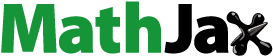
ABSTRACT
We investigate the effects of standardisation as a means of direct control on digital platform innovation. Specifically, we study the standardisation of parameters and procedures implemented in the web editing API on the popular geodata platform, OpenStreetMap. Using a regression-based approach to interrupted time series analysis, we assess the quantity and quality of new content generated on the platform before and after the standardisation. We find that the intervention had positive and negative effects on the generation of platform content on OpenStreetMap, which we summarise in three different effects (control, simplification, spill-over). Through the control effect, standardisation decreases the generation of content in quantity by enforcing conformity and reducing complementor’s freedom in producing certain outcomes. Through the simplification effect, standardisation increases the generation of new content in quantity by simplifying and streamlining the production of certain outcomes. Lastly, through the spill-over effect, standardisation increases the generation of content in quality and new areas of the platform by improving the compatibility and interoperability of content. Framing these findings through the rich body of work on standardisation and innovation in the technology management literature, we engage a long-standing tension in research on digital platforms – the balance between control and innovation. We discuss the prospect of standardisation as one way to directly control the balance between desirable and undesirable variation necessary for platforms to innovate, as it restricts some activities while enabling others.
Introduction
Digital platforms innovate by enabling external actors to generate new outputs by interacting with the platform (Boudreau, Citation2010; Cennamo & Santaló, Citation2019; Gawer, Citation2022). Depending on the type of digital platform, this innovation materialises in different forms of platform content, such as software code (e.g., extensions for SAP), media content (e.g., videos on YouTube), or information (e.g., reviews on TripAdvisor) (Hukal et al., Citation2020). This creates value for all connected parties since novel and varied content extends a platform’s possible uses, which, in turn, grows the addressable market by attracting actors to the supply and demand side of the platform. While it is desirable for platform operators to have varied and novel content created, this can never be entirely uncontrolled; undesirable and unforeseen variation reduces the quality of content and hence diminishes value for actors on either side of the platform (Boudreau, Citation2012; Wareham et al., Citation2014).
The platform literature exhibits increasing interest in controlling innovation on platforms. Much attention is paid to the challenge of attracting outside contributions in line with the strategic intentions of the platform operator (e.g., Boudreau (Citation2010), Rietveld et al. (Citation2019), Hukal et al. (Citation2020)). Reflecting some conceptual variation, this challenge has been described as the tension between ‘resourcing and securing’ (Ghazawneh & Henfridsson, Citation2013), ‘generativity and control’ (Eaton et al., Citation2015), or ‘autonomy and control’ (Wareham et al., Citation2014). A central idea echoed in this stream of research is that attracting and guiding innovation by outside contributors on platforms needs some control.
Effectively implementing any kind of control on platforms is not straightforward since it must balance ‘desirable and undesirable variation’ (Wareham et al., Citation2014) to create a situation of ‘constrained serendipity’ (Faraj et al., Citation2011). In this vein, standardisation is heralded as a promising form of control that directly engages the question of which and how innovation is enabled on a platform (e.g., Lindgren et al. (Citation2021)). Yet, finding that balance through standardisation implies identifying and controlling trade-offs between defining a common ground while allowing sufficient flexibility for new innovation to emerge.
Standardisation refers to the ‘result of intentional or unintended actions that generate order by reducing the variety of processes’ (Wright et al., Citation2012, p. 652) and work on standardisation draws on a rich tradition in the broader technology and innovation management literature (e.g., Varian et al. (Citation2005), Hawkins and Blind (Citation2017)). This body of work, however, offers conflicting views on the effects of standardisation on innovation. On the one hand, standards are seen as antithetical to innovation due to the fact that they represent organisational control and regulation, limiting the number of options to be realised (David & Rothwell, Citation1996; Wright et al., Citation2012). On the other hand, some literature views standardisation as an increasingly important factor in enabling innovation and shaping its direction (Blind, Citation2016; Teece, Citation2018; Wen et al., Citation2022). Especially as a form of formalisation, standardisation can foster innovation, for instance, when used to improve task performance rather than to control work (Adler & Borys, Citation1996; Wright et al., Citation2012; Zoo et al., Citation2017).
Work that addresses the intersection of control, platform innovation, and standardisation has thus far received scarce attention, limiting our understanding of standardisation on digital platforms. While standardisation can be promising to control activity in complex technical systems (Lindgren et al., Citation2021), the effects of standardisation are difficult to predict for digital platforms: on platforms, the generation of content is a function of diverse actors engaging with each other, and, thus, platforms innovate thanks to many very different actors whose presence and activity are not always under the control of the platform operator (Eaton et al., Citation2015; Ens et al., Citation2023; Wareham et al., Citation2014). Surprisingly, the rich body of work on standardisation and technology innovation is largely ignored in work on digital platforms. The scarce work that does exist on standardisation on platforms either surmises specific, unidirectional effects of standardisation on platform innovation (Hein et al., Citation2019; Miric et al., Citation2023; Wen et al., Citation2022) or does not speak to the nature of the relationship between standardisation and innovation on digital platforms at all (Costabile et al., Citation2022; Grøtnes, Citation2009; van de Kaa et al., Citation2022). Work on platform control, on the other hand, normally does not expose its findings to the standardisation literature, even when the means of control in focus resemble standardisation (Croitor & Benlian, Citation2019; Elaluf-Calderwood et al., Citation2011), which makes their ex-post interpretation through a lens of standardisation difficult.
Therefore, whether standardisation stifles or enables innovation is not fully understood in the platform literature, leaving much to be learned about controlling the quality and quantity of innovative content on platforms through standardisation. In this study, we engage this issue and ask: What are the effects of standardisation on digital platform innovation?
To address this research question, we assess a discrete standardisation effort in the context of the geodata platform OpenStreetMap. Specifically, we examine the standardisation of the public web editing application programming interface (API), which controls information flows between the platform and its complementors across the platform’s boundary. In this context, we collected data on the number and detail of geospatial data objects across the 100 largest European cities, spanning 100 weeks (50 weeks before and after the standardisation, respectively). Implementing an interrupted time series design, we then assessed the input and output of geo data on the platform by comparing the quantity and quality of data objects provided on the platform before and after the standardisation took effect.
We find that the standardisation had varying effects on platform innovation in the form of new content. While the number and growth rate of new data objects decreased after the standardisation, the level of detail with which data objects are stored in the database increased – but only for data objects that were not directly affected by the standardisation intervention. We summarise and explain these findings through three different effects of standardisation in the context of digital platforms. We suggest that direct control through standardisation can be considered a viable way to influence innovation on digital platforms by balancing desirable and undesirable variation across the platform.
The remaining paper is structured as follows: In the next section, we ground the paper in work on platform innovation, platform control, and standardisation. Next, we present the research design, including the empirical context, data, and analysis. Then, we present the results of our analysis and summarise them in three effects of standardisation on platform innovation. Finally, we discuss implications for theory and practice, outline boundary conditions of our findings, and provide avenues for future research.
Background
Digital platform innovation
Digital platforms provide infrastructures conducive to outside complementor contributions, allowing for the integration of innovative software applications, media content, or information that enrich the platform experience (Gawer, Citation2022; Kim et al., Citation2012; Parker et al., Citation2017). In this study, we refer to platform innovation as any new content that facilitates interaction between users or entities on a platform and provides a new source of value and growth. Platforms foster innovative contributions by establishing and maintaining arm’s-length relationships with complementing parties (Boudreau, Citation2010; Foerderer et al., Citation2021; Rietveld et al., Citation2019). In enabling others to innovate by being connected to the platform, platforms enable continuous value creation without having to create every innovation themselves.
The above poses an enormous challenge for digital platform operators: While innovative contributions are necessary and desired for the platform to create value, contributions that remain entirely unchecked are not. Leaving complementors without guidance may lead to low-quality services and complements, which harms end user’s experiences and the operator’s reputation and can ultimately contribute to the platform’s demise (Boudreau, Citation2012; Wareham et al., Citation2014). A host of studies has documented the practices platform operators engage in to guide complementors, such as selective promotion (Rietveld et al., Citation2019) or indirect communication via signalling (Adam et al., Citation2022; Hukal et al., Citation2020). Collectively, this body of work aligns with the idea that generating content on platforms requires some control to ensure the platform remains valuable to all parties (Boudreau, Citation2010; Parker et al., Citation2017).
Platform control
The platform literature acknowledges a wide array of possibilities to implement control on platforms. Drawing from control in management literature, control on platforms in this study refers to formal mechanisms used by a controller – the platform operator – to influence the behaviour of a controlee – actors on the supply and demand side – in accordance with the operator’s objectives (e.g., Ouchi (Citation1979)). As a management tool to control activity on platforms, control is typically formal and, as such, exercised through rules, regulations, or incentives that, when adopted, result in activities and outcomes that are in line with the controller’s goals (e.g., Saunders et al. (Citation2020)). Formal control requires the controlee to either reach a certain objective or adhere to specified procedures and routines during the process (Kirsch et al., Citation2002).
Work on formal control over the production of innovative platform content is far from conclusive. A prevalent idea in this space describes the abstract tension of control versus innovation that operators ought to manage. Therein, control is often described as restrictive, aiming to constrain variation that is not in line with the platform’s strategic objectives (Eaton et al., Citation2015; Ghazawneh & Henfridsson, Citation2013; Wareham et al., Citation2014). On digital platforms, this type of control commonly occurs through the manipulation of artefacts deployed by the operator to implement the platform. For example, platform operators may use control points in the technical architecture to steer interactions across platform boundaries (Ghazawneh & Henfridsson, Citation2013). This includes all forms of ‘standardised and stable interfaces’ (Eaton et al., Citation2015; Yoo et al., Citation2010), such as application programming interfaces (APIs), software development kits, or licences, used as ‘obligatory passage points’ (Tilson et al., Citation2010) through which the platform operator aims to control the quality, quantity, and variety of complements.
However, exactly how the often-cited tension between control and innovation can be resolved is mainly left unanswered. The operator’s motivation is clearly to orchestrate a portfolio of complements that encourage user adoption (e.g., Croitor and Benlian (Citation2019)). Yet, received notions of process and output control have clear drawbacks: on the one hand, output control is antithetical to the logic of innovation on platforms. On the other hand, process control, while crucial, can diminish innovation if control over the process is too tight. Instead, the upside of control is that it can be an enabler of innovation, e.g., by restricting variability in one area of the platform, which, in turn, allows variation to be introduced in another (Gawer & Henderson, Citation2007). By the same token, output control would aim to control what is – until it is generated – deliberately unknown since the whole purpose of innovation on platforms is that it can unfold freely to generate outputs that were not anticipated yet provide value (e.g., Boudreau (Citation2012)).
Standardisation as a means to control platform innovation
A promising way to implement direct formal control and ease the tension between control and innovation on platforms is through standardisation. Standardisation results from intentional or unintended actions that generate order by reducing the variety of processes (Wright et al., Citation2012). While standardisation can generally follow a committee-based, market-based, or government-based mode (Wiegmann et al., Citation2017), Nylund and Brem (Citation2023) argue that the hierarchical coordination through platform operators set standards resembles a governmental approach. It can, therefore, be understood as a mechanism of formal control, yet despite the rich body of work in the technology and innovation management literature, little attention is being paid to standardisation in the platform literature.
In general, standardisation serves different economic functions, which can be classified into four aspects: (i) achieving compatibility (i.e., standards specify properties that a product must have to work with complementary products or services), (ii) securing quality (i.e., standards mitigate information asymmetries between suppliers and customers by specifying product or service performance), (iii) codifying knowledge (i.e., standards reduce transaction costs between actors and/or organisations by defining knowledge that has gained authority through common consent), and (iv) reducing variety (i.e., standards limit products to certain range or number of characteristics, thereby reducing variety to attain economies of scale) (Swann, Citation2000; Tassey, Citation2000).
When enforcing control, platform operators often apply variety reduction standards to non-physical or functional attributes such as data formats or peripheral interfaces (Tiwana et al., Citation2010). Understanding the effects of such actions on innovative outcomes on platforms is, however, poorly understood since any variety reduction is difficult to assess due to its equifinality: it can both enhance and inhibit innovation (Tassey, Citation2000).
The traditional view in management research holds that standardisation and innovation are opposing forces (Blind, Citation2016; Swann, Citation2010). On the one hand, standards are frequently seen as ‘the antithesis of innovation’ since they represent organisational control and regulation aimed at limiting the number of options (David & Rothwell, Citation1996; Wright et al., Citation2012). Creating and implementing such options requires freedom and diversity as well as loose and more informal organisational structures. Further, setting standards in early stages of technology development can lead to premature and inferior choices, claimed to inhibit innovation. Such variety reduction creates market concentration, which lowers the pressure to innovate due to less competition (Blind, Citation2016; Swann, Citation2010). On the other hand, some literature views standardisation as an increasingly important factor in enabling innovation and shaping its direction. Evidence from industry, for example, shows how adopting standards for basic components facilitated and stimulated technological improvements (Thompson, Citation1954). The assumption is that a degree of conformity enables firms to identify areas for differentiation that lead to competitive advantages (Hawkins & Blind, Citation2017). In this vein, standardisation surpasses its alleged passive role as a conduit of innovation diffusion (Blind & Gauch, Citation2009; Zoo et al., Citation2017). Hence, standards may help coordinate innovation processes by reducing complexity and uncertainty (e.g., Varian et al. (Citation2005)). Standardisation of products and processes, for example, reduces variety, but this offers improvements through simplification or helps firms attain economies of scale and critical mass for market success. Moreover, standardisation can enable innovation by fostering a common language or by providing formalisation that helps to improve task performance rather than control work (Adler & Borys, Citation1996; Wright et al., Citation2012).
Understanding the effect of standardisation on platform innovation has received only little attention. The focus in much of this work is often on the identification of specific and unidirectional effects. For instance, Hein et al. (Citation2019) show the importance of standardising work processes through platform boundary resources to achieve value co-creation at scale to benefit new platform outputs. Additionally, Nylund and Brem (Citation2023) investigate the impact of dominant platforms on standardisation in innovation ecosystems, finding that setting standards can either drive subsequent innovation by fostering technological and organisational modularity while also inhibiting innovation when standardisation disproportionately skews value appropriation in favour of the platform operator. Further, Miric et al. (Citation2023) evaluate how the creation of new products in the console video game market is influenced by complementors using standardised development tools (e.g., game engines) instead of creating the functionality themselves. They find that using standardised tools is associated with less novel but more commercially successful products. Lastly, Wen et al. (Citation2022) study the effects of standardisation of enabling technologies on complementary innovations. They find that complementors benefit from standards (by obtaining innovations of high social value) as they reduce technological and legal uncertainty, which decreases development costs and increases innovation productivity.
In sum, the literature offers some wisdom to those wishing to understand standardisation and innovation on digital platforms. However, as David (Citation1995) has already pointed out, within the domain of information economics, the key questions to understanding their interaction puts conformity and variety at odds. Standardisation must thus tackle questions about degrees of freedom and how these are increased or decreased through interventions (Hawkins & Blind, Citation2017). Here, the understanding of the potentially varying effects of standardisation on platform innovation is incomplete. As platform content is not produced by the platform alone but by many different actors whose activity is not always under full control of the platform, any action to control must be sufficiently understood to be able to carry it out properly.
Research design
We take the conflicting views of standardisation on innovation as an opportunity to empirically explore the effects of standardisation on digital platform innovation without the test of formal hypotheses. Specifically, we study the case of the standardisation of the web editing API v0.6 on the popular geodata platform OpenStreetMap (OSM) to assess the quantity and quality of new content generated on the platform before and after the standardisation took effect. The following sections explain the study´s methodological choices, research context, and analysis techniques.
Method
We follow the tradition of exploratory, natural experiments involving policy changes in platform settings (e.g., Claussen et al. (Citation2013), Ye et al. (Citation2014), Wessel et al. (Citation2017)). Natural experiments investigate the effects of an unplanned or naturally occurring contrast between a treatment and a comparison condition that cannot be manipulated by researchers, for example, because the intervention occurred before the effect is measured (Sadish et al., Citation2002).
Analysing such an intervention, we conducted quantitative analyses of OSM geospatial data. As we explain in detail below, we tracked and compared the type and number of data objects before and after a standardisation effort (i.e., the intervention) in an approach that is referred to as interrupted time series analysis (Gottmann, Citation1981; Linden, Citation2015). We explain the details of the intervention and its assessment below.
Context
OpenStreetmap
The OpenStreetMap (OSM) platform offers and maintains a freely accessible database of geospatial data of the world. It is representative of many digital platforms (such as YouTube, Spotify, or Instagram) that rely on third-party contributions in the form of platform content (Hukal et al., Citation2020; Nagaraj, Citation2021). Launched in 2004, OSM now contains a rich variety of geographical objects (e.g., road networks, buildings, points of interest) contributed by millions of volunteers, leading to the label as the ‘Wikipedia of maps’ (Fox, Citation2012). Neither use nor contributions to the data are charged, and hence, users are ‘free of restrictions that hinder the productive use of the data’ (Ramm et al., Citation2010, p. 3). This circumstance, paired with the high level of detail, accuracy, and recency, makes the use of OSM geodata popular with developers in many complementor products.
On OpenStreetMap, users contribute content in the form of geospatial data objects. The data model consists of three types of objects: nodes, defining points in space (e.g., points of interest); ways, defining linear features (e.g., roads) and area boundaries (e.g., buildings); and relations, defining logical or geographic relationships between objects or complex objects consisting of both nodes and ways. Objects of any type can be grouped together to represent all sorts of natural or man-made geographical features (Ramm et al., Citation2010). Contributed data objects are subsequently annotated with additional metadata in the form of ‘key=value’ pairs, called tags, to carry semantic information. This is valuable to users of OSM output since more detailed geodata objects increase the number of possible use cases enabled by drawing from detailed descriptions of the geodata in various external applications (Hukal et al., Citation2020).
Enabling easy and fast content generation, OpenStreetMap uses an editing API as the central point of entry for all geodata to its database. By design, it serves as the central tool for the platform to allow specific operations on the database (i.e., storing or retrieving (meta-)data). Initially, almost no restrictions were in place for users to store and manipulate data objects. To this day, the API does not control for semantic correctness as a point of principle to invite variation. However, the API does check for some formal criteria of correctness (e.g., an object of type way can contain a maximum of 2000 nodes). In an open and collaborative environment, this led to users working differently to create objects without any repercussions (Budhathoki & Haythornthwaite, Citation2013). In many cases, this resulted in limits to how much recombination of data objects was possible due to a lack of consistency, clarity, and interoperability.
Standardisation of the OpenStreetMap API v0.6
In response to the situation described above, the OpenStreetMap community decided to apply stricter control on the data model by standardising parameters and operations implemented through the editing API. To investigate the effect of standardisation on platform innovation, we focus on a specific standardisation effort that took place with the launch of a then-new API version (0.6) of OpenStreetMap. We draw on content analysis of interviews (five background interviews with OSM developers and core members) and discussion data (self-crawled mail threads from the OSM public mailing list) to understand the standardisation effort taking place as well as pre- and post-change characteristics.
With the release of the API version 0.5, OpenStreetMap introduced relations as a new data type. Relations consist of several members (nodes, ways or other relations) that take specific roles to model logical, local, or geographical relationships between objects. Originally, members of relations were not ordered, meaning there was no database scheme to track the order that mappers deliberately sorted the members during their upload. With time, mappers also used relations for novel use cases which required ordering; for example, a relation describing a public transport route consisting of multiple (route-)segments and stops would produce useless graphs if the relations’ members were unordered. In addition, data consumers had to look into raw data and potentially stitch the segments of data together or examine the semantic information on data objects to understand whether and how the ordering of objects is justified. For illustration, consider this comment by an OSM mapper posted to the public mailing lists of the project:
I have not been adding bus stops to the routes, however, it is not really possible to add the stops “in order” since there are likely to be a number of different variants for the route. (in both directions, short running and deviations off the main route for special reasons - market days, end of school day etc)
For these and other problems, the OSM core community, acting in the fashion of the platform operator despite the community-based collaboration model (cf. Hukal et al., Citation2020), decided to enforce a standardised ordering of relation members with the next API version (0.6) to tackle the increasing interaction problems. This, however, required to make a trade-off between innovation and control (e.g., Eaton et al. (Citation2015)). In the Appendix, we present some insightful quotes from discussions on the OSM public mailing list on this topic (see ). summarises the timeline of changes.
Data
In the context described above, we constructed a unique dataset of OSM objects that covers the timeframe of ±50 weeks around the API version 0.6 change (April 2009). We downloaded an excerpt of the OSM database holding the European continent. We then created weekly time-slices of all objects and extracted all relations within the city proper of Europe’s one hundred largest citiesFootnote1 (by inhabitants). We chose this approach for several reasons: first, our observation period falls into the early phase of OSM when most mapping activities focused on Europe. Second, large urban areas represent more diverse options for mapping and are, therefore, more suitable for tracking the different uses of relations objects. Third, the higher population density in cities likely correlates with the number of mappers in the area, promising to observe a high volume of activity.
The data consist of 100 weeks, containing a total of 1.52 million relation objects and 5.15 million metadata tags describing the relation objects (see ). Each week contains the total number of relations in each of the hundred cities and a list of all tags describing them. In the following analysis, all figures of objects and metadata tags refer to numbers in weekly periodicity.
The data are particularly well suited for our analyses for the following reasons: first, there are no other (relevant) changes on the API or the OSM data model during the timeframe, making the ‘ordering of relation members’ the only intervention. Further, the natural experiment-like setting of the API change allows for a similar identification of effects as a field experiment. Second, there are no other distortions happening in the sampled locations and/or timeframe (e.g., mass data imports), which increases the validity. Last, OpenStreetMap is typical for many digital platforms that rely on third parties to generate and reuse content (especially digital data or information), making the results relevant for many other platforms.
Variables
Dependent variables
We develop several variables to measure the generation of platform content in terms of quantity and quality ( and ). In keeping with the interrupted time series design, we constructed a time series of the absolute number of data objects added to the database each week over the number of deleted objects.
Table 1. Dependent variables description and summary statistics (weekly basis).
Table 2. Correlation matrix for variables included in the regression analysis.
First, we measure the total number of relations added per week (Relations_Total). This variable describes the overall growth of relations on the OSM platform. Further, we split the number of total relations into those affected by the standardised ordering (Relations_Ordered) and those that can be regarded as unaffected because they do not require ordering (Relations_Unordered). This division accounts for the fact that not all relations on the OSM platform are affected by the intervention in the same way, and we are interested in the effects of the standardisation change on relations of different types. To classify the relations, we listed all ‘key=value’ pairs and evaluated the semantic information contained therein as to whether relations were likely required to be in a specific order. We classified relations as ‘ordered’ if they contained at least one tag of that list. This yielded a set of relations describing collections of nodes or ways (e.g., transport networks, routes, boundaries), for example, relations annotated with tags such as ‘route=’ (with values such as bicycle, horseback riding, foot, etc.) or ‘public_transport=’ (with values such as bus, tram, train, etc.). Vice versa, this approach excluded keys that are either irrelevant (e.g., ‘name=’) or are generated automatically by an editor program (e.g., ‘created_by=’), and we classified relations with these tags as ‘unordered’. Further, as one specific goal followed by the standardisation effort was to simplify and refine contributions of public transport information, we measure the number of new relations of this type added per week (Relations_Public_Transport). We derived a subset of relations from our dataset and classified those relations that used at least one key describing public transportation infrastructures (i.e., ‘subway’, ‘waterway’, ‘light_rail’). These steps were largely informed by the informant interviews with OSM core community members and the authors’ experience with OSM data.
Second, we measure the average number of tags on the absolute number of added relations per week. This variable describes the quality of new content generated on the OSM platform, as more tags used to describe a single object account for more detail and diverse use. Tags on OSM can be of three types: administrative tags, which carry internal OSM data; technical tags, which carry necessary data for the correct rendering of the object on the map (e.g., a specific symbol to use when displaying the object); and usage tags, which relate to all information that describe an object in detail and are therefore of particular interest for third parties (e.g., contact information or opening hours of a restaurant used by FourSquare). Again, we split the variable into one that measures the number of tags on relations classified as ‘ordered’ by the standardisation (Total_Tags_Ordered) and one that measures the number of tags on relations labelled as ‘unordered’ (Total_Tags_Unordered). Further, for each variable, we also measure the average number of those tags that are indicative of new use cases for consumers (Usage_Tags_Ordered and Usage_Tags_Unordered). We calculate variables 5 and 6 as the simple arithmetic mean of the number of tags over all relations each week. To calculate variables 7 and 8, we extracted a list of the unique keys (n = 993) of all tags in the dataset, manually classified each tag as administrative, technical, or usage, and tracked the growth in each category. As before, informant interviews and our own experiences guided this step.
Independent variables
Interrupted Time Series Analysis (ITSA) relies on three indicator variables: Time, a simple running count variable denoting the weeks since the start of the observation. Intervention, a binary variable that is 0 before the intervention and 1 after. Last, After is an interaction term of Time x Intervention, which starts at 1 and counts the number of weeks since the intervention.
Control variables
We include a set of variables to control for alternative explanations. In particular, we controlled for the size and growth of the entire database. We create User_Growth as a variable representing the per cent increase per week in the number of OSM mappers. This controls for the effect of more content generation due to more mappers. Further, we create GPS_Data_Growth as a variable representing the per cent increase per week of all GPS data points in the OSM database. Uploading GPS data points denotes a different way of contributing content to OSM (in comparison to basic ‘map editing’) and thus captures general mapper activity. Last, we create Other_Objects_Growth as a variable representing the per cent increase per week of data objects that are not relations (i.e., nodes and ways). This controls for potential effects of shifting mapper activity to other domains (e.g., more mapping of less complex geographical objects unaffected by the standardisation).
Approach: interrupted time series analysis
Interrupted time series analysis (ITSA) is a quasi-experimental research design in which a single treatment unit’s outcome is studied serially over time, and an intervention is expected to ‘interrupt’ the level and/or trend of that outcome (Linden, Citation2018). While contrasting the treatment unit’s outcome to a control group is regarded as the ‘gold standard’ for investigating the impact of an intervention, this is not always feasible. Interventions that impact an entire unit’s population or have occurred in the past may rule out the ability to include a control group (Turner et al., Citation2021). In these cases, ITSA is an advantageous design choice primarily due to its control over regression to the mean and high degree of internal validity (Sadish et al., Citation2002).
In ITSA, data are collected at multiple time points before and after the intervention. Modelling the data in the pre-interruption period allows estimation of the underlying non-manipulated trend, which, when modelled correctly and extrapolated into the post-interruption period, yields a counterfactual for what would have occurred in the absence of the interruption. Differences between the counterfactual and observed data at various points post interruption can be estimated (e.g., immediate and long-term effects), having accounted for the underlying trend (Turner et al., Citation2021). The underlying assumption for this is that any time-varying unmeasured confounder has a weaker impact relative to the intervention and thus can be differentiated from a sharp jump of the intervention indicator.
Statistical analyses used for ITSA must account for autocorrelated data. Approaches most frequently used in ITSA are ordinary least squares (OLS) regression models with adjusted standard errors for serial correlation, general least squares (GLS) regression models designed to adjust for heteroskedasticity and serial correlation, and autoregressive integrated moving-average (ARIMA) models (Gottmann, Citation1981). Most studies, however, rely on OLS since it is often more flexible and broadly applicable in ITSA (Linden, Citation2015).
Estimation model
Following the design of a single-group linear regression model, we assume an OLS model of the following form for our analysis:
Yt is the dependent variable (i.e., number of relations added per week) measured at equally spaced time point t. The model parameter β0 represents the intercept, β1 is the pre-intervention slope, β2 represents the change in the level of the outcome at the intervention, and β3 shows the difference between pre and post-intervention slopes. We include a vector of the three control variables to account for alternative explanations. The error term εt represents the residual of the fitted model to any value.
To adjust for potential autocorrelation of the time series, we use a first-order (lag-1) term (so-called Newey-West SE correction), where the autocorrelation parameter p is the correlation coefficient between the actual and the previous error terms and the disturbances ut are normally distributed ‘white noise’ (ut ~ N(0, o2)) (Linden, Citation2015; Turner et al., Citation2021):
Results
We report the results regarding innovation in terms of the quantity and the quality of novel contributions on the OSM platform before and after the standardisation. We follow previous studies on policy changes by first providing econometric evidence of our time series analysis before explaining and interpreting the meaning of these changes in the context of OpenStreetMap.
Effect of standardisation on quantity of new content
Descriptive statistics of the key variables measuring the quantity of content (variables 1–4) on the platform level for the whole observational period are reported in . When estimated using formulas (1) and (2) above (Models 1–4 in ), our regression-based analysis paints an ambivalent yet consistent picture of the effects of standardisation on the number of relations added to the OSM database.
Table 3. Model results for quantity of new content (number of added relations).
Across all relations (Model 1), the intervention led to a sharp drop in the overall level of new relations added to the database (β2 Interventiont = −0.539, p < 0.001). Additionally, the weekly growth after the standardisation decreases slightly but remains positive in absolute terms (β3 Aftert = −0.018, p < 0.001). For relations that were in focus of the standardisation (Model 2), the effects are similarly mixed; while the overall production of relations after the standardisation decreases (β2 Interventiont = −0.467, p < 0.01), the weekly growth remains positive albeit, slightly slows down (β3 Aftert = −0.023, p < 0.001). For relations that were not directly affected by the standardisation (Model 3), the effect is also a drop in the overall production (β2 Interventiont = −0.664, p < 0.001) as well as a decelerated growth (β3 Aftert = −0.015, p < 0.001). For relations describing public transportation (Model 4), the intervention led to a strong increase in the overall production level (β2 Interventiont = 2.094, p < 0.001). These associations are robust for controls for the relative growth in the userbase, the GPS data points in the database, and the number of objects that are not relations over the same time horizon.
is an exemplary effect plot of the linear estimate produced for the model regressed on the number of added ordered relations per week over the course of 50 weeks prior and post intervention. Therein, a drop in the overall level of content produced is discernible consistent with the coefficients of the intercept changes of all models once the standardisation takes effect (β2 in Models 1–3). In addition, the slopes of the linear fits for the period before and after the intervention clearly flatten in all three instances (coefficients β3 Aftert in Models 1–3 are negative but small enough that the coefficient of the absolute trend, β1Timet, remains positive). Taken together, this indicates that the standardisation had a significant effect on the overall growth of relations on the OSM platform in our data, slowing down the weekly growth of all relation objects after the standardisation took effect. For the subset of relations describing public transport routes, however, we find that the intervention led to a jump in the production level and stronger growth, indicating more novel relations of this type being generated. We will discuss these effects in the following section.
Effect of standardisation on quality of new content
Descriptive statistics on the platform level for the key variables (variables 5–8) measuring the level of detail (i.e., quality of content) are reported in . Overall, the findings of the analysis of the average number of tags per relation object (Models 5–8) indicate that the level of detail of new content was affected differently across relation types and tag categories.
Table 4. Model results for quality of new content (number of metadata tags on relations).
First, we find a divergent effect of the standardisation on the average number of all tags on objects before and after the intervention across ordered and unordered relations. While the effect is negative and statistically weak for ordered relations (Model 5: β2 Interventiont = −0.069, p < 0.1), it is positive and significant for relations that require no ordering (Model 6: β2 Interventiont = 0.092, p < 0.05). However, both for relations that are directly affected, as well as those relations that are not, the growth of the average number of all metadata tags per object increases after the intervention (Model 5: β3 Aftert = 0.004, p < 0.05; Model 6: β3 Aftert = 0.006, p < 0.001).
When differentiating tags by whether they contribute distinct new use cases or not, the analysis shows that the average number of tags with clear use cases only increases for unordered relations (Model 8: β2 Interventiont = 0.188, p < 0.001) but did have no effect on ordered relations (Model 7: β2 Interventiont = −0.040, n.s.). In addition, we find no effect on the growth of usage tags on either type of relation (Models 7 and 8: β3 Aftert = n.s.), indicating that the rate with which tags of any type are added to either type of relation objects is not affected by the standardisation. In combination with the results from Model 6, this suggests that the overall increase in the average number of tags on unordered relations is mainly driven by an increase in the subset of tags providing completely new use cases to users and complementors.
is an exemplary effect plot of the linear estimate produced for the model regressed on the mean number of usage tags on unordered relations per week over the course of 50 weeks prior and post intervention. Therein, a jump in the mean number of usage tags on these relations is observable after the standardisation took effect.
Robustness checks
To increase confidence in the reported associations in the data, we estimated our regression models with two alternative specifications. First, we tested our results with Prais-Winsten OLS to take potential AR(1) serial correlation into account. Second, we used a restricted likelihood general least squares estimator with a first-order autocorrelation structure in the error term. Both specifications yielded similar significance levels and identical directions of all relevant coefficients. Further, we also tested our OLS regression with an alternative specification of the dependent variable by expressing it in terms relative to the number of users in each month (by anchoring the ratio of users to relations in Time1 to x = 100) and obtained similar results.
Findings: three effects of standardisation on platform innovation
In this section, we summarise and synthesise our findings into three varying effects of standardisation on platform innovation in the form of platform content generation (see ). These effects are not an exhaustive list of the interaction of standardisation and platform content but rather an initial presentation of potential relationships based on our findings. We explain each effect in turn below, illustrated with examples from the context of OpenStreetMap.
Table 5. Overview of the observed effects of standardisation on platform innovation.
Control effect: reducing overall content generation
First, our findings imply standardisation had a controlling effect. That is, the impact standardisation had on the generation of new content in quantity. Here, standardisation appears to be an ordinary control mechanism – a quality gate that controls for conformity and that reduces the freedom of complementors in the generation of certain content – even though risking to potentially limit desirable outcomes as well (e.g., Tilson et al. (Citation2010)). This finding is consistent with what other work describes as negative impact resulting from variety reduction (Blind, Citation2016; Tassey, Citation2000). In the context of OSM, mappers needed to pay particular attention to the ordering of objects of relations after the intervention and develop new approaches to deal with it. This increased the development efforts and required adaptation, which is evident in our analysis as both the level of new data objects and their growth rate decreased (Model 2: β2 and β3). In that respect, the standardisation clearly decelerated the generation of content in absolute terms.
Simplification effect: increasing ease of new content generation
However, since the growth of new objects was not outright stopping, the picture painted by standardisation is more complex, and its effects on content quantity are not entirely negative. We summarise this as ‘simplification’ to describe the positive benefits resulting from standardisation on the generation of new content. For instance, the diffusion of innovation is often coupled with standardisation – in product or process (Blind & Gauch, Citation2009). New technologies are often so complex that a minimum skill level is necessary to interact with innovations effectively. At this stage, the use of innovations is limited and requires standardisation to make them amenable for mass adoption (Acemoglu et al., Citation2012). In the context of OSM, the diffusion of relation objects (for example, as a means to introduce public transport information to OSM) was difficult at first. Relations were so complex that only skilled mappers could store and edit such objects (and even they struggled and had to exert substantial effort). Standardising parameters and operations of the most complex types of relations plausibly increased the ease of generating these relation objects. In turn, this allowed more mappers to engage with it and create objects for envisioned public transportation use cases (Model 4: β2). In our findings, this coincided with the contraction in the number of all relation objects as per the control function of the standardisation described above.
Spill-over effect: qualitatively new content in new areas of the platform
A third effect we observed implies spill-overs in content generation, suggesting that the standardisation was also beneficial in another platform area. Here, our results show that the number of tags that can directly be tied to new use cases has increased on those relations that did not require ordering (Model 8: β2). This implies spill-overs to objects that are not in focus of standardisation from the objects that were directly affected by the standardisation. Spill-overs are part of the upsides heralded in research on standardisation (e.g., Zoo et al. (Citation2017), Lee (Citation2021)). In a best-case scenario, standardisation would level the playing field for users and complementors by enforcing a unified format for data objects to be used. The resulting increases in compatibility and interoperability might explain why the quality of output in terms of the level of detail increased on relations that were not initially affected by the standardisation (cf. Blind and Gauch (Citation2009)).
In summary, our findings suggest that standardising increased the innovative potential of OSM to generate new content even if the number of new objects initially decreased. This aligns our work with a burgeoning view on standardisation that asserts that tensions of innovation and control can be resolved through setting standards in complex technological systems (cf. Lindgren et al. (Citation2021)).
Discussion
In this paper, we study the effects of standardisation on platform innovation. Platform operators must control the generation of new content to steer the platform according to their strategy and prevent the network of participants from diminishing as a result of low-quality complements (e.g., Rietveld et al. (Citation2019), Hukal et al. (Citation2020)). This, however, is difficult: while some control is needed to stimulate innovation in the form of generating new content, too much, the wrong type or ill-informed control can stifle innovation and harm the platform in the long run (e.g., Ghazawneh and Henfridsson (Citation2013), Wareham et al. (Citation2014)). Against this backdrop, we studied standardisation as a form of direct control, which is critically discussed in the literature. We chose the popular geodata platform OpenStreetMap as an empirical context and assessed the quantity and quality of new content generated on the platform before and after a standardisation change of the platform´s editing API. We find that the standardisation had varying effects – both beneficial and detrimental – on platform innovation. As such, the findings of our study speak directly to the rich body of work on standardisation and innovation in technology and innovation management and its application as a form of control on digital platforms.
Implications for research
Our research holds several important implications for our understanding of control on digital platforms. A first implication is the recognition that standardisation can serve as an adaptable method for controlling the generation of platform content. Unlike other forms of direct control that often require precise knowledge of what should be controlled (cf. Eaton et al. (Citation2015)), standardisation operates by providing boundaries rather than rigid directives that still provide complementors freedom to work within. Thus, while restricting variability in one dimension, it allows new variation to be introduced in another to address the tension between control and innovation (Eaton et al., Citation2015; Gawer & Henderson, Citation2007). This characteristic renders standardisation particularly well-suited for governing the generation of platform content, where the landscape is dynamic and rapidly evolving. Further, it addresses limitations associated with more traditional control mechanisms (cf. Ens et al. (Citation2023)), which often struggle in environments marked by uncertainty or change. Hence, our results suggest that standardisation offers a middle ground between the rigidity of formal control and the ambiguity of informal control.
A second implication is the more nuanced view of three different effects that standardisation exerts on platform innovation in quantity and quality. While we derive the explanations of the three effects from existing knowledge (i.e., weakened innovation through enforcement of uniformity of actions and outputs (e.g., David and Rothwell (Citation1996) or Miric et al. (Citation2023)) and increased innovation through formalisation improving task performance (e.g., Adler and Borys (Citation1996) or Hein et al. (Citation2019)), we offer novel insights through the distinction between innovation quantity and quality as well as the integrated view of the effects. Therefore, the effects contribute to a richer understanding of the dynamics involved in leveraging standardisation as a form of control. Recognising these effects holistically is crucial, as they describe positive and negative outcomes, presenting a multifaceted landscape for platform operators to navigate. Understanding their interplay is necessary for better governance of ever-evolving platforms as technological systems (e.g., Gawer (Citation2022)).
Last, our results align with prevailing views on the conflicting relationship between standardisation and innovation (e.g., Blind (Citation2016), Teece (Citation2018)) while also contributing nuanced insights into how this relationship plays out within digital platforms. Thereby, our study enriches the broader discourses in management literature to better understand their general connection (see the recent review by Blind et al. (Citation2023)). Further, we contribute to delineating how this connection unfolds on digital platforms. As the expansion of platform businesses evolves, incorporating these specifics becomes imperative to benefit from insights that acknowledge the unique opportunities and challenges presented by digital platforms.
Limitations and future research
Our work comes with certain limitations that help explicate the boundary conditions of our findings. Highlighting them, therefore, serves as inspiration for future work on platforms that differ, among others, by the type of content they provide, the focus on complementors or the platform business model, to name but three.
First, our study focuses on OpenStreetMap, a unique platform where users contribute geospatial data as new content. Geospatial data is a specific type of platform content (similar to, for instance, reviews and data on TripAdvisor) that helps build more complex information products on the platform. The identified effects of standardisation may exhibit variations when considering other types of platform content. For instance, on a video-sharing platform such as YouTube, the user group – like on OSM – are mainly private individuals. However, the content format produced is limited in variation relative to geospatial data objects. Here, standardisation of which content format is allowed on the platform and the consequences arising from it can conceivably take different forms that we have not observed. Hence, future research should study diverse platforms, exploring how standardisation influences content generation beyond geospatial data.
Second, while we capture different effects, there might be additional externalities associated with standardisation that our focus on OSM users did not capture. For instance, exploring standardisation´s impact on OSM complementors will likely reveal additional insight. Take the example of navigation systems: here, the standardisation of (relation objects describing) route descriptions may have served as a quality signal, which could have influenced the development and performance of third-party navigation applications. Hence, future research on standardisation promises to reveal additional effects by examining the consequences for complementary products developed on top of standardised content.
Lastly, our study provides insights into the effects of standardisation on innovation but does not explicitly differentiate between its impact on ‘radical’ and ‘incremental’ innovation. This is likely of interest for platform operators implementing multi-sided business models for profit. Future research could explore how standardisation influences the nature and pace of innovation on digital platforms as it intersects with performance outcomes that are of interest to commercial operations: Does standardisation promote incremental improvements within established norms and drive current business, or does it facilitate the emergence of radical innovation and create new business? Investigating these and other aspects would contribute to a better understanding of the role of standardisation in shaping the trajectory of platform innovation.
Implications for practice
Our findings have implications for platform operators seeking to govern the generation of platform content. They showcase the potential of standardisation as a control mechanism for platform operators. By providing guidelines for complementors on the generation of platform content, operators can strike a balance between guiding content generation in desired ways (e.g., Wareham et al. (Citation2014), Foerderer et al. (Citation2021)) while maintaining a dynamic environment. This allows for flexible governance, fostering innovation within defined boundaries without stifling innovation too much. Further, as our results indicate overall positive effects for OSM´s innovative capacity, platform operators in other contexts may consider leveraging these benefits, too. This may be especially valuable in contexts where standardisation of interfaces may not be as widespread, such as for B2B or industrial platforms (e.g., Pauli et al. (Citation2021)).
Conclusion
In summary, our research highlights the value of studying control on digital platforms through a lens of standardisation and innovation. In that light, the pattern found in our data speaks to platform researchers wishing to study questions of whether and how innovation can be controlled on digital platforms. The varying effects of standardisation we found align well with work in this space by suggesting that standardisation restricts variety in one area of the platform while allowing it to spawn in another. Past work has referred to this as the balancing act of managing ‘desirable and undesirable variation’ to create ‘constrained serendipity’ (Wareham et al., Citation2014). Our findings provide further evidence for this stream of work that control – exercised through variety reduction standards (Blind, Citation2016; Tassey, Citation2000) – does not run counter to innovation on digital platforms. Instead, our study indicates that standardisation can be a powerful lever for platform operators to set control points in their architecture (Tilson et al., Citation2010; Wareham et al., Citation2014) in a way that favours innovation.
Disclosure statement
No potential conflict of interest was reported by the author(s).
Notes
1. Excluding countries that were stored incomplete on the OSM database excerpt (such as Ukraine, Russia, Belarus) as well as the United Kingdom which experienced mass data imports from public institutions during the time covered in our data.
References
- Acemoglu, D., Gancia, G., & Zilibotti, F. (2012). Competing engines of growth: Innovation and standardization. Journal of Economic Theory, 147(2), 570–601. https://doi.org/10.1016/j.jet.2010.09.001
- Adam, M., Croitor, E., Werner, D., Benlian, A., & Wiener, M. (2022). Input control and its signalling effects for complementors’ intention to join digital platforms. Information Systems Journal, 33(3), 437–466. https://doi.org/10.1111/isj.12408
- Adler, P. S., & Borys, B. (1996). Two types of bureaucracy: Enabling and coercive. Administrative Science Quarterly, 41(1), 61. https://doi.org/10.2307/2393986
- Blind, K. (2016). Chapter 14: The impact of standardisation and standards on innovation. In J. Edler, P. Cunningham, A. Gök, & P. Shapira (Eds.), Handbook of innovation policy (pp. 423–449). Edward Elgar Publishing.
- Blind, K., & Gauch, S. (2009). Research and standardisation in nanotechnology: Evidence from Germany. The Journal of Technology Transfer, 34(3), 320–342. https://doi.org/10.1007/s10961-008-9089-8
- Blind, K., Kenney, M., Leiponen, A., & Simcoe, T. (2023). Standards and innovation: A review and introduction to the special issue. Research Policy, 52(8), 104830. https://doi.org/10.1016/j.respol.2023.104830
- Boudreau, K. J. (2010). Open platform strategies and innovation: Granting access vs. Devolving control. Management Science, 56(10), 1849–1872. https://doi.org/10.1287/mnsc.1100.1215
- Boudreau, K. J. (2012). Let a thousand flowers bloom? An early look at large numbers of software app developers and patterns of innovation. Organization Science, 23(5), 1409–1427. https://doi.org/10.1287/orsc.1110.0678
- Budhathoki, N. R., & Haythornthwaite, C. (2013). Motivation for open collaboration. American Behavioral Scientist, 57(5), 548–575. https://doi.org/10.1177/0002764212469364
- Cennamo, C., & Santaló, J. (2019). Generativity tension and value creation in platform ecosystems. Organization Science, 30(3), 617–641. https://doi.org/10.1287/orsc.2018.1270
- Claussen, J., Kretschmer, T., & Mayrhofer, P. (2013). The effects of rewarding user engagement: The case of Facebook Apps. Information Systems Research, 24(1), 186–200. https://doi.org/10.1287/isre.1120.0467
- Costabile, C., Iden, J., & Bygstad, B. (2022). Building digital platform ecosystems through standardization: An institutional work approach. Electronic Markets, 32(4), 1877–1889. https://doi.org/10.1007/s12525-022-00552-0
- Croitor, E., & Benlian, A. (2019). Perceived input control on online platforms from the application developer perspective: Conceptualisation and scale development. Journal of Decision Systems, 28(1), 19–40. https://doi.org/10.1080/12460125.2019.1616977
- David, P. (1995). Standardization policies for network technologies: The flux between freedom and order revisited. In R. Hawkins, R. Mansell, & J. Skea (Eds.), Standards, innovation and competitiveness: The politics and economics of standards in natural and technical environments (pp. 15–35). Edward Elgar Publishing.
- David, P., & Rothwell, G. S. (1996). Standardization, diversity and learning: Strategies for the coevolution of technology and industrial capacity. International Journal of Industrial Organization, 14(2), 181–201. https://doi.org/10.1016/0167-7187(95)00475-0
- Eaton, B., Elaluf-Calderwood, S., Sorensen, C., & Yoo, Y. (2015). Distributed tuning of boundary resources: The case of Apple’s iOS service system. MIS Quarterly, 39(1), 217–243. https://doi.org/10.25300/MISQ/2015/39.1.10
- Elaluf-Calderwood, S., Eaton, B. D., Sorensen, C., & Yoo, Y. (2011). Control as a strategy for the development of generativity in business models for mobile platforms. 15th International Conference on Intelligence in Next Generation Networks.
- Ens, N., Hukal, P., & Blegind Jensen, T. (2023). Dynamics of control on digital platforms. Information Systems Journal, 33(4), 890–911. https://doi.org/10.1111/isj.12429
- Faraj, S., Jarvenpaa, S. L., & Majchrzak, A. (2011). Knowledge collaboration in online communities. Organization Science, 22(5), 1224–1239. https://doi.org/10.1287/orsc.1100.0614
- Foerderer, J., Lueker, N., & Heinzl, A. (2021). And the winner is … ? The desirable and undesirable effects of platform awards. Information Systems Research, 32(4), 1155–1172. https://doi.org/10.1287/isre.2021.1019
- Fox, K. (2012). OpenStreetmap: “It’s the wikipedia of maps”: A map of the world anyone can edit. https://www.theguardian.com/theobserver/2012/feb/18/openstreetmap-world-map-radicals
- Gawer, A. (2022). Digital platforms and ecosystems: Remarks on the dominant organizational forms of the digital age. Innovation, 24(1), 110–124. https://doi.org/10.1080/14479338.2021.1965888
- Gawer, A., & Henderson, R. (2007). Platform owner entry and innovation in complementary markets: Evidence from Intel. Journal of Economics & Management Strategy, 16(1), 1–34. https://doi.org/10.1111/j.1530-9134.2007.00130.x
- Ghazawneh, A., & Henfridsson, O. (2013). Balancing platform control and external contribution in third-party development: The boundary resources model. Information Systems Journal, 23(2), 173–192. https://doi.org/10.1111/j.1365-2575.2012.00406.x
- Gottmann, J. M. (1981). Time-series analysis: A comprehensive introduction for social scientists. Cambridge University Press.
- Grøtnes, E. (2009). Standardization as open innovation: Two cases from the mobile industry. Information Technology & People, 22(4), 367–381. https://doi.org/10.1108/09593840911002469
- Hawkins, R., & Blind, K. (2017). Introduction: Unravelling the relationship between standards and innovation. In R. Hawkins & K. Blind (Eds.), Handbook of innovation and standards (pp. 1–18). Edward Elgar Publishing.
- Hein, A., Weking, J., Schreieck, M., Wiesche, M., Böhm, M., & Krcmar, H. (2019). Value co-creation practices in business-to-business platform ecosystems. Electronic Markets, 29(3), 503–518. https://doi.org/10.1007/s12525-019-00337-y
- Hukal, P., Henfridsson, O., Shaikh, M., & Parker, G. (2020). Platform signaling for generating platform content. MIS Quarterly, 44(3), 1177–1205. https://doi.org/10.25300/MISQ/2020/15190/
- Kim, J., Lee, S., Geum, Y., & Park, Y. (2012). Patterns of innovation in digital content services: The case of App Store applications. Innovation, 14(4), 540–556. https://doi.org/10.5172/impp.2012.14.4.540
- Kirsch, L. J., Sambamurthy, V., Ko, D.-G., & Purvis, R. L. (2002). Controlling information systems development projects: The view from the client. Management Science, 48(4), 484–498. https://doi.org/10.1287/mnsc.48.4.484.204
- Lee, P.-C. (2021). Investigating the knowledge spillover and externality of technology standards based on patent data. IEEE Transactions on Engineering Management, 68(4), 1027–1041. https://doi.org/10.1109/TEM.2019.2911636
- Linden, A. (2015). Conducting interrupted time-series analysis for single- and multiple-group comparisons. The Stata Journal, 15(2), 480–500. https://doi.org/10.1177/1536867X1501500208
- Linden, A. (2018). Using forecast modelling to evaluate treatment effects in single-group interrupted time series analysis. Journal of Evaluation in Clinical Practice, 24(4), 695–700. https://doi.org/10.1111/jep.12946
- Lindgren, R., Mathiassen, L., & Schultze, U. (2021). The dialectics of technology standardization. MIS Quarterly, 45(3), 1187–1212. https://doi.org/10.25300/MISQ/2021/12860
- Miric, M., Ozalp, H., & Yilmaz, E. D. (2023). Trade‐offs to using standardized tools: Innovation enablers or creativity constraints? Strategic Management Journal, 44(4), 909–942. https://doi.org/10.1002/smj.3457
- Nagaraj, A. (2021). Information seeding and knowledge production in online communities: Evidence from OpenStreetMap. Management Science, 67(8), 4908–4934. https://doi.org/10.1287/mnsc.2020.3764
- Nylund, P. A., & Brem, A. (2023). Standardization in innovation ecosystems: The promise and peril of dominant platforms. Technological Forecasting and Social Change, 194, 122714. https://doi.org/10.1016/j.techfore.2023.122714
- Ouchi, W. G. (1979). A conceptual framework for the design of organizational control mechanisms. Management Science, 25(9), 833–848. https://doi.org/10.1287/mnsc.25.9.833
- Parker, G., van Alstyne, M., & Jiang, X. (2017). Platform ecosystems: How developers invert the firm. MIS Quarterly, 41(1), 255–266. https://doi.org/10.25300/MISQ/2017/41.1.13
- Pauli, T., Fielt, E., & Matzner, M. (2021). Digital industrial platforms. Business & Information Systems Engineering, 63(2), 181–190. https://doi.org/10.1007/s12599-020-00681-w
- Ramm, F., Topf, J., & Chilton, S. (2010). OpenStreetmap: Using and enhancing the free map of the world. UIT Cambridge.
- Rietveld, J., Schilling, M. A., & Bellavitis, C. (2019). Platform strategy: Managing ecosystem value through selective promotion of complements. Organization Science, 30(6), 1232–1251. https://doi.org/10.1287/orsc.2019.1290
- Sadish, W. R., Cook, T. D., & Campbell, D. T. (2002). Experimental and quasi-experimental designs for generalized causal inference. Houghton Mifflin.
- Saunders, C., Benlian, A., Henfridsson, O., & Wiener, M. (2020, November 23). IS control & Governance. MIS Quarterly Research Curations. http://misq.org/research-curations
- Swann, P. (2000). The economics of standardisation: Final report for standards and technical regulations directorate: Department of trade and industry. University of Manchester.
- Swann, P. (2010). The economics of standardization: An update. https://assets.publishing.service.gov.uk/government/uploads/system/uploads/attachment_data/file/32444/10-1135-economics-of-standardization-update.pdf
- Tassey, G. (2000). Standardization in technology-based markets. Research Policy, 29(4–5), 587–602. https://doi.org/10.1016/S0048-7333(99)00091-8
- Teece, D. J. (2018). Profiting from innovation in the digital economy: Enabling technologies, standards, and licensing models in the wireless world. Research Policy, 47(8), 1367–1387. https://doi.org/10.1016/j.respol.2017.01.015
- Thompson, G. V. (1954). Intercompany technical standardization in the early American automobile industry. The Journal of Economic History, 14(1), 1–20. https://doi.org/10.1017/S0022050700063592
- Tilson, D., Lyytinen, K., & Sørensen, C. (2010). Research commentary —Digital infrastructures: The missing IS research agenda. Information Systems Research, 21(4), 748–759. https://doi.org/10.1287/isre.1100.0318
- Tiwana, A., Konsynski, B., & Bush, A. A. (2010). Research commentary —Platform evolution: Coevolution of platform architecture, governance, and environmental dynamics. Information Systems Research, 21(4), 675–687. https://doi.org/10.1287/isre.1100.0323
- Turner, S. L., Karahalios, A., Forbes, A. B., Taljaard, M., Grimshaw, J. M., & McKenzie, J. E. (2021). Comparison of six statistical methods for interrupted time series studies: Empirical evaluation of 190 published series. BMC Medical Research Methodology, 21(1), 134. https://doi.org/10.1186/s12874-021-01306-w
- van de Kaa, G., Viardot, E., & McCarthy, I. P. (2022). Standardization for platform ecosystems. Electronic Markets, 32(4), 1807–1811. https://doi.org/10.1007/s12525-022-00611-6
- Varian, H. R., Farrell, J., & Shapiro, C. (2005). The economics of information technology: An introduction. Cambridge University Press.
- Wareham, J., Fox, P. B., & Cano Giner, J. L. (2014). Technology ecosystem governance. Organization Science, 25(4), 1195–1215. https://doi.org/10.1287/orsc.2014.0895
- Wen, W., Forman, C., & Jarvenpaa, S. L. (2022). The effects of technology standards on complementor innovations: Evidence from the IETF. Research Policy, 51(6), 104518. https://doi.org/10.1016/j.respol.2022.104518
- Wessel, M., Thies, F., & Benlian, A. (2017). Opening the floodgates: The implications of increasing platform openness in crowdfunding. Journal of Information Technology, 32(4), 344–360. https://doi.org/10.1057/s41265-017-0040-z
- Wiegmann, P. M., Vries, H. J. D., & Blind, K. (2017). Multi-mode standardisation: A critical review and a research agenda. Research Policy, 46(8), 1370–1386. https://doi.org/10.1016/j.respol.2017.06.002
- Wright, C., Sturdy, A., & Wylie, N. (2012). Management innovation through standardization: Consultants as standardizers of organizational practice. Research Policy, 41(3), 652–662. https://doi.org/10.1016/j.respol.2011.12.004
- Ye, S., Gao, G., & Viswanathan, S. (2014). Strategic behavior in online reputation systems: Evidence from revoking on eBay. MIS Quarterly, 38(4), 1033–1056. https://doi.org/10.25300/MISQ/2014/38.4.05
- Yoo, Y., Henfridsson, O., & Lyytinen, K. (2010). Research commentary - The new organizing logic of digital innovation: An agenda for information systems research. Information Systems Research, 21(4), 724–735. https://doi.org/10.1287/isre.1100.0322
- Zoo, H., Vries, H. J. D., & Lee, H. (2017). Interplay of innovation and standardization: Exploring the relevance in developing countries. Technological Forecasting and Social Change, 118, 334–348. https://doi.org/10.1016/j.techfore.2017.02.033
Appendix A
Table A1. Exemplary quotes discussing the status quo and (then) current problems of unordered relation members.
Table A2. Exemplary quotes arguing against the standardisation effort.
Table A3. Exemplary quotes arguing in favour of the standardisation effort.