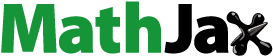
Abstract
Objective
To validate a serum biomarker developed in the USA for preterm birth (PTB) risk stratification in Viet Nam.
Methods
Women with singleton pregnancies (n = 5000) were recruited between 19+0-23+6 weeks’ gestation at Tu Du Hospital, Ho Chi Minh City. Maternal serum was collected from 19+0-22+6 weeks’ gestation and participants followed to neonatal discharge. Relative insulin-like growth factor binding protein 4 (IGFBP4) and sex hormone binding globulin (SHBG) abundances were measured by mass spectrometry and their ratio compared between PTB cases and term controls. Discrimination (area under the receiver operating characteristic curve, AUC) and calibration for PTB <37 and <34 weeks’ gestation were tested, with model tuning using clinical factors. Measured outcomes included all PTBs (any birth ≤37 weeks’ gestation) and spontaneous PTBs (birth ≤37 weeks’ gestation with clinical signs of initiation of parturition).
Results
Complete data were available for 4984 (99.7%) individuals. The cohort PTB rate was 6.7% (n = 335). We observed an inverse association between the IGFBP4/SHBG ratio and gestational age at birth (p = 0.017; AUC 0.60 [95% CI, 0.53-0.68]). Including previous PTB (for multiparous women) or prior miscarriage (for primiparous women) improved performance (AUC 0.65 and 0.70, respectively, for PTB <37 and <34 weeks’ gestation). Optimal performance (AUC 0.74) was seen within 19-20 weeks’ gestation, for BMI >21 kg/m2 and age 20-35 years.
Conclusion
We have validated a novel serum biomarker for PTB risk stratification in a very different setting to the original study. Further research is required to determine appropriate ratio thresholds based on the prevalence of risk factors and the availability of resources and preventative therapies.
Introduction
Preventing preterm birth (PTB, defined as delivery <37 weeks’ gestation) is a global priority. Of the estimated 15 million babies born preterm each year [Citation1,Citation2], approximately one million will die from complications, and many more will be left with lifelong disabilities [Citation3].
PTB prediction in otherwise low-risk women could improve neonatal outcomes by targeting evidence-based strategies such as dedicated PTB clinics, vaginal progesterone supplementation, cervical cerclage or pessary, nutritional supplementation, and/or improved parental education and birth preparedness [Citation4–7].
To date, preventative interventions for PTB have only been partially effective. A heterogenous syndrome, PTB involves both known and unknown pathways [Citation8,Citation9]. Better targeting of preventative strategies could mean fewer premature babies, with cost savings to society and the health system. Given the importance of environmental, nutritional, genetic, medical, and infectious exposures to PTB [Citation10], protein expression associated with PTB could vary by context. Exclusion of populations living in high-burden settings from PTB biomarker discovery and validation work reduces the global impact of screening and therapeutic insights. External validation should be performed, using data from patients who are at risk for the same disease/event, are not included in model development, and, ideally, are from a different population. This step should be performed before evaluating the biomarker’s clinical impact in the intended population [Citation11].
In a previous discovery and validation study [Citation12] in a United States (US) population, two placentally expressed proteins involved in uteroplacental insufficiency and inflammation were identified as biomarkers of spontaneous PTB (sPTB) when measured at 19+0-20+6 weeks’ gestation. The ratio of serum insulin-like growth factor-binding protein 4 (IGFBP4) to sex hormone-binding globulin (SHBG) was shown to be predictive of sPTB in asymptomatic women, with an area under the receiver operating characteristic curve (AUC) of 0.75 (95% confidence interval [CI], 0.56-0.91) and improved performance (e.g. AUC = 0.80) with more accurate gestational age (GA) dating [Citation13], when case/control assignments are more certain. Importantly, Markenson et al. (2020) [Citation14] demonstrated that IGFBP4/SHBG predicts not only sPTB, but also very early PTB (medically indicated PTB [miPTB] +sPTB) and more direct measures of adverse neonatal outcomes associated with prematurity. The predictor performed better in women with body mass index (BMI) between 22-37 kg/m2 and in identifying those at risk of birth at earlier GAs, when the clinical consequences of prematurity are greatest. Highest predictive performance was seen for deliveries at <32 weeks’ gestation, with deliveries including both sPTB and miPTB.
When adjusted for BMI and gestational age at blood draw (GABD), IGFBP4/SHBG significantly predicted sPTB in a subsequent study [Citation15] of pregnant women recruited from sites in Bangladesh, Pakistan, and Tanzania as part of the Alliance for Maternal and Newborn Health Improvement (AMANHI) biorepository study [Citation16]. However, AMANHI was not designed to evaluate all-cause PTB predictive performance or different sPTB etiologies, and subject numbers limited further stratification (e.g. geography-specific variation). Predictor performance outside a US population needs better characterization, as it is possible that pathways contributing to PTB vary globally.
Here, we aimed to explore the hypothesis that the IGFBP4/SHBG predictor would stratify PTB risk in a larger, non-US population by: (1) externally validating the IGFBP4/SHBG predictor for all-cause PTB, in addition to sPTB, in a Vietnamese population; (2) tuning the test using clinical factors available at screening; and (3) identifying sub-populations in whom the test’s discriminatory value is highest.
Methods
The Viet Nam Preterm Birth Biomarker study was a case-cohort study of PTB and term deliveries. The study was conducted with a prospective protocol and reported in line with TRIPOD (Transparent Reporting of a multivariable prediction model for Individual Prognosis Or Diagnosis) recommendations [Citation17].
Study population
Pregnant women presenting to the ultrasound department at Tu Du Hospital, Ho Chi Minh City, for their mid-pregnancy anomaly ultrasound scan from September 27, 2016-May 9, 2018 were invited to participate in the study. Tu Du Hospital is one of the largest maternity hospitals in Southeast Asia, conducting around 60000 deliveries per year. It serves as a local hospital for the inner city population, as well as a referral hospital for higher-risk cases from the greater city area and surrounding provinces.
Eligible participants were ≥18 years of age with a viable singleton pregnancy at 19+0-22+6 weeks’ gestation, with BMI <35 kg/m2. Individuals were ineligible if they planned to deliver at another hospital, had evidence of major fetal abnormality, had active bleeding at the time of enrollment, were taking heparin during the pregnancy, had received blood products during pregnancy, or were clinically jaundiced. Individuals taking progesterone in pregnancy were initially excluded. This exclusion resulted in lower recruitment and raised the possibility that the cohort would not be representative of the eventual target population. Thus, following a protocol amendment in October 2017, progesterone intake was no longer an exclusion. Specifically, progesterone use recorded in this study was for women who experienced threatened miscarriage or any bleeding in the first trimester. These women were prescribed 20 mg of micronized desogestrol orally, taken daily for 2-4 weeks before 16 weeks’ gestation.
Baseline measurements
Medical and obstetric histories obtained from participants included gravidity and parity, previous PTB, prior low birth weight baby (defined as birth weight <2500 g), previous miscarriages, and previous pregnancy terminations. Crown-rump length measurement from an ultrasound performed <14 weeks’ gestation was used to estimate GA at recruitment and delivery.
Outcome measurements
After birth, information was collected on pregnancy complications; initiation and mode of delivery; neonatal size and status (liveborn, stillborn); and neonatal complications in babies admitted to the neonatal intensive care unit (NICU). Primary outcomes included all-cause PTBs (all-PTB), defined as any birth ≤37 weeks’ gestation, and sPTBs, defined as birth ≤37 weeks’ gestation with clinical signs of initiation of parturition, as per Villar et al. [Citation18]. Two obstetricians (JEH and LPD), blinded to IGFBP4/SHBG predictor results, independently reviewed all PTB case notes and assigned sPTB and medically or obstetrically indicated PTB (miPTB) categorization. Birth weight percentiles and small for GA were defined using INTERGROWTH-21st standards for GA and sex [Citation19]. Stillbirth was defined as any baby born with no signs of life after 20 weeks’ gestation. Neonatal deaths were captured up until the time of hospital discharge only.
Case cohort selection
Within the full cohort of 5000 participants, all-PTB was compared to 500 participants selected randomly (case cohort design) (Supplemental Figure 1). Twenty random samples of 500 participants (which also included those delivering preterm) were drawn from the full cohort using adaptive minimization. Subjects most closely matching the study population prevalence on key variables (BMI, maternal age, GABD, and GA at birth) were selected. Laboratory testing was performed on this cohort of 500 individuals and all remaining PTB cases from the full cohort (n = 297) (Supplemental Figure 1). The adaptive minimization process was then repeated to partition PTB cases and controls into three equally sized sub-cohorts (A, B, and C), which were matched across the same key exposure and outcome variables (Supplemental Table 1). For prediction of all-cause PTB, controls were defined as term births, and PTBs from the 500-subject cohort were grouped with cases. For sPTB prediction, controls were defined as both term births and the miPTBs selected as a part of the cohort, and cases excluded miPTBs.
IGFBP4/SHBG predictor performance was first tested in sub-cohort A. Sub-cohorts A and B were then combined to identify clinical factors that complemented the IGFBP4/SHBG predictor using the method described below. The modified predictor was trained on sub-cohorts A and B, and performance was validated on the held-out sub-cohort C. The three sub-cohorts were then combined to demonstrate performance of the final tuned models of the IGFBP4/SHBG predictor + clinical variables and to identify subpopulations with maximal performance.
Sample collection and processing
Venous blood samples were collected in serum separator tubes. Following clot formation at room temperature and centrifugation at 3000 rpm, serum was aliquoted within 2 h of collection for −80 °C storage at the Oxford University Clinical Research Unit (Ho Chi Minh City, Viet Nam). At the end of the study, samples were shipped to Sera Prognostics in two batches in liquid nitrogen shipping cannisters at −150 °C. In a US Clinical Laboratory Improvement Amendments- and College of American Pathologists-certified laboratory, samples were analyzed by liquid chromatography-multiple reaction monitoring mass spectrometry following a previously described procedure [Citation12,Citation21]. Individual samples were randomized to assay batches that also contained replicates of two pooled quality control sera samples: QC1, from non-pregnant women aged 21-33; and QC2, from pregnant women at 17-27 weeks’ gestation (Golden West Biologicals, Temecula, CA, USA). All batches were required to meet Westgard criteria for acceptance based on QC samples [Citation21,Citation22].
Clinical factor selection and development of ObRisk variable
We performed a statistical search for clinical factors predictive of PTB using the Least Absolute Shrinkage and Selection Operator (LASSO) method [Citation23]. Two hundred bootstrapped samples from sub-cohorts A and B with replacement were created, and the models were cross validated on leftover samples in each bootstrap iteration. Clinical factors selected via LASSO were recorded for each iteration. This process was repeated for nulliparous and multiparous subgroups. We created a new variable, ObRisk, with a score of 1 for multiparous women with previous PTB, and 1 for nulliparous women with prior miscarriage. Women not meeting either criterion were assigned a score of 0.
Predictor models
To calculate the predictor score, relative IGFBP4 and SHBG levels were expressed as response ratios (RR) of the peak area for the endogenous peptide divided by the peak area of its corresponding SIS peptide [Citation12,Citation21]. The base IGFBP4/SHBG predictor score was calculated as the natural logarithm of (RRIGFBP4/RRSHBG).
Sample size estimation
Sample size was estimated using NCSS PASS statistical software [Citation24]. Prior to the study, we assumed an sPTB rate of 2% based on historical data, with a total PTB rate of 10% due to provider-initiated deliveries from other causes. The recruitment target of 5000 women was selected to provide at least 100 sPTB cases and 400 PTB cases from other causes. This allowed testing of the null hypothesis: AUC = 0.5 with 90% power, assuming a 2:1 control-to-case allocation ratio.
Missing data
Owing to low (<5%) missing data across all clinical variables and outcomes, no imputation was performed, and a complete-case analysis is presented.
Blinding
Sera Prognostics personnel were blinded to clinical endpoints. Analyses were performed by an independent, third-party statistician, who initially received only mass spectrometry data and outcomes for sub-cohort A. Following verification, testing of ranked hypotheses, classifier tuning, and generation of new ranked hypotheses, this statistician was unblinded to the validation cohort.
Statistical analysis
All statistical tests were two-tailed and performed at the 5% significance level unless stated otherwise. Analyses were performed using SAS Version 9.3 (or higher) [Citation25] or R Version 3.1.3 (or higher) [Citation26]. Demographic and baseline variables were compared between cohort participants using chi-squared tests for categorical variables and Kruskal-Wallace tests for continuous variables [Citation27].
We assessed models to predict sPTB or all-PTB, <37 or <34 weeks’ gestation. Discriminative performance of the model was assessed using the C-statistic and presented as the AUC. AUC was assessed directionally, with cases hypothesized to have higher predictor scores than non-cases. Significance was tested via the one-tailed Wilcoxon-Mann-Whitney test, and 95% CIs were generated using DeLong’s method [Citation28]. Sensitivity, specificity, and positive and negative likelihood ratios (LR±) were calculated at thresholds corresponding to screen-positive rates (SPR) of 10%, 20% and 30%. Accurate reporting of a test’s clinical predictive performance requires demonstration of discrimination and calibration, and avoidance of pitfalls such as selective subject exclusion by GA [Citation29]. Calibration, defined as the agreement between the observed and the predicted outcomes, was assessed [Citation30,Citation31]; a slope of 1.0 represented a perfectly calibrated test.
Subgroup analysis and differences between development and validation populations
The IGFBP4/SHBG predictor was identified and validated originally in a US population. A key difference in the present study is a shift in the gestational blood draw window to align it with the Viet Nam mid-trimester ultrasound scan. Optimal performance of the US test was originally established as 19+0-20+6 weeks [Citation12]. In Viet Nam, the mid-trimester ultrasound scan is between 21+0-22+6 weeks. Additionally, prior data indicated that IGFBP4/SHBG predictive performance has optimal BMI and GA ranges [Citation12]. Performance was assessed by AUC for IGFBP4/SHBG + ObRisk for sliding windows across ranges of BMI, maternal age, and GABD. Calculation of prevalence-dependent performance metrics used the PTB prevalence in the analyzed study population.
Results
Cohort characteristics
Of 10711 women approached, 5000 were eligible and consented to participate. Reasons for nonparticipation are shown in . Complete end-point data were available for 4984 (99.7%) participants (). Median age was 28 years, and median GA at recruitment and blood draw was 21+2 weeks. Just over half (2666/4984) of participants were in their first pregnancy; amongst multiparous women, 11.6% (268/2318) reported a previous PTB. Median BMI was 22.5 kg/m2, and median time in formal education was 13 years.
Figure 1. Study overview.
SGABD, gestational age at blood draw; miPTB, medically indicated preterm birth; PTB, preterm birth; sPTB, spontaneous preterm birth.
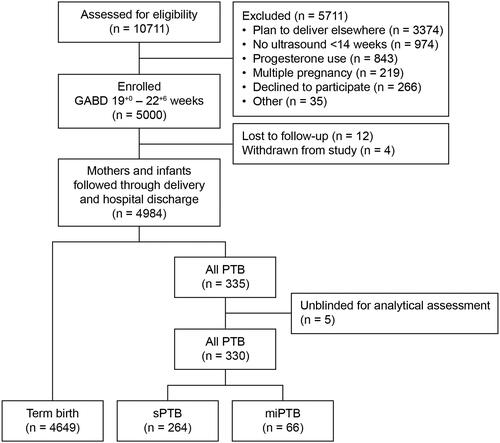
Table 1. Maternal characteristics and pregnancy outcomes in the Viet Nam study cohort.
There were 4968 live births and 16 stillbirths. Thirteen babies died after birth and before discharge. Within the cohort, 335 participants delivered preterm (PTB rate 6.7%): 66 were miPTB, and 269 were sPTBs. Five sPTBs delivered outside the hospital and were removed from the primary analysis. Nearly one-third (30.5%; 1522/4984) of participants and 44.5% (149/335) of those delivering preterm reported taking progesterone prior to 16 weeks during the index pregnancy.
Preterm birth prediction based on IGFBP4/SHBG
We observed an inverse association between IGFBP4/SHBG and GA at birth (p = 0.017). The predictor significantly differentiated participants who delivered sPTBs from the background cohort population; however, overall discrimination was modest (AUC 0.60; 95% CI, 0.53-0.68), with similar performance in predicting any PTB (0.60; 0.53-0.67) (). Performance was better for predicting PTB at lower GA thresholds: for example, for birth <34 weeks’ gestation, AUCs for sPTB and all-cause PTB were 0.67 (0.53-0.81) and 0.68 (0.56-0.79), respectively ().
Table 2. Performance of the IGFBP4/SHBG predictor alone and when applying the clinical ObRisk factor, evaluated for preterm births at <37 and <34 weeks’ gestation.
IGFBP4/SHBG predictor tuning
We explored the additive value of clinical factors predictive of PTB. Bootstrapped sampling using LASSO regression repeatedly identified the number of prior miscarriages for nulliparas as a strong clinical factor (Supplemental Figure 2 A), whereas for multiparas, the number of previous PTBs was selected repeatedly (Supplemental Figure 2B). The ObRisk variable improved predictive performance of IGFBP4/SHBG for PTB ().
The fully specified predictor developed for this population and its associated score is defined as:.
Validation on the independent sub-cohort C showed that prediction of all-PTB <37 and <34 weeks’ gestation produced AUCs of 0.62 (0.55-0.69) and 0.67 (0.55-0.79), respectively.
Clinical predictive performance
Both IGFBP4/SHBG alone and IGFBP4/SHBG + ObRisk demonstrated good calibration, with predicted probabilities falling on the diagonal (). Adding ObRisk led to tighter CIs and improved calibration at extremes of risk (). Women were assigned as being high- or low-risk according to a predictor score threshold corresponding to an SPR of 20%. Kaplan-Meier plots of GA at birth demonstrate that women identified as high-risk delivered earlier than those at low-risk, using both the base IGFBP4/SHBG (p = 0.031) and IGFBP4/SHBG + ObRisk (p < 0.001) predictors (). Diagnostic accuracy for risk of all-PTB <37 or <34 weeks’ gestation at different thresholds is summarized for combined Cohorts A, B and C in . We assessed threshold performance at three cutoff points defined by calling either 10%, 20%, or 30% of the population positive for risk of all-PTB. Specificities ranged from 70-90% at sensitivities of 25-64%. PPV and NPV ranged from 0.4-16% and 94-99%, respectively, consistent with the low prevalence of these outcomes. LRs ranged as high as 3.0 and as low as 0.5, and ORs reached 4.3. Predictive performance for sPTB and miPTB risk <37 weeks’ gestation in combined cohorts A, B, and C was similar to that for all-PTB (Supplemental Table 2).
Figure 2. Calibration plots for (a) IGFBP4/SHBG and (b) IGFBP4/SHBG + ObRisk.
CI, confidence interval; IGFBP4, insulin-like growth factor-binding protein 4; ObRisk, a dichotomous clinical factor based on prior miscarriage (nulliparas) or prior preterm birth (multiparas); SHBG, sex hormone-binding globulin.
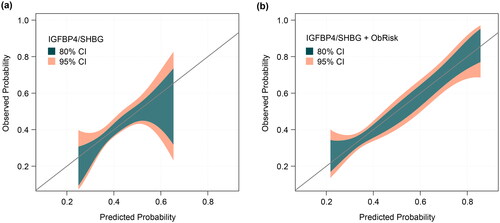
Figure 3. Kaplan-Meier plots of gestational age at birth for subjects evaluated to be at lower or higher PTB risk, stratified by (a) IGFBP4/SHBG ratio and (b) IGFBP4/SHBG ratio + ObRisk, at a screen-positive rate of 20%.
IGFBP4, insulin-like growth factor-binding protein 4; ObRisk, clinical factor based on prior miscarriage (nulliparas) or prior preterm birth (multiparas); SHBG, sex hormone binding globulin.
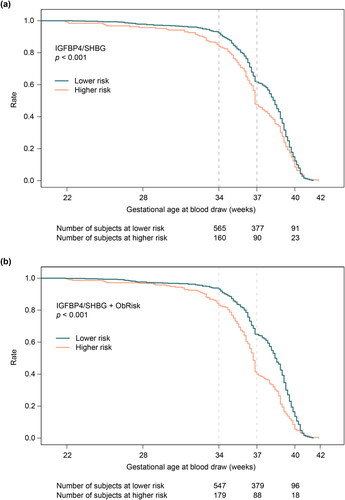
Table 3. IGFBP4/SHBG + ObRisk predictor performance for all-cause preterm birth under selected testing scenarios in combined cohorts A, B, and C.
In addition to prior PTB and miscarriage, the clinical variable search identified associations of BMI and maternal age with PTB (Supplemental Figure 2). IGFBP4/SHBG and IGFBP4/SHBG + ObRisk predictive performance improved as BMI and maternal age increased and when blood was drawn between 19+0-20+6 weeks’ gestation, as originally described in the US validation study. Based on this, we assessed discrimination in a subgroup analysis of participants meeting certain stratifications of these characteristics (Supplemental Table 3). The model performed superiorly for women with BMI >21 kg/m2, age 20-35 years, and GABD 133-146 days, with AUC 0.74 for all-PTB <37 and <34 weeks’ gestation. LRs ranged as high as 5.5 and low as 0.5, respectively, with ORs reaching 11 for prediction of PTB <34 weeks’ gestation.
Discussion
We present an updated PTB prediction model using the ratio of serum IGFBP4/SHBG, measured around 20 weeks’ gestation in asymptomatic women in Viet Nam. Adding ObRisk, a simple clinical variable incorporating prior PTB in multiparous women or history of miscarriage in those in their first ongoing pregnancy, improved model performance. Importantly, the strongest predictive performance was seen in those delivering <34 weeks’ gestation, when prematurity complications are most severe. Predictor performance was greatest in women with BMI >21 kg/m2, aged 20-35 years, with GABD 19+0-20+6 weeks’ gestation. Performance in this subgroup was moderate, similar to that observed in the US population [Citation12]. This is likely an underestimation of actual predictive performance, as it does not account for the impact of GA imprecision on the assignment of “true” and “false” cases and controls. For example, we have demonstrated previously that use of only those subjects dated by early ultrasound (e.g. prior to 9+0 weeks) improves predictor performance [Citation13]. Our study reaffirms the need for external validation, model tuning and testing of predictive models before implementation in a new population/geography – a step frequently missing from predictive models developed for pregnancy care [Citation32].
The IGFBP4/SHBG predictor appeared to perform better in Viet Nam than in the AMANHI cohort [Citation15]. A major difference in study design may largely explain this difference: Blood samples from the AMANHI cohort were drawn between 17+0-20+6 (median 18+2) weeks’ gestation, i.e. outside the optimal window of 19+0-20+6 weeks. GABD was, therefore, included in the linear regression, although modeling cannot fully compensate for this difference. AMANHI participants also had a lower median BMI (21.8 kg/m2) than both the US and Viet Nam populations. Adding BMI to the model improved predictive performance in AMANHI participants, but more than half of the cohort had a BMI below the optimum defined for the US population (>22 kg/m2). Lastly, cohort stratification was not performed due to limited sample numbers, and incorporation of clinical variables was not tested.
Notably, despite the population-level differences between Viet Nam and the USA, including the lower rate of PTB in Viet Nam (6.5% vs. 10%), a significant IGFBP4/SHBG signal persists, suggesting a common underlying biological pathway for certain PTB cases. IGFBP4 regulates insulin-like growth factors involved in sustaining adequate nutrient delivery to the fetus [Citation33]. It is expressed by the placenta [Citation34,Citation35] and has been reported to be upregulated (increased in circulation) in pregnancies with growth-restricted fetuses [Citation34,Citation35], upregulated in the placentas of small-for-gestational-age babies [Citation36], and downregulated in the placentas of large-for-gestational-age babies [Citation36]. These observations may indicate that IGFBP4 is a biomarker of uteroplacental insufficiency. SHBG regulates levels of free/biologically active sex steroids [Citation37], is expressed by the placenta [Citation38], and is reported to be suppressed by proinflammatory cytokines such as tumor necrosis factor alpha and interleukin 1 beta [Citation39]. Biomarkers for both placental insufficiency and inflammation may combine for clinically meaningful sPTB risk prediction.
Strengths and limitations
Key strengths of this study are the rigorous clinical and biological data collection, few losses to follow-up, and standardized definitions of sPTB and miPTB based on signs of initiation of parturition rather than eventual mode of birth. The random and representative nature of the case-cohort design enables its application to future outcome studies [Citation20]. The study was rigorously conducted with regular external quality monitoring. GA was established in all participants using ultrasound before 14 weeks’ gestation, minimizing the risk of misclassifying PTB and term births. Blood draw timing, 19+0-22+6 weeks’ gestation, was matched to the routine mid-pregnancy anomaly scan in Viet Nam, demonstrating how the test might perform if incorporated into routine practice. All laboratory analyses were conducted blinded to the clinical outcome, and in developing the ObRisk predictor, care was taken to select variables that could be easily collected at the time of blood draw.
Limitations of the study include the lower-than-anticipated PTB rate in the study population (6.5% vs 10.0%); however, this rate is in keeping with international estimates for Viet Nam (6.5%) published after our study concluded [Citation2]. It is noteworthy that women with multiple gestations were excluded from this study. Given the limited study scope and budget, we were unable to validate the predictor for multifetal pregnancies in this study, but this aspect should be examined. A motivation for this study was to validate the test in a high-burden setting, which was not reflected in the lower PTB prevalence observed. Nevertheless, the consequences for babies born preterm remain concerning, and PTB prevention is a national priority: UNICEF estimates that 45% of newborn deaths in Viet Nam are related to complications secondary to PTB [Citation40]. Our study was a hospital-based sample, and thus performance could differ at population level. Processing of all samples was performed in the US in a private laboratory, which would not be feasible or affordable should the predictor be used commercially in Viet Nam. We are developing a low-cost enzyme linked immunosorbent assay (ELISA) that, after validation, might be used in other settings. Finally, demonstration of clinical utility of the IBP4/SHBG biomarker in Viet Nam should precede its broad clinical use.
Conclusion
Despite an ever-increasing number of prediction models for pregnancy outcomes, very few are externally validated. Consequently, most published models are never used in clinical practice [Citation41]. Arguably, it is as important to understand the implications of implementing of a positive (or negative) test [Citation42], particularly when considering use in resource-limited settings. For Viet Nam, any health and financial benefits of prediction will need to outweigh the costs of the test and resources needed for treating those identified at high-risk – including those who go on to deliver at term (false positives). IGFBP4/SHBG could be useful in stratifying women to target specialized clinical care. Such benefits and costs, including the efficacy of lower-cost approaches, should be the focus of ongoing research.
Ethics approval
The study was approved by the University of Oxford Tropical Research Ethics Committee (OXTREC reference number 28-16) and the Tu Du Hospital ethics committee. Written informed consent was obtained from all participants.
Contribution to authorship
Study conceptualization was by JEH, JJB, DEH, SK, LQT. Data curation was performed by LPD, VKTT, TTD, BTHN, EK. Formal analysis was performed by JJB, AP, TCF. Funding acquisition was attained by SK. Investigations were performed by JEH JJB, LPD, TCF. Methodology was developed by JEH, JJB, LPD, AP, VKTT, TTD, SK, LQT. Project administration was performed by LPD, VKTT, TTD, BTHN, GT, EK. Project supervision was the responsibility of GT, EK, LQT and JEH. Validation of data was performed by JJB and AP. AP also performed the visualizations of data. Writing of the original draft was by JEH, JJB, AP, ACF, TCF. Writing - reviewing and editing of subsequent drafts was by all authors.
Supplemental Material
Download MS Word (302.2 KB)Acknowledgments
We wish to acknowledge the study participants, the research site personnel at Tu Du Hospital and OUCRU Clinical Trials Unit, and the Sera Prognostics, Inc. clinical laboratory and clinical operations teams. Babak Shahbaba, PhD, Mike Walker, PhD, and Jing Shi, PhD provided statistical analysis support. Jennifer Logan, PhD, an employee of Sera Prognostics, Inc., contributed to the writing of this article.
Disclosure statement
JJB, ADP, ACF, TCF, DEH, and PEK were employees, consultants and stockholders of Sera Prognostics, Inc., at the time of this work. JJB, ADP, TCF, and PEK are named on issued and pending patents on potential protein biomarkers of preterm birth. All other authors report no conflicts relevant to this work.
Data sharing
Data supporting the findings of this study are present in the paper and/or the Supplemental Materials. Additional data related to this paper may be requested from the authors.
Correction Statement
This article has been corrected with minor changes. These changes do not impact the academic content of the article.
Additional information
Funding
References
- World Health Organization. Preterm Birth [March 1, 2023]. Available from: https://www.who.int/news-room/fact-sheets/detail/preterm-birth.
- Chawanpaiboon S, Vogel JP, Moller AB, et al. Global, regional, and national estimates of levels of preterm birth in 2014: a systematic review and modelling analysis. Lancet Glob Health. 2019;7(1):1–13. Jan doi: 10.1016/S2214-109X(18)30451-0.
- Perin J, Mulick A, Yeung D, et al. Global, regional, and national causes of under-5 mortality in 2000-19: an updated systematic analysis with implications for the sustainable development goals. Lancet Child Adolesc Health. 2022;6(2):106–115. Feb doi: 10.1016/S2352-4642(21)00311-4.
- Jin W, Hughes K, Sim S, et al. The contemporary value of dedicated preterm birth clinics for high-risk singleton pregnancies: 15-year outcomes from a leading maternal Centre. J Perinat Med. 2021;49(9):1048–1057. Nov 25 doi: 10.1515/jpm-2021-0020.
- Campbell F, Salam S, Sutton A, et al. Interventions for the prevention of spontaneous preterm birth: a scoping review of systematic reviews. BMJ Open. 2022;12(5):e052576. May 13 doi: 10.1136/bmjopen-2021-052576.
- Abdel-Aleem H, Shaaban OM, Abdel-Aleem MA, et al. Cervical pessary for preventing preterm birth in singleton pregnancies. Cochrane Database Syst Rev. 2022;12(12):CD014508. Dec 1 doi: 10.1002/14651858.CD014508.
- Wennerholm UB, Bergman L, Kuusela P, et al. Progesterone, cerclage, pessary, or acetylsalicylic acid for prevention of preterm birth in singleton and multifetal pregnancies - A systematic review and meta-analyses. Front Med (Lausanne). 2023;10:1111315. doi: 10.3389/fmed.2023.1111315.
- Parry S, Leite R, Esplin MS, et al. Cervicovaginal fluid proteomic analysis to identify potential biomarkers for preterm birth. Am J Obstet Gynecol. 2020;222(5):493 e1-493–e13. May doi: 10.1016/j.ajog.2019.11.1252.
- Law KP, Han TL, Tong C, et al. Mass spectrometry-based proteomics for pre-eclampsia and preterm birth. Int J Mol Sci. 2015;16(5):10952–10985. May 14 doi: 10.3390/ijms160510952.
- Goldenberg RL, Gravett MG, Iams J, et al. The preterm birth syndrome: issues to consider in creating a classification system. Am J Obstet Gynecol. 2012;206(2):113–118. Feb doi: 10.1016/j.ajog.2011.10.865.
- Steyerberg EW, Moons KG, van der Windt DA, et al. Prognosis research strategy (PROGRESS) 3: prognostic model research. PLoS Med. 2013;10(2):e1001381. doi: 10.1371/journal.pmed.1001381.
- Saade GR, Boggess KA, Sullivan SA, et al. Development and validation of a spontaneous preterm delivery predictor in asymptomatic women. Am J Obstet Gynecol. 2016;214(5):633 e1-633–e24. May doi: 10.1016/j.ajog.2016.02.001.
- Burchard J, Saade GR, Boggess KA, et al. Better estimation of spontaneous preterm birth prediction performance through improved gestational age dating. J Clin Med. 2022 May 19;11(10):2885.
- Markenson GR, Saade GR, Laurent LC, et al. Performance of a proteomic preterm delivery predictor in a large independent prospective cohort. Am J Obstet Gynecol MFM. 2020;2(3):100140. Aug doi: 10.1016/j.ajogmf.2020.100140.
- Khanam R, Fleischer TC, Boghossian NS, et al. Performance of a validated spontaneous preterm delivery predictor in South asian and Sub-Saharan african women: a nested case control study. J Matern Fetal Neonatal Med. 2022;35(25):8878–8886. Dec
- Aftab F, Ahmed S, Ali SM, et al. Cohort profile: the alliance for maternal and newborn health improvement (AMANHI) biobanking study. Int J Epidemiol. 2022;50(6):1780–1781i. Jan 6 doi: 10.1093/ije/dyab124.
- Collins GS, Reitsma JB, Altman DG, et al. Transparent reporting of a multivariable prediction model for individual prognosis or diagnosis (TRIPOD): the TRIPOD statement. BMC Med. 2015;13(1):1. Jan 6(doi: 10.1186/s12916-014-0241-z.
- Villar J, Papageorghiou AT, Knight HE, et al. The preterm birth syndrome: a prototype phenotypic classification. Am J Obstet Gynecol. 2012;206(2):119–123. Feb doi: 10.1016/j.ajog.2011.10.866.
- Villar J, Giuliani F, Bhutta ZA, et al. Postnatal growth standards for preterm infants: the preterm postnatal follow-up study of the INTERGROWTH-21(st) project. Lancet Glob Health. 2015;3(11):e681-91–e691. Nov doi: 10.1016/S2214-109X(15)00163-1.
- Lash TL. Rothman K. Case-control studies. In: lash TL, Vanderweele TJ, Haneuse S, Rothman KJ, editors. Modern epidemiology., 4th edition. Philadelphia: lippincott, Williams & Wilkins; 2021. p. 111–127.
- Bradford C, Severinsen R, Pugmire T, et al. Analytical validation of protein biomarkers for risk of spontaneous preterm birth. Clin Mass Spectrom. 2017; 2017/01/01/3:25–38. doi: 10.1016/j.clinms.2017.06.002.
- Westgard JO, Barry PL, Hunt MR, et al. A multi-rule shewhart chart for quality control in clinical chemistry. Clin Chem. 1981;27(3):493–501. Mardoi: 10.1093/clinchem/27.3.493.
- Tibshiriani R. Regression shrinkage and selection via the lasso. J R Statist Soc B. 1996;3:273–282.
- NCSS PASS Statistical Software. [March 1, 2023]. Available from: https://www.ncss.com/software/pass/.
- SAS Version 9.3 [August 1, 2022]. Available from: http://support.sas.com/software/93/.
- R Foundation. The R Project for Statistical Computing [August 1, 2022]. Available from: https://www.r-project.org/.
- Yoshida K, Bartel A. tableone: Create ‘Table 1’ to Describe Baseline Characteristics with or without Propensity Score Weights; R package version 0.13.2 2022 [November 21,2022]. Available from: https://cran.r-project.org/web/packages/tableone/index.html.
- DeLong ER, DeLong DM, Clarke-Pearson DL. Comparing the areas under two or more correlated receiver operating characteristic curves: a nonparametric approach. Biometrics. 1988; Sep44(3):837–845.
- Boniface JJ, Burchard J, Saade GR. Effects of selective exclusion of patients on preterm birth test performance. Obstet Gynecol. 2019; Dec134(6):1333–1338. doi: 10.1097/AOG.0000000000003511.
- Harrell FE, Jr., Lee KL, Mark DB. Multivariable prognostic models: issues in developing models, evaluating assumptions and adequacy, and measuring and reducing errors. Statist. Med. 1996;15(4):361–387. Feb 28doi: 10.1002/(SICI)1097-0258(19960229)15:4<361::AID-SIM168>3.0.CO;2-4.
- Finazzi S, Poole D, Luciani D, et al. Calibration belt for quality-of-care assessment based on dichotomous outcomes. PLoS One. 2011;6(2):e16110. Feb 23 doi: 10.1371/journal.pone.0016110.
- Allotey J, Whittle R, Snell KIE, et al. External validation of prognostic models to predict stillbirth using international prediction of pregnancy complications (IPPIC) network database: individual participant data meta-analysis. Ultrasound Obstet Gynecol. 2022;59(2):209–219. Feb doi: 10.1002/uog.23757.
- Forbes K, Westwood M. Maternal growth factor regulation of human placental development and fetal growth. J Endocrinol. 2010; Oct207(1):1–16. doi: 10.1677/JOE-10-0174.
- Crosley EJ, Dunk CE, Beristain AG, et al. IGFBP-4 and -5 are expressed in first-trimester villi and differentially regulate the migration of HTR-8/SVneo cells. Reprod Biol Endocrinol. 2014;12(1):123. Dec 4 doi: 10.1186/1477-7827-12-123.
- Qiu Q, Bell M, Lu X, et al. Significance of IGFBP-4 in the development of fetal growth restriction. J Clin Endocrinol Metab. 2012;97(8):E1429–39. Aug doi: 10.1210/jc.2011-2511.
- Nawathe AR, Christian M, Kim SH, et al. Insulin-like growth factor axis in pregnancies affected by fetal growth disorders. Clin Epigenetics. 2016;8(1):11. doi: 10.1186/s13148-016-0178-5.
- Hammond GL. Diverse roles for sex hormone-binding globulin in reproduction. Biol Reprod. 2011; Sep85(3):431–441. doi: 10.1095/biolreprod.111.092593.
- Larrea F, Díaz L, Cariño C, et al. Evidence that human placenta is a site of sex hormone-binding globulin gene expression. J Steroid Biochem Mol Biol. 1993;46(4):497–505. Oct doi: 10.1016/0960-0760(93)90104-5.
- Simó R, Sáez-López C, Barbosa-Desongles A, et al. Novel insights in SHBG regulation and clinical implications. Trends Endocrinol Metab. 2015;26(7):376–383. Jul doi: 10.1016/j.tem.2015.05.001.
- UNICEF. Maternal Child Health [June 1, 2023]. Available from: https://www.unicef.org/vietnam/maternal-child-health.
- Cheong-See F, Allotey J, Marlin N, et al. Prediction models in obstetrics: understanding the treatment paradox and potential solutions to the threat it poses. BJOG. 2016;123(7):1060–1064. Jun doi: 10.1111/1471-0528.13859.
- Moons KG, Kengne AP, Grobbee DE, et al. Risk prediction models: II. External validation, model updating, and impact assessment. Heart. 2012;98(9):691–698. May doi: 10.1136/heartjnl-2011-301247.