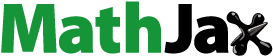
Abstract
Background: It is estimated that one in 10 people in the US have a diagnosis of diabetes. Type 2 diabetes accounts for 95% of all cases in the US, with annual costs estimated to be $246 billion per year. This study investigated the impact of a glucose-measuring intervention to the burden of type 2 diabetes.
Objective: This analysis seeks to understand how professional continuous glucose monitoring (professional CGM) impacts clinical and economic outcomes when compared to patients who are not prescribed professional CGM.
Methods: This study utilized a large healthcare claims and lab dataset from the US, and identified a cohort of patients who were prescribed professional CGM as identified by CPT codes 95250 and 95251. It calculated economic and clinical outcomes 1 year before and 1 year after the use of professional CGM, using a generalized linear model.
Results: Patients who utilized professional CGM saw an improvement in hemoglobin A1C. The “difference-in-difference” calculation for A1C was shown to be –0.44%. There was no statistically significant difference in growth of total annual costs for people who used professional CGM compared to those who did not ($1,270, p = .08). Patients using professional CGM more than once per year had a –$3,376 difference in the growth of total costs (p = .05). Patients who used professional CGM while changing their diabetes treatment regimen also had a difference of –$3,327 in growth of total costs (p = .0023).
Conclusion: Significant clinical benefits were observed for patients who used professional CGM. Economic benefits were observed for patients who utilized professional CGM more than once within a 1-year period or who used it during a change of diabetes therapy. This suggests that professional CGM may help decrease rising trends in healthcare costs for people with type 2 diabetes, while also improving clinical outcomes.
Introduction
Diabetes, especially type 2 diabetes, is an epidemic on a global and national scale. The International Diabetes Federation estimated that 415 million adults have been diagnosed with diabetes, and that every 6 seconds a person dies from diabetesCitation1. In addition to the individual burden of diabetes, there is a tremendous economic burden associated with this disease. The same study estimates $673 billion dollars is spent annually on diabetes healthcare worldwide, while in the US, this is estimated to be $246 billion. Healthcare costs continue to rise exponentiallyCitation2,Citation3.
There is an unmet need for effective and low cost diabetes interventions. One such approach is to use professional continuous glucose monitoring (CGM) to help identify glycemic patterns and fashion patient-specific treatment regimens. Professional CGM is a type of continuous glucose monitoring, where the patient wears the device for a short period of time (3–7 days), and the information gathered from the device is assessed by a healthcare provider. Professional CGM may also be referred to as “masked” or “retrospective” CGMCitation4. This is substantially different from personal CGM, which is used primarily by patients with type 1 diabetes to guide their personal management throughout the day and night for indefinite periods of time. As a result, the cost of professional CGM is a small fraction of that of personal CGM, because it is used on an intermittent basis, as opposed to continuously.
The use of professional CGM has shown to improve hemoglobin A1C (A1C), a blood test reflecting the mean glucose over the previous 2–3 months, in people with both type 1 and type 2 diabetesCitation5–9. Currently, there is a paucity of evidence about the economic impact of this device and procedure. Despite the widespread use of the device, a literature search of MEDLINE/PubMed conducted for the years 2005–2016 for terms including health economics, healthcare utilization, claims data, and cost effectiveness for professional CGM yielded no results related to the associated healthcare utilization and its health insurance claims data.
The primary objective of this study was to identify the expenditures and clinical outcomes, i.e. A1C, associated with the use of professional CGM in patients with type 2 diabetes using data from a de-identified healthcare claims dataset from the US.
Methods
Data source
We utilized a sub-set of data from Clinformatics Datamart (OptumInsight, Eden Prairie, MN) which contains de-identified health plan administrative data for individuals in the US. This database is composed of de-identified patient level healthcare insurance eligibility, enrollment, medical and pharmacy claims from commercial employer-sponsored and Medicare-age populations. All data were completely de-identified and in compliance with the Health Insurance Portability and Accountability Act (HIPAA). Therefore, Institutional Review Board (IRB) approval was unnecessary for this analysis.
To measure costs, all available claims for inpatient, outpatient, and pharmacy benefits were used. The cost data include both the payer’s cost and the patient’s out of pocket responsibility. The date of service for an individual claim was used to determine relevant date variables. Patients with claim adjustments resulting in a total cost of $0 or less during the analysis periods were excluded. All costs measured were adjusted for inflation to be equivalent to 2015 US Dollars.
Study population
We used a sub-set of claims data for people with a diagnosis of diabetes as identified by their ICD-9 codes. There were 6.2 million patients from the data contained in years 2009–2014.
To determine patients who used professional CGM, we identified individual patients with a claim containing CPT codes 95250 and subsequently a claim for CPT code 95251. CPT code 95250 represents the initial placement of a professional CGM device on the patient, while CPT code 95251 represents the healthcare provider’s subsequent interpretation of data from the device. The date of service associated with the patient’s first instance of CPT code 95250 was considered the index date. We identified 16,647 individuals who had professional CGM. In order to ensure that only professional CGM users were identified and not personal CGM users, we excluded patients who had evidence of personal CGM claims (HCPCS codes A9276, A9277, A9728, and respective NDC codes) within 1 year before or 1 year after the index date. We then confirmed a patient’s diagnosis of type 2 diabetes by evidence of two outpatient visits for the patient containing a diagnosis of type 2 diabetes, or at least one inpatient or emergency room claim with a diagnosis of type 2 diabetes. The patients’ dataset also had to have evidence of use of oral or injectable glucose-lowering medication within 12 months before or 12 months after the index date. To ensure that full cost data was included in the analysis, patient data was excluded where patients did not have a status of continuous enrollment in their health plan 1 year before and 1 year after the index date. We excluded patients who were less than 18 years old and who did not stay on a diabetes therapy continuously within 1 year after the index date. This resulted in a cohort of 6,113 professional CGM patients. We also identified a sub-set of individuals whose A1C test data were available and had a professional CGM evaluation during the study period.
In order to identify a control cohort, we initially identified 1.08 million unique patients who did not use a CGM product. Patients with two consecutive calendar years of continuous claims data were selected in order to match the timespan of the professional CGM study period. In order for these patients to be included in this control cohort, the patients had to have two consecutive years of continuous enrollment to the health plan, had to have at least two claims with a diagnosis of type 2 diabetes, at least one diabetes medication prescribed, were at least 18 years old, and had no claims for professional CGM or other CGM products in their relevant dataset. If a patient had two or more time periods meeting the criteria above, then one of the 2-year periods were selected at random. Identical to the professional CGM study cohort, patients who discontinued therapy during the study period were excluded, as well as those who had total costs less than $0 due to factors such as claim adjustments. This resulted in 670,812 unique patients included in the control cohort prior to propensity-score matching. illustrates the flow and number of patients included in the analysis.
Baseline characteristics
Before propensity score matching, as shown in , the professional CGM cohort and the control cohort had a similar mix of patients by gender and age. There were discrepancies among other key demographics and diabetes co-morbidity status for the patients in the control cohort. Discrepancies included that the control patients were slightly younger, and had less co-morbid conditions as measured by Charlson Comorbidity Index (CCI) than the professional CGM group.
Table 1. Baseline characteristics based on data from pre-index period (1 year prior to index date).
Propensity score matching was used to isolate the effects of the professional CGM treatment on outcomes separate from any other confounding factors. In order to mitigate this discrepancy of baseline characteristics, we utilized propensity score matching in a generalized linear model to create a matched pair for the professional CGM group that had similar baseline characteristics as calculated from the data from 1 year prior to the index date.
Due to the rise in healthcare costs, most patients were expected to have an increase of healthcare spending on average over the study period. Therefore, we utilized a “difference-in-difference” approach to calculate the change in cost growth or trajectory between the professional CGM cohort and control cohorts. In we represent the basic design of the analysis approach.
Table 2. Difference-in-difference design.
In order to calculate the propensity score, a logistic regression was used based on the following covariates from the baseline period: the patients’ age, gender, insurance, co-morbidities, geographic region, inpatient admission frequency and costs, 1 year total costs from the baseline period, the patient’s Charlson Comorbidity Index (CCI), year of data source, and evidence of the use of endocrinology specialists or primary care physicians.
Patients were included in the control group based on their individual propensity score falling within a set range (caliper) of the professional CGM group. The caliper was set as 0.20 of pooled standard deviation of logit of the propensity score. There were 5,677 individuals in the overall cohorts identified for matching. A sub-set of 1,408 patients with professional CGM and A1C data were matched to an identical number of patients from the control cohort. The patients’ data contained at least one A1C lab test value in both the baseline and post-periods. describes the final populations after matching.
A generalized linear regression was used to mitigate the impacts of unobserved characteristics that are constant across time between the professional CGM and control cohorts. The model is described below, where Y is the annual outcome for person i in period t. Post is an indicator variable indicating whether time is X. TX is an indicator for whether the member had professional CGM. Xs represents baseline characteristics. The coefficient β3 represents the difference-in-difference.
To conduct these analyses, we used SAS version 9.3, Microsoft SQL, and Microsoft Excel 2010. Microsoft SQL was used to extract the data from the claims dataset. SAS 9.3 was used to conduct statistical analysis, including the propensity score matching, and generalized linear model. Microsoft Excel was used for summary statistical analysis, and development of tabular references for the study.
Sub-group analysis
We identified multiple sub-groups of patients to determine how professional CGM impacted two common methods of delivery. The sub-groups of interest were consistent with indications of the therapy that were used in many health plan’s medical policies for professional CGMCitation11. We analyzed the data where professional CGM was used during a change in therapy, and, separately, we analyzed data where patients received professional CGM services more than once within 1 year. A change in therapy was defined as adding, removing, or changing a category of diabetes therapy from one 6-month period compared to the preceding 6-month period. For example, a patient who used Metformin 6 months prior to the index date, and then used Metformin and a DPP-4 inhibitor medication 6 months after the index date would be included as a patient experiencing a diabetes therapy change.
Results
The study cohort, and all sub-cohorts assessed, are described below and in , and . In the baseline period, mean total costs for the Pro CGM cohort were $23,021 per patient per year compared to the control cohort average cost per patient per year of $21,502. For the year following the index date, Pro CGM patient mean total cost increased to $26,525, and the control cohort mean costs increased to $23,736 per patient per year on average. This resulted in a non-statistically significant “difference-in-difference” of growth of total cost of $1,270 per patient per year higher for the users of professional CGM (p = .08).
Table 3. Baseline characteristics after propensity score matching at baseline.
Table 4. Economic analysis results.
Table 5. A1C analysis results.
We analyzed a sub-cohort of the patients described above with A1C lab values that were available during the analysis periods. There were 1,408 patients in each group after propensity matching. There was a clinically and statistically significant “difference-in-difference” of –0.44% in A1C favoring the Pro CGM cohort (p < .001).
There were 1,652 patients who experienced a change in diabetes therapy compared to the pre-index baseline period. After propensity matching, the mean total costs in the baseline period for the Pro CGM and control cohorts were $23,490 and $22,106, respectively. Following the index dates, both the Pro CGM and control cohorts’ costs per patient per year were $26,694 and $28,637, respectively. The calculated “difference-in-difference” of growth in 1-year total cost was –$3,327 lower for the Pro CGM cohort (p = .0023).
There were 621 patients who received at least one additional Pro CGM service after the index date. The mean 1-year baseline total costs were $24,403 in the pre-index period for those who used Pro CGM, compared to $22,070 for the propensity-matched control cohort. In the 1 year following the index date, Pro CGM patients’ total costs increased to $25,616 per patient per year, and, for the control cohort, the costs rose to $27,059 per patient per year. The resulting “difference-in-difference” of annual cost growth was –$3,776 less per patient per year for the Pro CGM cohort (p = .05).
Discussion
The data suggest that, when compared to a control group of patients with similar demographics and clinical characteristics, professional CGM improves both clinical (reduced A1C) and economic (total cost) outcomes. The overall improvement in A1C seen in the cohort containing all patients was significant. While it is important to note that there was a difference in the value of mean baseline A1C between the professional CGM and control cohorts, there was reduction of A1C from the baseline period to the post-period for the professional CGM cohort. The mean A1C levels observed in the analysis for both the professional CGM and control cohort were higher than what is recommended by the American Diabetes AssociationCitation5. A slightly higher growth in total cost was observed overall for the professional CGM cohort, but that growth in cost was not statistically significant compared to the control cohort.
There was a significant avoidance of costs (and improvement of glycemic control) for those who had a therapy change or had a second professional CGM service. For the analysis of patients who had a second professional CGM service, the p value was .05. While this meets our threshold for significance, the value is the minimum value of significance. The improvements measured in these analyses are not surprising since the purpose of a professional CGM examination is to identify glycemic patterns and then individualize treatment based on these observations.
There are several potential factors that could contribute to the difference in growth of total costs among the analyzed patient data. The analysis demonstrates that patients who utilize professional CGM more than one time per year see both a clinical benefit and an economic impact. The magnitude of the A1C result is consistent with the published literature regarding professional CGMCitation6–10. The difference in costs could be explained by individual patient selection of points of care (e.g. using an emergency department compared to a physician’s office), by changes in diabetes or non-diabetes therapy selection (e.g. by the responsible healthcare provider), or the patient’s therapy adherence.
Diabetes therapies are often adjusted for patients who are not meeting their treatment goals. This is a common practice, and not all therapy adjustments are successful. For the groups of patients who experienced a change in diabetes therapy regimen, there were clinical and economic improvements observed for the cohort who used professional CGM. Since both the intervention and control cohorts were identified to have therapy changes, this result is particularly informative. This suggests that professional CGM may be a more efficient means to assess and monitor a patient’s glycemic control during a change of diabetes therapy, compared to therapy changes without professional CGM. The result quantifies how professional CGM can be used as a tool for healthcare providers and how such action has the potential to reduce the growth of diabetes-related healthcare spending.
There are limitations to this study. The first is the large reduction in the number of patients who were included in the A1C study cohort compared to those included in the overall study cohort. This was the result of the unavailability of baseline and post-professional CGM A1C values for many patients in the dataset. This limitation is inherent in the database that was used for the study, as not all patients with cost data had lab data available during the analysis periods. In addition, by not including baseline A1C values as a covariate for propensity matching, it is possible that there may have been significant differences related to the health of patients in the control cohort when compared to the professional CGM cohort. It is important to note that, despite matching of diabetes comorbidities, there is disparity between A1C values in the control cohort and professional CGM cohort. This may raise questions about the correlation of A1C and presence of diabetes complications through diagnosis coding in this analysis.
The second limitation is that, due to the design of the study, it is possible that the values that were assigned for patients’ A1C information were not from the most clinically relevant time period. The standard of care is that A1C levels are determined 2–4-times per year based on the patient’s underlying glycemic control and clinical symptoms. For example, it is possible that a patient may have their A1C value from a very short time period after the index date. Conversely it is possible that a patient's A1C value may be from a date exceedingly far prior to the index date. This may lead to over or under-representation of patients’ A1C values. In this analysis for the baseline period, we used the A1C value that was within 1 year prior to the index date and closest in time to the index date. For the study period, the A1C value used was the one nearest to the index date, but within 1 year subsequent to that date.
The third limitation is that our data demonstrate association of professional CGM with economic and clinical benefits, but does not necessarily prove causation. Despite the use of propensity score matching, it is possible that differences in the cohorts that were not observable in the data sets could have affected the results. These are common limitations of retrospective claims data analysis. Another limitation which may impact our conclusions is the possibility of selection bias on changes over time, such as the presence of unknown factors affecting the patient or the patient’s data that coincides with the analysis periods. Another possible effect on the outcomes of this analysis is the chance of “confounding by indication”, where patients using a certain intervention, such as professional CGM, happen to be immeasurably different than those who did not use the intervention. In addition, the dataset might have contained incomplete information as patients commonly change health plans in the US on a yearly or biannual basisCitation12. It is also possible that the results were influenced by the medications or individual physician actions associated with that patient. We believe that the sample size as presented in the analysis is sufficient to show a generalizable population of patients that would be seen in a health plan in the US.
While the results of the analysis show positive outcomes for patients using professional CGM, the results may be influenced by factors other than professional CGM. Selection bias in patient treatment could be a significant factor driving the difference in cost growth. For example, a significant number of professional CGM cohort patients could be associated with seeking more treatment procedures, compared to the control cohort. There is some evidence that A1C is associated with variance in costCitation13, and, therefore, one potential reason for the difference in costs could be due to the difference in baseline A1C levels observed between the professional CGM and control cohort.
The methods by which we identified a therapy change in patients were based on the date a patient filled their prescription and the amount of medication dispensed. Our analysis was not able to detect smaller therapy changes that may have occurred, such as a changed dosage or a change in brand of medication used.
For the timeframe of data that were included in the study, there were only two FDA approved professional CGM products available on the market. These products were the Medtronic iPro 2 professional CGM, and the Dexcom G4 professional CGM. Since professional CGM is represented and documented in the healthcare claims as a CPT code, it is not possible for us to ascertain the brand name or the specific professional CGM product that was used during that specific professional CGM service. As stated in the method section, we excluded patients who had any claims for personal CGM within their dataset. While this exclusionary filter was applied, it is possible that some of the patients were using personal CGM devices during their professional CGM encounter.
Conclusion
Type 2 diabetes remains a major burden for patients and healthcare systems. Our data suggest that the use of professional CGM as a diagnostic tool is associated with decreased growth of cost and improved outcomes for people with type 2 diabetes, when compared to a control cohort. Accordingly, healthcare providers and health plans should consider such tools that benefit both patients and the healthcare system when developing policies for management of type 2 diabetes.
Transparency
Declaration of funding
This study was sponsored by Medtronic Diabetes.
Declaration of financial/other relationships
JS, MS, ZF, HC, MG, FK, and RV were employees of the sponsor at the time of study completion and manuscript development. Peer reviewers on this manuscript have received an honorarium from JME for their review work, but have no other relevant financial relationships to disclose.
Acknowledgments
None reported.
References
- International Diabetes Federation. Diabetes Atlas 7th Edition. Brussels, Belgium: IDF. 2017. http://www.diabetesatlas.org/
- American Diabetes Association. Economic costs of diabetes in the U.S. in 2012. Diabetes Care 2013;36:1033–46
- Dieleman JL, Baral R, Birger M, et al. US spending on personal health care and public health, 1996–2013. JAMA 2016;316:2627-46
- Fonseca VA, Grunberger G, Anhalt H, et al. Continuous glucose monitoring: a consensus conference of the American Association of Clinical Endocrinologists and American College of Endocrinology. Endocr Pract 2016;22:1008-21
- American Diabetes Association. Standards of Medical Care in Diabetes – 2017: Summary of revisions, glycemic targets. Diabetes Care 2017;40(Suppl 1):S48-S56
- Kim SK, Kim HJ, Kim T, et al. Effectiveness of 3-day continuous glucose monitoring for improving glucose control in type 2 diabetic patients in clinical practice. Diabetes Metab J 2014;38:449-55
- Cosson E, Hamo-Tchathchouang E, Dufaitre-Patouraux L, et al. Multicentre, randomised, controlled study of the impact of continuous sub-cutaneous glucose monitoring (GlucoDay®) on glycaemic control in type 1 and type 2 diabetes patients. Diabetes Metab 2009;35:312-8;2
- Young LA, Duclos M, Marquis A, et al. Examining the role of continuous glucose monitoring (CGM) in noninsulin treated type 2 diabetes. Diabetes Care 2015;64:A235-A382. https://doi.org/10.2337/db15-932-1471
- Viswanathan M, Jain SM, Kesavadev J, et al. Use of iPro2 in real-life management of type 2 diabetes patients in India. Poster presented at the 15th Annual Diabetes Technology Society Meeting, Bethesda, Washington, 6–8 November 2014
- Kesavadev J, Vigersky R, Shin J, et al. Assessing the therapeutic utility of professional continuous glucose monitoring in type 2 diabetes across various therapies: a retrospective evaluation. Adv Ther. Published online June 30, 2017. DOI 10.1007/s12325-017-0576-s
- Highmark Medicare Services. Medicare Local Coverage Determination for Continuous Glucose Monitoring (CGM) (L31165). Baltimore, Maryland: Centers for Medicare & Medicaid Services (CMS). 2010. http://localcoverage.cms.gov/mcd_archive/viewlcd_pdf.asp?lcd_id=31165&lcd_version =11&contractor_id =167
- Short PF, Graefe DR, Schoen C. Churn, churn, churn: how instability of health insurance shapes America’s uninsured problem. The Commonwealth Fund Task Force on the Future of Health Insurance. November 2003
- Menzin J, Korn JR, Cohen J, et al. Relationship between glycemic control and diabetes-related hospital costs in patients with type 1 or type 2 diabetes mellitus. J Manag Care Pharm 2010;16:264-75