Abstract
Background
Benign prostate hyperplasia (BPH) and prostate cancer (CaP) are among the most frequently occurring prostatic diseases. When CaP progressed to castration-resistant CaP (CRPC), the prognosis is poor. Although CaP/CRPC and BPH frequently coexist in prostate, the inter-relational mechanism between them is largely unknown.
Methods
Single-cell RNA sequencing, bulk-RNA sequencing, and microarray data of BPH, CaP in the Gene Expression Omnibus database were obtained and comprehensively analyzed. Weighted Gene Co-Expression Network Analysis (WGCNA) and lasso regression analysis were performed to explore the potential biomarkers.
Results
With WGCNA, five modules in BPH, two in CaP, and three in CRPC were identified as significant modules. Pathway enrichment analysis found that the epigenetics and chromosomal-related signaling were dominantly clustered in the CaP group but not in BPH and CRPC. Lasso regression analysis was used to analyze further the mutual genes between the BPH module and the CRPC module. As a result, DDA1, ERG28, OGFOD1, and OXA1L were significantly correlated with the transcriptomic features in both BPH and CRPC. More importantly, the role of the four gene signatures was validated in two independent anti-PD-1 immunotherapy cohort.
Conclusion
This study revealed the shared gene signatures and immune microenvironment between BPH and CRPC. The identified hub genes, including DDA1, ERG28, OGFOD1, and OXA1L, might be potential therapeutic targets for facilitating immunotherapy in prostate cancer.
Introduction
Benign prostate hyperplasia (BPH) is the main reason for lower urinary tract symptoms (LUTS) in aging men, which seriously impact the quality of life and health [Citation1]. The development of BPH is highly associated with aging. Significantly, the age-specific incidence rates of prostate cancer (CaP), the most prevalent cancer type in men worldwide, also rise steeply from around age 45–49, peaking in the 75–79 years old. In keeping with that, CaP patients frequently have pathological or symptomatic BPH. It is extensively acknowledged that the above problem of the prostate does not lead to the other, although they coexist at the same gland. However, evidence also suggested that BPH could be a risk factor for CaP or bladder cancer [Citation2,Citation3]. Thus, whether the association between CaP and BPH is a causal link remains controversial.
Patients with CaP are initially responsive to anti-androgen therapies but eventually develop into castration-resistant prostate cancer (CRPC). Androgen deprivation therapy (ADT) remains the primary palliative strategy for men with locally advanced or metastatic disease [Citation4]. Ultimately, ADT becomes ineffective in 18–24 months, yielding to lethal CRPC [Citation5]. To date, emerging evidence indicates that immunotherapy, especially immune checkpoint inhibitors (ICIs), has the potential to enable long-term survival in cancer patients [Citation6]. Unfortunately, the response rate to ICIs varies across tumors and is limited to immunologically “hot” tumors, but not “cold” tumors, especially prostate [Citation7,Citation8]. Therefore, it is critical to further understand the immune microenvironment in different prostatic pathologies and find molecular targets for screening the potential patients with response to ICIs.
Clinically, BPH is not treated as a premalignant lesion. But BPH and CaP share multiple biological features [Citation9]. The etiology of BPH remains largely elusive [Citation10]. Cellular senescence, metabolic disruption, genetic variation, and chronic inflammation are common risk factors for BPH and CaP [Citation2,Citation11]. In addition, the PI3K-Akt pathway and CDK4 play a critical role in both BPH and CaP [Citation12–14]. Preclinical evidence supports the potential role of chronic inflammation in the malignant transformation of epithelial cells in the prostate [Citation15]. In keeping with that, epidemiological evidence revealed that prostatitis and BPH lead to escalating risks of CaP [Citation16]. Chronic inflammation leads to the accumulation of proinflammatory mediators, infiltration of immune suppressor cells, and activation of immune checkpoint pathways in effector T cells, which facilitate the formation of an immune suppressive microenvironment in the “cold” tumors. However, the difference of the immune microenvironment among BPH, CaP, and CRPC remains poorly understood.
Here, in this study, we pooled three scRNA-seq datasets of samples from BPH and CaP and 10 bulk transcriptomic datasets derived from BPH, CaP, as well as CRPC. Briefly, we identified the shared gene signatures and immune microenvironment between BPH and CRPC. The identified hub genes, including DDA1, ERG28, OGFOD1, and OXA1L, might be potential therapeutic targets for facilitating immunotherapy in prostate cancer. Understanding the links between BPH and CRPC would improve cancer prevention, screening, and decision-making in integrated therapy.
Materials and methods
Datasets download and process
Bulk-transcriptomic and single-cell RNA sequencing (scRNA-seq) studies of BPH, CaP, and CRPC were obtained by a systematic search of literature from the Gene Expression Omnibus (GEO) database (https://www.ncbi.nlm.nih.gov/geo/). As a result, three single-cell RNA sequencing studies (GSE141445/GSE145838/GSE145843) and 10 bulk-RNA sequencing study (GSE80609/GSE28204/GSE89223/GSE104749/GSE119195/GSE132714/GSE65343/GSE32982/GSE134073/GSE5377) were included in this study. To further validate our findings in scRNA-seq analysis, the bulk-RNA sequencing dataset on the prostate from the TCGA database (PRAD) and the clinical data were collectively downloaded. In addition, the clinical information and transcriptomic profile of two independent cohorts of anti-PD1 therapy (GSE190265/CheckMate 025) were included for validation. Ethical approval was waived by the institutional ethics committee because data are obtained from public databases, and all the patients are de-identified.
Quality control of scRNA-seq datasets
The scRNA-seq data set was downloaded from the GEO database (GSE141445, GSE145838, and GSE145843). The duplicated data were checked and removed. During quality control process, the mitochondrial reads ratio was 0.01. The resolution parameter used in this study was 1.2. After removal of doublets, 60,668 single-cells have been identified. Cell expression profiles were quantile normalized and analyzed using the Seurat R package (http://satijalab.org/seurat/). The standard Seurat workflow was performed with the pooled scRNA-seq data from 10X Genomics by using the R package Seurat (version 4.05). Samples with less than 3 cells and/or less than 300 detected genes per cell were excluded from further analysis. Doublets were identified and filtered by R package DoubletFinder, with 4% doublets expected.
Integration of scRNA-seq datasets
To integrate multiple samples, integration anchors were identified in the list of samples using the Canonical Correlation Analysis (CCA) method. For clustering, principal component analysis (PCA), T-distributed Stochastic Neighbor Embedding (t-SNE), and Uniform Manifold Approximation and Projection (UMAP) were performed for dimension reduction. Furthermore, marker genes of each respective cluster were identified by the function of “FindAllMarkers” and the package of “SingleR” previous to manual annotation [Citation17].
Weighted gene co-expression network analysis
The weighted gene co-expression network analysis (WGCNA) algorithm was used in this study to find the co-expressed modules among BPH, CaP, and CRPC with high biological significance and investigate the association between gene networks and diseases [Citation18]. The module eigengene (M.E.) was defined to summarize the expression profiles of each module. Furthermore, the significance of the correlation between M.E.s and lesion types was calculated. The mutual genes in M.E.s positively associated with both BPH and CRPC were overlapped using interactive [Citation19].
Analysis of sub-clusters in epithelial cells
Following primary annotation, luminal and basal epithelial cells were extracted via the “SubsetData” function. Then, the “FindClusters” and “FindAllMarkers” functions were conducted, and the epithelial were reclustered by TSNE and UMAP. The sub-clusters were annotated by the dominant expression cell markers. The following cutoff threshold values were applied to reveal the marker genes for each cluster: adjust P-value < 0.01, and absolute log2 fold change > 0.5.
Analysis of copy number variations
The gene expression data of epithelial cells extracted from the Seurat object were analyzed for the CNVs with the inferCNV program of the Trinity CTAT Project (https://github.com/broadinstitute/inferCNV). The transcriptomic profiles of the epithelial cells from the normal prostate tissue were set as reference cells. For the normal group, BPH group, and CaP group, 3000 cells from each group were randomly sampled after quality control filtering with greater than 3000 UMIs. Each CNV was annotated to be either a gain or a loss.
Transcriptional factor analysis
Single-cell regulatory network inference and clustering (SCENIC) analysis was performed on luminal and basal epithelial cells from different groups of samples using the “SCENIC” package [Citation20]. SCENIC can identify targets of translational factors (TFs) based on the mRNA expression network. In addition, SCENIC was used to perform TFs motif enrichment analysis to identify direct targets and score the activity of the regulators (AUCell algorithm). The activity TFs were visualized in a heatmap, and certain TFs were visualized in a dot plot.
Differentially expressed gene analysis
The “Limma” package was used to perform the analysis for a differentially expressed gene (DEG). An empirical Bayesian method was conducted to estimate the fold change between the normal group, BPH group, CaP group, and CRPC group identified by the pathologist. The adjusted P-value for multiple testing was calculated using the Benjamini–Hochberg correction. The genes with an absolute log2 fold change greater than two were identified as DEGs between the two groups.
Gene ontology enrichment and protein–protein interaction (PPI)
Here, the gene lists were analyzed by the DAVID website for the enrichment of biological processes. Moreover, the GO terms were further investigated and visualized as functionally grouped networks by a Cytoscape plug-in tool, namely ClueGO. The P-value < 0.05 was considered significant during the gene ontology analysis. The PPI network and cluster analysis were performed with the STRING plug-in tools under a confidence cutoff value of 0.2 in Cytoscape software (version: 3.9.1).
Single sample GSEA and ImmuCellAI algorithm
Single sample Gene set enrichment analysis was conducted between normal, adjacent tissues, BPH, CaP, and CRPC to explore different immune cell infiltration states. The association between gene expression and immune infiltration was visualized in the violin plot. p < 0.05 was considered statistically significant. Moreover, immunogenomic analysis of TCGA datasets was performed by the CellAI algorithm with 24 immunes cells, which could be accessed by an online database (http://bioinfo.life.hust.edu.cn/GSCA).
Statistical analysis
Gene expression data were analyzed using the P-value, fold changes (FC), and ranks. The statistical difference between groups in the bioinformatics analysis was calculated using the Wilcoxon Signed-rank test. p < 0.05 was considered statistically significant.
Results
GEO datasets of cohorts with prostate disease
A systematic review of the literature with keywords including “prostate hyperplasia”, “prostate disease”, “prostate cancer”, or “prostate” in GEO query found 10 bulk-RNA sequencing or microarray studies and 3 scRNA-seq studies that meet the previously set criteria. The GSE numbers, detection platforms, and number of BPH cases were listed in Supplementary Table S1. illustrates the workflow of this study. As a result, 7 normal prostates, 6 adjacent prostate tissues, 67 pathologically confirmed BPH tissues, 124 treatment naïve prostate cancer tissues, and 12 CRPC samples were included for subsequent analysis.
Figure 1. Weighted gene co-expression network analysis (WGCNA).
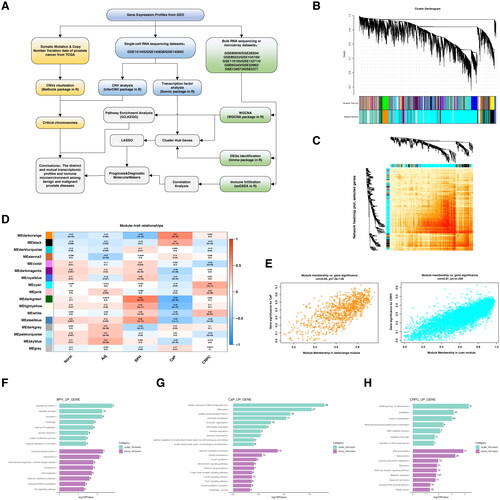
The landscape of co-expression modules among different prostatic lesions
A total of 17 modules were identified in the above 10 bulk transcriptomic datasets through WGCNA, with each color representing a distinct module. The transcriptomic profiles of the 206 cases could be clustered into dozens of subclusters with high levels of correlations (). Furthermore, the module–trait relationships based on the Spearman correlation coefficient were evaluated and visualized in a heatmap (). Five modules (dark turquoise, dark green, light yellow, white, and steelblue) were highly associated with BPH. The dark orange and black modules were highly correlated with CaP, while the cyan, pink, and white modules had a high association with CRPC (). G.O. analysis indicated that translation and spliceosome pathways were mutually increased in BPH and CRPC, while the chromatin remodeling and DNA damage repair pathways were exclusively overexpressed in the CaP (Figure 1F–H, Supplementary Table S2–S4).
The analysis of copy number variations in BPH and prostate cancer
Next, we sought to validate the differences in structural variations of chromosomes between BPH and CaP. Here, the cells from the prostate tissues were clustered into nine populations according to classical markers for indicated cell types (). The tumor cells could be clustered into luminal-like cells and basal-like cells based on their expression of keratin or PSA (). Overall, the CNVs more frequently happened in CaP compared with BPH. However, the increase of CNVs in CaP was mainly contributed by the luminal cells, while the basal cells of the BPH had significantly increased CNVs than CaP (, Figure S1). The CNVs results calculated by InferCNV from scRNA-seq datasets were further confirmed by the somatic mutation data from the TCGA-PRAD project (). Notably, the 10q23.31 was frequently deleted in CaP, where PTEN was located ().
Figure 2. Analysis of copy number variations in benign prostate hyperplasia and prostate cancer.
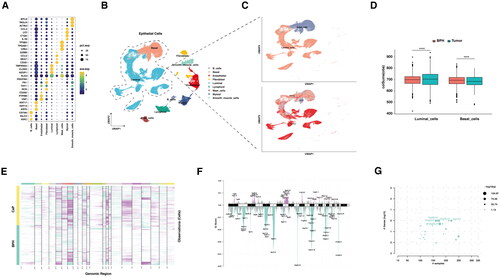
The specific activation of MYC and FOS transcriptional factors in BPH
To further understand the driving force of transcriptomic changes between BPH and CaP, the activity of transcriptional factors (TFs) was screened across scRNA-seq datasets. Of all the TFs regulons (TFs and their target genes), most of them were generally active in both BPH and CaP. Here, the relatively exclusive TFs were selected and visualized in a heatmap (). To be more specific in the location of cell types, feature plots of TFs activity indicated that FOSL1 and MYC were activated explicitly in BPH, but not normal prostate or CaP (). SPDEF was exclusively activated in luminal cells of CaP (). CEBPD and ELF3 were more active in normal prostate, compared with BPH or CaP, while XBP1 was suppressed in normal prostate, but not BPH or CaP (). The G.O. analysis of shared genes between the BPH regulons and modules indicated that the cytokines signaling pathway was the most significantly enriched (Figure S2). In addition, lipid metabolism and histone deacetylation were dominantly enriched in the prostate cancer group (Figure S3).
Figure 3. The difference in transcription factors between BPH and prostate cancer.
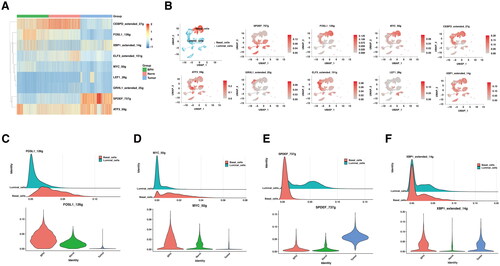
Identification of unique and shared gene signatures in BPH and prostate cancer
In keeping with previous findings, we explore the transcriptomic features in BPH, CaP, and CRPC, to further validate the conclusions of genomic and TFs analysis. Here, the genes of DEGs and significant modules from WGCNA were selected to find the overlap signatures (). Of note, the BPH and CaP possess reversed important modules according to WGCNA (). In keeping with that, none of the genes in the DEGs and modules between BPH and CaP were overlapped (). However, five overexpressed genes (H2BC15, PSMA2, H4C3, LIMD2, ATP1B2) were found in the BPH DEGs and significant modules (). In addition, the five gene signatures were not significantly associated with the prognosis of prostate cancer (). Their shared genes were found to be functionally active in molecular functions toward translation, post-translational modification, or metabolic pathways (). As BPH and CRPC shared mutual modules, we next select the overlapped genes derived from DEGs and WGCNA modules (, ). Forty-two genes were shared by BPH and CRPC according to WGCNA, which were mainly focused on the MAPK phosphorylation and MYC signaling pathways (). PPI network analysis suggested that the RPL4, FARSA, EIF3G, SNRPA, and OXA1L were the top five hub genes. To further filter the gene signatures related to the prognosis of prostate cancer, DDA1, ERG28, OGFOD1, and OXA1L were found by lasso regression analysis (). The mRNA expression of four gene signatures was significantly increased in BPH compared with normal prostate and was further upregulated in CaP (). Interestingly, the expression of four genes was decreased after the progression to CRPC (, Figure S4A–C). Importantly, these genes failed to be correlated with the prognosis of CaP in TCGA (). However, the expression of DDA1 and ERG28 (C14orf1) were significantly associated with the poor prognosis of patients with anti-PD1 therapy (, Figure S4D–F).
Figure 4. The unique gene signatures and signaling pathways in BPH.
PPI, protein–protein interaction; DEGs, differential expression of genes; BPH, benign prostate hyperplasia; CaP, prostate cancer; CRPC, castration-resistant prostate cancer; G.O., gene ontology.
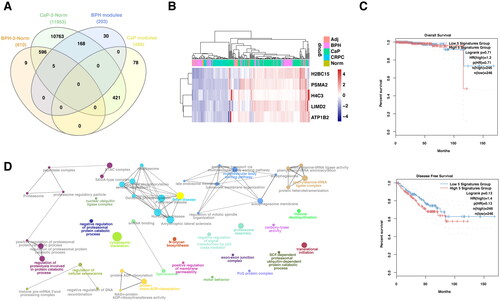
Figure 5. The role of mutual gene signatures between BPH and CRPC in prognosis and anti-PD1 response.
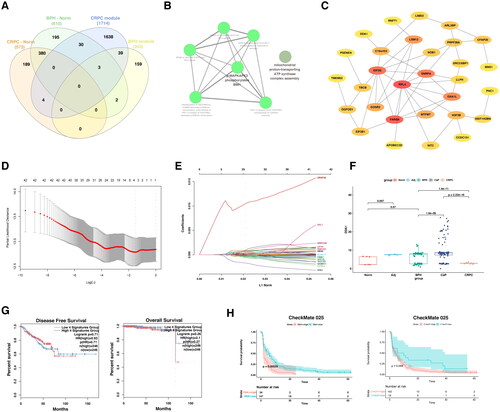
The shared immune microenvironment in BPH and CRPC
To investigate the immune state of prostate tissues, the enrichment levels of 29 immune-related cells and types in the normal prostate, adjacent tissues, BPH, CaP, and CRPC were shown in the heatmap. The ESTIMATE Score, Immune Score, Stromal Score, and Stromal Score of each case were presented and colored by intensity (). After calculating the ssGSEA score representing the immune cells in the prostate, a Pearson’s correlation analysis between each immune cell was performed (). The ESTIMATE score, immune score, stromal score, and tumor purity were statistically compared among prostate lesions (). Notably, the high level of CD274 (encoding PDL1) and TIGIT expression, the canonical immune co-inhibitory factors, were significantly increased in the CaP group, compared with BPH or CRPC groups (). The relative percentage of annotated immune cells in 206 prostate tissues was shown in a bar plot (). Compared with CaP, the infiltration of CD8+T cells, M1 macrophage, and N.K. cells was significantly increased in BPH and CRPC (). However, the proportion of M2 macrophage was relatively high in both CaP and CRPC but not in BPH, adjacent tissues, and normal prostate ().
To further illustrate the relation between the gene signatures and the immune microenvironment, the ssGSEA and ImmuCellAI algorithms were applied [Citation21,Citation22]. The individuals of the gene set were significantly correlated with a suppressive immune profile (). Of note, DDA1, ERG28, OGFOD1, and OXA1L were all negatively and significantly correlated with the infiltration of CD8+ T cells (). In keeping with previous findings, the increase of indicated genes’ CNVs also correlated with the decrease of CD8+ T cells, and increase of exhausted infiltrate in prostate cancer (, Figure S5A). More importantly, the gene set constituted of DDA1, ERG28, OGFOD1, and OXA1L significantly correlated with an immune-suppressive tumor-microenvironment (, Figure S5B).
Figure 6. The comparison of immune microenvironment between BPH and CRPC.
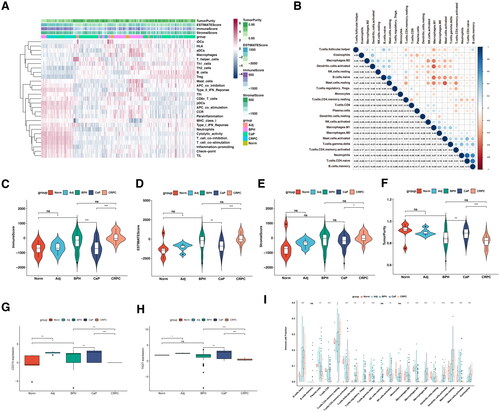
Figure 7. The correlation between selected gene signatures with the tumor immune microenvironment.
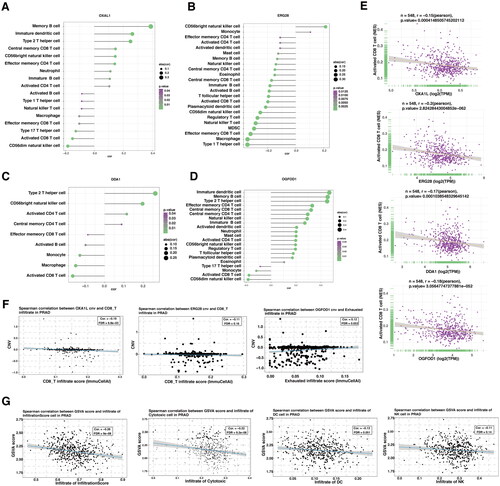
Discussion
In this study, WGCNA analysis indicated that the significant modules in BPH and CaP were almost mutually exclusive. However, the BPH and CRPC shared multiple significant modules in WGCNA. Further analysis suggested that the mutual gene signature was significantly associated with decreased infiltration of anti-tumor immune cells and a suppressive microenvironment. Above all, a four-gene signature (DDA1, ERG28, OGFOD1, and OXA1L) was associated with the shared immune features in BPH and CRPC, as well as the formation of tumor microenvironment in CaP.
Previous findings indicated that the deprivation of androgen signaling altered in the immune microenvironment in CaP contributed to the failures of various therapies for CRPC [Citation23]. But the role of the immune system in the development of CRPC is largely unclear. Our data suggested that the transcriptomic profiles between BPH and CaP were distinct. This could be explained by the dominant expansion of luminal cells in CaP and the proliferation of basal epithelial cells in BPH [Citation24]. Further analysis proved that these mutual gene signatures were mainly activated in MAPK phosphorylation and MYC signaling pathways and correlated with the suppressive tumor microenvironment. The ssGSEA analysis of the constitution of immune cells showed that M2-type macrophage was increased in both CaP and CRPC, compared with BPH and normal prostate. However, the infiltration of CD8+ T lymphocytes, natural killers, and M1-type macrophages was significantly increased in both BPH and CRPC compared with hormone-native prostate cancer. Overall, the immune infiltration scores in CPRC were improved compared to CaP. Since the androgen receptor inhibited the CD8+ T cell-driven anti-tumor immune response, it is plausible that the long-term administration of androgen-axis blockade could remodel the tumor microenvironment to a less suppressive state [Citation25]. Clinically, the autologous cellular product consisting of activated antigen-presenting cells has proved effective in metastatic CRPC [Citation26]. Moreover, preclinical studies have shown that the anti-IL23 antibody had synergistic effects with enzalutamide in the disease control of CRPC [Citation27]. Of note, an ongoing phase 3 clinical trial will confirm the role of anti-PD1 antibodies with or without docetaxel and prednisone/prednisolone in metastatic CRPC patients [Citation28]. Above all, although CaP is a “cold” tumor, patients with CRPC could benefit from immunotherapy. Unlike renal cell carcinoma, the biomarkers for tumor immune microenvironment in prostate cancer have not been extensively explored [Citation29].
Although multiple strategies are available for patients with prostate cancer, there remains an unmet need for biomarkers to predict or prevent the occurrence and progression of prostate cancer. Rebeccca et al. reported that activation of Wnt signaling in prostate cancer may serve as prognostic biomarkers [Citation30]. In this study, we globally screened the potential biomarkers based on immune features in BPH and CRPC. Briefly, the expression of DDA1, ERG28, OGFOD1, and OXA1L were significantly correlated with the immune microenvironment in BPH and PCa. DDA1, a novel oncogene, has been reported to be a potential novel target for lung cancer treatment, and a biomarker for tumor prognosis [Citation31]. ERG28 was functionally active in cholesterol synthesis [Citation32]. OGFOD1, a prolyl hydroxylase, could promote cancer proliferation by regulating the expression of cell cycle regulators [Citation33]. Recently, OXA1L was found to be required for the assembly of multiple respiratory chain complexes [Citation34]. Further studies are needed to elucidate the molecular role of DDA1, ERG28, OGFOD1, and OXA1L in BPH and CaP.
However, the asymmetric sample size during the WGCNA analysis could introduce potential bias, which was a primary limitation of this study. It should be noted that the tissues from BPH, CaP, and CRPC, but not the normal prostate and adjacent tissues, were the focus of this study. Thus, the relatively small size of the normal control might not affect the major results.
In conclusion, our study identifies novel and mutual gene signatures (DDA1, ERG28, OGFOD1, and OXA1L) in BPH and CRPC, and was closely related to a suppressive tumor microenvironment and clinical prognosis. Targeting these gene signatures would be a promising way to remodel the tumor microenvironment and ameliorate the risk of prostate cancer. Thus, our study provides novel insights into immunotherapeutic strategies for prostate cancer patients.
Ethical approval
Ethical approval was waived by the institutional ethics committee because data are obtained from public databases, and all the patients are de-identified.
Supplemental Material
Download MS Word (3.8 MB)Supplemental Material
Download MS Excel (228.5 KB)Acknowledgments
This work benefited from open source of public databases. We appreciated the efforts made by the researchers and staffs to expand and improve the databases.
Disclosure statement
No potential conflict of interest was reported by the authors.
Data availability statement
The original contributions presented in the study are included in the article/Supplementary Table S1, further inquiries can be directed to the corresponding author. All the scripts used in this study could be obtained by request to the corresponding author.
Additional information
Funding
References
- Lerner LB, McVary KT, Barry MJ, et al. Management of lower urinary tract symptoms attributed to benign prostatic hyperplasia: AUA GUIDELINE PART I-Initial work-up and medical management. J Urol. 2021;206(4):806–817.
- Fiard G, Stavrinides V, Chambers ES, et al. Cellular senescence as a possible link between prostate diseases of the ageing male. Nat Rev Urol. 2021;18(10):597–610.
- Sarkar P, Malik S, Banerjee A, et al. Differential microbial signature associated with benign prostatic hyperplasia and prostate cancer. Front Cell Infect Microbiol. 2022;12:894777.
- Iversen P, Roder MA. The early prostate cancer program: bicalutamide in nonmetastatic prostate cancer. Expert Rev Anticancer Ther. 2008;8(3):361–369.
- Crawford ED, Eisenberger MA, McLeod DG, et al. A controlled trial of leuprolide with and without flutamide in prostatic carcinoma. N Engl J Med. 1989;321(7):419–424.
- Kraehenbuehl L, Weng CH, Eghbali S, et al. Enhancing immunotherapy in cancer by targeting emerging immunomodulatory pathways. Nat Rev Clin Oncol. 2022;19(1):37–50.
- Passaro A, Brahmer J, Antonia S, et al. Managing resistance to immune checkpoint inhibitors in lung cancer: treatment and novel strategies. J Clin Oncol. 2022;40(6):598–610.
- Zhang J, Huang D, Saw PE, et al. Turning cold tumors hot: from molecular mechanisms to clinical applications. Trends Immunol. 2022;43(7):523–545.
- Alcaraz A, Hammerer P, Tubaro A, et al. Is there evidence of a relationship between benign prostatic hyperplasia and prostate cancer? Findings of a literature review. Eur Urol. 2009;55(4):864–873.
- Qian X, Xu D, Liu H, et al. Genetic variants in 5p13.2 and 7q21.1 are associated with treatment for benign prostatic hyperplasia with the alpha-adrenergic receptor antagonist. Aging Male. 2017;20(4):250–256.
- Sreenivasulu K, Nandeesha H, Dorairajan LN, et al. Gene expression of insulin receptor, insulin-like growth factor increases and insulin-like growth factor-binding protein-3 reduces with increase in prostate size in benign prostatic hyperplasia. Aging Male. 2018;21(2):138–144.
- Sreenivasulu K, Nandeesha H, Dorairajan LN, et al. Over expression of PI3K-AkT reduces apoptosis and increases prostate size in benign prostatic hyperplasia. Aging Male. 2020;23(5):440–446.
- Song JH, Hwang B, Chung HJ, et al. Peanut sprout extracts cultivated with fermented sawdust medium inhibits benign prostatic hyperplasia in vitro and in vivo. World J Mens Health. 2020;38(3):385–396.
- Fu X, Wang D, Shu T, et al. LncRNA NR2F2-AS1 positively regulates CDK4 to promote cancer cell proliferation in prostate carcinoma. Aging Male. 2020;23(5):1073–1079.
- Zhao AN, Yang Z, Wang DD, et al. Disturbing NLRP3 acetylation and inflammasome assembly inhibits androgen receptor-promoted inflammatory responses and prostate cancer progression. FASEB J. 2022;36:e22602.
- Zhang L, Wang Y, Qin Z, et al. Correlation between prostatitis, benign prostatic hyperplasia and prostate cancer: a systematic review and meta-analysis. J Cancer. 2020;11(1):177–189.
- Aran D, Looney AP, Liu L, et al. Reference-based analysis of lung single-cell sequencing reveals a transitional profibrotic macrophage. Nat Immunol. 2019;20(2):163–172.
- Langfelder P, Horvath S. WGCNA: an R package for weighted correlation network analysis. BMC Bioinf. 2008;9:559.
- Heberle H, Meirelles GV, da Silva FR, et al. InteractiVenn: a web-based tool for the analysis of sets through Venn diagrams. BMC Bioinf. 2015;16(1):169.
- Aibar S, Gonzalez-Blas CB, Moerman T, et al. SCENIC: single-cell regulatory network inference and clustering. Nat Methods. 2017;14(11):1083–1086.
- Miao YR, Zhang Q, Lei Q, et al. ImmuCellAI: a unique method for comprehensive T-cell subsets abundance prediction and its application in cancer immunotherapy. Adv Sci. 2020;7(7):1902880.
- Liu CJ, Hu FF, Xia MX, et al. GSCALite: a web server for gene set cancer analysis. Bioinformatics. 2018;34(21):3771–3772.
- Qian S, Xia J, Liu H, et al. Integrative transcriptome analysis identifies genes and pathways associated with enzalutamide resistance of prostate cancer. Aging Male. 2018;21(4):231–237.
- Xie Q, Liu Y, Cai T, et al. Dissecting cell-type-specific roles of androgen receptor in prostate homeostasis and regeneration through lineage tracing. Nat Commun. 2017;8:14284.
- Pala L, De Pas T, Conforti F. Boosting anticancer immunotherapy through androgen receptor blockade. Cancer Cell. 2022;40(5):455–457.
- Kantoff PW, Higano CS, Shore ND, I.S. Investigators, et al. Sipuleucel-T immunotherapy for castration-resistant prostate cancer. N Engl J Med. 2010;363(5):411–422.
- Calcinotto A, Spataro C, Zagato E, et al. IL-23 secreted by myeloid cells drives castration-resistant prostate cancer. Nature. 2018;559(7714):363–369.
- Petrylak DP, Ratta R, Gafanov R, et al. KEYNOTE-921: phase III study of pembrolizumab plus docetaxel for metastatic castration-resistant prostate cancer. Future Oncol. 2021;17(25):3291–3299.
- Ke J, Chen J, Liu X. Analyzing and validating the prognostic value and immune microenvironment of clear cell renal cell carcinoma. Anim Cells Syst (Seoul). 2022;26(2):52–61.
- Fisher RR, Pleskow HM, Bedingfield K, et al. Noncanonical WNT as a prognostic marker in prostate cancer: you can’t always get what you WNT. Expert Rev Mol Diagn. 2020;20(2):245–254.
- Cheng L, Yang Q, Li C, et al. DDA1, a novel oncogene, promotes lung cancer progression through regulation of cell cycle. J Cell Mol Med. 2017;21(8):1532–1544.
- Capell-Hattam IM, Fenton NM, Coates HW, et al. The non catalytic protein ERG28 has a functional role in cholesterol synthesis and is coregulated transcriptionally. J Lipid Res. 2022;63(12):100295.
- Fujisaki T, Saito K, Kikuchi T, et al. The prolyl hydroxylase OGFOD1 promotes cancer cell proliferation by regulating the expression of cell cycle regulators. FEBS Lett. 2022 Dec 4. doi: 10.1002/1873-3468.14547. Epub ahead of print. PMID: 36464654.
- Thompson K, Mai N, Olahova M, et al. OXA1L mutations cause mitochondrial encephalopathy and a combined oxidative phosphorylation defect. EMBO Mol Med. 2018;10(11):e9060.