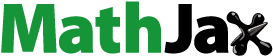
Abstract
In order to explain the negative slope of versus inhibitor concentration observed in the study of epigallocatechin gallate acting as an inhibitor of aldose reductase, a kinetic analysis was performed to rationalise the phenomenon. Classical and non-classical models of complete and incomplete enzyme inhibition were devised and analysed to obtain rate equations suitable for the interpretation of experimental data. The results obtained from the different approaches were discussed in terms of the meaning of the emerging kinetic constants. A decrease of
versus the inhibitor concentration was revealed to be a valuable indication of the occurrence of an incomplete inhibition. This indication, which is univocal in the case of an uncompetitive inhibition, may be especially useful when the residual activity resulting from inhibition is rather low.
Introduction
The apparent character of the parameters derived from the kinetic analysis of enzymatic reactions is an aspect of kinetic characterisation of enzymes rarely taken into adequate consideration in the interpretation of experimental data. The apparent character of the kinetic constants is intrinsically linked to the kinetic equation emerging from the analysis of the presumed mechanism satisfying the experimental results. Specifically, we refer to the inference on the calculated parameters of both the assumptions made in the model and the consequent experimental conditions adopted to fulfil the restrictions of the model itself. It is known for instance, that KM, an index of the affinity between the enzyme and the substrate, as usually determined, may be different from the thermodynamic equilibrium dissociation constant of the ES complex because of the clearly evident kinetic perturbation factor (i.e. k+2 or, in a more complicated model, the combination of a number of kinetic constants). The apparent character of KM may also arise from physical events, not detectable through the kinetic analysis, such as for instance the occurrence of a multistep interactive process between the substrate and the enzyme. Similar considerations apply also to the apparent character of kcat. In fact, the measured value of this parameter is linked to possible ES form(s) catalytically competent, rather than to the nominal total enzyme concentration. In addition, the measured kcat value is linked to possible reactions, even not productive, which may divert the ES complex(es) from product generation. To be clearer, the kcat, intended as the kinetic constant describing the transformation of the ES complex to products, is generally evaluated by dividing the measured Vmax value, in well-defined assay conditions, by the nominal concentration of the enzyme present in the assay. This calculation is correct only if the enzyme present in the assay is fully active and if the maximal ES concentration ([ES] at Vmax) equals the nominal concentration of the enzyme. This implies that only one ES form can be generated and that it is fully competent to generate products. If evidence of this is not given, as it occurs in the majority of kinetic characterisation of enzymes, we must be aware that the given value of kcat might not univocally represent the effectiveness of the evolution of the enzyme-substrate complex to products; in other words, the kcat is simply an apparent kinetic constant. Just as an example it is sufficient to consider the pH effect on KM and Vmax , in which kinetic constants are associated with specific ionic forms of the enzyme and enzyme-substrate complex. On these bases, the interpretation of the measured kinetic parameters becomes obviously even more difficult when inhibitors or activators are inserted into the reaction. Thus, the attempt of connecting the absolute value of the inhibition constants with an inhibition model of action and/or with the inhibitor features may lead to conclusions whose apparent solidity can be easily subverted.
The problem of possible misleading conclusions in terms of a real model of the inhibitory action arising from the interpretation of inhibition constants derived from a kinetic analysis has been faced by WalshCitation1. In that study, in which the question of binding versus efficacy of inhibitors is debated, the possible negative consequences of the use of inappropriate kinetic models in terms of efficient drug discovery processes have been highlighted. In any case, once conscious of the apparent character of the constants derived from kinetic measurements, the inhibition kinetic analysis remains an informative approach that is worth to be performed. However, in order to identify the most satisfactory model according to the physical interactive process, it may be advisable to substantiate the consistency of the kinetic measurements with non-kinetic analytic approaches.
The present study deals with aldose reductase (E.C. 1.1.1.21; AKR1B1), a NADPH-dependent reductase that, since its ability to transform glucose within the polyol pathway, is involved in the onset of a number of pathological states linked to hyperglycaemic conditionsCitation2. Thus, this enzyme is subjected to an intense investigation aiming to inhibit its activity. Since AKR1B1 is also able to reduce lipid peroxidation derived cytotoxic aldehydes, such as 4-hydroxy-2-nonenal (HNE), the inhibition of the enzyme may be causative of a lack or an impairment of its detoxification action. A new strategy (the “differential inhibition” approach) to inhibit the enzyme activity when acting on glucose reduction without affecting or with a limited effect on HNE reduction has been proposedCitation3. Generally talking, the term “differential inhibition” may apply to multispecific enzymes and refers to the inhibition of the enzymatic action on one or more specific substrates, while the transformation of other substrates remains unaffected or affected to a reduced extentCitation4.
In the present study, different models of enzyme inhibition were considered to explain the inhibition data observed for the reduction of two different substrates, i.e. L-idose and HNE, catalysed by AKR1B1 in the presence of epigallocatechin gallate (EGCG). This compound was recently shown to inhibit the reduction of the aforementioned substrates by two different mechanisms, namely a mixed inhibition for L-idose and an uncompetitive inhibition for HNECitation5. Here we report that the inhibitory action exerted by EGCG resulted to be incomplete towards HNE, but not towards L-idose. Possibly because not adequately furthered, incomplete inhibition reports are not so usual in inhibitory studiesCitation6. Nevertheless, as reported for a variety of enzymes, the phenomenon occurs and may be exerted either by metabolitesCitation7–9 or by abiotic moleculesCitation10–15.
A variety of graphical approaches have been proposed to disclose and characterise incomplete inhibitionCitation16–24. In this study, the rate equations derived from the classical approachCitation16,Citation17, considering the inhibitor targeting the free enzyme or the enzyme-substrate complex were used to fit experimental rate measurements through non-linear regression analysis. In this regard, the trend of the appKM/appkcat versus the inhibitor concentration is proposed as a useful tool to easily disclose the occurrence of an incomplete inhibition. Moreover, the analysis of both complete and incomplete inhibition was here performed also through a non-classical approach, in which it is the substrate that interacts with either the free enzyme or the enzyme-inhibitor complex.
Materials and methods
Materials
EGCG, NADPH and L-idose were obtained from Carbosynth (Compton, England). HNE and 3-glutathionyl-4-hydroxynonanal (GSHNE) were synthesised as describedCitation25. All other chemicals were of a reagent grade.
Assay and purification of human recombinant AKR1B1
The AKR1B1 activity was spectrophotometrically measured following the absorbance decrease at 340 nm as previously describedCitation26. AKR1B1 was expressed and purified to electrophoretic homogeneity, as previously describedCitation27. The purified enzyme preparation used in the study displayed a specific activity of 5.3 U/mg of protein. The conversion of rate measurements into appkcat was performed on the basis of an AKR1B1 molecular mass of 34 kDa.
Kinetic parameters analysis
The kinetic parameters appkcat and appKM were evaluated by non-linear regression analysis of rate measurements vs. substrate concentration according to the Michaelis-Menten equation. The analysis was performed through GraphPad Prism 7.04 software by a non-linear “Robust Regression” analysis in which each point is individually weighted through iterative weighing of the smallest squaresCitation28. The same approach was adopted to analyse the dependence of appkcat , appKM and appKM/appkcat from [I], making use of the proper equations (see text).
Results and discussion
The experimental ante fact
In a previous study on the inhibition ability of green tea components on AKR1B1 activityCitation5, EGCG resulted to display a differential inhibitory action on L-idose reduction with respect to HNE reduction. EGCG displayed an apparent uncompetitive inhibition on HNE reduction with a K’i (dissociation constant of the EIS ternary complex) of 116 ± 11 µM. Ki (dissociation constant of the EI complex), evaluated from the intercept with the abscissa of the secondary plot of appKM/appkcat versus [I] was considered as not detectable being the slope of appKM/appkcat vs [I] close to zero. Nevertheless, furthering on the EGCG inhibitory features, a refinement of the rate measurements disclosed a rather peculiar, previously unrevealed, behaviour of the appKM/appkcat versus [I] plot. As shown in , a marked unequivocal decrease of the appKM/appkcat values with the increase of the inhibitor concentration can be observed. This trend is difficult to be fitted into the so far adopted kinetic approach. These data, which in the figure are provisionally interpolated with a straight line, were indeed stimulating evidence to search for an adequate interpretative kinetic model.
Figure 1. The secondary plot of apparent kinetic parameters ratio appKM/appkcat versus [I] for the EGCG inhibition on HNE reduction. The dotted line refers to linear regression analysis fixing the intercept with the y-axis at the appKM/appkcat control value. Each value represents the appKM/appkcat ratio evaluated at the indicated EGCG concentrations. Data were obtained through non-linear regression analysis of vo vs [S] measurements using the Michaelis Menten equation. Bars (when not visible are within the symbols size) represent the standard error of the measurements.
![Figure 1. The secondary plot of apparent kinetic parameters ratio appKM/appkcat versus [I] for the EGCG inhibition on HNE reduction. The dotted line refers to linear regression analysis fixing the intercept with the y-axis at the appKM/appkcat control value. Each value represents the appKM/appkcat ratio evaluated at the indicated EGCG concentrations. Data were obtained through non-linear regression analysis of vo vs [S] measurements using the Michaelis Menten equation. Bars (when not visible are within the symbols size) represent the standard error of the measurements.](/cms/asset/3c8c5a25-12e2-42f5-adca-92925d03e624/ienz_a_2076089_f0001_b.jpg)
Kinetic models of enzyme inhibition: the classical approach
Without pretending to present any news on the steady state analysis of an enzymatic reaction, but only aiming to find a rationale for the observed data and to better define the question of the apparent character of the kinetic constants, let’s consider an inhibition process as described in . In the basic general model of inhibition of an enzymeCitation29 is shown. For the sake of simplicity, let’s avoid cooperative phenomena considering a simple (i.e. Michaelian) enzyme working either in a steady state or in equilibrium conditions. In the scheme, the possibility that the ternary complex may evolve into products (i.e. ), leading to an incomplete inhibition, is considered.
Figure 2. Inhibition models of an enzyme (E): a general model of inhibition (Panel A) and an alternative model of inhibition following “the classical approach” (Panel B). (S): substrate; (I): inhibitor; (ES): enzyme-substrate complex; (EI): enzyme-inhibitor complex; (EIS): enzyme-inhibitor-substrate ternary complex; k+1, k−1, k+2, k+3, k−3, k+4 refer to kinetic constants; Ki and K’i refer to EI and EIS dissociation constants, respectively. See text for details.
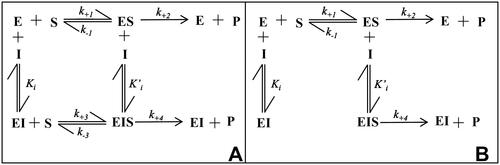
Taking advantage of the microscopic reversibility principle, it is common practice to represent the inhibitory action as in Panel B (the “classical approach”), in which the inhibitor is considered to target either the free enzyme or/and the ES complex. Then, the inhibitor will be defined, due to the relative values of apparent inhibition constants, as “competitive”, “mixed non-competitive” or “uncompetitive”Citation30.
Let’s consider for the moment the very frequent condition of a complete inhibition (i.e. k+4 = 0). In this case, appVmax ranges from k+2[ET] to zero with the increase of the inhibitor concentration, depending on the factor (1 + [I])−1; at the same time, appKM may either increase, when
or decrease, when
to a maximum of
or to a minimum of
respectively.
Now, looking at the relative changes between the appKM and appVmax, going from a mixed inhibition model to an uncompetitive model, the slope of appKM/appVmax = f ([I]), will decrease from positive values to zero. Such a limit, which refers to the uncompetitive model of inhibition, is the result of the fact that both KM and Vmax decrease of the same factor
It is evident that this approach fails in giving a rationale for the inhibition data reported above (), in which appKM/appVmax versus [I] decreases. appKM can either increase or decrease with, at maximum, the same steepness of appVmax. If we (at the moment) rule out that the inhibitor may induce an increase in the affinity of the enzyme for the substrate, the explanation of a negative slope of the appKM/appVmax = f ([I]) must be searched in the conversion of the enzyme-substrate complex to products. In other words, the decrease in appVmax by increasing [I] must be less steep than the appKM decrease. This situation may occur if the ternary complex is able to evolve to products, as it occurs for incomplete inhibitors. Here the decrease of appVmax from k+2 [maxES]= k+2 [ET]) in the absence of the inhibitor, will not tend to zero, with the increase of [I] but to appVmax =k+4 [maxESI]= k+4 [ET] (See .
For the sake of simplicity, let’s consider an incomplete uncompetitive inhibition (Ki at least 100 times higher than Ki’) in which appKM can only decrease when the inhibitor is present. In this case (see Appendix I), considering the assumption of conversion of EIS to product, the model must be analysed in steady state conditions in order to avoid losing the kinetic effect on the appKM changes. Then, the analytic approach in steady state conditions for both ES and EIS, will lead to the following rate equation:
(1)
(1)
The apparent kinetic constants
and appKM/appkcat are defined as follows:
(2)
(2)
(3)
(3)
(4)
(4)
In these equations Equation Equation (1)
(1)
(1) defines a hyperbola as a function of substrate concentration, as it occurs for k+4 = 0. The dependence of the kinetic parameters
and
(EquationEquations 2
(2)
(2) and Equation3
(3)
(3) ), upon the inhibitor, concentration is expected to have an exponential behaviour approaching an asymptote value >0 (. In this regard, it is worth noting that the inclusion of the
term (>0) in the
implies an effect of the incomplete inhibition not only, as expected, on appVmax, but also on
In fact, instead of tending to zero, when the inhibitor concentration increases,
will asymptotically tend to
Thus, it appears from this analysis that the decrease of both appVmax and appKM is buffered when EIS is able to evolve to products. Nevertheless, appKM will tend to a value (
) reasonably much lower than k+4, which is the limit value for appVmax, thus giving the rationale for the decrease of appKM/appkcat versus [I] (EquationEquation (4)
(4)
(4) , ).
Figure 3. Incomplete uncompetitive inhibition. (Panel A) represents the dependence of appkcat on the increase of [I] according to EquationEquation (2)(2)
(2) ; (Panel B) represents the dependence of appkM on the increase of [I] according to EquationEquation (3)
(3)
(3) ; (Panel C) represents the dependence of appKM/appkcat on the increase of [I] according to EquationEquation (4)
(4)
(4) .
![Figure 3. Incomplete uncompetitive inhibition. (Panel A) represents the dependence of appkcat on the increase of [I] according to EquationEquation (2)(2) kcatapp=Ki*k+2+k+4[I]Ki*+[I] (2) ; (Panel B) represents the dependence of appkM on the increase of [I] according to EquationEquation (3)(3) KMapp=Ki*KM+k+4k+1[I]Ki*+[I](3) ; (Panel C) represents the dependence of appKM/appkcat on the increase of [I] according to EquationEquation (4)(4) KMappkcatapp=Ki*KM+k+4k+1[I]Ki*k+2+k+4[I](4) .](/cms/asset/1d376f2d-6ad4-4116-aa3b-42c8f9683b28/ienz_a_2076089_f0003_c.jpg)
Independently on the inhibition model, the evaluation of the incomplete action of an inhibitor is not an easy task, especially when k+4 is rather low. Indeed, looking at the significant effect on the appKM/appkcat versus [I] exerted by relative small values of k+4 with respect to k+2 (), it appears that the experimental observation of a decrease of appKM/appkcat versus the inhibitor concentration becomes a valuable indication that the inhibition is not complete.
Figure 4. Predicted effect of the inhibitor concentration on the apparent kinetic parameters. The dependence of 1/appkcat (Panel A) and appKM/appkcat (Panel B) on the inhibitor concentration for different incomplete uncompetitive inhibition situations is reported, according to EquationEquations (2(2)
(2) and Equation4)
(4)
(4) , respectively. The following arbitrary values were fixed: k+2 = 1, k+1 = 100, KM = 0.5 and
= 0.001. Curves 1–6 refer to k+4 values of zero (complete inhibition), 0.02, 0.05, 0.1, 0.15 and 0.2, respectively.
![Figure 4. Predicted effect of the inhibitor concentration on the apparent kinetic parameters. The dependence of 1/appkcat (Panel A) and appKM/appkcat (Panel B) on the inhibitor concentration for different incomplete uncompetitive inhibition situations is reported, according to EquationEquations (2(2) kcatapp=Ki*k+2+k+4[I]Ki*+[I] (2) and Equation4)(4) KMappkcatapp=Ki*KM+k+4k+1[I]Ki*k+2+k+4[I](4) , respectively. The following arbitrary values were fixed: k+2 = 1, k+1 = 100, KM = 0.5 and Ki* = 0.001. Curves 1–6 refer to k+4 values of zero (complete inhibition), 0.02, 0.05, 0.1, 0.15 and 0.2, respectively.](/cms/asset/2df4caaf-3e91-4cc8-bc83-d8b847b37c05/ienz_a_2076089_f0004_c.jpg)
This approach will also apply to a mixed inhibition model in which the appKM changes may occur in both directions, depending on the relative apparent values of and
In this case, however, it is obvious that the incomplete inhibitory action of the inhibitor cannot be any more univocally associated with a decrease of the appKM/appkcat versus [I].
The rather complex kinetic equation (EquationEquation (5)(5)
(5) for a mixed inhibition model, derived from a steady state assumption for ES and EIS and an equilibrium assumption for EI (see Appendix II) still defines a hyperbola as a function of substrate concentration.
(5)
(5)
The apparent kinetic constants
and appKM/appkcat are defined as follows:
(6)
(6)
(7)
(7)
(8)
(8)
reports the predicted effect of inhibitor concentration on apparent kinetic parameters.
Figure 5. Predicted effect of the inhibitor concentration on the apparent kinetic parameters. The dependence of 1/appkcat (Panels A and D), appKM (Panels B and E) and appKM/appkcat (Panels C and F) on the inhibitor concentration for different incomplete mixed inhibition situations is reported, according to EquationEquations (6–8), respectively. The following arbitrary values were fixed: k+2 = 1, k+1 = 100, KM = 0.5. Curves 1–6 refer to k+4 values of zero (complete inhibition), 0.02, 0.05, 0.1, 0.15 and 0.2, respectively. (Panels A–C) refer to and Ki values of 0.001 and 0.01, respectively. (Panels D–F) refer to
and Ki values of 0.01 and 0.001 respectively. In (Panels B and E), curves 1–6 are essentially superimposable. Having imposed a reasonable rather low value of k+2/k+1 (0.01), k+4/k+1 will be negligible making the curves of appKM versus [I] at different k+4 are essentially superimposable.
![Figure 5. Predicted effect of the inhibitor concentration on the apparent kinetic parameters. The dependence of 1/appkcat (Panels A and D), appKM (Panels B and E) and appKM/appkcat (Panels C and F) on the inhibitor concentration for different incomplete mixed inhibition situations is reported, according to EquationEquations (6–8), respectively. The following arbitrary values were fixed: k+2 = 1, k+1 = 100, KM = 0.5. Curves 1–6 refer to k+4 values of zero (complete inhibition), 0.02, 0.05, 0.1, 0.15 and 0.2, respectively. (Panels A–C) refer to Ki* and Ki values of 0.001 and 0.01, respectively. (Panels D–F) refer to Ki* and Ki values of 0.01 and 0.001 respectively. In (Panels B and E), curves 1–6 are essentially superimposable. Having imposed a reasonable rather low value of k+2/k+1 (0.01), k+4/k+1 will be negligible making the curves of appKM versus [I] at different k+4 are essentially superimposable.](/cms/asset/55ba230d-8b8a-4509-8358-8295bdfe90ed/ienz_a_2076089_f0005_c.jpg)
It is clearly evident in the effect of the /
the ratio on the dependence of appKM and of appKM/appkcat on inhibitor concentration. Thus, while a decrease of appKM/appkcat versus [I] is a univocal indication of an incomplete inhibition phenomenon, the incomplete inhibition cannot be ruled out when an increase of appKM/appkcat versus [I] is observed.
To conclude, once verified the occurrence of an incomplete inhibition, the above classical approach may give the rationale even for the experimental observation of a decrease of appKM/appkcat versus [I] as reported for EGCG in . However, as shown, when the inhibition is not complete (i.e. 0<k+4<k+2), we must expect an exponential trend (either an increase or a decrease) of 1/appkcat, 1/appKM or appKM/appkcat as a function of [I]. Thus, the possible apparent linearity of experimental plots (i.e. 1/appkcat, 1/appKM or appKM/appkcat versus [I]) may be part of an overall exponential function. In any case, the analysis of experimental data must be performed through nonlinear regression.
Kinetic models of enzyme inhibition: the non-classical approach
As mentioned in the introduction, serious problems may be encountered in attempting to correlate the apparent inhibitory constants, with a physical interactive model. In fact, a different legitimate model of action, absolutely equivalent to the classic approach, is the one reported in , in which the equilibrium generating ESI is omitted, based on the microscopic reversibility principle.
Figure 6. Inhibition model of an enzyme (E) following “the non-classical approach”. (S): substrate; (I): inhibitor; (ES): enzyme-substrate complex; (EI): enzyme-inhibitor complex; (EIS): ternary enzyme-inhibitor-substrate complex; k+1, k−1, k+2, k+3, k−3, k+4 are kinetic constants; Ki is the EI complex dissociation constant. See text for details.
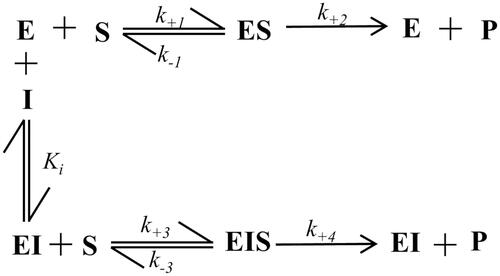
Here, the interaction of the inhibitor with the enzyme is described through a unique event, and it is the substrate that interacts either with the free enzyme or with the inhibitor-enzyme (EI) complex. It is evident that the interpretation of the same experimental data v0 = f ([S], [I]) will lead, now, to a completely different conclusion with respect to the classical approach.
The apparently simplest non-classical model is the case of a complete inhibition, which refers to the scheme of in which k+4 = 0. In this case (see Appendix III), from a steady state condition for ES and an equilibrium condition for both EI and EIS, the following kinetic equation will be obtained:
(9)
(9)
In EquationEquation (9)(9)
(9) ,
represents the dissociation constant for the ternary complex EIS,
The apparent kinetic constants are defined as follows:
(10)
(10)
(11)
(11)
(12)
(12)
The dependence of the kinetic parameters (EquationEquations 10–12), upon the inhibitor concentration, is reported in ; here is supposed that K’M>KM. It is evident that a different affinity of the substrate for E and EI will not affect the progressive decline of appkcat to zero, so that will increase with the increase of [I] ()).
Figure 7. Non-classical approach for complete inhibition. (Panel A) represents the dependence of appKM on the increase of [I] according to EquationEquation (10)(10)
(10) ; (Panel B) represents the dependence of 1/appkcat on the increase of [I] according to EquationEquation (11)
(11)
(11) ; Panel C represents the dependence of appKM/appkcat on the increase of [I] according to EquationEquation (12)
(12)
(12) .
![Figure 7. Non-classical approach for complete inhibition. (Panel A) represents the dependence of appKM on the increase of [I] according to EquationEquation (10)(10) KMapp=kM(1+[I]Ki)1+[I]kMKiKM′ (10) ; (Panel B) represents the dependence of 1/appkcat on the increase of [I] according to EquationEquation (11)(11) kcatapp=KiKM′k+2KiKM′+kM[I](11) ; Panel C represents the dependence of appKM/appkcat on the increase of [I] according to EquationEquation (12)(12) KMappkcatapp=KMk+2+KMKik+2[I] (12) .](/cms/asset/f8238889-4083-41e8-832d-1f0dbba978bc/ienz_a_2076089_f0007_c.jpg)
When an incomplete inhibition for the non-classical inhibition model is considered (i.e. 0<k+4 <k+2) (), the analysis performed considering the steady state for both branches of product formation and the inhibitor binding at equilibrium, leads to the following kinetic equation (see Appendix IV).
(13)
(13)
in which
and
The apparent kinetic parameters are defined as follows:
(14)
(14)
(15)
(15)
(16)
(16)
The dependence of the kinetic parameters (EquationEquations (14)–(16)), upon the inhibitor concentration, is reported in . Also in this case K’M>KM is assumed.
Figure 8. Non-classical approach for incomplete inhibition. (Panel A) represents the dependence of appKM on the increase of [I] according to EquationEquation (14)(14)
(14) ; (Panel B) represents the dependence of 1/appkcat on the increase of [I] according to EquationEquation (15)
(15)
(15) ; (Panel C) represents the dependence of appKM/appkcat on the increase of [I] according to EquationEquation (16)
(16)
(16) .
![Figure 8. Non-classical approach for incomplete inhibition. (Panel A) represents the dependence of appKM on the increase of [I] according to EquationEquation (14)(14) KMapp=KiKMKM′+KMKM′[I]KM′Ki+KM[I](14) ; (Panel B) represents the dependence of 1/appkcat on the increase of [I] according to EquationEquation (15)(15) kappcat= k+2KM′Ki+k+4KM[I]KM′Ki+ KM[I](15) ; (Panel C) represents the dependence of appKM/appkcat on the increase of [I] according to EquationEquation (16)(16) KMappkappcat=KiKMKM′+KMKM′[I]k+2KM′Ki+k+4KM[I](16) .](/cms/asset/efcd6220-73d8-40be-849b-905f816d7db4/ienz_a_2076089_f0008_c.jpg)
This approach predicts that the substrate binds to EI, leading to a ternary complex that is susceptible to transformation to products. Here, except for the parameters related to the not inhibited reaction (i.e. [I] = 0), and for the definition of the kinetic parameter k+4, which defines the ability of EIS to generate products, the meaning of axis intercepts and asymptotic values of the graphs is completely different from those emerging from the classical approach. It is worth noting that, for the non-classic kinetic model, the limit values of appKM (i.e. KM and K’M) represent intrinsic features of the substrate when interacting with two different enzyme forms (i.e. E and EI), whose relative abundance is the consequence only of the efficiency of the inhibitor in binding the free enzyme.
Having imposed k+4<k+2 and K’M>KM it will be difficult to envisage a decrease of appKM/appkcat versus [I]. However, a decrease may occur either when k+4/k+2 > K’M/KM or, more intriguingly, admitting a positive effect of the inhibitor on the binding of the substrate (i.e. K’M < KM).
Kinetic analysis of AKR1B1 inhibition by EGCG
The above considerations offer a rationale for a more adequate fitting of the results shown in , obtained when EGCG was tested as an inhibitor of the AKR1B1-dependent reduction of HNE. Here, the original experimental points are reported in . The resulting appKM and appkcat values, obtained at different EGCG concentrations, were analysed through nonlinear regression using EquationEquations (6(6)
(6) and Equation7)
(7)
(7) , respectively (). To evaluate the apparent dissociation constant of EIS (Ki*) and the kinetic constant of the ternary complex (k+4), a kcat value of 78 min−1 (78 ± 4 min−1) for HNE reduction in the absence of the inhibitor was inserted in EquationEquation (6)
(6)
(6) , as k+2. To determine the ES dissociation constant (Ki), whose value was imposed being >0, a value of 51 µM (51 ± 5 µM) for the KM for the substrate HNE in the absence of EGCG was used. Once verified the expected extremely low values of k+4/k‒1, EquationEquations (7
(7)
(7) and Equation8)
(8)
(8) could be simplified assuming this ratio equals to zero. Finally, since Ki value resulted far exceeding the Ki* value of 124 ± 19 µM (Ki/Ki*>100), the appKM/appkcat versus [I] data were interpolated by EquationEquation (4)
(4)
(4) as an uncompetitive inhibition. The insertion of the above Ki* value in EquationEquation (4)
(4)
(4) , in which no restrictions were imposed to k+4, gives rise to the curve fitting of the experimental data shown in , to be compared with that used in . The curve fitting allowed to obtain a k+4 value of 17 ± 2 min−1. In conclusion, these data, besides confirming the uncompetitive action of EGCG on the AKR1B1-dependent HNE reduction (Ki’ = 116 ± 11 μM)Citation5, disclose the occurrence of an incomplete inhibition. This phenomenon is characterised by a k+4 of 12 ± 2 min−1, as the average of values emerging from appKM/appkcat versus [I] (EquationEquation (4)
(4)
(4) ) and 1/appkcat versus [I] (EquationEquation (2)
(2)
(2) ) plots. Thus, the inhibition of EGCG leads to residual activity of approximately 15% of the reaction rate measured in the absence of the inhibitor.
Figure 9. Kinetic analysis by the classical approach of EGCG inhibition of the AKR1B1-dependent reduction of HNE. (Panel A) Hanes-Wolff representation of rate measurements of HNE reduction in the presence of the indicated concentrations of EGCG. Bars (when not visible are within the symbols size) represent the standard deviations of the means from at least three independent measurements. (Panels B–D) secondary plots of apparent kinetic parameters at different [I] derived from the non-linear regression Michaelis-Menten analysis of primary kinetic data. Bars (when not visible are within the symbols size) represent the standard error of the measurements. The parameters appKM, 1/appkcat and appKM/appkcat versus [I] were interpolated by non-linear regression analysis according to EquationEquations (4)(6)
(6) , Equation(6)
(7)
(7) and Equation(7)
(4)
(4) , respectively. See the text for details.
![Figure 9. Kinetic analysis by the classical approach of EGCG inhibition of the AKR1B1-dependent reduction of HNE. (Panel A) Hanes-Wolff representation of rate measurements of HNE reduction in the presence of the indicated concentrations of EGCG. Bars (when not visible are within the symbols size) represent the standard deviations of the means from at least three independent measurements. (Panels B–D) secondary plots of apparent kinetic parameters at different [I] derived from the non-linear regression Michaelis-Menten analysis of primary kinetic data. Bars (when not visible are within the symbols size) represent the standard error of the measurements. The parameters appKM, 1/appkcat and appKM/appkcat versus [I] were interpolated by non-linear regression analysis according to EquationEquations (4)(6) kcatapp=Ki*k+2+k+4[I]Ki*+[I] (6) , Equation(6)(7) KMapp=KMKi*+(Ki*KiKM+k+4k+1)[I]+k+4Kik+1[I]2Ki*+[I] (7) and Equation(7)(4) KMappkcatapp=Ki*KM+k+4k+1[I]Ki*k+2+k+4[I](4) , respectively. See the text for details.](/cms/asset/bbcccd58-c4c3-4f53-b7b9-fe5231aeecff/ienz_a_2076089_f0009_b.jpg)
This analysis was applied also to the inhibition by EGCG on L-idose reduction, considering a k+2 of 195 min−1 (195 ± 6 min−1) and a of 4.26 mM (4.26 ± 0.35 mM) measured in the absence of the inhibitor. In this case (), a classical behaviour as a mixed type of complete inhibition was verified, with a k+4 of 1.7 ± 0.5 min−1 (i.e. less than 1% of k+2) and Ki* and Ki values of 75 ± 2 µM and 330 ± 26 µM, respectively. These values were in line with previous characterisation (61 ± 9 µM and 425 ± 64 µM for Ki’ and Ki, respectively)Citation5.
Figure 10. Kinetic analysis by the classical approach of EGCG inhibition of the AKR1B1-dependent reduction of L-idose. (Panel A) Hanes-Wolff representation of rate measurements of L-idose reduction in the presence of the indicated concentrations of EGCG. Bars (when not visible are within the symbols size) represent the standard deviations of the means from at least three independent measurements. (Panels B–D) secondary plots of apparent kinetic parameters at different [I] coming from the non-linear regression Michaelis-Menten analysis of primary kinetic data. Bars (when not visible are within the symbols size) represent the standard error of the measurements. The parameters appKM, appkcat and appKM/appkcat versus [I] were interpolated by non-linear regression analysis through EquationEquations (4)(6)
(6) , Citation(6) and Citation(7), respectively.
![Figure 10. Kinetic analysis by the classical approach of EGCG inhibition of the AKR1B1-dependent reduction of L-idose. (Panel A) Hanes-Wolff representation of rate measurements of L-idose reduction in the presence of the indicated concentrations of EGCG. Bars (when not visible are within the symbols size) represent the standard deviations of the means from at least three independent measurements. (Panels B–D) secondary plots of apparent kinetic parameters at different [I] coming from the non-linear regression Michaelis-Menten analysis of primary kinetic data. Bars (when not visible are within the symbols size) represent the standard error of the measurements. The parameters appKM, appkcat and appKM/appkcat versus [I] were interpolated by non-linear regression analysis through EquationEquations (4)(6) kcatapp=Ki*k+2+k+4[I]Ki*+[I] (6) , Citation(6) and Citation(7), respectively.](/cms/asset/ff6748ce-e48a-4c9e-a72f-7024e53f35e3/ienz_a_2076089_f0010_b.jpg)
In light of the above mechanistic considerations, while the inhibition by EGCG on L-idose reduction is confirmed to fit a classical mixed type of complete inhibition, a rationale for the data observed for the same inhibitor on HNE reduction can be envisaged. In fact, the decrease of appKM/appkcat versus [I] appears as the result of the combined effect of an incomplete and uncompetitive inhibition. As predicted by the above kinetic models, in fact, both conditions are required to observe the phenomenon. It emerges from the study that a negative trend of appKM/appkcat with the increase of the inhibitor, concentration is an indication that an incomplete inhibition is occurring. This may be useful, especially when the residual activity upon inhibition is rather low. In these cases, the identification of the incompleteness of the inhibitory process may not result in an easy task due to the low reliability of rate measurements at high inhibitor concentrations.
Concerning the non-classical model analysis () of the above inhibitory data, rate measurements in the presence of HNE () or L-idose () at different inhibitor concentrations were analysed through regression analysis of EquationEquations (9)(9)
(9) and Equation(13)
(13)
(13) , respectively. Being the inhibition of L-idose reduction an apparently complete phenomenon, the emerging values of 1/appkcat, appKM and appKM/appkcat at different [I], were interpolated through EquationEquations (10)–(12), respectively. The best fitting of the data through the above equations ()) is compatible with an inhibition constant Ki of 385 ± 18 µM and a K’M of 831 ± 12 µM, accounting for an apparent increase of affinity of the substrate for the EI complex of approximately 5 folds.
Figure 11. Kinetic analysis by the non-classical approach of EGCG inhibition of the AKR1B1-dependent reduction of L-idose. The parameters 1/appkcat, appKM and appKM/appkcat obtained from data in , were reported as a function of [I] and interpolated by non-linear regression analysis through EquationEquations (10)–(12), respectively. Bars (when not visible are within the symbols size) represent the standard error of the measurements.
![Figure 11. Kinetic analysis by the non-classical approach of EGCG inhibition of the AKR1B1-dependent reduction of L-idose. The parameters 1/appkcat, appKM and appKM/appkcat obtained from data in Figure 10(A), were reported as a function of [I] and interpolated by non-linear regression analysis through EquationEquations (10)–(12), respectively. Bars (when not visible are within the symbols size) represent the standard error of the measurements.](/cms/asset/4def16d7-8c5a-4b4b-97de-3ed61f69659c/ienz_a_2076089_f0011_b.jpg)
In the case of HNE reduction, for which an incomplete inhibition apparently occurs, a k+4 value of 12 ± 4 min−1, as derived from the classic analysis, was used. Here, 1/appkcat, appKM and appKM/appkcat were derived from EquationEquation (13)(13)
(13) at different [I]. The secondary plots of the obtained data as a function of [I], ()) were interpolated through EquationEquations (14)–(16), respectively.
Figure 12. Kinetic analysis by the non-classical approach of EGCG inhibition of the AKR1B1-dependent reduction of HNE. The parameters 1/appkcat, appKM and appKM/appkcat obtained from data in , were reported as a function of [I] and interpolated by non-linear regression analysis through EquationEquations (14)–(16), respectively. Bars (when not visible are within the symbols size) represent the standard error of the measurements.
![Figure 12. Kinetic analysis by the non-classical approach of EGCG inhibition of the AKR1B1-dependent reduction of HNE. The parameters 1/appkcat, appKM and appKM/appkcat obtained from data in Figure 9(A), were reported as a function of [I] and interpolated by non-linear regression analysis through EquationEquations (14)–(16), respectively. Bars (when not visible are within the symbols size) represent the standard error of the measurements.](/cms/asset/b485db72-96f2-49f2-a751-cdef23a8b081/ienz_a_2076089_f0012_c.jpg)
In this case, an acceptable, even though not optimal, fitting of the data, including the decline of appKM/appkcat versus [I] was observed. However, the values of the kinetic parameters emerging from this interpolation were, unfortunately, corrupted by very high uncertainty determination (Ki =4,626 ± 25,000 and K’M = 0.9 ± 4) to be worthy of further consideration. It is hard to envisage whether such an unsatisfactory result derives from a different possible error propagation in the latter analysis or/and from the strong restrictions required by the model to be applicable. In fact, admitting valid the emerging high Ki value (which, incidentally, is in line with the uncompetitive action resulting from the classical analysis), a marked increase in the affinity of the HNE for the EI complex, with respect to the free enzyme, of approximately 50 folds would be required in order to fit the model. To support this interpretation and to confirm the model, an improvement of kinetic measurements and/or the availability of experimental data from a non-kinetic approach would be necessary.
The possibility to select between the two models, thus going inside the mechanism, necessarily requires the extension of the inhibition study to measurements not related to the steady state kinetic analysis. Thus, for instance, the evaluation of binding constants coming from fast kinetic approaches, spectroscopic and/or calorimetric measurements, or computational analysis might be considered.
In conclusion, the view of a differential inhibition as the result of a different targeting of an inhibitor depending on the substrate undergoing transformation can be changed, through the non-classic model approach, towards a kinetic equivalent view, which implies that different substrates differently target the EI complex. In any case, specifically referring to aldose reductase, the incomplete inhibition discovered for EGCG, which exclusively targets HNE reduction, discloses a new aspect to be furthered in searching for differential inhibitors, molecules that should preferentially inhibit the deleterious glucose reduction while preserving the reduction of toxic aldehydes.
Disclosure statement
The authors report there are no competing interests to declare.
Additional information
Funding
References
- Walsh R. Are improper kinetic models hampering drug development? Peer J 2014;2:e649.
- Thakur S, Gupta SK, Ali V, et al. Aldose reductase: a cause and a potential target for the treatment of diabetic complications. Arch Pharm Res 2021;44:1426–67.
- Del Corso A, Balestri F, Di Bugno E, et al. A new approach to control the enigmatic activity of aldose reductase. PLOS One 2013;8:e74076.
- Cappiello M, Balestri F, Moschini R, et al. Intra-site differential inhibition of multi-specific enzymes. J Enzyme Inhib Med Chem 2020;35:840–6.
- Balestri F, Poli G, Pineschi C, et al. Aldose reductase differential inhibitors in green tea. Biomolecules 2020;10:1003.
- Grant GA. The many faces of partial inhibition: revealing imposters with graphical analysis. Arch Biochem Biophys 2018;653:10–23.
- Gold MH, Farrand RJ, Livoni JP, Segel IH. Neurospora crassa glucogen phosphorylase: interconversion and kinetic properties of the “active” form. Arch Biochem Biophys 1974;161:515–27.
- Burger RM, Lowenstein JM. 5′-Nucleotidase from smooth muscle of small intestine and from brain. Inhibition of nucleotides. Biochemistry 1975;14:2362–6.
- Grant GA. Regulatory mechanism of Mycobacterium tuberculosis phosphoserine phosphatase SerB2. Biochemistry 2017;56:6481–90.
- Baici A, Salgam PK, Fehr K, Böni A. Inhibition of human elastase from polymorphonuclear leucocytes by a glycosaminoglycan polysulfate (Arteparon). Biochem. Pharmacol 1980;29:1723–7.
- Wangsgard WP, Meixell GE, Dasgupta M, Blumenthal DK. Activation and inhibition of phosphorylase kinase by monospecific antibodies raised against peptides from the regulatory domain of the gamma-subunit. J Biol Chem 1996;271:21126–33.
- Sharma RK, Wang JH, Wu Z. Mechanisms of inhibition of calmodulin-stimulated cyclic nucleotide phosphodiesterase by dihydropyridine calcium antagonists. J Neurochem 1997;69:845–50.
- González Tanarro CM, Gütschow M. Hyperbolic mixed-type inhibition of acetylcholinesterase by tetracyclic thienopyrimidines. J Enzyme Inhib Med Chem 2011;26:350–8.
- Nihei KI, Kubo I. Benzonitriles as tyrosinase inhibitors with hyperbolic inhibition manner. Int J Biol Macromol 2019;133:929–32.
- Zhang Q, Duan SX, Harmatz JS, et al. Mechanism of dasabuvir inhibition of acetaminophen glucuronidation. J Pharm Pharmacol 2022;74:131–8.
- Segel IH, Enzyme kinetics, behavior and analysis of rapid equilibrium and steady-state enzyme systems. New York: John Wiley and Sons; 1975.
- Cleland WW. Determining the chemical mechanisms of enzyme-catalyzed reactions by kinetic studies. Adv Enzymol Relat Areas Mol Biol 1977;45:273–387.
- Baici A. The specific velocity plot. A graphical method for determining inhibition parameters for both linear and hyperbolic enzyme inhibitors. Eur J Biochem 1981;119:9–14.
- Yoshino M. A graphical method for determining inhibition parameters for partial and complete inhibitors. Biochem J 1987;248:815–20.
- Palatini P. A simple method for distinguishing between full and partial inhibition. Biochem Int 1988;16:359–68.
- Fontes R, Ribeiro JM, Sillero A. A tridimensional representation of enzyme inhibition useful for diagnostic purposes. J Enzyme Inhib 1994;8:73–85.
- Whitely CG. Enzyme kinetics: partial and complete competitive inhibition. Biochem Educ 1997;25:144–6.
- Whitely CG. Enzyme kinetics: partial and complete non-competitive inhibition. Biochem Educ 1999;27:15–8.
- Whitely CG. Enzyme kinetics: partial and complete uncompetitive inhibition. Biochem Educ 2000;28:144–7.
- Balestri F, Barracco V, Renzone G, et al. Stereoselectivity of aldose reductase in the reduction of glutathionyl-hydroxynonanal adduct. Antioxidants 2019;8:502.
- Balestri F, De Leo M, Sorce C, et al. Soyasaponins from Zolfino bean as aldose reductase differential inhibitors. J Enzyme Inhib Med Chem 2019;34:350–60.
- Balestri F, Cappiello M, Moschini R, et al. L-Idose: an attractive substrate alternative to D-glucose for measuring aldose reductase activity. Biochem Biophys Res Commun 2015;456:891–5.
- Mavrevski R, Traykov M, Trenchev I, Trencheva M. Approaches to modeling of biological experimental data with GraphPad prism software. WSEAS Trans Syst Control 2018;13:242–7.
- Botts J, Morales M. Analytical description of the effects of modifiers and of enzyme multivalency upon the steady state catalyzed reaction rate. Trans Faraday Soc 1953;49:696–707.
- Dixon M, Webb EC, Thorne CJ, Tipton KF, Enzymes. Third Edition. London: Longman; 1979.
Appendix I
Incomplete uncompetitive inhibition
Referring to , the analysis is performed by assuming that
with
and
as the kinetic constants of EIS formation and dissociation to ES, respectively. The model is analysed by assuming both ES and EIS are in steady state conditions.
It follows a general kinetic equation as:
(A.1)
(A.1)
and an enzyme mass balance equation as:
(A.2)
(A.2)
The steady state conditions for both ES and EIS will be:
(A.3)
(A.3)
(A.4)
(A.4)
From (A.4):
(A.5)
(A.5)
in which
Replacing in (A.3) and resolving for
(A.6)
(A.6)
Replacing and
(A.5) and (A.6) into the mass balance EquationEquation (A.2)
(A.2)
(A.2) :
(A.7)
(A.7)
Replacing (A.5) in the rate EquationEquation (A.1)
(A.1)
(A.1) and then normalising for
(A.7)
(A.8)
(A.8)
Dividing numerator and denominator by
and dividing the denominator by
the final kinetic equation is obtained:
(A.9)
(A.9)
where
Appendix II
Incomplete mixed inhibition
Referring to , the analysis is performed by assuming EI at equilibrium
(A.10)
(A.10)
and both ES and EIS in steady state conditions. It follows a general kinetic equation as:
and an enzyme mass balance equation as:
The steady state conditions for both ES and EIS will be:
(A.11)
(A.11)
(A.12)
(A.12)
From A.12
(A.13)
(A.13)
Replacing in A.11 and resolving for
(A.14)
(A.14)
Being EI considered at equilibrium (A.10):
Thus, replacing from A.14 and then dividing numerator and denominator by
(A.15)
(A.15)
being
Replacing in A.15 and dividing numerator and denominator of the second term by we will have:
(A.16)
(A.16)
in which
Through simple algebra we will have as a function of
(A.17)
(A.17)
Replacing [EIS], [E] and [EI] from A.13, A.13, and A.17, respectively, into the mass balance equation:
Proceeding through simple algebra
(A.18)
(A.18)
Replacing [EIS] (A.13), into the general kinetic equation and normalising for the enzyme equation balance of A.18:
Through simple algebra:
Dividing numerator and denominator by
Dividing numerator and denominator by we obtained the kinetic equation, which is still a hyperbola with respect to substrate concentration.
(A.19)
(A.19)
Appendix III
Complete non-classical inhibition
Referring to , the analysis is performed by assuming that both EI and EIS at equilibrium and ES in steady state condition.
It follows a general kinetic equation as:
and an enzyme mass balance equation as:
From the steady state condition for ES:
and from the equilibrium conditions for EI and EIS
the concentrations of the different components can be expressed as a function of [ES]:
(A.20)
(A.20)
(A.21)
(A.21)
(A.22)
(A.22)
Replacing [E], [EI] and [EIS] from A.20, A.21, and A.22, respectively, into the mass balance equation:
(A.23)
(A.23)
in which
normalising the general kinetic equation for the enzyme equation balance of A.23:
proceeding through simple algebra we will reach:
Dividing numerator and denominator by we obtained the kinetic equation, which is still a hyperbola with respect to substrate concentration.
(A.24)
(A.24)
Appendix IV
Incomplete non classical inhibition
Referring to , the analysis is performed by assuming EI at equilibrium:
(A.25)
(A.25)
and both ES and EIS in steady state conditions.
It follows a general kinetic equation as:
and an enzyme mass balance equation as:
The steady state conditions for both ES and EIS will be:
(A.26)
(A.26)
(A.27)
(A.27)
From A.25 and A.26 it follows
(A.28)
(A.28)
(A.29)
(A.29)
In which:
From A.27 it follows:
(A.30)
(A.30)
From A.28, A.29 and A.30, the mass balance equation for the enzyme will be:
(A.31)
(A.31)
By replacing in the general kinetic equation with A.30, and normalising for A.31, it follows:
Simplifying and proceeding through simple algebra:
Multiplying numerator and denominator by [S] and dividing numerator and denominator by we obtained the kinetic equation for the incomplete non classical inhibition model in the usual hyperbolic form.
(A.32)
(A.32)