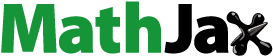
ABSTRACT
Using the US Survey of Consumer Finances, we explore the empirical relationship between borrowing constraints and financial portfolio allocation by American households. To help motivate our empirical analysis we initially draw insights from a mean-variance model of optimal portfolio allocation with three tradable asset classes defined by increasing risk, and establish a link between borrowing restrictions and portfolio allocation in the presence of background risk. Our main contribution, however, lies in estimating the role that borrowing constraints play in shaping households' financial portfolio allocation. This is achieved using an ordered fractional probit model. In addition to revealing the significant empirical role played by household borrowing constraints in determining portfolio allocation, our analysis helps us to resolve ambiguity surrounding the behaviour of the medium-risk asset in our motivational theoretical framework. Further, the empirical distribution of medium-risk assets is found to be remarkably similar to that for high-risk assets, suggesting the presence of a more general ‘risk puzzle’, which our borrowing constraints measures partially explain.
1. Introduction
The borrowing constraints faced by governments, firms, and households can play a significant role in shaping economic behaviour and influencing economic outcomes, both at the microeconomic and macroeconomic level. Significantly, the presence of such constraints has been shown to hold implications for the sustainability of government deficits (Wilcox Citation1989), the behaviour of output and prices (Scheinkman and Weiss Citation1986), and consumption behaviour (Carroll Citation2001). Borrowing constraints have also been shown to affect the composition of a household's financial portfolio (see, for instance: Bucciol, Miniaci, and Pastorello Citation2017; Guiso, Jappelli, and Terlizzese Citation1996; Haliassos and Hassapis Citation1999).
Using data from the United States Survey of Consumer Finances (SCF) from 1995 to 2019, this contribution investigates the role that borrowing constraints play in determining the composition of US households' financial portfolios. Significantly, our empirical investigation is supported by insights from a mean-variance model of optimal portfolio allocation with a basis in Markowitz (Citation1952), which as elaborated on in more detail below, helps to motivate our ensuing econometric analysis. More generally, we stress here that from a public policy perspective, investigating the role of financial portfolio allocation in US households is not just of academic interest. Since the early 1990s, the United States has witnessed increased efforts to provide additional borrowing opportunities for households that were traditionally constrained by credit markets (Lyons Citation2003). The effectiveness of such efforts takes on additional significance when considered against the backdrop of a more recent report published by the Federal Reserve Bank of New York (Hamdani et al. Citation2019), which found that for the fourth quarter of 2018, over sixty million US adults were unlikely to access credit at choice, and noted ‘…wide heterogeneity of credit conditions at the state and county levels in America’ (Hamdani et al. Citation2019, 12).Footnote1 Given the high incidence of borrowing-constrained US households that persists to this day, exploring the extent to which access to borrowing affects household portfolios is thus particularly apposite.Footnote2
Our mean-variance model of optimal portfolio choice, in which households are assumed to face short-selling constraints as in Jappelli, Julliard, and Pagano (Citation2010), provides a useful starting point for our main empirical analysis by establishing a link between borrowing constraints and asset allocation at the household level. However, as in Eichner and Wagener (Citation2012), we also consider a role for so-called ‘background risk’ which assumes the form of non-tradable background wealth which is assumed to be exogenous and uninsurable. Such risk corresponds to shocks that may impact on the stream of household income associated with labour or entrepreneurial activity, human capital or government transfers.Footnote3 The most notable feature of our motivational theoretical framework is that we explicitly model the behaviour of a medium-risk asset class, which reflects the existing literature's taxonomy of a household's financial assets into three distinct risk-based categories: low-risk; medium-risk; and high-risk.Footnote4 Households exploit the information contained in the background risk covariance structures, which we demonstrate has implications for household portfolio diversification and risk reduction. Whereas the mean-variance framework often has clear behavioural implications for the optimal allocation of the household's low-risk and high-risk assets, the implied behaviour of the medium-risk asset is more nuanced. Numerical predictions of our model using plausible values of the first and second moments of our three asset categories, are indicative of lower levels of risk aversion being associated with higher shares of both medium-risk and high-risk assets. Support for this finding is found in our ensuing econometric analysis. Our numerical exercise also suggests that when modelling the impact of borrowing constraints empirically, it is appropriate to account for the possible presence of background risk.Footnote5
Turing to the main contribution of our paper, the emphasis placed on asset shares in the mean-variance framework described above is explicitly reflected in our econometric strategy, in which the impact of borrowing constraints on our three empirically observable asset shares is directly estimated. Specifically, we use the ordered fractional model (hereafter ‘OF’) model of Kawasaki and Lichtenberg (Citation2014), which enables us to impose an inherent ordering on the fraction of assets held in each risk category. This estimation approach contrasts with the majority of contributions, where the propensity to hold only a single asset type is modelled using, for example, censored or fractional regression techniques (see inter alia, Cardak and Wilkins Citation2009; Edwards Citation2008; Rosen and Wu Citation2004). We thus consider the impact of borrowing constraints on household financial risk taking in a broader sense, which extends beyond accounting for the propensity of households to directly invest in stocks. In doing so we build on contributions to the ‘stockholding puzzle’ literature in which assets are categorised as being either ‘safe’ or ‘risky’ (see for instance: Bertaut Citation1998; Fratantoni Citation2001; Haliassos and Bertaut Citation1995) and explore the extent to which an avoidance of stockholding is part of a more general ‘risk puzzle’ that characterises household portfolio allocation. Here, exploring the determinants of medium-risk asset holding is of particular interest given that this asset class is classified as including retirement funds and life insurance policies. Holding such financial products may help to avoid shortfalls in retirement income, or shield household members from catastrophic financial shocks – such as the inability to maintain mortgage payments due to the death of a breadwinner. In this regard, the consequences of shunning medium-risk assets may in practice be far more damaging to households than not directly holding stocks. We now turn to the literature.
2. Literature
Our contribution builds on an established empirical literature, of which notable early contributions include Jappelli (Citation1990), Jappelli, Pischke, and Souleles (Citation1998) and Haliassos and Michaelides (Citation2003). Jappelli (Citation1990) exploits information contained in the 1983 SCF to construct a liquidity constraint measure based on whether the household has been rejected for a request for credit from a financial institution. The study explores the type of households who are credit constrained and finds race, wealth, income and family composition all impact the probability of being constrained. Jappelli, Pischke, and Souleles (Citation1998) exploit data from both the SCF and the United States Panel Study of Income Dynamics (PSID) and use a two-stage two-sample least squares approach. Using information in the SCF, liquidity constraint measures are constructed; these measures are used to impute the probability of liquidity constraints in the PSID data in order to estimate Euler equations. Haliassos and Michaelides (Citation2003) theoretically explore household portfolio allocation in the presence of liquidity constraints. The authors study an infinite-horizon model of portfolio allocation under liquidity constraints to explore the portfolio specialisation puzzle, and report that relatively small fixed stock market entry costs are sufficient to deter households from participating in the stock market.
More recent studies that are related to our contribution include Carroll, Holm, and Kimball (Citation2021), Toussaint-Comeau (Citation2021) and Insler, Compton, and Schmitt (Citation2016). Carroll, Holm, and Kimball (Citation2021) explore the interactions between precautionary saving and liquidity constraints in a theoretical setting, where they allow liquidity constraints and risk to influence so-called consumption concavity. Their results suggest that the introduction of risks or liquidity constraints affects the concavity of the consumption function, which in turn changes the precautionary saving motive. The effect of introducing an additional constraint or risk on precautionary saving depends on whether it is correlated with pre-existing constraints or risks, and the way in which an additional constraint interacts with existing constraints can for some levels of wealth reduce the precautionary saving motive. Analysing the SCF, Toussaint-Comeau (Citation2021) explores how liquidity constraints affect the propensity to save. Liquidity constraints are found to influence a range of saving decisions. In particular, the effects of liquidity constraints are amplified by both high-debt payments and high interest rate credit cards, which serve to reduce savings. In a related study, Insler, Compton, and Schmitt (Citation2016) analyse survey data relating to students at the US Naval Academy, all of whom have the opportunity to take out a Career Starter Loan (CSL). As a CSL is a significant personal loan offered at low interest rates, these young adults are not bound by they same type of liquidity constraints that are typically experienced by other individuals in this age range. The authors found that greater cognitive ability, individuals with prior investment experience, and those who viewed themselves as financially literate were found to invest more per se, and had a greater tendency to invest in riskier options.
In exploring the role of credit constraints, our study also controls for the impact of (risky) background wealth on portfolio allocation.Footnote6 Significantly, Canner, Mankiw, and Weil (Citation1997, 188), note that, ‘Human capital – in the form of future labour earnings – is probably the most important non-traded asset’.Footnote7 In this setting, Bertaut (Citation1998) and Haliassos and Bertaut (Citation1995) find that labour income risk is inversely related to holding risky assets. Related work by Fratantoni (Citation2001) reports that in addition to labour income risk, expenditure risk associated with housing has an inverse relationship with household stock holding. Koo (Citation1998) finds that investors will take a smaller fraction of risky assets in their portfolio when they have liquidity constraints and uninsurable income risk. Heaton and Lucas (Citation2000) calibrate labour income risk with personal income data, finding that both labour income risk and the positive correlation between labour income and risky asset returns tend to reduce investments in risky assets. We incorporate some of these features into our motivating theoretical model.
The remainder of the paper is organised as follows. Section 3 presents our theoretical framework, which in addition to linking household borrowing constraints and portfolio allocation, helps to motivate our empirical analysis. Section 4 describes our data, and Section 5 sets out our empirical modelling strategy. Section 6 discusses our empirical findings. Finally, Section 7 concludes.
3. Theoretical motivation
To motivate our empirical analysis, we develop a model which has a basis in the work of Markowitz (Citation1952), and is a variant of Eichner and Wagener (Citation2012), who analyse risk-taking behaviour in a linear portfolio selection problem with non-tradable background wealth.Footnote8 Assume that households face a linear portfolio choice problem such that the final wealth of a household is given by
(1)
(1) where L, M and H are random variables representing the return from allocating all of wealth to these tradable assets, which are defined as being low-risk (L), medium-risk (M), or high-risk (H).Footnote9 The three tradable assets are assumed to be increasing in risk and return, and capture the structure of US households' financial portfolios as defined in the existing literature, see for example, Carroll (Citation2002) and Hurd (Citation2002), where financial assets are classified as one of three distinct risk-based categories. The weights that households choose to allocate to these assets are denoted
,
, such that
.Footnote10 B denotes non-tradable background wealth which as noted in the introduction, can be viewed as the ‘stream of household income arising due to labour or entrepreneurial activity, human capital, and government transfers’ (Eichner and Wagener Citation2012, 424).
An exogenous parameter is used to scale background wealth B up or down; the non-negativity constraint on background wealth reflects the fact that households cannot easily borrow against their human capital in the way they can against financial assets.Footnote11 Our model is also characterised by restrictions on short-selling which lead to the portfolio weights of the household's tradable assets being subject to non-negativity constraints. This entails that the individual asset shares are characterised by
. Although short-selling is potentially attractive for households – it markedly increases the total return of a portfolio albeit at the cost of a greatly increased risk – the presence of constraints on this form of borrowing is a highly plausible assumption in the context of household finance.Footnote12 Households are unlike institutional investors, in that the former cannot fund stock market investments through borrowing from financial intermediaries such as banks. Here, Perraudin and Sorensen (Citation2000) suggest that in the context of US households, it is highly unlikely that the majority of households are characterised by optimal, unconstrained portfolios, which involve short-selling stocks; similarly, Poterba (Citation2002, 141) notes that the assumption of short-selling is ‘…probably not appropriate for a large set of households’.Footnote13
The expected return on total wealth, W, is given by
(2)
(2) where
is the mean of variable
. The corresponding variance,
, is given by
(3)
(3) In (Equation3
(3)
(3) ), the correlation coefficient between any two assets Y and Z is given by
, such that
, i = Y, Z denotes the standard deviation of a variable,
is the covariance, and
. Given space constaints, and to facilitate a more focussed analysis of the interplay between background risk and borrowing constraints, our subsequent analysis permits background wealth B to be correlated with L, M, and H, but we assume that the tradable assets are not correlated with each other. Although this does not detract from the main thrust of our analysis, it requires setting
.Footnote14. We also stress here that despite the SCF being one of the most comprehensive surveys of household finances in the United States, we do not have information about the specific stocks and assets that individual households own. Consequently, we have no information on the extent to which the low, medium and high-risk assets in our sample are correlated over time. In the absence of this information, it therefore seems appropriate to set the tradable asset correlations to zero, which is clearly beneficial in terms of keeping the motivational model parsimonious.Footnote15
Now suppose the household specifies ; by the efficient set theorem (Markowitz Citation1952) it sets out to minimise the variance of wealth,
. Appendix 1 derives the efficient frontier and asset share allocations consistent with portfolio variance minimisation, from which we make the following claim:
Claim 3.1
In the absence of short-sale constraints, the optimal shares of the tradable assets are related to on the efficient frontier according to
(4)
(4) where
,
, and
denote the variance minimising shares for low, medium and high-risk assets.
Proof.
See Appendix 1.
That is, on the efficient frontier, a higher expected portfolio return is associated with an increase (decrease) in the share of assets in the high-risk (low-risk) category. Significantly, Equation (Equation4
(4)
(4) ) shows that for the medium-risk asset, the direction of this effect on
is a function of the relationship between the ratio of the variances of the lowest risk (
) and highest risk (
) assets, and the expected returns to the three tradable assets. From an empirical perspective, this finding is of interest: even in a simple tractable framework like ours, the conditions governing the behaviour of the medium-risk asset may be subject to some ambiguity, even when the efficient frontier is unconstrained. Specifically, we find that,
(5)
(5) It is straightforward to verify that the condition in expression (Equation5
(5)
(5) ) also holds in the presence of independent background risk when the tradable assets are not correlated with each other. The results in Appendix 1 also imply that independent background risk has no impact on the composition of the minimum variance portfolio on the efficient frontier, a finding consistent with Lusk and Coble (Citation2008) and Jiang, Ma, and An (Citation2010).Footnote16 This finding is overturned when the assumption of independent background risk is relaxed.Footnote17 Moreover, Appendix 1 also demonstrates that under correlated background risk the
, i = L,M,H are all linear functions of background risk,
. This finding enables us to make the following claim:
Claim 3.2
In the presence of both correlated background risk and short-sale constraints ,
, and
, the domain of the non-binding risk-return space will also be a function of background risk.
Proof.
See Appendix 2.
As a prelude to our econometric analysis, and to explore the implications of the above results for US households, it is informative to conduct simple numerical experiments. This approach aligns with Jappelli, Julliard, and Pagano (Citation2010), who construct an efficient frontier for Italian households both with and without short-sale constraints based on historical returns data, although unlike our contribution, the impact of background risk is not considered.Footnote18 We also note here that as our low-risk category includes wealth held in savings accounts, the short-sale constraint in this category could be interpreted as a borrowing constraint in which the household is not permitted to hold negative balances. In the context of our model, this would entail households not having the ability to obtain a loan to purchase medium-risk or high-risk assets.Footnote19
Figure plots efficiency frontiers in the –
space, and demonstrates how the presence of correlated background risk can exacerbate the impact of short-sale conditions by further restricting the domain of the non-binding return-risk space available to households.Footnote20 For the tradable assets, we choose parameterisations based on historical data for the annualised returns and volatility of the S&P 500 (‘high-risk’), the Bloomberg Barclays Municipal Bond Index (‘medium-risk’), and average effective fed funds rate (‘low-risk’), for the years 1995–2019, which matches the sample period used in our empirical analysis.Footnote21 Although these proxies are arguably crude, they tentatively capture the differences in the nature of returns and volatilities associated with the SCF asset categories, which are discussed in more detail in Section 3. Our parameterisations are:
,
;
,
; and
,
. For background wealth B we set
and
, and consider two scenarios: one without background risk (
); and another where background risk is assumed to be positively correlated with the high-risk asset (
,
). Using expression (Equation5
(5)
(5) ) our parameterisations imply that
, which entails that lower levels of risk aversion (and hence a higher
) will be associated with a higher share of medium-risk assets.
Figure 1. The efficient frontier and household preferences under short-sale constraints, with () and without (
) background risk.
Notes: The following parameterisations are used: ,
;
,
;
,
;
,
;
. Black curves capture the absence of background risk (
). Grey curves correspond to the case of positively correlated background risk (
,
). For the tradable assets, our parameterisations are based on annual historical data for the years 1995–2019 corresponding to: the average effective fed funds rate (‘low-risk’); the Bloomberg Barclays Municipal Bond Index (‘medium-risk’); and the annualised returns and volatility of the S&P 500 (‘high-risk’).

To explore how background risk may affect the composition of the household's optimal portfolio, we assume that household preferences are given by the mean-variance utility function
(6)
(6) where
is a risk aversion parameter such that greater values imply lower levels of risk tolerance, and set
.Footnote22
Footnote23 Curves corresponding to the case of no background risk are labelled using a ‘
’ (black lines), whereas under positively correlated background risk, ‘
’ is used (grey lines). The dotted lines show the unconstrained efficient frontiers under each scenario, and the thicker solid lines capture the impact of the corresponding binding constraints on the respective frontiers.Footnote24 The dashed lines capture household preferences. Table reports the corresponding optimal asset allocations and the effects on household welfare.
Table 1. Optimal portfolio composition predicted by the model under alternative background risk scenarios.
In the absence of background risk (), the tangency point of household preferences with the efficient frontier corresponds to an optimal portfolio allocation spread across all three assets. Introducing background risk has the effect of shifting the efficient frontier to the right,Footnote25 and when B is positively correlated with H (
), holdings of high-risk assets fall to zero and holdings of L and M rise. Of special interest here is the impact of correlated background risk on the model's binding constraints. Figure shows that in the absence of background risk, the most risk averse households are not subject to binding constraints: for instance, at the global minimum, the optimal asset allocation is
,
, and
. As in Jappelli, Julliard, and Pagano (Citation2010), risk-loving households suffer the greatest welfare loss due to the presence of binding constraints (solid black line),Footnote26 and risk-averse households are unaffected. This finding contrasts with the case of
, where unlike in Jappelli, Julliard, and Pagano (Citation2010), the introduction of correlated background risk entails that the most risk averse households will also suffer welfare loss due to binding constraints coming into effect. Under positive correlation of the form
the point of tangency between the unconstrained efficient frontier and household preferences shown in Figure is infeasible due to
, and the optimal allocation associated with the constrained frontier is associated with zero holdings of high-risk assets.Footnote27 Households situated at both ends of the risk aversion spectrum are observed to be impacted by binding constraints.
Table also reports the results under our model parameterizations of scenarios that are not depicted in Figure , namely the cases of independent background risk, and, of negative correlation, where . In the former case, the optimal portfolio allocation is identical to that under no background risk, albeit household welfare is markedly lower. Significantly, Table shows that the presence of background risk reduces household welfare relative to the zero background risk case. Under
, households are characterised by riskier portfolios in that a much higher (lower) proportion of wealth is held in the form of high-risk (low-risk) assets.
More generally, the findings reported above are consistent with Arrondel and Calvo-Pardo (Citation2002) who find that ‘…the sign and magnitude of the correlation may exacerbate or counterbalance the optimal portfolio response to the introduction of a background risk.’ Notwithstanding our focus on the behaviour of the medium-risk asset, the confluence of borrowing constraints with background risk holds non-negligible ramifications for portfolio allocation in US households. As US households are subject to short-sale constraints in practice (Perraudin and Sorensen Citation2000; Poterba Citation2002), this suggests that when econometrically modelling the determinants of household portfolio selection, controlling for the potential effects of background risk is advisable. In this regard, our results align with a wider literature (e.g. Heaton and Lucas Citation2000) which predicts that the dual presence of borrowing constraints and background risk will affect household portfolio selection. Given the insights of our mean-variance framework, we now turn to the analysis of SCF data.
4. Data
The SCF has been used extensively in the existing literature relating to household finances, see for example, Haliassos and Bertaut (Citation1995), Browning and Lusardi (Citation1996), Bertaut (Citation1998), Spaenjers and Spira (Citation2015), and Fulford (Citation2015), amongst many others. The SCF is a repeated cross-sectional triennial survey of US households conducted on behalf of the Federal Reserve System's Board of Governors. We exploit data from the 1995–2019 waves of the SCF.Footnote28
The SCF contains detailed information on household assets and liabilities, in addition to a range of income, socioeconomic and demographic characteristics. It is notable for containing household level information on a wide range of financial assets including: transaction accounts; certificates of deposit; bonds; stocks; pooled investment funds; and retirement funds amongst others. This feature permits us to explore the household's portfolio allocation decisions. To do so, we allocate assets into three distinct risk-based categories: high-risk; medium-risk; and low-risk. This taxonomy is consistent with the three tradable asset types in our motivating theoretical model. Moreover, our asset classification is employed widely in the household finance literature, see for example, Barasinska, Schäfer, and Stephan (Citation2012), Carroll (Citation2002) and Hurd (Citation2002). The precise distinction between low, medium and high-risk assets, as used in theoretical analysis, however is not easily applicable to empirical research, as we are unable to identify the specific assets held by the household. Moreover, we do not know the returns on each individual asset and as a result defining riskiness according to the mean–variance approach is not possible.
In line with the existing literature, we construct a measure of high-risk assets that comprises of both direct and indirect risky asset holding, through for example, retirement accounts. Low-risk assets include, for example, checking accounts and certificates of deposit, while medium-risk assets include for example, non-risky pension accounts, tax mutual funds and tax free bonds. Full descriptions of these asset classes are presented in Table . As is standard in the empirical literature, we explore the ratio of these asset classes to total financial wealth in the household, that is the proportion of financial wealth allocated to each asset class.
Table 2. Variable definitions.
In our empirical specifications, we control for a wide range of demographic and socioeconomic characteristics which are based on the existing literature. These include: age; gender; race; the presence of children in the household; education; and labour market status. We also control for measures capturing risk attitudes, income (and wider economic) expectations in the coming years, home ownership and the household's saving horizon. A full description of these variables and corresponding summary statistics are provided in Table .
Moreover, in line with our theoretical motivation, we control for a set of variables which capture the household's exposure to a range of background risks. These draw on the existing literature and include risks associated with income, health, expenditure, business ownership, wealth shocks associated with future inheritance and whether there are a multiple earners in the household. Income risk is captured by two variables: (i) if the household has a reasonable idea of what the household's income will be in the future; and (ii) if the household's previous annual income was above or below what was expected.Footnote29 Health risk is captured by the insurance cover of the household, whilst expenditure risk is captured by whether major financial expenses are expected in the next five years. A full description of these variables is also given in Table .
4.1. Measuring borrowing constraints
The key aim of this paper is to explore the impact of borrowing constraints on portfolio allocation decisions. In the literature more broadly, the term liquidity constrained is used to refer to households who are credit or borrowing constrained, see for example, Guiso and Paiella (Citation2008), Le Blanc et al. (Citation2015) and Campbell and Hercowitz (Citation2019). Generally, households are defined to be borrowing constrained if they are unable to borrow the amount they asked for or they have been charged a higher interest rate than the ‘market clearing’ interest rate.
Previous studies have exploited a range of constraint measures to test the validity of the Life-Cycle/Permanent Income Hypothesis models (see for example, Hall and Mishkin Citation1982; Hayashi Citation1985; Zeldes Citation1989). A major limitation of these studies is that constrained households are identified via indirect evidence, such as the household's wealth to income ratio or the household's saving rate. Subsequent studies have used more direct measures of such constraints, which are revealed directly from survey questions.
The SCF includes information which enables us to directly observe constrained households, in line with Jappelli (Citation1990). A full description of these measures is given in Table , whilst the corresponding summary statistics are presented in Table . We use information on whether households have been turned down for credit at a financial institution or if they did not apply because they believed they would be turned down for credit in order to define a range of borrowing constraint measures. We incorporate information based on whether the household did not apply for credit due to a belief they would be turned down, in addition to actually being turned down for credit, since, as argued in Jappelli (Citation1990), if there is a cost to apply, this subjective likelihood of being turned down may not apply initially due to the likelihood they will be refused credit.
Table 3. Borrowing constraint measures.
Table 4. Summary statistics.
Initially, we exploit information on whether the household chose not to apply for credit based on a belief that it might be rejected (Constraint 1). Specifically, this form of constraint is associated with the response to the question: ‘Was there any time in the past 12 months that you thought of applying for credit at a particular place, but changed your mind because you thought you might be turned down?’ We construct a binary variable which assumes a value of 1 if the response was ‘yes’, and 0 if ‘no’. Table shows that 14% of the households in our sample did not apply for credit in the past 12 months for this reason.
Our second constraint measure (Constraint 2) is based on the question: ‘In the past twelve months, has a particular lender or creditor turned down any request you (or your husband/wife/partner) made for credit, or not given you as much credit as you applied for?’Footnote30 Respondents can respond in three ways: Yes, turned down; Yes, not as much credit; or No. A household is defined to be constrained if they respond either ‘turned down’ or did not receive as much credit as applied for. Households are not constrained if they respond ‘no’. In our sample, 25% are defined to be constrained according to this measure.
Our next measure augments the above with information contained in the following question: ‘Were you later able to obtain the full amount you requested by reapplying to the same institution or by applying elsewhere?’ If a household was subsequently able to obtain the full amount applied for, they are defined as not being constrained in our third borrowing constraint measure (Constraint 3). As presented in Table , once we account for households who were able to obtain credit by reapplying (either at the same institution or else where), the proportion of constrained households falls to 16%, as compared to Constraint 2.
Finally, in the spirit of Jappelli (Citation1990) we combine the above questions relating to being turned down and not applying due to a belief of being turned down. Specifically, we construct binary variables which capture if a household has not obtained credit due to either not applying for credit or if they have been unsuccessful in their credit application (Constraints 4 and 5). The proportions of households defined to be constrained when we account for both being discouraged and turned down are 29% and 20% for Constraints 4 and 5, respectively.
We restrict our analysis to the sample of households who have made, or thought about making, a credit application in recent years. We argue that, if a household has not applied for credit, then we do not observe whether they are constrained or not. This results in a sample of 27,433 households across the time period 1995–2019.
4.2. Asset share distributions
Figure presents the distributions of the dependent variables considered in our analysis, that is, the ratios of high, medium and low-risk assets to total financial wealth, whilst Figure present the distributions conditional on holding each asset category. The distributions are clearly non-normal suggesting that linear regression is not an appropriate modelling approach. It is also apparent that there are spikes at various parts of the distribution, particularly at 0 and 1. Considering the distributions shown in Figure , approximately 65% of households hold some sort of high-risk asset either directly or indirectly. The summary statistics presented in Table reveal that households hold around 33% of their financial wealth in high-risk assets, either directly or indirectly, whilst around 41% of financial assets are held in safe assets. Furthermore, the majority of households hold some form of safe asset (which is not surprising as this asset category includes checking and current accounts), with only 0.8% of households not holding any safe assets.
5. Empirical strategy
For the purposes of comparison to the existing literature, we initially consider univariate models of portfolio allocation where a single high-risk asset is the focus. In these specifications, the share of high-risk assets in a household portfolio is modelled using a univariate fractional response model (FRM) (Papke and Wooldridge Citation1996).Footnote31 For brevity, we do not present the formulations of these standard econometric techniques. Given the different underlying assumptions of the FRM approach, its consideration serves as a robustness check. Following this, we estimate the OF model which is appropriate given that our data on household asset shares is characterised by an inherent risk ordering. This estimation approach is now described below.
5.1. The ordered fractional (OF) model
We are concerned with modeling the household's financial portfolio, where a household can allocate their financial assets to three distinct categories based on their risk exposure, namely, high, medium and low. Our interest lies in modelling the share of the household's financial portfolio allocated to each type of financial asset and the partial effects of observed covariates on these. Consequently, we employ the ordered fractional model of Kawasaki and Lichtenberg (Citation2014). Recall from Section 4 that our household asset shares are represented by proportions that all lie in the domain of zero and one, sum to one, and are classified according to their riskiness. Intuitively, our choice of econometric modelling strategy is consistent with the theoretical model in Section 3, where similarly, our concern was with accounting for the behaviour of the portfolio shares of a household with three tradable assets. As described below, using an OF model is also consistent with our assumption that US households are subject to short-sale constraints.Footnote32 In our set up, we label the shares j = 0, 1, 2, such that they increase with risk as j increases, that is, j = 0 represents the low-risk category, represents medium-risk assets, and j = 2 represents the high-risk assets.Footnote33
In order to motivate the OF model, it is intuitive to relate it to the standard ordered probit (OP) model (W. Greene Citation2012). Here, agents are assumed to have an underlying latent variable, , related to observed characteristics with unknown weights
, and a random, normally distributed error term
, such that
(7)
(7) Denoting the total number of outcomes available as J (here J = 3, such that j = 0, 1, 2), the outcome j that household i chooses will depend on the relationship between
and the inherent boundary parameters in the OP model (W. H. Greene and Hensher Citation2010),
, according to
(8)
(8) Household i’s corresponding likelihood when J = 3 is
(9)
(9) where
is the standard normal cumulative distribution function. The indicator function
is such that
, where unlike an OF model, the household can be in only one of the j = 0, 1, 2 outcomes. As such, Equations (Equation7
(7)
(7) ) and (Equation9
(9)
(9) ) are not sufficient to model ordered fractional data.
What is required is the effect of covariates on
(10)
(10) where E denotes the expected value of the term in parentheses. Replacing
with
yields the likelihood function as in Kawasaki and Lichtenberg (Citation2014):
(11)
(11) Note that here the OF model is consistent with the inherent ordering, in risk, of the asset bundles (and not of the value of the shares themselves). This allocation equation is now characterised by
(12)
(12) which by construction satisfy
, and are consistent with the risk ordering of the j asset bundles in the household's portfolio. Moreover, boundary observations of zero or unity shares are also easily dealt with here. Such a model thus provides a means of modelling the household's portfolio allocations, which takes into account the inherent risk ordering of the asset categories. This empirical strategy mirrors the theoretical setup presented in Section 3.
6. Results
Prior to discussing the results relating to the OF model, we consider the univariate models of high-risk asset holding. As reported earlier, high-risk assets are defined to include both direct and indirect risky asset holding. In these specifications, we model the share of high-risk assets relative to the household's total financial assets using a univariate specification. We then discuss the results relating to the OF model.
6.1. Univariate model estimates
Tables and report the coefficients corresponding to our respective Tobit and FRM specifications in which the proportion of high-risk assets is the dependent variable. Accordingly, Tables and present the respective marginal effects for these specifications. The results are generally consistent with the existing literature (see for example, Bertaut Citation1998; Fratantoni Citation2001; Haliassos and Bertaut Citation1995; Rosen and Wu Citation2004), with variables such as income, wealth, education and ethnicity displaying a statistically significant association with risky asset holding.
Our analysis also reveals that there is an inverse relationship between our borrowing constraint measures and the proportion of high-risk assets held. These results are consistent across all borrowing constraint measures. For example, the variable Constraint 1, which corresponds to an individual not applying for credit due to a belief that they would be turned down, is associated with holding a lower level of high-risk assets in both the Tobit and FRM specifications. In the latter specification, Table suggests that being constrained by this measure is associated with a reduction in the share of risky assets by 2.5%. The magnitudes across all five borrowing constraint measures are similar, irrespective of how we define being borrowing constrained or the modelling approach adopted.
6.2. Ordered fractional (OF) model estimates
We now turn to the estimates relating to the OF model, which constitutes our preferred estimation framework. As described previously, this approach entails jointly modelling the household's financial portfolio in the form of the proportion of financial wealth allocated to high, medium and low-risk asset categories. Table presents the coefficients and the marginal effects for the OF model. A positive coefficient indicates that an increase in a variable leads to households holding a greater proportion of assets in higher-risk categories. However, the precise size and direction of a variable's effect on each respective asset class is given by the reported marginal effect, which is evaluated by holding all variables at their means.
Table 5. Ordered fractional (OF) model estimates.
It is evident from Table that the impact of the standard variables on the household's portfolio allocations are generally in accordance with the univariate models and the existing literature. For example, a household whose head is white holds a higher proportion of high-risk assets in its portfolio relative to households headed by other ethnic groups, whilst having children is associated with a household holding a safer portfolio. It is notable here that households headed by individuals who are ethnically white hold a 5.0% greater share of high-risk assets and a 0.5% greater share of medium-risk assets in their financial portfolios relative to households where the head is non-white. The figures are offset by a 5.5% reduction in the share of low-risk assets. Similarly, education is strongly related to the household's portfolio composition. Relative to having below high school level education, a college education is associated with a 16.0% greater high-risk asset share, and a 17.7% lower low-risk asset share. Moreover, households whose heads are retired are associated with holding a greater proportion of high-risk assets relative to where the head of a household is not in the labour force. The marginal effects indicate that relative to this latter cohort, retired heads of household are associated with holding asset shares in high and medium-risk assets that are respectively 5.4% and 0.6% higher.
With regards to attitudes towards risk, saving horizons, and subjective economic expectations, we find relationships that align with our priors. Having a positive economic outlook and being more risk tolerant is associated with a riskier portfolio allocation. For example, a one-unit increase in the risk attitudes index is associated with an increase in the share of high-risk assets by 7.3% and a reduction in the share of low-risk assets by 8.0%.
The estimates corresponding to our background risk variables indicate that an expectation of major financial expenditure, holding no health insurance cover, receiving an inheritance, and having a good idea of what the household's income for next year, all have statistically significant impacts on portfolio composition. For example, a household with no health insurance cover is more likely to hold a safer portfolio compared to a household that is covered by such a policy. Here, the impact is sizable: the absence of health insurance cover is associated with holding an 11.1% greater share of low-risk assets, which is offset by holding a 10.0% lower share of high-risk assets, and a 1.1% lower share of medium-risk assets.
Turning our attention to the borrowing constraint measures reveals broadly similar results to the univariate specifications, in that households who are defined to be borrowing constrained hold, on average, safer financial portfolios.Footnote34 These results reveal that in addition to being associated with holding a lower share of high-risk assets, borrowing constraints lead to a reduction in the share of medium-risk assets held. Such reductions in both of our riskier asset classes are offset by a rise in low-risk asset holdings. This effect is true for all borrowing constraint measures and is in line with the theoretical model presented in Section 3. For example, Constraint 5 is associated with a reduction in the holdings of high and medium-risk assets by 1.8% and 0.2% respectively. These falls are counterbalanced by a 2.0% increase in the share of low-risk assets held by the household. We note here that although prima facie, the magnitude of the borrowing constraints may not appear to be large, our findings are, for example, comparable in size to the effect of children being present in the household. Moreover, we have demonstrated that in the presence of a standard set of controls and proxies for background risk measures, borrowing constraints are influential in determining household portfolio allocation decisions. This reflects the fact that household portfolio allocation may invariably be the result of a complex and multifaceted decision-making process, albeit one in which borrowing constraints – along with numerous other factors – have a role to play. What is also notable is that our findings are entirely robust to alternative empirical measures of borrowing constraints. Significantly, our constraints are unanimously associated with households selecting less risky financial portfolios, such that we observe increases in the share of the low-risk asset class, which is counterbalanced by falls in shares held in both the high-risk and medium-risk asset classes.
6.3. Robustness analysis
To explore the robustness of our findings it is informative to investigate how our results in Section 6.2 are affected when alternative definitions for our dependent variables are used.Footnote35 As outlined above, the limitations of the SCF data mean that classifying a household's financial assets using information based on their mean-variance characteristics is not possible. As a result, we present results consistent with a more general classification, which entails allocating different asset types to high, medium or low-risk asset classes, and in some cases, omitting certain asset types from our taxonomy.
Table presents OF estimation results corresponding to a number of alternative dependent variable definitions, where we emphasise that the right hand side variables are identical to those used in Table . In Panel A, Specification 1 reproduces the panel estimates for the borrowing constraint variables in Table for comparative purposes. Specification 2 in Panel B presents estimates in which high-risk assets are defined as directly held stocks and shares, and all indirect risky asset holding is allocated to medium-risk assets. This includes, for instance, stocks and shares held in retirement accounts. In Panel C, Specification 3 omits all retirement accounts and life insurance policies from our definitions. This specification aims to remove a household's retirement and insurance choices from their ‘investment portfolio’ given that these type of financial products potentially serve different purposes in the household's investment decisions. Finally, Panel D presents the estimates for Specification 4, in which we reallocate all bonds – except government and saving bonds – to the medium-risk asset class.
Table 6. Ordered fractional (OF) model estimates: robustness analysis.
The estimates reported in Table are generally consistent across the alternative dependent variable definitions in terms of sign, size and statistical significance. Interestingly, our re-allocation of indirect stock holding – which arises predominantly through the holding of retirement accounts – to the medium-risk asset category, appears to have the largest influence on our reported estimates in terms of the proportions of both high-risk and medium-risk assets held. For example, Constraint 5 in Specification 2 is associated with share reductions in holdings of high and medium-risk assets – by 0.7% and 1.0%, respectively – as compared to 1.8% and 0.2% for Specification 1. The overall association with low-risk asset holding is however similar, with Constraint 5 being associated with a 1.7% increase in the share of low-risk assets held. Overall, our additional results are consistent with our main finding that borrowing constraints reduce the share of household wealth allocated to both high-risk and medium-risk assets, which is offset by an increase in the proportion of low-risk assets. We have also highlighted the potential importance of how the dependent variables are defined.
7. Conclusion
This paper has contributed to the household finance literature in a number of ways. To motivate our empirical analysis, we have used insights from a mean-variance model of portfolio allocation structured around three tradable assets that are differentiated by increasing rates of risk and return. Significantly, the assumption of three tradable assets reflects the make-up of empirically observable SCF data according to which household financial wealth is classified as being either low-risk, medium-risk, or high-risk. Under non-restrictive assumptions the behaviour of the medium-risk asset in our mean-variance framework was found to be ambiguous. Simple model simulations based on plausible realisations of the first and second moments of our tradable assets, coupled with our subsequent econometric analysis enabled us to empirically resolve this ambiguity.
Most saliently, our empirical findings indicate that borrowing-constrained households, and further, households that are exposed to higher levels of background risk, are inclined to allocate their financial wealth towards less risky asset categories. Here, we emphasise that borrowing constraints may have significant behavioural ramifications for the medium-risk asset class, and not just assets that are classified as being high-risk. This particular finding may be important: our sample data suggests that in addition to there being a ‘stockholding puzzle’ – which affects high-risk assets – US households also shun holding medium-risk assets, which our empirical proxies for borrowing constraints appear to partially explain. Here, our econometric estimations found that a lack of access to borrowing is not only negatively associated with stockholding, but, the holding of medium-risk assets. This finding has important public policy implications, which are potentially more far-reaching for US households than their propensity to shun holding stocks. For example, medium-risk assets include retirement funds and life insurance polices. Non-participation in the markets for these financial products may substantially increase the likelihood of being in poverty in old age, or increase the likelihood of family members suffering a catastrophic financial shock – such as the inability to maintain mortgage payments – in the event of early death. In this regard, public policies aimed at ensuring greater household access to borrowing and credit may be essential to increasing the uptake of some medium-risk financial products, which in turn may mitigate the financial impacts of events such as retirement and death across the lifecycle of a household. In this sense, our contribution suggests that further work directed towards accounting for the allocation of medium-risk assets in US households should be considered expedient.
Acknowledgments
We thank Alberto Montagnoli, Christoph Thoenissen and Mark Tippett for valuable comments and suggestions. The usual disclaimer applies. The authors have no declarations of interest to make.
Disclosure statement
No potential conflict of interest was reported by the author(s).
Additional information
Notes on contributors
Raslan Alzuabi
Raslan Alzuabi is a Lecturer in Economics at the University of Sheffield.
Sarah Brown
Sarah Brown is a Professor of Economics at the University of Sheffield.
Daniel Gray
Daniel Gray is a Lecturer in Economics at the University of Sheffield.
Mark N. Harris
Mark N. Harris is a Professor of Economics at Curtin University.
Christopher Spencer
Christopher Spencer is Senior Lecturer in Economics at Loughborough University.
Notes
1 These authors also report that the number of Americans who cannot easily access loans may be twice as many as previously estimated, when people who cannot easily qualify for loans because of blemishes in their credit histories are taken into account.
2 This is of course, notwithstanding the detrimental effect that the inability to borrow plays in exacerbating social inequalities, and its implications for household consumption smoothing.
3 In this paper, our concept of background risk therefore corresponds to risk associated with the household wealth process, and not so-called ‘systemic risk’, which is a type of background risk that is present in financial markets. Previous theoretical and empirical research has indicated that the presence of background risk can have a significant impact on the allocation of household portfolios.
4 See for instance (Carroll Citation2002; Hurd Citation2002). Our motivational theoretical model may have implications for other empirical contributions where financial portfolios are treated as being comprised of low, medium, and high-risk assets. From an empirical perspective, many asset management companies describe the portfolio structure of their investment products using this taxonomy.
5 In addition to using a standard set of controls, our subsequent econometric analysis therefore includes variables that proxy for such risk when estimating the impact of borrowing constraints on a household's financial portfolio allocation.
6 As argued below, the exogenous and directly uninsurable nature of background risk amounts to the assumption of incomplete markets. The presence of such risk can lead a decision-maker to ‘reduce exposure to another risk even if the two risks are statistically independent’ (Kimball Citation1992), a concept described as ‘temperance’ (Kimball Citation1991). For related theoretical contributions see Pratt and Zeckhauser (Citation1987), Kimball (Citation1991), Gollier and Pratt (Citation1996), and Heaton and Lucas (Citation2000)
7 Canner, Mankiw, and Weil (Citation1997) explore portfolio allocation among cash, bonds, and stocks, with a view to reconciling the household stockholding puzzle in light of the financial advice received by households.
8 We choose to use a Markowitz mean-variance framework due to its relative simplicity and tractability. In this regard, our model provides a useful starting point for motivating our subsequent empirical analysis. Nevertheless, since the publication of Markowitz (Citation1952)–which uses variance as a measure of risk–more sophisticated techniques to inform portfolio selection have been developed. Although these techniques go beyond the scope of our contribution, they include conditional value at risk (Rockafellar and Uryasev Citation2000) and distortion risk measures (Wang Citation2000). We thank an anonymous referee for raising this issue.
9 Although ‘safe’ instruments such as government bonds may be riskless in nominal terms, inflation means that their return may be uncertain in real terms.
10 Eichner and Wagener (Citation2012) consider the case of two tradable assets whose weights sum to unity. We extend this approach to the case of three tradable assets, which is uncommon in the literature. Unlike our contribution, Eichner and Wagener (Citation2012) do not consider the impact of short-sale constraints on the tradable assets, which in the context of our contribution, are important model features.
11 Whilst this admits a natural, albeit simplistic, interpretation as a borrowing constraint, Kaplow (Citation1994, 1505) notes that: ‘Individuals’ ability to borrow against human capital is limited, but some such borrowing occurs'. For instance, residential mortgages are based in part on predictions of future earnings; further, in the United States, the largest student loans are available to law and medical school students, due to the high and stable expected future income stream associated with jobs in the legal and medical professions.
12 Restricting the portfolio selection by only having positive weights of the assets limits the amounts of possible portfolios and introduces complexity that cannot be handled by closed-form mathematics, and requires computation of the corresponding Kuhn-Tucker conditions.
13 In the context of Italian households, Jappelli, Julliard, and Pagano (Citation2010) also suggest that whereas institutional investors are able to borrow at low-risk to invest in high-risk assets with a greater expected return, households cannot act the same way, for example by funding stock market investments through borrowing from financial intermediaries such as banks. Using household data, the authors show that explicitly considering the no short-selling constraint helps significantly in reconciling individual portfolio choices with the efficient ones implied by portfolio theory (Markowitz Citation1952). Borrowing constraints which prevent households from short-selling are also explored in Bucciol, Miniaci, and Pastorello (Citation2017), who also develop a model of household portfolio choice with borrowing restrictions in a mean-variance expected utility maximisation framework.
14 Under a Markowitz mean-variance approach, the presence of zero correlations between the tradable assets does not preclude a portfolio from benefitting from diversification. However, if assets are perfectly positively correlated, no benefit from diversification arises. In assuming zero correlations, households can be perceived as selecting uncorrelated tradable assets to help diversify risk.
15 In setting out our motivational theoretical framework, we are able to demonstrate that even in a relatively parsimonious model without correlated tradable assets, the impact of background risk on asset allocation is subject to some ambiguity. In our model, households exploit the information contained in background risk covariance structures, which has implications for portfolio diversification and risk reduction. We stress here that including correlations between the tradable assets introduces additional complexity to our findings as the predictions of the model become considerably more difficult to disentangle. Additional numerical experiments not presented here suggest that allowing for such correlations does not help to resolve the ambiguity described above. This leads us to suggest that an empirical approach such as that used in Section 5 can be usefully exploited to resolve such ambiguity.
16 Jiang, Ma, and An (Citation2010) also explore the impact of background risk on the efficient frontier, and similarly find that an increase in background risk will shift the frontier to the right. Unlike our contribution, however, these authors do not focus on the implications of shifts in the frontier on household welfare or the optimal composition of a household's portfolio given mean-variance preferences.
17 As is standard in financial portfolio theory our interest is with the part of the efficient frontier in the –
space such that
, where
denotes the value of
at the global minimum portfolio and
is a value of
on the efficient fronter, as captured by expression (EquationA6
(A6)
(A6) ) in Appendix 1. A discussion of
is provided in Appendix 3. An important corollary of the results in expressions (Equation4
(4)
(4) ) and (Equation5
(5)
(5) ) is that whilst in an unconstrained setting, increasing risk aversion is associated with a decrease (increase) in the share of assets in the high-risk (low-risk) category, for the medium-risk asset share
, the effect will also be a function of the ratio of
and
, and its relationship to the expected returns of the three tradable assets. Under certain conditions, risk-loving households may hold a smaller share of their wealth in medium-risk assets relative to risk-averse households.
18 Although our empirical examples are highly stylised and tied to an explicit formal model, similarities in the profiles of the efficient frontiers for US and Italian households can be observed. See Figure 2 in Jappelli, Julliard, and Pagano (Citation2010), and the associated discussion.
19 In practice, prohibitively high overdraft charges may act as an impediment to sustaining a negative balance for a considerable period of time.
20 We stress here that in the presence of a short-sale constraint, the non-binding return-risk space available to households satisfying ,
, and
may be limited, even when background risk is independent of the tradable assets. Under independent background risk, neither the optimal asset allocations associated with different points on the efficient frontier nor the regions of the non-binding risk-return space are functions of background risk.
21 Interest rates in US checking and savings accounts are closely tied to the fed funds rate, which is set by the Federal Open Market Committee (FOMC).
22 Our choice of mean-variance utility is commonplace in standard financial economics texts (Bailey Citation2005), and is appealing due to its analytical tractability. In our empirical analysis, we use measures of risk attitudes to capture a household's attitude towards risk. We stress here that these measures only proxy for risk preferences, and may not be tightly linked to the shape of a household's utility function.
23 Preference functions for which households are decreasingly absolute risk averse (DARA) do not lead to tractable results, and require solving by linear quadratic programming techniques. The expected log utility function given by , where W denotes wealth, would fall into this class of function (see Pulley Citation1993, Eqn (2), 686 and the corresponding discussion for further details).
24 The extent to which a change in impacts on the value of
at the point of tangency is provided in Appendix 4, as is the corresponding impact of a change in risk-aversion γ. The Kuhn-Tucker conditions for our model are set out in Appendix 5.
25 This effect arises irrespective of the nature of correlation between background risk and the tradable assets.
26 Although not shown in Figure , the constraint becomes binding at approximately . Under background risk, the constraint becomes binding at approximately
.
27 Under independent background risk an increase in will, ceteris paribus, reduce household welfare. The composition of the household's optimal portfolio will be identical to the no background risk case.
28 Given the high rate of non-response associated with micro-data relating to wealth information, the SCF provides five imputations which give a distribution of outcomes. These multiple imputations increase the efficiency of the estimation, whilst also providing uncertainty surrounding the imputed values. Our summary statistics are based on taking the average of the corresponding five implicates for each cross-section. However, the econometric estimates are based on implementing the repeated imputation inference (RII) method, as described by Little and Rubin (Citation1987), Rubin (Citation1987) and Montalto and Sung (Citation1996). The RII uses the average of the coefficients across the five imputations and adjusts the corresponding standard errors accordingly.
29 These measures are similar to those used by Fulford (Citation2015). However, we allow a differential impact of being above or below a household's normal income by entering positive and negative values as separate variables.
30 The question changed the time period from 5 years to 12 months in the 2016 and 2019 waves of the survey. If we restrict our analysis to the consistent questions (i.e. between 1995 and 2013) we find no differences in the results.
31 We also use Tobit analysis (Tobin Citation1958) to model high-risk asset shares in line with the existing literature. The coefficients and associated marginal effects are discussed in Appendix 6. Generally these results are in line with those for the FRM approach.
32 In our dataset, this assumption is also reflected in the values of US households' observable asset shares.
33 It would be possible to model the effects of drivers on each of the shares as a linear system, such that
where
is a matrix of (household) covariates and
is a random error. This would effectively be an extension of the linear probability model, the shortcomings of which are well-known (Gujarati Citation1995). Indeed, such an approach would not be ideal: it would not guarantee that
; would have repercussions on ensuring the adding-up constraint that
; it would also be unable to handle boundary observations of 0 or 1 shares; and would likely embody heteroskedasticity in
. We have also explored the use of a multi-nominal fractional response model, see for example, Becker (Citation2014). In this setting, the inherent risk ordering of asset classes is not accounted for in the estimation strategy, instead the multi-nominal probit model is used as the foundation of the estimation strategy. We obtain similar results to those presented when we adopt this alternative modelling strategy.
34 We have also explored the robustness of our results subject to the inclusion of a dummy variable that captures if an individual falls in the bottom 25% of the income distribution. We find that the results corresponding to the borrowing constraint variables remain consistent with those presented in the paper, in terms of both the signs and statistical significance of the estimated parameters. This suggests that our borrowing constraint variables are not merely capturing non-linear income effects.
35 We thank an anonymous referee for making this suggestion, and further, for proposing a number of alternative asset classifications which are used in our estimations.
36 Deriving (EquationA1(A1)
(A1) ) requires solving a two equation system with three unknowns. As there are an infinite number of solutions it is necessary to pin down the value of the scalar Γ. It is also possible to specify
in (EquationA1
(A1)
(A1) ) as
.
37 Alternatively, we can express (EquationA10(A10)
(A10) ) as
. Once a household's optimal portfolio allocation is bound by the no short-sale constraint, this condition will not hold.
38 In our simple static model, borrowing restrictions assume the form of short-selling constraints. However, in a dynamic setting, Dybvig and Huang (Citation1988) note that imposing a non-negativity constraint (or in fact any negative lower bound) on wealth is a plausible economic assumption, and admits a natural interpretation as a credit constraint. Another way is to prevent borrowing from future income. There are many institutional restrictions on the amount of credit an individual can obtain.
39 The impact on the efficient frontier when tradable assets are correlated is well explored in the literature. We therefore refrain from focusing on this area.
References
- Arrondel, L., and H. Calvo-Pardo. 2002. “Portfolio Choice with a Correlated Background Risk: Theory and Evidence.” DELTA Working Papers 2002-16, DELTA (Ecole normale supérieure).
- Bailey, R. E. 2005. The Economics of Financial Markets. Cambridge: Cambridge University Press. https://doi.org/10.1017/CBO9780511817458
- Barasinska, N., D. Schäfer, and A. Stephan. 2012. “Individual Risk Attitudes and the Composition of Financial Portfolios: Evidence From German Household Portfolios.” The Quarterly Review of Economics and Finance 52 (1): 1–14. https://doi.org/10.1016/j.qref.2011.10.001.
- Becker, G. 2014. “‘The Portfolio Structure of German Households: A Multinomial Fractional Response Approach With Unobserved Heterogeneity’, University of Tübingen Working Papers in Economics and Finance no. 74.
- Bertaut, C. C. 1998. “Stockholding Behavior of US Households: Evidence From the 1983-1989 Survey of Consumer Finances.” Review of Economics and Statistics 80 (2): 263–275. https://doi.org/10.1162/003465398557500.
- Browning, M., and A. Lusardi. 1996. “Household Saving: Micro Theories and Micro Facts.” Journal of Economic Literature 34 (4): 1797–1855. https://www.jstor.org/stable/2729595.
- Bucciol, A., R. Miniaci, and S. Pastorello. 2017. “Return Expectations and Risk Aversion Heterogeneity in Household Portfolios.” Journal of Empirical Finance 40: 201–219. https://doi.org/10.1016/j.jempfin.2016.08.002.
- Campbell, J. R., and Z. Hercowitz. 2019. “Liquidity Constraints of the Middle Class.” American Economic Journal: Economic Policy 11 (3): 130–155. https://doi.org/10.1257/pol.20180070.
- Canner, N., N. G. Mankiw, and D. N. Weil. 1997. “An Asset Allocation Puzzle.” American Economic Review 87 (1): 181–191. https://doi.org/10.3386/w4857.
- Cardak, B. A., and R. Wilkins. 2009. “The Determinants of Household Risky Asset Holdings: Australian Evidence on Background Risk and Other Factors.” Journal of Banking and Finance 33 (5): 850–860. https://doi.org/10.1016/j.jbankfin.2008.09.021.
- Carroll, C. D. 2001. “A Theory of the Consumption Function, with and Without Liquidity Constraints.” Journal of Economic Perspectives 15 (3): 23–45. https://doi.org/10.1257/jep.15.3.23.
- Carroll, C. D. 2002. “Portfolios of the Rich.” In Household Portfolios, edited by L. Guiso, M. Haliassos, and T. Jappelli, Chapter 10, 389–430. Cambridge, MA: MIT Press.
- Carroll, C. D., M. B. Holm, and M. S. Kimball. 2021. “Liquidity Constraints and Precautionary Saving.” Journal of Economic Theory 195: Article No. 105276. https://doi.org/10.1016/j.jet.2021.105276.
- Chiang, A. C. 1984. Fundamental Methods of Mathematical Economics. 3rd ed. Singapore: McGraw Hill Book Company.
- Dybvig, P. H., and C. Huang. 1988. “Nonnegative Wealth, Absence of Arbitrage, and Feasible Consumption Plans.” Review of Financial Studies 1 (4): 377–401. https://doi.org/10.1093/rfs/1.4.377.
- Edwards, R. D. 2008. “Health Risk and Portfolio Choice.” Journal of Business and Economic Statistics26 (4): 472–485. https://doi.org/10.1198/073500107000000287.
- Eichner, T., and A. Wagener. 2012. “Tempering Effects of (dependent) Background Risks: A Mean-variance Analysis of Portfolio Selection.” Journal of Mathematical Economics 48 (6): 422–430. https://doi.org/10.1016/j.jmateco.2012.09.001.
- Fratantoni, M. 2001. “Homeownership, Committed Expenditure Risk and the Stockholding Puzzle.” Oxford Economic Papers 53 (2): 241–259. https://doi.org/10.1093/oep/53.2.241.
- Fulford, S. L. 2015. “The Surprisingly Low Importance of Income Uncertainty for Precaution.” European Economic Review 79:151–171. https://doi.org/10.1016/j.euroecorev.2015.07.016.
- Gollier, C., and J. W. Pratt. 1996. “Risk Vulnerability and the Tempering Effect of Background Risk.” Econometrica 64 (5): 1109–1123. https://doi.org/10.2307/2171958.
- Greene, W. 2012. Econometric Analysis. 7th ed. Englewood Cliffs, NJ: Prentice Hall.
- Greene, W. H., and D. A. Hensher. 2010. Modeling Ordered Choices: A Primer. Cambridge: Cambridge University Press.https://doi.org/10.1017/CBO9780511845062.
- Guiso, L., T. Jappelli, and D. Terlizzese. 1996. “Income Risk, Borrowing Constraints, and Portfolio Choice.” American Economic Review 86 (1): 158–172. https://doi.org/10.2307/2937799.
- Guiso, L., and M. Paiella. 2008. “Risk Aversion, Wealth, and Background Risk.” Journal of the European Economic Association 6 (6): 1109–1150. https://doi.org/10.1162/jeea.2008.6.issue-6.
- Gujarati, D. N. 1995. Basic Econometrics. 2nd ed. New York: McGraw-Hill.
- Haliassos, M., and C. C. Bertaut. 1995. “Why Do so Few Hold Stocks?.” Economic Journal 105 (432): 1110–1129. https://doi.org/10.2307/2235407.
- Haliassos, M., and C. Hassapis. 1999. “Borrowing Constraints, Portfolio Choice and Precautionary Motives: Theoretical Predictions and Empirical Complications.” In Computational Methods in Decision-Making, Economics and Finance, edited by E. Kontoghiorghes, B. Rustem, and S. Siokos, Chapter 10, 185–212. Boston, MA: Springer.
- Haliassos, M., and A. Michaelides. 2003. “Portfolio Choice and Liquidity Constraints.�� International Economic Review 44 (1): 143–177. https://doi.org/10.1111/iere.2003.44.issue-1.
- Hall, R. E., and F. S. Mishkin. 1982. “The Sensitivity of Consumption to Transitory Income: Estimates From Panel Data on Households.” Econometrica 50 (2): 461–481. https://doi.org/10.2307/1912638.
- Hamdani, K., C. K. Mills, E. Reyes, and J. Battisto. 2019. Unequal Access to Credit -- The Hidden Impact of Credit Constraints. Report published by the Federal Reserve Bank of New York, September 2019.
- Hayashi, F. 1985. “The Effect of Liquidity Constraints on Consumption: A Cross-sectional Analysis.” The Quarterly Journal of Economics 100 (1): 183–206. https://doi.org/10.2307/1885741.
- Heaton, J., and D. Lucas. 2000. “Portfolio Choice in the Presence of Background Risk.” Economic Journal 110 (460): 1–26. https://doi.org/10.1111/1468-0297.00488.
- Hurd, M. 2002. “Portfolio Holdings of the Elderly.” In Household Portfolios, edited by L. Guiso, M. Haliassos, and T. Jappelli, Chapter 11, 431–472. Cambridge, MA: MIT Press.
- Insler, M., J. Compton, and P. Schmitt. 2016. “The Investment Decisions of Young Adults Under Relaxed Borrowing Constraints.” Journal of Behavioral and Experimental Economics 64:106–121. https://doi.org/10.1016/j.socec.2015.07.004.
- Jappelli, T. 1990. “Who is Credit Constrained in the US Economy?.” The Quarterly Journal of Economics 105 (1): 219–234. https://doi.org/10.2307/2937826.
- Jappelli, T., C. Julliard, and M. Pagano. 2010. “Households' Portfolio Diversification.” Studi Economici 100: 117–143. https://doi.org/10.3280/STE2010-100007.
- Jappelli, T., J.-S. Pischke, and N. S. Souleles. 1998. “Testing for Liquidity Constraints in Euler Equations with Complementary Data Sources.” Review of Economics and Statistics 80 (2): 251–262. https://doi.org/10.1162/003465398557492.
- Jiang, C., Y. Ma, and Y. An. 2010. “An Analysis of Portfolio Selection with Background Risk.” Journal of Banking and Finance 34 (12): 3055–3060. https://doi.org/10.1016/j.jbankfin.2010.07.013.
- Kaplow, L. 1994. “Human Capital Under An Ideal Income Tax.” Virginia Law Review 80 (7): 1477–1514. https://doi.org/10.2307/1073513.
- Kawasaki, K., and E. Lichtenberg. 2014. “Econometric Analysis of Grading Standards: The Ordered Fractional Approach.” American Journal of Agricultural Economics 96 (1): 345–365. https://doi.org/10.1093/ajae/aat088.
- Kimball, M. S. 1991. “Precautionary Motives for Holding Assets.” NBER Working Papers 3586, National Bureau of Economic Research, Inc.
- Kimball, M. S. 1992. “Precautionary Motives for Holding Assets.” In The New Palgrave Dictionary of Money and Finance, Vol. 3, edited by P. Newman, M. Milgate, and J. Eatwell, 158–161. London: McMillan Press Ltd.
- Koo, H. K. 1998. “Consumption and Portfolio Selection with Labor Income: A Continuous Time Approach.” Mathematical Finance 8 (1): 49–65. https://doi.org/10.1111/mafi.1998.8.issue-1.
- Le Blanc, J., A. Porpiglia, J. Zhu, and M. Ziegelmeyer. 2015. Household Saving Behavior and Credit Constraints in the Euro Area. Technical Report 1790, European Central Bank (ECB).
- Little, R. J., and D. B. Rubin. 1987. Statistical Analysis with Missing Data. New York: Wiley.
- Lusk, J. L., and K. H. Coble. 2008. “Risk Aversion in the Presence of Background Risk: Evidence From An Economic Experiment.” Research in Experimental Economics 12:315–340. https://doi.org/10.1016/S0193-2306(08)00006-9.
- Lyons, A. C. 2003. “How Credit Access Has Changed Over Time for U.S. Households.” Journal of Consumer Affairs 37 (2): 231–255. https://doi.org/10.1111/j.1745-6606.2003.tb00452.x.
- Markowitz, H. 1952. “Portfolio Selection.” Journal of Finance 7 (1): 77–91. https://doi.org/10.2307/2975974.
- Montalto, C. P., and J. Sung. 1996. “Multiple Imputation in the 1992 Survey of Consumer Finances.” Financial Counseling and Planning 7:133–46.
- Papke, L. E., and J. M. Wooldridge. 1996. “Econometric Methods for Fractional Response Variables with An Application to 401(K) Plan Participation Rates.” Journal of Applied Econometrics 11 (6): 619–32. https://doi.org/10.1002/(ISSN)1099-1255.
- Perraudin, W., and B. Sorensen. 2000. “The Demand for Risky Assets: Sample Selection and Household Portfolios.” Journal of Econometrics 97 (1): 117–144. https://doi.org/10.1016/S0304-4076(99)00069-X.
- Poterba, J. M. 2002. “Taxation and Portfolio Structure: Issues and Implications.” In Household Portfolios, Chapter 3, edited by L. Guiso, M. Haliassos, and T. Jappelli, 103–142. Boston: MIT Press.
- Pratt, J. W., and R. J. Zeckhauser. 1987. “Proper Risk Aversion.” Econometrica 55 (1): 143–54. https://doi.org/10.2307/1911160.
- Pulley, L. B. 1993. “Mean-variance Approximations to Expected Logarithmic Utility.” Operations Research 31 (4): 685–96. https://doi.org/10.1287/opre.31.4.685.
- Rockafellar, R. T., and S. P. Uryasev. 2000. “Optimization of Conditional Value-at-risk.” Journal of Risk2 (3): 21–42. https://doi.org/10.21314/JOR.2000.038.
- Rosen, H. S., and S. Wu. 2004. “Portfolio Choice and Health Status.” Journal of Financial Economics 72 (3): 457–484. https://doi.org/10.1016/S0304-405X(03)00178-8.
- Rubin, D. B. 1987. Multiple Imputation for Nonresponse in Surveys. New York: John Wiley and Sons.
- Scheinkman, J. A., and L. Weiss. 1986. “Borrowing Constraints and Aggregate Economic Activity.” Econometrica 54 (1): 23–45. https://doi.org/10.2307/1914155.
- Spaenjers, C., and S. M. Spira. 2015. “Subjective Life Horizon and Portfolio Choice.” Journal of Economic Behavior and Organization 116: 94–106. https://doi.org/10.1016/j.jebo.2015.04.006.
- Tobin, J. 1958. “Estimation of Relationships for Limited Dependent Variables.” Econometrica: Journal of the Econometric Society 26 (1): 24–36. https://doi.org/10.2307/1907382.
- Toussaint-Comeau, M. 2021. “Liquidity Constraints and Debts: Implications for the Saving Behavior of the Middle Class.” Contemporary Economic Policy 39 (3): 479–493. https://doi.org/10.1111/coep.v39.3.
- Wang, S. 2000. “A Class of Distortion Operators for Pricing Financial and Insurance Risks.” The Journal of Risk and Insurance 61 (1): 15–36. https://doi.org/10.2307/253675.
- Wilcox, D. 1989. “The Sustainability of Government Deficits: Implications of the Present-value Borrowing Constraint.” Journal of Money, Credit and Banking 21 (3): 291–306. https://doi.org/10.2307/1992415.
- Zeldes, S. P. 1989. “Consumption and Liquidity Constraints: An Empirical Investigation.” Journal of Political Economy 97 (2): 305–346. https://doi.org/10.1086/261605.
Appendices
Appendix 1.
Deriving explicit expressions for the tradable asset shares
Using expression (Equation2(2)
(2) ) subject to the constraint that
, Gauss–Jordan elimination can be applied to demonstrate that the tradable asset shares are given by
(A1)
(A1) where
(A2)
(A2) and Γ is a scalar that varies over the real line.Footnote36 Using the solutions for
,
, and
derived in expression (EquationA1
(A1)
(A1) ) entails that expression (Equation3
(3)
(3) ) can instead be expressed as
(A3)
(A3) Now suppose the household is assumed to specify
; by the efficient set theorem it sets out to minimise the variance of wealth,
. Differentiating expression (EquationA3
(A3)
(A3) ) with respect to Γ and solving for Γ constitutes a first step in deriving asset share allocations consistent with portfolio variance minimisation. This yields
(A4)
(A4) The variance minimising shares,
,
and
, are given by
(A5)
(A5) The efficient frontier,
, is thus given by
(A6)
(A6) Here, it is useful to note that the solution in expression (EquationA4
(A4)
(A4) ) is equivalent to
such that
(A7)
(A7) and
(A8)
(A8) Now, for simplicity, assume that the tradable assets are not correlated with each other, and that background wealth B is correlated with all of the tradable assets L, M and H. Using the results in expressions (EquationA2
(A2)
(A2) ), (EquationA4
(A4)
(A4) ) and (EquationA5
(A5)
(A5) )–(EquationA8
(A8)
(A8) ), it is straightforward to demonstrate that
(A9)
(A9) such that
(A10)
(A10) It is also straightforward to verify that the condition in expression (EquationA10
(A10)
(A10) ) also holds in the presence of independent background risk when the tradable assets are not correlated with each other. Further, inspection of expressions (EquationA2
(A2)
(A2) ), (EquationA4
(A4)
(A4) ), (EquationA5
(A5)
(A5) )–(EquationA8
(A8)
(A8) ) indicates that independent background risk will not influence asset allocations along the efficient frontier.Footnote37
As is noted below in Appendix 2, the presence of in the numerator of
implies that the domain of the non-binding risk-return space will also be a function of background risk when it is correlated with the model's tradable assets. Moreover, from expression (EquationA4
(A4)
(A4) ) it is evident that in the presence of correlated background risk, when the short-sale constraint is not binding, a change in
will impact on the three individual asset shares on the efficient frontier. Specifically, we have that:
(A11)
(A11)
(A12)
(A12)
(A13)
(A13) where
.
The impact of background risk of a change in asset shares in expressions (EquationA11(A11)
(A11) )–(EquationA13
(A13)
(A13) ), is summarised in Table , for which we assume that background risk is positively correlated with all respective tradable assets. We denote this restriction using the notation
and
. For each row, the positive and negative signs capture the direction of the background risk effect on the derivative
, i = L, M, B of the
, i = L, M, B. For instance, the impact of
is to reduce the share of low-risk assets in the household's portfolio, whereas the impact of
and
is to increase and reduce the share, respectively. The overall effect on the low-risk asset share
is therefore ambiguous, and determined by the values of
,
i = L, M, B. The presence of negative correlation between B and L, M, and H will be associated with a reversal of the signs in Table .
Table B1. The impact of background risk on optimal asset allocations when B is positively correlated with L, M, and H.
Appendix 2.
Conditions under which the non-negativity of 
, 
, and 
are satisfied when the short-sale constraint is non-binding
It is possible to use the results in expression (EquationA5(A5)
(A5) ) in Appendix 1 to determine the conditions under which
,
, and
are jointly satisfied when the short-sale constraint is not binding. From (EquationA5
(A5)
(A5) ), imposing
is equivalent to the constraint that
. For
we note that
can equivalently be written as
. Imposing
implies that
, or equivalently,
. Together, these results imply that
,
, and
will be positive – that is, the short-sale constraint will not be binding – when
lies in the interval
(A14)
(A14) Drawing on the fact that
and
in Appendix 1, means that the condition in expression (EquationA14
(A14)
(A14) ) can be re-expressed in terms of the expected returns, viz.,
(A15)
(A15) Condition (EquationA15
(A15)
(A15) ) suggests that in the presence of a short-sale constraint, the unconstrained space of returns and risks available to households satisfying
,
, and
may be limited. As shown in Figure of Section 3, this may lead to the no-short-sale constraint becoming binding for households with low-risk tolerance.Footnote38 Moreover, expression (EquationA7
(A7)
(A7) ) of Appendix 1 implies that for the fully correlated model, the domain of the non-binding risk-return space governed by condition (EquationA15
(A15)
(A15) ) will also be a function of background risk due to the presence of
in
. ▪
Appendix 3.
The global minimum portfolio and the optimal household portfolio with mean-variance preferences
A.1. Zero-correlation between assets
The point of tangency between the household's mean-variance utility function and the efficient frontier in the risk-return space is obtained by differentiating the efficient frontier in expression (EquationA6
(A6)
(A6) ) with respect to
, setting the resulting expression equal to
and solving for
. The absence of correlation requires setting all of the covariance terms in expression (Equation3
(3)
(3) ) to zero prior to the model being differentiated. Given the mean-variance preferences of the household captured by expression (Equation6
(6)
(6) ), namely
, the point of tangency between a household's indifference curve will be given by the solution to the equation
(A16)
(A16) and will satisfy the constraint
(A17)
(A17) Solving (EquationA16
(A16)
(A16) ) for
yields
(A18)
(A18) where
denotes the value of
on the frontier at the point of tangency with expression (Equation6
(6)
(6) ), such that terms A to E are defined as:
(A19)
(A19)
(A20)
(A20)
(A21)
(A21)
(A22)
(A22) and
(A23)
(A23) The value of
at the point of tangency, which we denote
(
), can be obtained by substituting Equation (EquationA18
(A18)
(A18) ) into expression (Equation3
(3)
(3) ). The global minimum portfolio is obtained by differentiating the efficient frontier
in expression (EquationA6
(A6)
(A6) ) with respect to
, setting the resulting expression to zero, and solving for
. This yields a solution as in expression (EquationA18
(A18)
(A18) ), albeit without the
term in the numerator. Finally, from expressions (EquationA18
(A18)
(A18) )–(EquationA23
(A23)
(A23) ) it is evident that under independent background risk
will be unaffected by change in
:
(and by implication
) is absent from (EquationA18
(A18)
(A18) ).
A.2. Correlated background risk
Now consider the restricted case of the correlated model where background risk is correlated with each of the tradable assets, but the tradable assets are not correlated with each other,Footnote39 so that the equation for the efficient frontier is given by
(A24)
(A24) Assuming mean-variance preferences as in expression (Equation6
(6)
(6) ), the point of tangency with the portfolio frontier is given by solving for
the expression
(A25)
(A25) Although somewhat cumbersome, this yields
(A26)
(A26) where
denotes the value of
on the frontier at the point of tangency in the risk-return space. Here, we note that the value of
at the global minimum variance can be obtained by omitting the term
which appears in the numerator of expression (EquationA26
(A26)
(A26) ). Significantly, expression (EquationA26
(A26)
(A26) ) implies that
will be partially determined by the presence of background risk,
, when it is correlated with the tradable asset shares.
Appendix 4.
The impact of background risk and risk preferences on 

In the correlated model, the additional presence in expression (EquationA26(A26)
(A26) ) of terms containing
, namely
(A27)
(A27)
(A28)
(A28) and
(A29)
(A29) implies that the presence of background risk will affect the point of tangency,
. In particular, the sign and magnitude of the correlation coefficients
,
, and
are central to determining how background risk, which appears as
, affects the point of tangency with household preferences. Consider the impact of correlation between background wealth B and the high-risk tradable asset H, whilst assuming
. For an increase in
, a negative (positive) value of
, will lead to a fall (rise) in the value of
, which using expression (EquationA29
(A29)
(A29) ) in (EquationA26
(A26)
(A26) ) is captured by
(A30)
(A30) Inspection of expression (EquationA26
(A26)
(A26) ) also indicates that a change in
will lead to a change in the value of
corresponding to the global minimum variance,
. This is unlike the case under independent background risk, in which the global minimum variance is unaffected by such a change.
For any given expected return in the upward sloping region of the frontier space where , the impact on
of a change in risk aversion
will be given by
(A31)
(A31) Expression (EquationA31
(A31)
(A31) ) says that an increase (decrease) in risk aversion γ will be associated with a decrease (increase) in the value of
at the new tangency point. Based on the results implied by the expressions for
i = L, M, H in (Equation4
(4)
(4) ), the associated increase in
will be associated with a fall (rise) in the proportion of high-risk assets held by the household, and a rise (fall) in the proportion of low-risk assets held at the new point of tangency. However, the impact on
will be ambiguous. We emphasise here that the change in
implied by expression (EquationA31
(A31)
(A31) ) is realised irrespective of whether background risk is assumed to be correlated with, or independent of, the tradable assets.
Appendix 5.
Short-selling restrictions and Kuhn-Tucker conditions
In the presence of short-selling restrictions, Kuhn-Tucker conditions are used so that the (inequality constrained) minimum variance portfolio can be determined. For our minimisation problem, we closely follow Chiang (Citation1984, pp.722-728) by first converting the problem into a maximization one by multiplying the objective function, , by
. For the fully correlated model in Appendix 1 we wish to calculate
(A32)
(A32) whilst ensuring the non-negativity of our assets shares, namely
(A33)
(A33) Using the results in (EquationA1
(A1)
(A1) ), it is useful to restate the inequalities in (EquationA33
(A33)
(A33) ) as
(A34)
(A34) Forming the Lagrangian gives
(A35)
(A35) which we wish to evaluate subject to
(A36)
(A36) and the following non-negativity constraints
(A37)
(A37) Setting up the Lagrangian,
, thus yields
(A38)
(A38) As
and
must be non-negative, the first order conditions on our variable set implies that for a maximum, the following set of conditions must hold,
(A39)
(A39) From expressions (EquationA38
(A38)
(A38) ) and (EquationA39
(A39)
(A39) ), it follows that if
, then
,
and
, or alternatively,
(A40)
(A40) However, it follows from the final line of (EquationA39
(A39)
(A39) ), which restates the modified constraints in (EquationA36
(A36)
(A36) ), that
. If this expression is substituted into (EquationA40
(A40)
(A40) ), the latter two lines of (EquationA39
(A39)
(A39) ) can be combined to yield
(A41)
(A41) Accordingly, the first order conditions in (EquationA39
(A39)
(A39) ) can now be restated in an equivalent form where the dummy variables are not present. Employing
to denote
the Kuhn-Tucker conditions for our maximisation problem can be expressed as
(A42)
(A42)
Appendix 6.
Univariate results: coefficients and marginal effects
Table G1. Tobit model: high-risk asset share -- coefficients.
Table G2. Tobit model: High-risk asset share -- Marginal effects.
Table G3. Fractional probit model -- coefficients.
Table G4. Fractional probit model -- marginal effects.