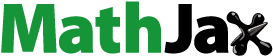
ABSTRACT
Hybrid renewable energy system-based reverse osmosis desalination has been identified as a reliable and sustainable solution for supplying potable water to the off-grid communities. In this respect, this study proposes a stand-alone hybrid energy system comprising solar PV, wind turbine, diesel generator, and a battery bank to power a reverse osmosis desalination plant. The proposed hybrid system is optimised using a novel metaheuristic optimisation technique named salp swarm algorithm (SSA) to minimise the three objective functions: levelised cost of energy (LCOE, $/kWh), life cycle emissions (LCE, CO2 kg-eq/yr.), and the excess energy (EE, kWh/yr.). Moreover, the performance of the novel algorithm is scrutinised using the benchmark solutions achieved from the widely utilised non-dominated sorting genetic algorithm II (NSGA-II) and particle swarm optimisation (PSO) techniques. In addition, the study also investigates the effects of water demand and the loss of power supply probability on the techno-economic performance. The results reveal that SSA outperforms NSGA-II and PSO algorithms towards achieving Pareto optimality hence provides financially rewarding solutions. The LCOE for the optimal HES is 0.179 $/kWh, and the LCE is 5921 CO2 kg-eq/yr. with an EE of 402 kWh/yr., whereas the cost of water for this system is 1.37 $/m3.
1. Introduction
Freshwater is a biological necessity for human wellbeing (Feizizadeh et al. Citation2021) since drinking contaminated water causes health hazards to the human body. Although 71% of the world is covered in water, only 2.5% is safe and potable (Dinka Citation2018). Every year, more than 3.4 million people die from waterborne diseases, making it one of the leading causes of illness and death worldwide (Organization Citation2017). However, freshwater supply is limited, and their sources are increasingly threatened due to population growth and industrial development (Hajibabaei, Nazif, and Sereshgi Citation2018). Furthermore, global climate change influences the freshwater resources and causes water shortage due to the drying up of lakes, rivers, or streams (Makkeh et al. Citation2020; Balogun et al. Citation2020). The scarcity of freshwater increasingly affects the global economy, environment and even creates conflicts amongst countries and regions. According to the World Wildlife Fund (WWF), around 1.1 billion people worldwide have no access to safe, clean drinking water, and 2.7 billion faces water scarcity for at least one month per annum (Abdusamatov Citation2012). Moreover, global water demand is expected to increase by 20–30% by 2050 (Boretti and Rosa Citation2019). In contrast, water scarcity will continue to spread in areas due to the depletion of freshwater resources.
The global energy demand is ever-escalating due to population, economic and technological growth. The current energy sector extensively depends on fossil fuels, which negatively impacts our environment by contributing significantly to carbon emissions and subsequent global climate change (Yuan et al. Citation2020; Das et al. Citation2021e). The shifting of the conventional fossil fuel system to renewable-based cleaner energy will certainly mitigate global climate change (Abu-Rayash and Dincer Citation2021). Desalination processes based on the conventional fossil fuels cause CO2 emissions and, consequently, have detrimental effects on the environment in addition to the extra expenses (Sharif et al. Citation2020). A review on the hybrid energy application for developing countries suggests that the diesel option has a higher cost than the hybrid system and the hybrid renewable option is quicker to install than the grid extension (Zebra et al. Citation2021). Therefore, renewable energy-driven desalination processes would be a potential alternative method for producing fresh water and overcoming the global water crisis (Santosh et al. Citation2019). The recent technological development promotes solar and wind technologies extensively for stand-alone energy system (Fathollahzadeh et al. Citation2021; Luo, Xia, and Liu Citation2021). However, renewable resources are extremely intermittent due to seasonal patterns and uncontrollable availability. Therefore, multiple renewable energy resources are combined with non-renewable energy sources to increase efficiency and reliability (Halabi and Mekhilef Citation2018). The hybrid energy system (HES) has various advantages, including low energy cost, fuel-saving, continuous energy supply, lower maintenance, and high efficiency (Halabi and Mekhilef Citation2018). However, the energy management techniques in hybrid systems and component sizing can be very complicated. The appropriate optimisation of hybrid systems can be more cost-effective and reliable for small-scale saltwater desalination plants, particularly in off-grid areas. Numerous HES combinations have been reported for reverse osmosis (RO) desalination, including PV/Diesel (Ghenai et al. Citation2018), PV/WT/Diesel (Gökçek Citation2018), PV/WT (Charrouf et al. Citation2020), PV/WT/BG/HT/Diesel (Mousavi et al. Citation2020), PV/WT/Bat (Wang et al. Citation2019), PV/WT/Bat/Diesel (Elmaadawy et al. Citation2020), PV/Bat/Fuel cell (Clarke, Al-Abdeli, and Kothapalli Citation2015), and PV/WT/Biomass/FC (Roy, Hassan, and Das Citation2022). The hybrid system combinations primarily depend on energy demand, renewable energy resources, weather, metrological conditions, and project economics (Halkos and Skouloudis Citation2018; Das, Hasan, and Das Citation2021b). However, the optimal sizing of the HES is essential to achieve multiple objectives, such as meeting reliable load demand, fuel-saving, and reducing CO2 emission while maintaining a minimum cost of energy (COE) (Shezan et al. Citation2016; Movahediyan and Askarzadeh Citation2018).
To summarise, the objectives of the HES sizing optimisation for desalination purposes are mostly economic and environmental. Net present system cost, COE and water cost have been the proven indicators around which the determination of economic feasibility revolved. However, the concern of ecological betterment has been confined only in the emission from non-renewable generators because the operation of the renewable part of the hybridised systems does not produce any emission. Nevertheless, there are emissions associated with different phases of their life cycle starting from manufacture to disposal (Barakat, Ibrahim, and Elbaset Citation2020) termed as lifecycle emission (LCE). This parameter projects towards the actual emission scenario of the HES, however, the LCE for the HES for meeting the RO demand has rarely been investigated. Excess energy (EE) is another aspect of HESs, worth considering in the design process, but has mostly been investigated as a consequential parameter. The potential for a large reduction in component size due to the reduction of surplus energy will ultimately result in a lower system cost (Samy, Barakat, and Ramadan Citation2019). Evidence suggests that a 20% reduction in EE would have about one and half effect on the COE (Hossam-Eldin, El-Nashar, and Ismaiel Citation2012).
The discussed parameters have been introduced separately in different optimisation studies. However, the combined application of these parameters in the design process has the enormous prospect of furthering the economic and environmental benefits which is yet to be examined. The present study is intended for filling that gap. Therefore, the following are the study’s primary contributions:
Solar PV, wind, battery, and diesel generator-based hybrid renewable energy systems for powering a reverse osmosis desalination plant have been proposed for coastal area application in Bangladesh. The system optimisation has been carried out with the multi-objectives of minimising the levelised COE, lifecycle emissions (LCE), and EE generation.
A novel metaheuristic optimisation algorithm named salp swarm algorithm (SSA) has been introduced for optimal sizing of the HES. Additionally, widely utilised algorithms non-dominated sorting genetic algorithm II (NSGA-II) and Particle swarm optimisation (PSO) have been employed to compare the performances while achieving the best possible HES sizing in agreement with the objective functions.
The reliability of energy supply has been secured by including the widespread reliability indicator loss of power supply probability (LPSP) as one of the constraints. Each of the systems are optimised to ensure more than 99% reliability.
The effects of water demand and reliability on the techno-economic and environmental aspects are comprehensively investigated through sensitivity analysis. The study is further extended to investigate the techno-economic benefits over a diesel-driven ROD unit.
2. Review of related literature
Various optimisers have been exploited for the purpose of obtaining the sizing optimality of HESs. For instance, different metaheuristic evolutionary algorithms (Jahannoosh et al. Citation2020), linear and nonlinear programming (Zhang et al. Citation2020, Wang et al. Citation2021), artificial neural networks (Cheng et al. Citation2017), numerical iterative methods (Sanajaoba and Fernandez Citation2016), and various commercially available software tools (El-Houari et al. Citation2020; Nyeche and Diemuodeke Citation2020) are worth mentioning. Various relevant studies have been presented in . In one of such works, Kyriakarakos et al. (Citation2017) designed a PV/Bat/RO system with fuzzy cognitive maps for variable load management for 200 m3 freshwater production. The sizing of the components was optimised using PSO. Variable load management considerably increased drinking water production from 41% to nearly 54%. Wu et al. (Citation2018) designed a PV/Bat/DG-based HES for the RO desalination and optimised the system using an efficient heuristic Tabu search algorithm. They reported that the Tabu search method provides good results compared to harmony search (HS) and simulated annealing (SA). Additionally, they analysed the sensitivity by varying fuel cost, interest rate, and capital cost. Zhao et al. (Citation2021) analysed a renewable-based PV/WT configuration with compressed air storage for sea water RO system along with both off-grid and grid-connected modes. The study revealed that COE was 0.12 $/kWh for an on-grid system and 0.34 $/kWh with a stand-alone system. El Boujdaini et al. (Citation2022) optimised a PV/WT/DG/bat system with PSO algorithm to meet house load in three different locations and obtained optimum COE of 0.57$/kWh, RF 29%, LPSP 3.9%, and dump energy 15,000 kWh. In a similar study, Sanaye and Sarrafi (Citation2021) proposed an innovative PV/WT/LPG system to meet power, cooling, heating, and clean water demand for a remote household using combined artificial neural network (ANN) and PSO optimisation. They reported that this method decreases the run time by 10%, and it is possible to save 73,361 kg of CO2 annually. Zhang et al. (Citation2018) investigated a hybrid PV/WT/Bat reverse osmosis desalination (ROD) plant. They optimise the HES using an SA-chaotic search algorithm to minimise the life cycle cost. Results indicated that the hybrid algorithm offers a cost-effective solution than the single algorithm, such as a chaotic search and SA. Ibrahim et al. (Citation2020) optimised two different combinations, PV/WT/Bat/DG and PV/HKT/Bat/DG, to operate a desalination plant capable of producing 1 m3/h water. The systems were analysed using the HOMER optimisation tool, and PV/HKT/Bat/DG was the optimum system with a COE of 0.122 $/kWh and the water cost of 0.56 $/m3. Lilas et al. (Citation2022) utilised renewable energy to produce fresh water and electricity with battery and compressed air storage.
Table 1. Different HRES configurations to meet drinking water demand with ROD system.
Gtari, Hamdi, and Hazami (Citation2022) used PV-thermal solar system to power RO desalination system and the study offered 0.354 $/kWh water cost with emission reduction. Moreover, Hossam-Eldin, El-Nashar, and Ismaiel (Citation2012) optimised an economical desalination plant using renewable energy sources for the purpose of electricity and fresh water. Hemeida et al. (Citation2022) studied a real-case micro-grid renewable energy system, where they obtained COE of 0.272 $/kWh, LPSP of 0.1397, and RF as 92.37%. Moreover, Das et al. (Citation2022) analysed a hybrid renewable energy system to supply fresh water as well electricity. In this study, EE was considered for the ROD system, and it resulted in COE of 0.234 $/kWh, job creation of 1.64 jobs, and 24,038 kWh/yr. EE. Delgado-Torres and Garcia-Rodriguez (Citation2022) analysed a tidal/PV hybrid system for the RO desalination system. In the study, they analysed overall investment cost per unit production and non-useful energy for water desalination.
Likewise, Mokheimer et al. (Citation2013) simulated a hybrid PV/WT/Bat with a 1 kW fixed RO load. They simulated the mathematical model in the MATLAB software package and compared the results with HOMER. The corresponding energy cost and desalination costs were 0.672 $/kWh and $3.693–$3.812/m3. Liu, Wu, and Maleki (Citation2022) studied a stand-alone solar diesel-based system for the RO desalination system with the tabu search algorithm and HS algorithm in order to reduce the production cost and emission. In another study, Ghaithan et al. (Citation2022) optimised a PV/wind hybrid system to power the RO desalination system. The study resulted in 0.0557 $/kWh COE and 90,899 kg CO2-eq/yr. emission. Moreover, Najjar et al. (Citation2022) concluded with a cost of water (COW) of 0.94–1.47 $/m3 for the renewable-based RO system. Furthermore, Shafaghat, Eslami, and Baneshi (Citation2023) analysed a PV-thermal collectors for the RO desalination purposes. From the study, it is seen that the system reduced the price of water by 12–13.8% and emission of 79–85.7% compared to the conventional PV-based RO system.
Peng et al. (Citation2018) compared 19 evolutionary optimisation algorithms to size a stand-alone PV/WT/Bat system for a ROD in Iran. The objective functions were life cycle cost and loss of power supply probability (LPSP) for higher reliability. Zhang et al. (Citation2019) used a PV/WT/Hydrogen combination for a ROD system and optimised the system using weather forecasting and a hybrid algorithm. The proposed hybrid algorithm developed from chaotic search, HS, and SA. Additionally, weather forecasting, coupled with artificial neural networks, was used to improve sizing accuracy. Abdelshafy, Hassan, and Jurasz (Citation2018) used a multi-objective hybrid particle swarm optimisation–Grey Wolf optimiser (PSO–GWO) optimisation method to design a grid-connected PV/WT/Bat powered desalination plant that reduces both freshwater production cost and CO2 emissions. They also checked the sensitivity of the system, varying solar irradiance, and wind speed. Sawle, Gupta, and Bohre (Citation2018) reviewed hybrid off-grid system optimisation and reported that the intelligent optimisation techniques offer lower cost with higher reliability than the other modelling tools such as HOMER. The hybrid system sizing considering the cost, lifecycle emissions, and at the same time reducing the EE generation is rarely available in the literature. Additionally, the present study uses newly developed SSA for sizing the HES for meeting the freshwater demand in the coastal area and the outcomes are compared with the NSGA-II and PSO optimisation techniques.
3. Materials and methods
This section offers the technical and financial details of the proposed HES. Moreover, the energy management strategy (EMS) and the detailed optimisation frameworks of this study have been included in the respective subsections.
3.1. System architecture
represents a conceptual design of a PV/WT/DG-based hybrid power-driven ROD system with a battery bank. It also includes a bidirectional inverter to convert direct current to alternating current or vice versa. The main source of the power, PV modules, is connected to the DC bus with a battery bank. The diesel generator, wind turbine, and RO desalination system are connected to the AC bus. Battery bank stores the surplus part of generation and releases in occasions of deficiency. The generator is generally used as a backup source of power. Sea water is desalinated in the RO unit and eventually sent to the community as per the demand. In this study, the RO unit's capital cost, membrane replacement cost, O&M cost, chemical cost, and water tank cost are calculated as 532 $/m3/day, 0.06 $/m3, 0.2 $/m3, and 255.4 $/m3 correspondingly [46]. The system’s daily water requirement is 20 m3/day, and the membranes are replaced twice every year (Das et al. Citation2022). The technical and financial details of the HES components supplying the required energy to the desalination process are presented in and , respectively.
Table 2. Technical information of the proposed HES components.
Table 3. Financial information of the proposed HES components.
3.2. Energy management strategy
The HES’s energy dispatch plan for supplying electricity to the RO desalination process is shown in . The input parameters are hourly solar irradiation and wind velocity data from HOMER, as well as hourly load demand. In the initial stage, Enet(t) is calculated by subtracting the load demand from the total renewable outputs for each time step t. The excess quantity is used to charge the battery if the total renewable supply exceeds the load needed and the battery SOC (Ebatt(t)) is larger than the maximum battery energy (Ebatt, max). EE, also known as wasted energy, is dumped when the battery energy (Ebatt(t)) reaches its extreme capability. Enet(t) = 0 is the desired scenario of the study, where neither unmet load nor EE is produced. Furthermore, if RE sources fail to fulfil the load demand, the EMS first determines whether the battery SOC (Ebatt(t)) is larger than the minimum SOC (Ebatt,min). If the summation of RE sources and battery energy is larger than load demand, the battery is discharged to meet the load demand until its minimum SOC (Ebatt,min) is reached. When the cumulative output from RE sources and battery banks is insufficient to fulfil the load demand, the diesel generator is started if the shortage is equal to or more than the diesel generator’s minimum starting threshold. Unmet load occurs when RE and battery banks are unable to meet load demand and the load deficiency is less than the start-up threshold. The algorithm continues to iterate through each timestep until t = 8760 is reached and the optimiser takes on from there.
3.2. Feasibility indicators
The best configuration of HES must be feasible in terms of economic, environmental, and technical aspects. This section is dedicated to defining such indicators adopted in this study.
3.2.1. Economic indicators
a) | Net present cost |
The net present cost (NPC) is considered as one of the initial indicators for economic feasibility. It is made up of the component's initial cost (Cini), O&M cost (CO&M), and replacement cost (Crep), less the current salvage (Csalvage) value that is left unused over its lifetime. The NPC for each component is calculated using Equation (1) (Kharrich et al. Citation2021)
(1)
(1) Equation (2) can be used to calculate the capital cost of each component, where Ncomp denotes the number of optimal components and Ccap,comp denotes the component's capital procurement price ($/kW)
(2)
(2) The replacement cost for each component can be attained as follows:
(3)
(3) whereas
and
where Crep,comp denotes the component’s replacement cost at the end of its lifetime, Nrep-comp denotes the number of times a component has been replaced during its lifetime, LFproject denotes the project lifetime (25 years), LFcomp denotes the component lifetime, i denotes the real discount rate (10%), i′ denotes the nominal discount rate, and f denotes the inflation rate. The following are the costs of O&M for each component:
(4)
(4) where CO&M,comp is the operation and maintenance cost of the component.
The salvage value for each component can be calculated as follows:
(5)
(5) whereas
(6)
(6)
(7)
(7) The cost of fuel for a diesel generator can be calculated using Equation (8)
(8)
(8) where Pfuel is the yearly fuel consumption (L/year) and Ffuel is the annual fuel cost ($/L).
The RO's overall cost may be computed as follows (Maleki Citation2018, Zhang et al. Citation2018, Wu et al. Citation2018):
(9)
(9) where CCWTa denotes the capital cost of the freshwater tank, and CCRO, MCRO, TCMR, and TCChem are the capital cost, O&M cost, total membrane replacement cost, total chemical cost, and TCChem are the capital costs of the RO system, correspondingly. All these expenses can be calculated as follows:
(10)
(10)
(11)
(11)
(12)
(12)
(13)
(13)
(14)
(14)
(15)
(15)
As shown in Equation (15), CRO represents the cost of a RO system per unit volumetric capacity of daily generated desalinated water by ROD, CaWD denotes the daily volumetric capacity of the ROD system, and CRF signifies the capital recovery factor. Again, the annual ROD maintenance cost is CMnt-RO, the daily water demand is DWD, the membrane replacement cost is CMR, and the number of membrane replacements per year is NMe. Furthermore, CCH stands for chemical cost, while CWTa stands for water tank cost, which comprises the price of the water tank as well as the cost of installation.
b) | Levelised COE |
The LCOE refers to the cost associated with per unit energy production from the system. It is calculated by EquationEquation (16)(16)
(16) (Das, Al-Abdeli, and Kothapalli Citation2017)
(16)
(16)
3.2.2. Environmental indicators
a) | Lifecycle emission |
The lifecycle emission of a system component is attributed to the GHG emissions associated with different phases of its lifecycle such as: manufacturing, assembly, installation, and disposal. All sorts of GHG emissions are termed as CO2 equivalent emission and in that way the total emission from the HES is calculated as follows (Das, Hassan, et al. 2021c; Alshammari and Asumadu Citation2020):
(17)
(17) where
denotes lifetime equivalent CO2 emissions of the HES component
, and the quantity of energy delivered by that component of the system in a period of T (8760 h) is denoted by Ep. presents the lifecycle emission factors of different components in the system.
b) | Renewable fraction |
Table 4. CO2 life cycle equivalent emission for HES desalination process.
The renewable fraction is the portion of the total energy provided by the HES that is generated by renewable sources (Das, Tushar, and Hassan Citation2021d)
(18)
(18) Here,
is energy produced by a non-renewable source, in this case a diesel generator.
3.2.3. Technical indicators
a) | Excess energy |
EE is the portion of energy output that is not used while meeting demand and is thus dumped. In the present case, the EE produced by the system in each time step is calculated as follows (Das et al. Citation2022):
(19)
(19)
b) | Loss of power supply probability |
It refers to the probability that the system will fail to supply the required demand over a certain period and can be calculated by EquationEquation (20)(20)
(20) (Das, Al-Abdeli, and Kothapalli Citation2017)
(20)
(20) In Equation (20),
stands for entire electrical demand (kWh), and LPS stands for loss of power supply throughout the time period T, as given by Equation (21)
(21)
(21)
2.4. Optimisation problem formulation
The optimisation problem for this study is formulated by selecting the proper objective functions and constraints from the feasibility indicators. The primary goal of the optimisation method is to determine the best size of HES components while minimising the system's levelised cost of energy (LCOE), life cycle emissions (LCE), and surplus energy (EE). Accordingly, the multi-objective functions may well be well-defined as follows:
(22)
(22) These objective functions are subjected to some constraints. The integer number of PV modules (NPV), number of wind turbines (NWT), number of diesel generators (NDG), number of batteries (NBatt), and number of inverters are all optimal variables in the current sizing problem (NInv). The numbers of each decision variable are confined to predefined boundaries for the reduction of the solution space (Equation (23)). Moreover, energy balance constraint and battery storage capacity constraints have been taken into consideration as presented in Equations (24), (25), and (26), respectively
(23)
(23)
(24)
(24)
(25)
(25)
(26)
(26) EPV, EWT, EBatt and stand for PV, wind turbine, battery bank, and diesel generator energy, respectively, while PL stands for the study’s load demand; DOD refers to the battery bank’s maximum depth of discharge. Ebatt,min and Ebatt,max show the battery’s least and maximum state of charge. Furthermore, as a dependability indicator, the study looks at the following LPSP restriction:
(27)
(27) The ideal LPSP in this investigation is set at 1 ± 0.5%.
2.5. Proposed optimisation algorithms
Optimum sizing of HES components is prerequisite for efficient and cost-effective hybrid energy utilisation to satisfy reliable water load demand at fewer environmental emissions. In this study, SSA, NSGA-II, and PSO algorithms are used to optimise the HES-based ROD system.
2.5.1. Salp swarm algorithm (SSA)
SSA is introduced by Mirjalili et al. (Citation2017) which is inspired by the mechanism of swarm creation of jelly fish like biological entity named salps. The swarm of salps is called salp chain (Mirjalili et al. Citation2017). To form a salp chain it is required to select a leader which is the salp that locates the potential source of food. The other salps of the swarm then act as follower positioning in a queue just behind the leader one by one. This distinctive feature of swarm creation acted as the galvaniser to incorporate this behaviour in a mathematical approach which eventually formed the structure of SSA.
For any problem, the random solution can be represented as the random combinations of decision variables. Any random solution can be represented as follows:
(28)
(28) where
is the
th random solution and
is the
th decision variable of
th random solution.
For any swarm, the initial swarm can be represented as the cumulation of different random solutions. Any initial swarm can be expressed as follows:
(29)
(29) To construct the mathematical structure of SSA we need to identify the location of potential food source
. Alike in meaning compared with biological attribute, the best solution in the swarm can be represented as the potential food location
which resembles as the virtual food location in respect to optimisation model.
Then, the position of leader can easily be obtained following the food location modified through bounds of decision variables along with some random numbers. To attribute this characteristic, the following equation is maintained:
(30)
(30) where
is the value of the leader (first salp) for
th decision variable,
is the value of potential food source for
th decision variable,
is the value of upper bound for
th decision variable,
is the value of lower bound for
th decision variable,
is the tuning factor between exploration and exploitation and
,
are random numbers.
The co-efficient can be represented as follows:
(31)
(31) where
is the current iteration number and
is the maximum iteration number. The value of
is of very important as it balances between exploration and exploitation followed by exploration in the early stage and eventually moving to exploitation gradually. It enhances the performance of the algorithm.
The parameter and
are random numbers generated uniformly in the range of [0,1]. They control if the next
th decision variable tends to move towards positive infinity or negative infinity as well as determining the step size (Mirjalili et al. Citation2017).
To update the values of follower salps, the following equation is incorporated:
(32)
(32) where
is the value of
th decision variable of
th follower salp.
The total swarm is created and finally, modified using Equations (30) and (32) running through the loop until the end criteria are not met. The optimisation flow chart of PSO is presented in . The values of the different optimisation parameters are adopted as follows: Nrun = 30, number of salp = 100, Niteration = 300.
2.5.2. Non-dominating sorting genetic algorithm II
NSGA-II is a multi-objective evolutionary algorithm used to attain a required set of non-dominated solutions, which is generally approached by weighted or aggregated sum approaches (Zaman et al. Citation2019). It uses a fast non-dominated sorting approach and an exclusive strategy, which reserves its solutions and converges towards the best solution (Huang et al. Citation2019). The working principle of NSGA-II is shown in . The values of the different optimisation parameters are adopted for better performance as follows: Nrun = 30, population size = 300, Niteration = 500, crossover rate = 0.9, mi = 0.1, infeasible ratio = 0.2.
2.5.3 Particle swarm optimisation
PSO is a population-based metaheuristic algorithm that generates optimum global results by imitating animal community behaviour (e.g. flocking of birds, schooling of fish). It is a swarm-based evolutionary algorithm that operates based on personal and global best perceptions where personal best means achieved best solution quality, i.e. optimised fitness of each particle so far, and global best means most optimised solution achieved among all the particles so far. After each iteration, both personal best and global best are updated through vector updating. Abdelshafy, Hassan, and Jurasz (Citation2018) provided the mathematical expressions. depicts the PSO optimisation flow chart. The values of the different optimisation parameters are adopted as follows: Nrun = 30, particle size = 100, Niteration = 300, wmax = 0.9, wmin = 0.2, c1 = 2, c2 = 2.
3. Case study
The case study area is located at Kutubdia Island in the Bay of Bengal, Bangladesh (21° 49.0′ N, 91°51.5′ E). illustrates a location map of the area. The research region has no grid electricity, and one of the key issues is the saline water. The salinity level of this area is very high (Total Dissolved Solid, TDS ≥ 2000 mg/L; and NaCl ≥ 2000 mg/L) than the standard value of World Health Organization (WHO) (TDS ≤ 1000 mg/l; NaCl ≤ 150–1000 mg/L) (Rahman et al. Citation2017). In the present study, a remote area with 1000 population is considered to supply fresh drinking water.
3.1. Meteorological data and renewable resources
The study area is well furnished with a sufficient sunshine hour and excellent wind velocity. The solar irradiance and wind velocity profile of the study area are taken from NASA's HOMER software (HOMER Pro version 3.14.5) database. Using the coordinate of the study area (Kutubdia Island, Latitude: 21° 49.0′ N, Longitude: 91°51.5′ E), the climatic data has been extracted from the HOMER software tool. shows the hourly solar irradiance and wind velocity of the study location. The average solar irradiation and wind velocity of the selected area are 4.80 kWh/m2/day and 3.5 m/s, respectively. The area enjoys sufficient sunshine year-round, except for the drop in July–August and maximum wind velocity is obtained in July, where minimum speed is attained in December. It is evident from that the area has solar irradiation in the range of 0.1–0.5 kW/m2 for over 3200 h, whereas it receives irradiation of 0.6 kW/m2 or above for over 1500 h. From , it is clearly shown that the wind flows in the ranges of 2–6 m/s over the study for around 85% times of the year.
3.2. Load profile assessment
According to the WHO, each individual needs 20 L of fresh water every day (Ibrahim et al. Citation2020). Therefore, around 20 m3 daily freshwater is necessary per person. It is estimated that 1 m3 of freshwater production with an RO desalination system consumes 4.38 kWh of electrical energy (Ibrahim et al. Citation2020). In this context, to provide 20 m3 of desalinated freshwater for 1000 people, approximately 87.6 kWh/day of energy is required. shows hourly load demand and water demand per day, where the maximum load demand is 10 kW with an average load of 3.65 kW and the maximum water demand is 2.3 m3/day.
4. Results and discussion
A PV/WT/DG-based hybrid power system with battery storage for a community-scale RO seawater desalination system is modelled and optimised using three different artificial techniques: NSGA-II, PSO, and SSA in the MATLAB environment. The study employs fuzzy decision-making technique modelled according to this study (Brka, Al-Abdeli, and Kothapalli Citation2015) to extract the optimum result i.e. the solution with the best trade-off from the set of non-dominant solutions on the Pareto front. Two configurations are studied based on the inclusion of wind turbine generators. The optimised results are presented in .
Table 5. Optimised results of the RO desalination HES.
4.1. Financial analysis
Results presented in show that the PV/Bat/DG offers cost-effective solutions in each case with NPC ranging between $141,940 and $146,230. The PV/Bat/DG cost is lower than the PV/WT/Bat/DG-based HES regardless of the optimisation techniques due to the high capital cost of the wind turbine and insufficient wind energy potential of the studied area. The NPC for the PV/WT/Bat/DG-based HESs ranges from $167,110 to $188,680. Additionally, the SSA algorithm offers cost-effective solutions than the NSGA-II and the PSO optimisation techniques, as reported in . The system offers excellent reliability with zero LPSP and no unmet load. Conversely, the worst case is the PV/WT/Bat/DG with the GA from the perspective of system cost ($188,680), which is the maximum compared to all studied configurations.
shows the comparison amongst various hybrid arrangements based on LCOE and COW obtained from different algorithms. It is observed that PV/WT/Bat/DG system is costlier than the PV/Bat/DG regardless of the techniques used. The addition of wind turbines to the system increases the energy cost while supporting a limited number of loads. As a result, PV/Bat/DG offers cheaper means of producing freshwater. The LCOE varies from only 0.179 to 0.185 $/kWh for different algorithms, where SSA gives preferable results. Similarly, the COW also changes with technologies and optimisation techniques. The lowest water cost was 1.37 $/m3 for PV/Bat/DG with the SSA algorithm, where the maximum COW was 1.83 $/m3 for PV/WT/Bat/DG with GA.
demonstrates the breakdown of cost components of total NPC for various hybrid configurations with different algorithms. The economic analysis includes all the costs of PV, WT, DG, battery, inverter, RO plant, and fixed costs. It is found that batteries dominate the cost components of the system and consume approximately 33–44% of the total NPC, followed by RO desalination plant (21–28%) depending on the system structure and algorithm. The renewable technologies PV and wind need only 20–29% of the cost due to their lower operating and maintenance cost over the lifetime. Since the algorithm aims to reduce the life cycle emission, the diesel generator shares only 1–3% costs being the component with the highest emission factor. The SSA-optimised results for PV/WT/Bat/DG and PV/Bat/DG are summarised in and .
Figure 13. Optimal system sizing summary of PV/WT/Bat/DG-based HES configuration using SSA algorithm.

The capital, operating, and maintenance costs, along with the membrane replacement and chemical costs are shown in . As discussed, the battery is an expensive component in the system, and it comes with high replacement and maintenance costs as well. The RO desalination plant needs considerable expense due to high operating, membrane replacement, and chemical costs. The PV and wind technologies need very high capital investment, although their operating and maintenance cost is negligible. The capital cost is insignificant for diesel generators compared to other components. However, their uses are limited due to high operational and maintenance costs. Ibrahim et al. (Citation2020) investigated an off-grid PV/WT/Bat/DG powered ROD unit having a COE of 0.22 $/kWh and COW of 1.10 $/m3 for the application in Egypt. Atallah et al. (Citation2020) found an optimal configuration of a PV/Bat/DG-based HES for powering a ROD unit with a COE and NPC of 0.107 $/kWh and $502,662, respectively. A large-scale ROD unit powered by a PV/WT/Bat/DG is optimised by Elmaadawy et al. (Citation2020) and the study reported a COE of 0.164 $/kWh and the NPC of 3.12 M$. The outcomes of the economic metrices for this study agree with the acceptable standard of the outcomes reported in the previous works.
4.2. Technical analysis
presents the annual energy production of different configurations with different algorithms. For the optimal case (PV/Bat/DG with SSA), renewable sources dominate the energy production with the highest of 83.2% from the PV system. The optimum system generates 35,052 kWh/year, whereas PV supports 29,150 kWh/year. The remaining 16.8% is supplied by the diesel generator, which compensates for the lack of renewables and thereby meets the RO plant's power requirements. Furthermore, the system can deliver loads with 99.9% dependability, with only minor unmet loads. The optimal system dumps 402 kWh of EE which is 35.5% higher than the lowest value provided by GA (259 kWh). However, this is lower than the other cases considered.
The overall assessment indicates, sizing done by GA tends to produce lesser EE than the other two algorithms. Another aspect worth noticing is, the configurations with wind turbine produce more EE in every sizing outcome obtained. This is found in maximum quantity in PV/WT/Bat/DG system optimised by PSO (1580 kWh/yr.). In every configuration, PV domination in the production is notable.
shows the energy profile for each unit, PV, DG, and battery, to manage the power requirement of PV/Bat/DG with the SSA algorithm for four days in May. The PV, DG, and battery successfully satisfy the load demand.
4.3. Environmental impacts
The environmental impact of the different structures with GA, PSO, and SSA algorithms are compared and presented in . It is observed that PV/WT/Bat/DG with SSA is environmentally attractive and eco-friendly due to the lowest pollution (LCE = 4397 kg/yr.). Moreover, the optimal system achieved a maximum of 89% renewable penetration compared to the other systems. Eliminating WT from the configuration causes an obvious reduction in the renewable fraction, and the largest descend of 7.8% is attributed to the SSA-optimised systems. This corresponds with the significantly increased life cycle emission in the wind generator less systems.
4.4. Optimised (PV/Bat/DG) system vs. conventional diesel-only system
The LCOE of the optimal configuration is 0.179 $/kWh, which is much lower than the diesel-only system (0.22 $/kWh) as reported in . Furthermore, the NPC ($170,200) and COW (1.65 $/m3) of the diesel-only system are quite high compared to the optimised system NPC ($141,940) and COW (1.37 $/m3), respectively. However, both the systems are highly reliable, although PV/Bat/DG produces 402 kWh EE per year and unmet load is 27 kWh/yr. Since the cost and the environmental benefits of the PV/Bat/DG system are far better than the diesel-only system, integration of the proposed system for the ROD plant is techno-economically effective for freshwater generation in remote areas.
Table 6. Comparison of optimised PV/Bat/DG with diesel-only system using SSA algorithm.
4.5. Effects of load demand
shows the effect of daily water demand on the COW for PV/WT/Bat/DG with different algorithms. It has been observed that COW for all algorithms reduces significantly with the increasing daily water load. The GA always produces freshwater at a comparatively higher cost compared to the other two algorithms. The maximum water price by GA is 2.32 $/m3 at 10 m3/day and the minimum (1.43 $/m3) at 35 m3/day. Similarly, PSO produces freshwater at a higher price compared to optimised SSA. The SSA algorithm gives the cheapest rate at lower load demand compared to GA and PSO, and the COW decreases continuously with the increase in water demand up to 35 m3/day.
shows the effect of daily freshwater demand on COW for PV/Bat/DG hybrid system for three algorithms GA, PSO, and SSA, respectively. The COW decreases from 2.05 to 1.37 $/m3 due to an increased water demand load from 10 to 20 m3/day in the case of SSA. However, the water price becomes steady and changes slowly for the water demand beyond 20 m3/day. It is also noticed that PV/Bat/DG produces freshwater at a comparatively lower price than the PV/WT/Bat/DG for the respective algorithms. The addition of WT increases the capital investment, and per unit energy generation from WT is costly compared to PV. Since the constraints in the algorithms are set to minimise the energy cost, the structure without WT produces freshwater at a lower price.
4.6. Effects of load supply reliability
shows the variation of component sizing with the variation of LPSP for the optimal hybrid system. When the system reliability is higher (LPSP = 0%), the system configuration, particularly the number of generators, batteries and photovoltaic panels, is larger for the same load and water capacity. It is also observed that when the value of LPSP increases the number of PV panels, batteries, and diesel generators decreases. The reliability constraint in the algorithm limits the use of more PV, generators, and batteries if there is a minor reduction of LPSP (even 1%). The number of generators obtained for different LPSPs, 0%, 1%, 5%, 10%, and 12% are 2, 2, 2, 1, and 1, respectively.
shows the effect of changing LPSP on the LCOE ($/kWh) and NPC ($) of the optimised system PV/Bat/DG with the SSA algorithm. The LPSP varied from 0% to 12% to observe the effect on both the LCOE and NPC. It is noted that both the LCOE and NPC decrease with the increase in LPSP. Moreover, only 1% of changes in LPSP bring a significant reduction in both LCOE (3.2%) and NPC (3.66%) values due to a decrease in PV and battery size. The system can supply the RO load with a 12% deficiency at only a 0.169 $/kWh rate and $139,000 NPC. The rise of LPSP from 5% to 10% reduces the LCOE from 0.173 to 0.170 $/kWh. Therefore, with minor changes of the unmet load initially for the RO unit, a significant LCOE reduction can be achieved.
represents the effects of LPSP on EE and LCE for PV/Bat/DG configuration with the SSA algorithm. Again, the LPSP was varied from 0% to 12% to observe EE and LCE characteristics. It is seen that the LCE and EE have opposite characteristics with the increase of unmet load. The EE gradually decreases while the LCE gradually increases with the rise in LPSP from 0% to 12%. The size of both PV and battery decreases with the increasing LPSP. Therefore, the diesel generator supplies the deficient load to satisfy RO load demand and resulting in high LCE. The EE drops from 795 to only 402 kWh if 1% load demand is compromised. However, this 1% LPSP causes 1161 kg emissions to the environment.
5. Conclusions
A community-scale RO desalination plant powered by a hybrid renewable energy system is studied using three optimisation algorithms (NSGA-II, PSO, and SSA) for the coastal area application in Bangladesh. The HES is designed to satisfy the reliable load demand with an LPSP of 1 ± 0.5% for sea water desalination using the locally available renewable energy resources. The HES powering the ROD plant is optimised to minimise the COE, LCE, and EE simultaneously. The main outcomes of this investigation are listed as follows:
Results indicate that PV/Bat/DG is the best configuration with a COE of 0.179 $/kWh and COW of 1.37 $/m3. The optimum system comprises 18.6 kW PV, 123 kWh battery storage, 6 kW diesel generator, and 19 kW system converter. The system mitigates a substantial amount of CO2 emissions (5921 kg/yr.) while generating only EE of 402 kWh/yr. (1.25%).
The SSA algorithm gives better solutions on the Pareto optimal front than the widely utilised ones NSGA-II and PSO. Around 3–11% cost reduction can be achieved using SSA while optimising the HRES.
The PV/Bat/DG provides better reliability and lower cost than the PV/WT/Bat/DG in all the optimised cases; however, the LCE generation is significantly higher in the former case (5921–6115 CO2 kg-eq/yr.) than the later (4397–4629 CO2 kg-eq/yr.).
Results also indicate that the COW decreases by around 33% when the water demand increases from 10 to 20 m3/day. Moreover, the system reliability has significant effects on the NPC, COE, and EE generations.
A comparative study also reveals that the proposed HES-powered ROD has substantial costs and environmental benefits over the diesel-only system.
The above analysis has clearly identified that the proposed HES-powered ROD system is economically feasible to meet the water demand for a remote coastal community. The proposed assessment presented in this study could help the government policy makers and different stakeholders to identify the enormous prospect of integrating RE technology into the ROD unit. Further research is warranted to model and size the optimal HES to serve the community electric demand, local cooking gas demand via hydrogen to methane gas conversion process, and the ROD demand side-by-side utilising the unconsumed EE. An experimental investigation of the hybrid system using the actual climatic and freshwater load demand data would be worth of future research. Moreover, the present study considers generic values when it comes to the maintenance issues of the RO system which may not agree with the actual scenario. For instance, the nature and degree of membrane degradation due to scaling and fouling can significantly vary affecting the permeability of the membrane. This, in addition to the productivity of the RO system in meeting the demands, may also impact the overall system economy by necessitating more frequent membrane replacement. Future research factoring a closer look into these matters will provide more acceptable outcomes. The inclusion of socio-demographic and socio-political factors like the social cost of carbon, portfolio risk, etc. in the assessment criteria is also warranted further study. The proposed system’s applicability might be limited due to the issue of waste (brine) disposal from the ROD plant. For coastal regions, like the considered case study, waste disposal is easier due to their adjacency to the ocean. However, for inland desalination systems integrated with hybrid renewable energy, a large volume of waste brine might affect the overall sustainability of the system. With 67% of the population living away from the coastal areas, studies concerning desalination systems must consider proper planning for waste disposal and minimisation in addition to other design parameters. Future efforts will factor in such aspects for a sustainable solution to energy and water issues facing the planet.
Author contribution statement
Pronob Das: Conceptualisation, Simulation execution, Methodology, Software, Writing – original draft, Writing – review & editing, Investigation. Barun K. Das: Conceptualisation, Methodology, Writing – original draft, Writing – review & editing, Investigation. M. S. Islam: Methodology, Visualisation, Writing – review & editing. Mushfiqur Rahman: Data curation, Software, Visualisation, Algorithms, Rakibul Hassan: Data curation, Software, Visualisation, Writing - review & editing. Kumar Abir Shuvo: Methodology, Writing – review & editing, Investigation.
Acknowledgement
The support from the Department of Mechanical Engineering, Rajshahi University of Engineering & Technology, Bangladesh is highly acknowledged.
Disclosure statement
No potential conflict of interest was reported by the authors.
References
- Abdelshafy, Alaaeldin M, Hamdy Hassan, and Jakub Jurasz. 2018. “Optimal Design of a Grid-Connected Desalination Plant Powered by Renewable Energy Resources Using a Hybrid PSO–GWO Approach.” Energy Conversion and Management 173: 331–347. doi:10.1016/j.enconman.2018.07.083
- Abdusamatov, Kh I. 2012. “Bicentennial Decrease of the Solar Constant Leads to the Earth’s Unbalanced Heat Budget and Deep Climate Cooling.” Kinematics and Physics of Celestial Bodies 28 (2): 62–68. doi:10.3103/S088459131202002X
- Abu-Rayash, Azzam, and Ibrahim Dincer. 2021. “Development of Integrated Sustainability Performance Indicators for Better Management of Smart Cities.” Sustainable Cities and Society 67: 102704. doi:10.1016/j.scs.2020.102704
- Alshammari, Nahar, and Johnson Asumadu. 2020. “Optimum Unit Sizing of Hybrid Renewable Energy System Utilizing Harmony Search, Jaya and Particle Swarm Optimization Algorithms.” Sustainable Cities and Society 60: 102255. doi:10.1016/j.scs.2020.102255
- Atallah, Mohamed Osman, M. A. Farahat, Mohammed Elsayed Lotfy, and Tomonobu Senjyu. 2020. “Operation of Conventional and Unconventional Energy Sources to Drive a Reverse Osmosis Desalination Plant in Sinai Peninsula, Egypt.” Renewable Energy 145: 141–152. doi:10.1016/j.renene.2019.05.138
- Balogun, Abdul-Lateef, Danny Marks, Richa Sharma, Himanshu Shekhar, Chiden Balmes, Dikman Maheng, Adnan Arshad, and Pourya Salehi. 2020. “Assessing the Potentials of Digitalization as a Tool for Climate Change Adaptation and Sustainable Development in Urban Centres.” Sustainable Cities and Society 53: 101888. doi:10.1016/j.scs.2019.101888
- Barakat, Shimaa, Haitham Ibrahim, and Adel A Elbaset. 2020. “Multi-objective Optimization of Grid-Connected PV-Wind Hybrid System Considering Reliability, Cost, and Environmental Aspects.” Sustainable Cities and Society 60: 102178. doi:10.1016/j.scs.2020.102178
- Boretti, Alberto, and Lorenzo Rosa. 2019. “Reassessing the Projections of the World Water Development Report.” NPJ Clean Water 2 (1): 1–6. doi:10.1038/s41545-018-0028-4
- Brka, Adel, Yasir M Al-Abdeli, and Ganesh Kothapalli. 2015. “The Interplay Between Renewables Penetration, Costing and Emissions in the Sizing of Stand-Alone Hydrogen Systems.” International Journal of Hydrogen Energy 40 (1): 125–135. doi:10.1016/j.ijhydene.2014.10.132
- Charrouf, O., Achour Betka, Sabrina Abdeddaim, and A. Ghamri. 2020. “Artificial Neural Network Power Manager for Hybrid PV-Wind Desalination System.” Mathematics and Computers in Simulation 167: 443–460. doi:10.1016/j.matcom.2019.09.005
- Cheng, Hui, Chundi Chen, Shengjun Wu, Zakaria A Mirza, and Zhimei Liu. 2017. “Emergy Evaluation of Cropping, Poultry Rearing, and Fish Raising Systems in the Drawdown Zone of Three Gorges Reservoir of China.” Journal of Cleaner Production 144: 559–571. doi:10.1016/j.jclepro.2016.12.053
- Clarke, Daniel P, Yasir M Al-Abdeli, and Ganesh Kothapalli. 2015. “Multi-objective Optimisation of Renewable Hybrid Energy Systems with Desalination.” Energy 88: 457–468. doi:10.1016/j.energy.2015.05.065
- Das, Barun K, Yasir M Al-Abdeli, and Ganesh Kothapalli. 2017. “Optimisation of Stand-Alone Hybrid Energy Systems Supplemented by Combustion-Based Prime Movers.” Applied Energy 196: 18–33. doi:10.1016/j.apenergy.2017.03.119
- Das, Barun K, Majed A Alotaibi, Pronob Das, M. S. Islam, Sajal K Das, and Md Alamgir Hossain. 2021a. “Feasibility and Techno-Economic Analysis of Stand-Alone and Grid-Connected PV/Wind/Diesel/Batt Hybrid Energy System: A Case Study.” Energy Strategy Reviews 37: 100673. doi:10.1016/j.esr.2021.100673
- Das, Pronob, Barun K Das, Nirendra N Mustafi, and Md Takmil Sakir. 2021e. “A Review on Pump-Hydro Storage for Renewable and Hybrid Energy Systems Applications.” Energy Storage 3 (4): e223. doi:10.1002/est2.223.
- Das, Pronob, Barun K Das, Mushfiqur Rahman, and Rakibul Hassan. 2022. “Evaluating the Prospect of Utilizing Excess Energy and Creating Employments from a Hybrid Energy System Meeting Electricity and Freshwater Demands Using Multi-Objective Evolutionary Algorithms.” Energy 238: 121860. doi:10.1016/j.energy.2021.121860
- Das, Barun K, Mahmudul Hasan, and Pronob Das. 2021b. “Impact of Storage Technologies, Temporal Resolution, and PV Tracking on Stand-Alone Hybrid Renewable Energy for an Australian Remote Area Application.” Renewable Energy 173: 362–380. doi:10.1016/j.renene.2021.03.131
- Das, Barun K, Rakibul Hassan, Mohammad Shahed HK Tushar, Forhad Zaman, Mahmudul Hasan, and Pronob Das. 2021c. “Techno-economic and Environmental Assessment of a Hybrid Renewable Energy System Using Multi-Objective Genetic Algorithm: A Case Study for Remote Island in Bangladesh.” Energy Conversion and Management 230: 113823. doi:10.1016/j.enconman.2020.113823
- Das, Barun K, Mohammad Shahed HK Tushar, and Rakibul Hassan. 2021d. “Techno-economic Optimisation of Stand-Alone Hybrid Renewable Energy Systems for Concurrently Meeting Electric and Heating Demand.” Sustainable Cities and Society 68: 102763. doi:10.1016/j.scs.2021.102763
- Delgado-Torres, Agustín M, and Lourdes García-Rodríguez. 2022. “Off-grid SeaWater Reverse Osmosis (SWRO) Desalination Driven by Hybrid Tidal Range/Solar PV Systems: Sensitivity Analysis and Criteria for Preliminary Design.” Sustainable Energy Technologies and Assessments 53: 102425. doi:10.1016/j.seta.2022.102425
- Dinka, Megersa Olumana. 2018. “Safe Drinking Water: Concepts, Benefits, Principles and Standards.” Water Challenges of an Urbanizing World, IntechOpen, London, 163–181. doi:10.5772/intechopen.68339.
- El Boujdaini, Latifa, Ahmed Mezrhab, Mohammed Amine Moussaoui, Francisco Jurado, and David Vera. 2022. “Sizing of a Stand-Alone PV–Wind–Battery–Diesel Hybrid Energy System and Optimal Combination Using a Particle Swarm Optimization Algorithm.” Electrical Engineering 104 (5): 3339–3359. doi:10.1007/s00202-022-01529-0.
- El-Houari, H., A. Allouhi, S. Rehman, M. S. Buker, T. Kousksou, A. Jamil, and B. El Amrani. 2020. “Feasibility Evaluation of a Hybrid Renewable Power Generation System for Sustainable Electricity Supply in a Moroccan Remote Site.” Journal of Cleaner Production 277: 123534. doi:10.1016/j.jclepro.2020.123534
- Elmaadawy, Khaled, Kotb M Kotb, M. R. Elkadeem, Swellam W Sharshir, András Dán, Ahmed Moawad, and Bingchuan Liu. 2020. “Optimal Sizing and Techno-Enviro-Economic Feasibility Assessment of Large-Scale Reverse Osmosis Desalination Powered with Hybrid Renewable Energy Sources.” Energy Conversion and Management 224: 113377. doi:10.1016/j.enconman.2020.113377
- Fathollahzadeh, Mohammad Hassan, Andrew Speake, Paulo Cesar Tabares-Velasco, Zoheir Khademian, and Lisa Lone Fight. 2021. “Renewable Energy Analysis in Indigenous Communities Using Bottom-up Demand Prediction.” Sustainable Cities and Society 71: 102932. doi:10.1016/j.scs.2021.102932
- Feizizadeh, Bakhtiar, Zahra Ronagh, Samreh Pourmoradian, Hassan Abedi Gheshlaghi, Tobia Lakes, and Thomas Blaschke. 2021. “An Efficient GIS-Based Approach for Sustainability Assessment of Urban Drinking Water Consumption Patterns: A Study in Tabriz City, Iran.” Sustainable Cities and Society 64: 102584. doi:10.1016/j.scs.2020.102584
- Ghaithan, Ahmed M, Awsan Mohammed, Ahmad Al-Hanbali, Ahmed M Attia, and Haitham Saleh. 2022. “Multi-objective Optimization of a Photovoltaic-Wind-Grid Connected System to Power Reverse Osmosis Desalination Plant.” Energy 251: 123888. doi:10.1016/j.energy.2022.123888
- Ghenai, Chaouki, Adel Merabet, Tareq Salameh, and Erola Colon Pigem. 2018. “Grid-tied and Stand-Alone Hybrid Solar Power System for Desalination Plant.” Desalination 435: 172–180. doi:10.1016/j.desal.2017.10.044
- Gökçek, Murat. 2018. “Integration of Hybrid Power (Wind-Photovoltaic-Diesel-Battery) and Seawater Reverse Osmosis Systems for Small-Scale Desalination Applications.” Desalination 435: 210–220. doi:10.1016/j.desal.2017.07.006
- Gtari, Oumayma, Mohamed Hamdi, and Majdi Hazami. 2022. “Analysis of an Uncovered Photovoltaic/Thermal Solar System Powering a Seawater Reverse Osmosis Desalination Plant.” Environmental Progress & Sustainable Energy 41 (3): e13753. doi:10.1002/ep.13753.
- Hajibabaei, Mohsen, Sara Nazif, and Fatemeh Tavanaei Sereshgi. 2018. “Life Cycle Assessment of Pipes and Piping Process in Drinking Water Distribution Networks to Reduce Environmental Impact.” Sustainable Cities and Society 43: 538–549. doi:10.1016/j.scs.2018.09.014
- Halabi, Laith M, and Saad Mekhilef. 2018. “Flexible Hybrid Renewable Energy System Design for a Typical Remote Village Located in Tropical Climate.” Journal of Cleaner Production 177: 908–924. doi:10.1016/j.jclepro.2017.12.248
- Halkos, George, and Antonis Skouloudis. 2018. “Corporate Social Responsibility and Innovative Capacity: Intersection in a Macro-Level Perspective.” Journal of Cleaner Production 182: 291–300. doi:10.1016/j.jclepro.2018.02.022
- Hassan, Ahmed, Magdi El-Saadawi, Mahmoud Kandil, and Mohammed Saeed. 2016. “Modeling and Optimization of a Hybrid Power System Supplying RO Water Desalination Plant Considering CO2 Emissions.” Desalination and Water Treatment 57 (26): 11972–11987. doi:10.1080/19443994.2015.1046148
- Hemeida, Ashraf Mohamed, Ahmed Shaban Omer, Ayman M Bahaa-Eldin, Salem Alkhalaf, Mahrous Ahmed, Tomnobu Senjyu, and Gaber El-Saady. 2022. “Multi-objective Multi-Verse Optimization of Renewable Energy Sources-Based Micro-Grid System: Real Case.” Ain Shams Engineering Journal 13 (1): 101543. doi:10.1016/j.asej.2021.06.028
- Hossam-Eldin, A., A. M. El-Nashar, and A. Ismaiel. 2012. “Investigation Into Economical Desalination Using Optimized Hybrid Renewable Energy System.” International Journal of Electrical Power & Energy Systems 43 (1): 1393–1400. doi:10.1016/j.ijepes.2012.05.019
- Huang, Zhiyu, Zhilong Xie, Caizhi Zhang, Siew Hwa Chan, Jarosław Milewski, Yi Xie, Yalian Yang, and Xiaosong Hu. 2019. “Modeling and Multi-Objective Optimization of a Stand-Alone PV-Hydrogen-Retired EV Battery Hybrid Energy System.” Energy Conversion and Management 181: 80–92. doi:10.1016/j.enconman.2018.11.079
- Ibrahim, Mostafa M, Nabil H Mostafa, Ahmed H Osman, and Ahmed Hesham. 2020. “Performance Analysis of a Stand-Alone Hybrid Energy System for Desalination Unit in Egypt.” Energy Conversion and Management 215: 112941. doi:10.1016/j.enconman.2020.112941
- Islam, M. S., Barun K Das, Pronob Das, and Md Habibur Rahaman. 2021. “Techno-economic Optimization of a Zero Emission Energy System for a Coastal Community in Newfoundland, Canada.” Energy 220: 119709. doi:10.1016/j.energy.2020.119709
- Jahannoosh, Mariye, Saber Arabi Nowdeh, Amirreza Naderipour, Hesam Kamyab, Iraj Faraji Davoudkhani, and Jiří Jaromír Klemeš. 2020. “New Hybrid Meta-Heuristic Algorithm for Reliable and Cost-Effective Designing of Photovoltaic/Wind/Fuel Cell Energy System Considering Load Interruption Probability.” Journal of Cleaner Production 278: 123406. doi:10.1016/j.jclepro.2020.123406
- Kharrich, Mohammed, Omar Hazem Mohammed, Nahar Alshammari, and Mohammed Akherraz. 2021. “Multi-objective Optimization and the Effect of the Economic Factors on the Design of the Microgrid Hybrid System.” Sustainable Cities and Society 65: 102646. doi:10.1016/j.scs.2020.102646
- Kotb, Kotb M, M. R. Elkadeem, Ahmed Khalil, Sherif M Imam, Mohamed A Hamada, Swellam W Sharshir, and András Dán. 2021. “A Fuzzy Decision-Making Model for Optimal Design of Solar, Wind, Diesel-Based RO Desalination Integrating Flow-Battery and Pumped-Hydro Storage: Case Study in Baltim, Egypt.” Energy Conversion and Management 235: 113962. doi:10.1016/j.enconman.2021.113962
- Kyriakarakos, George, Anastasios I Dounis, Konstantinos G Arvanitis, and George Papadakis. 2017. “Design of a Fuzzy Cognitive Maps Variable-Load Energy Management System for Autonomous PV-Reverse Osmosis Desalination Systems: A Simulation Survey.” Applied Energy 187: 575–584. doi:10.1016/j.apenergy.2016.11.077
- Lilas, Theodoros, Ioannis Dagkinis, Afrokomi-Afroula Stefanakou, Evanthia Antoniou, Nikitas Nikitakos, Artemis Maglara, and Athanasios Vatistas. 2022. “Energy Utilisation Strategy in an Offshore Floating Wind System with Variable Production of Fresh Water and Hybrid Energy Storage.” International Journal of Sustainable Energy 41 (10): 1572–1590. doi:10.1080/14786451.2022.2067160
- Liu, Hao, Baojia Wu, and Akbar Maleki. 2022. “Effects of Dispatch Strategies on Optimum Sizing of Solar-Diesel-Battery Energy Storage-RO Desalination Hybrid Scheme by Efficient Heuristic Algorithm.” Journal of Energy Storage 54: 104862. doi:10.1016/j.est.2022.104862
- Luo, Xi, Jinwen Xia, and Yanfeng Liu. 2021. “Extraction of Dynamic Operation Strategy for Standalone Solar-Based Multi-Energy Systems: A Method Based on Decision Tree Algorithm.” Sustainable Cities and Society 70: 102917. doi:10.1016/j.scs.2021.102917
- Makkeh, Seyed Ali, Abolfazl Ahmadi, Farbod Esmaeilion, and M. A. Ehyaei. 2020. “Energy, Exergy and Exergoeconomic Optimization of a Cogeneration System Integrated with Parabolic Trough Collector-Wind Turbine with Desalination.” Journal of Cleaner Production 273: 123122. doi:10.1016/j.jclepro.2020.123122
- Maleki, Akbar. 2018. “Design and Optimization of Autonomous Solar-Wind-Reverse Osmosis Desalination Systems Coupling Battery and Hydrogen Energy Storage by an Improved bee Algorithm.” Desalination 435: 221–234. doi:10.1016/j.desal.2017.05.034
- Mandal, Soumya, Barun K Das, and Najmul Hoque. 2018. “Optimum Sizing of a Stand-Alone Hybrid Energy System for Rural Electrification in Bangladesh.” Journal of Cleaner Production 200: 12–27. doi:10.1016/j.jclepro.2018.07.257
- Mehrjerdi, Hasan. 2020. “Modeling and Optimization of an Island Water-Energy Nexus Powered by a Hybrid Solar-Wind Renewable System.” Energy 117217. doi:10.1016/j.energy.2020.117217
- Mirjalili, Seyedali, Amir H Gandomi, Seyedeh Zahra Mirjalili, Shahrzad Saremi, Hossam Faris, and Seyed Mohammad Mirjalili. 2017. “Salp Swarm Algorithm: A bio-Inspired Optimizer for Engineering Design Problems.” Advances in Engineering Software 114: 163–191. doi:10.1016/j.advengsoft.2017.07.002
- Mokheimer, Esmail MA, Ahmet Z Sahin, Abdullah Al-Sharafi, and Ahmad I Ali. 2013. “Modeling and Optimization of Hybrid Wind–Solar-Powered Reverse Osmosis Water Desalination System in Saudi Arabia.” Energy Conversion and Management 75: 86–97. doi:10.1016/j.enconman.2013.06.002
- Mousavi, Seyed Ali, Ruhollah Asayesh Zarchi, Fatemeh Razi Astaraei, Roghayeh Ghasempour, and Farshid Mohammad Khaninezhad. 2020. “Decision-making Between Renewable Energy Configurations and Grid Extension to Simultaneously Supply Electrical Power and Fresh Water in Remote Villages for Five Different Climate Zones.” Journal of Cleaner Production 279: 123617. doi:10.1016/j.jclepro.2020.123617
- Movahediyan, Zahra, and Alireza Askarzadeh. 2018. “Multi-objective Optimization Framework of a Photovoltaic-Diesel Generator Hybrid Energy System Considering Operating Reserve.” Sustainable Cities and Society 41: 1–12. doi:10.1016/j.scs.2018.05.002
- Najjar, Elena, Mahmoud Al-Hindi, May Massoud, and Walid Saad. 2022. “Life Cycle Assessment and Cost of a Seawater Reverse Osmosis Plant Operated with Different Energy Sources.” Energy Conversion and Management 268: 115964. doi:10.1016/j.enconman.2022.115964
- Nyeche, E. N., and E. O. Diemuodeke. 2020. “Modelling and Optimisation of a Hybrid PV-Wind Turbine-Pumped Hydro Storage Energy System for Mini-Grid Application in Coastline Communities.” Journal of Cleaner Production 250: 119578. doi:10.1016/j.jclepro.2019.119578
- Padrón, Isidro, Deivis Avila, Graciliano N Marichal, and José A Rodríguez. 2019. “Assessment of Hybrid Renewable Energy Systems to Supplied Energy to Autonomous Desalination Systems in two Islands of the Canary Archipelago.” Renewable and Sustainable Energy Reviews 101: 221–230. doi:10.1016/j.rser.2018.11.009
- Peng, Wanxi, Akbar Maleki, Marc A Rosen, and Pouria Azarikhah. 2018. “Optimization of a Hybrid System for Solar-Wind-Based Water Desalination by Reverse Osmosis: Comparison of Approaches.” Desalination 442: 16–31. doi:10.1016/j.desal.2018.03.021
- Rahman, M Tauhid Ur, Md Rasheduzzaman, Md Arman Habib, Afzal Ahmed, Syed Mohammed Tareq, and Shah Md Muniruzzaman. 2017. “Assessment of Fresh Water Security in Coastal Bangladesh: An Insight from Salinity, Community Perception and Adaptation.” Ocean & Coastal Management 137: 68–81. doi:10.1016/j.ocecoaman.2016.12.005
- Roy, Dibyendu, Rakibul Hassan, and Barun K Das. 2022. “A Hybrid Renewable-Based Solution to Electricity and Freshwater Problems in the off-Grid Sundarbans Region of India: Optimum Sizing and Socio-Enviro-Economic Evaluation.” Journal of Cleaner Production 372: 133761. doi:10.1016/j.jclepro.2022.133761
- Samy, M. M., Shimaa Barakat, and H. S. Ramadan. 2019. “A Flower Pollination Optimization Algorithm for an off-Grid PV-Fuel Cell Hybrid Renewable System.” international Journal of Hydrogen Energy 44 (4): 2141–2152. doi:10.1016/j.ijhydene.2018.05.127
- Sanajaoba, Sarangthem, and Eugene Fernandez. 2016. “Maiden Application of Cuckoo Search Algorithm for Optimal Sizing of a Remote Hybrid Renewable Energy System.” Renewable Energy 96: 1–10. doi:10.1016/j.renene.2016.04.069
- Sanaye, S., and A. Sarrafi. 2021. “Cleaner Production of Combined Cooling, Heating, Power and Water for Isolated Buildings with an Innovative Hybrid (Solar, Wind and LPG Fuel) System.” Journal of Cleaner Production 279: 123222. doi:10.1016/j.jclepro.2020.123222
- Santosh, R., T. Arunkumar, R. Velraj, and G. Kumaresan. 2019. “Technological Advancements in Solar Energy Driven Humidification-Dehumidification Desalination Systems-A Review.” Journal of Cleaner Production 207: 826–845. doi:10.1016/j.jclepro.2018.09.247
- Sawle, Yashwant, S. C. Gupta, and Aashish Kumar Bohre. 2018. “Review of Hybrid Renewable Energy Systems with Comparative Analysis of off-Grid Hybrid System.” Renewable and Sustainable Energy Reviews 81: 2217–2235. doi:10.1016/j.rser.2017.06.033
- Shafaghat, A. H., M. Eslami, and M. Baneshi. 2023. “Techno-enviro-economic Study of a Reverse Osmosis Desalination System Equipped with Photovoltaic-Thermal Collectors.” Applied Thermal Engineering 218: 119289. doi:10.1016/j.applthermaleng.2022.119289
- Sharif, Arshian, Ozge Baris-Tuzemen, Gizem Uzuner, Ilhan Ozturk, and Avik Sinha. 2020. “Revisiting the Role of Renewable and non-Renewable Energy Consumption on Turkey’s Ecological Footprint: Evidence from Quantile ARDL Approach.” Sustainable Cities and Society 57: 102138. doi:10.1016/j.scs.2020.102138
- Shezan, S. K. A., S. Julai, M. A. Kibria, K. R. Ullah, R. Saidur, W. T. Chong, and R. K. Akikur. 2016. “Performance Analysis of an off-Grid Wind-PV (Photovoltaic)-Diesel-Battery Hybrid Energy System Feasible for Remote Areas.” Journal of Cleaner Production 125: 121–132. doi:10.1016/j.jclepro.2016.03.014
- Wang, Jiangjiang, Xiaoling Qi, Fukang Ren, Guoqing Zhang, and Jiahao Wang. 2021. “Optimal Design of Hybrid Combined Cooling, Heating and Power Systems Considering the Uncertainties of Load Demands and Renewable Energy Sources.” Journal of Cleaner Production 281: 125357. doi:10.1016/j.jclepro.2020.125357
- Wang, Xue, Huixian Wang, Yuanming Wang, Jian Gao, Jindun Liu, and Yatao Zhang. 2019. “Hydrotalcite/Graphene Oxide Hybrid Nanosheets Functionalized Nanofiltration Membrane for Desalination.” Desalination 451: 209–218. doi:10.1016/j.desal.2017.05.012
- World Health Organization. 2017. Progress on drinking water, sanitation and hygiene: 2017 update and SDG baselines.”.
- Wu, Baojia, Akbar Maleki, Fathollah Pourfayaz, and Marc A Rosen. 2018. “Optimal Design of Stand-Alone Reverse Osmosis Desalination Driven by a Photovoltaic and Diesel Generator Hybrid System.” Solar Energy 163: 91–103. doi:10.1016/j.solener.2018.01.016
- Yuan, Wenlin, Zhe Liu, Chengguo Su, and Xinqi Wang. 2020. “Photovoltaic Capacity Optimization of Small and Medium-Sized Hydro-Photovoltaic Hybrid Energy Systems Considering Multiple Uncertainties.” Journal of Cleaner Production 276: 124170. doi:10.1016/j.jclepro.2020.124170
- Zaman, Forhad, Saber M Elsayed, Ruhul Sarker, Daryl Essam, and Carlos A Coello. 2019. “Multi-method Based Algorithm for Multi-Objective Problems Under Uncertainty.” Information Sciences 481: 81–109. doi:10.1016/j.ins.2018.12.072
- Zebra, Emília Inês Come, Henny J van der Windt, Geraldo Nhumaio, and André PC Faaij. 2021. “A Review of Hybrid Renewable Energy Systems in Mini-Grids for off-Grid Electrification in Developing Countries.” Renewable and Sustainable Energy Reviews 144: 111036. doi:10.1016/j.rser.2021.111036
- Zhang, Yusheng, Jijian Lian, Chao Ma, Yang Yang, Xiulan Pang, and Lun Wang. 2020. “Optimal Sizing of the Grid-Connected Hybrid System Integrating Hydropower, Photovoltaic, and Wind Considering Cascade Reservoir Connection and Photovoltaic-Wind Complementarity.” Journal of Cleaner Production 274: 123100. doi:10.1016/j.jclepro.2020.123100
- Zhang, Weiping, Akbar Maleki, Marc A Rosen, and Jingqing Liu. 2019. “Sizing a Stand-Alone Solar-Wind-Hydrogen Energy System Using Weather Forecasting and a Hybrid Search Optimization Algorithm.” Energy Conversion and Management 180: 609–621. doi:10.1016/j.enconman.2018.08.102
- Zhang, Guozhao, Baojia Wu, Akbar Maleki, and Weiping Zhang. 2018. “Simulated Annealing-Chaotic Search Algorithm Based Optimization of Reverse Osmosis Hybrid Desalination System Driven by Wind and Solar Energies.” Solar Energy 173: 964–975. doi:10.1016/j.solener.2018.07.094
- Zhao, Pan, Feifei Gou, Wenpan Xu, Jiangfeng Wang, and Yiping Dai. 2022. “Multi-objective Optimization of a Renewable Power Supply System with Underwater Compressed air Energy Storage for Seawater Reverse Osmosis Under two Different Operation Schemes.” Renewable Energy 181: 71–90. doi:10.1016/j.renene.2021.09.041
- Zhao, Pan, Shiqiang Zhang, Feifei Gou, Wenpan Xu, Jiangfeng Wang, and Yiping Dai. 2021. “The Feasibility Survey of an Autonomous Renewable Seawater Reverse Osmosis System with Underwater Compressed air Energy Storage.” Desalination 505: 114981. doi:10.1016/j.desal.2021.114981