 8(2), 91–96.
- Teignier, M. (2018). The role of trade in structural transformation. Journal of Development Economics, 130, 45–65. https://doi.org/10.1016/j.jdeveco.2017.09.002
- Tnani, M. (2018). Relationships between economic growth, CO2 emissions, and innovation for nations with the highest patent applications. Environmental Economics, 9(2), 47–69. https://doi.org/10.21511/ee.09(2).2018.04
- Tudor, C. (2022). The nexus between pollution and obesity and the magnifying role of media consumption: International evidence from GMM systems estimates. International Journal of Environmental Research and Public Health, 19(16), 10260. https://doi.org/10.3390/ijerph191610260
- Tudor, C., & Sova, R. (2021). Benchmarking GHG emissions forecasting models for Global Climate Policy. Electronics, 10(24), 3149. https://doi.org/10.3390/electronics10243149
- Tugcu, C. T. (2018). Panel data analysis in the energy-growth nexus (EGN). In Angeliki N. Menegaki (Ed.), The economics and econometrics of the energy-growth nexus (pp. 255–271). Academic Press.
- Uchiyama, K. (2016). Environmental Kuznets curve hypothesis and carbon emissions. Springer.
- Ullah, S., Akhtar, P., & Zaefarian, G. (2018). Dealing with endogeneity bias: The generalized method of moments (GMM) for panel data. Industrial Marketing Management, 71, 69–78. https://doi.org/10.1016/j.indmarman.2017.11.010
- United Nations Framework Convention on Climate Change (UNFCCC). (2021). The Paris Agreement. https://unfccc.int/process-and-meetings/the-paris-agreement/the-paris-agreement.
- Urban, F., & Nordensvärd, J. (2018). Low carbon energy transitions in the Nordic countries: Evidence from the environmental Kuznets curve. Energies, 11(9), 2209. https://doi.org/10.3390/en11092209
- Usman, M., & Hammar, N. (2021). Dynamic relationship between technological innovations, financial development, renewable energy, and ecological footprint: fresh insights based on the STIRPAT model for Asia Pacific Economic Cooperation countries. Environmental Science and Pollution Research, 28(12), 15519–15536. https://doi.org/10.1007/s11356-020-11640-z
- Vuuren, D. P. V., Stehfest, E., Gernaat, D. E. H. J., Van Den Berg, M., Bijl, D. L., De, Boer., & Daioglou, V. H. S (2018). Alternative pathways to the 1.5 C target reduce the need for negative emission technologies. Nature Climate Change, 8(May), 1–7.
- Wang, Q., & Zhang, F. (2021). The effects of trade openness on decoupling carbon emissions from economic growth–evidence from 182 countries. Journal of Cleaner Production, 279, 123838. https://doi.org/10.1016/j.jclepro.2020.123838
- Wang, Q., Zhang, C., & Li, R. (2022). Towards carbon neutrality by improving carbon efficiency-A system-GMM dynamic panel analysis for 131 countries’ carbon efficiency. Energy, 258, 124880. https://doi.org/10.1016/j.energy.2022.124880
- Wang, Y., Liu, Y., & Gu, B. (2022). COP26: Progress, challenges, and outlook. Advances in Atmospheric Sciences, 39(8), 1209–1216. https://doi.org/10.1007/s00376-022-2097-z
- Wang, Z., Jebli, M. B., Madaleno, M., Doğan, B., & Shahzad, U. (2021). Does export product quality and renewable energy induce carbon emissions: Evidence from leading complex and renewable energy economies. Renewable Energy, 171, 360–370. https://doi.org/10.1016/j.renene.2021.02.066
- Wilson, I., Grant, A., & Staffell, I. (2018). Rapid fuel switching from coal to natural gas through effective carbon pricing. Nature Energy, 3(5), 365–372. https://doi.org/10.1038/s41560-018-0109-0
- Windmeijer, F. (2005). A finite sample correction for the variance of linear efficient two-step GMM estimators. Journal of Econometrics, 126(1), 25–51. https://doi.org/10.1016/j.jeconom.2004.02.005
- Wooldridge, J. M. (2010). Econometric analysis of cross-section and panel data (2nd ed.). MIT Press.
- World Bank. (2022). New World Bank country classifications by income level: 2022–2023. https://blogs.worldbank.org/opendata/new-world-bank-country-classifications-income-level-2022-2023
- World Economic Forum. (2020). This is the global economic cost of air pollution. https://www.weforum.org/agenda/2020/02/the-economic-burden-of-air-pollution.
- World Economic Forum. (2022). Why we need to invest in air quality. https://www.weforum.org/agenda/2022/08/why-we-need-to-invest-in-air-quality/.
- World Health Organization (WHO). (2019). Ten threats to global health in 2019. https://www.who.int/vietnam/news/feature-stories/detail/ten-threats-to-global-health-in-2019.
- Wu, R., Dong, J., Zhou, L., & Zhang, L. (2018). Regional distribution of carbon intensity and its driving factors in China: An empirical study based on provincial data. Polish Journal of Environmental Studies, 27(3), 1331–1341. https://doi.org/10.15244/pjoes/76364
- Xia, Q., Wang, H., Liu, X., & Pan, X. (2021). Drivers of global and national CO2 emissions changes 2000–2017. Climate Policy, 21(5), 604–615. https://doi.org/10.1080/14693062.2020.1864267
- Yamaji, K., Matsuhashi, R., Nagata, Y., & Kaya, Y. (1993). A study on economic measures for CO2 reduction in Japan. Energy Policy, 21(2), 123–132. https://doi.org/10.1016/0301-4215(93)90134-2
- Yang, M., & Tang, W. (2022). Air pollution, political costs, and earnings management. Emerging Markets Review, 51, 100867. https://doi.org/10.1016/j.ememar.2021.100867
- York, R. (2016). Decarbonizing the energy supply may increase energy demand. Sociology of Development, 2(3), 265–272. https://doi.org/10.1525/sod.2016.2.3.265
- Yu, S., Hu, X., Li, L., & Chen, H. (2020). Does the development of renewable energy promote carbon reduction? Evidence from Chinese Provinces. Journal of Environmental Management, 268, 110634.
- Zafar, M. W., Zaidi, S. A. H., Sinha, A., Gedikli, A., & Hou, F. (2019). The role of stock market and banking sector development, and renewable energy consumption in carbon emissions: Insights from G-7 and N-11 countries. Resources Policy, 62, 427–436. https://doi.org/10.1016/j.resourpol.2019.05.003
- Zehner, O. (2012). Green illusions: The dirty secrets of clean energy and the future of environmentalism. University of Nebraska Press.
- Zhang, X. P., & Cheng, X. M. (2009). Energy consumption, carbon emissions, and economic growth in China. Ecological Economics, 68(10), 2706–2712. https://doi.org/10.1016/j.ecolecon.2009.05.011
- Zheng, X., Wang, R., & Du, Q. (2020). How does industrial restructuring influence carbon missions: City-level evidence from China. Journal of Environmental Management, 276, 111093. https://doi.org/10.1016/j.jenvman.2020.111093
- Zhu, H., Duan, L., Guo, Y., & Yu, K. (2016). The effects of FDI, economic growth and energy consumption on carbon emissions in ASEAN-5: Evidence from panel quantile regression. Economic Modelling, 58, 237–248. https://doi.org/10.1016/j.econmod.2016.05.003