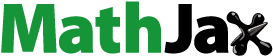
ABSTRACT
Geothermal energy is a renewable energy that has not been utilised as solar, wind, and biomass energy because of the cost of exploration, cost of implementation, and possible environmental concerns such as enhancing the chances of earthquakes, and tremors.The remote sensing technique and Surface Energy Balance model was proposed to determine the absorbed terrestrial radiation and other salient parameters. Forty years remote sensing dataset (1980–2019), was obtained from the MERRA-2 for three hundred and two (302) locations across Nigeria. The acquired thermal parameters were processed using known models. The heat catchment with the highest magnitude of 330.244 W/m2 are found in Nembe, Okirika, Yenagoa (Niger-Delta basin); 334.198 W/m2 in Ozubulu, Oguta, Obolo (Anambra basin); 332.877 W/m2 in Okitipupa, Atijere (Dahomey basin); 318.021 W/m2 in Eban, Mokwa (Bida basin); 318.682 W/m2 in Kumaganum, Gashua, Geidam (Chad basin); 321.050 W/m2 in Kuchi, Gwandi, Gwadabawa (Sokoto basin); 289.247 W/m2 in Gombe, Potiskum, Kafarati (Upper Benue basin); 312.964 W/m2 in Lokoja, Otukpa (Mid-Benue basin); and 338.962 W/m2 Malumfashi, Kano (Basement Complex). Likely geothermal wells were listed within the research. It is recommended that more ground measurements should be carried out in the basement complexes and sedimentary basins of Nigeria.
1. Introduction
Globally, as the human population grows, so does the demand for energy, a necessity for human development and sustainability, resulting in increased productivity and economic growth. For many years, fossil fuel has been the primary energy source in the world, particularly in Nigeria, and is usually used to generate electricity and transport people. The burning of fossil fuels emits greenhouse gases that have adverse effects such as climate change, global warming, acid rain, environmental concerns, and health complications to humans, animals, and plants, all of which are anthropogenic pollution (Emetere et al. Citation2016). This problem has led policymakers, intergovernmental agencies, interested parties, and the world at large to look forward to a sustainable future. This development has presented an opportunity in recent years to replace the hydrocarbons used in fossil fuel-based energy sources with a cleaner, eco-friendly, and sustainable energy source known as renewable energy (Oludamilare et al. Citation2020). Nigeria unquestionably has untapped renewable energy resources, but a lack of information on its unexploited potential, willing private sector sponsors, and widespread exploration within the country’s southern region create an undesirable barrier (Kehinde et al. Citation2018).
Nigeria is among the few countries endowed with geothermal resources, with several surface manifestations such as warm springs (Adedayo, Adio, and Oboirien Citation2021). The Ikogosi Warm Springs in Ekiti State and the Wikki Warm Springs in Bauchi State are the two most common geothermal locations in Nigeria (Elehinafe et al. Citation2021a). Also, the presence of volcanic rock, which is part of geothermal features (Ema et al. Citation2019), is found in various parts of the country. For example, Suleiman, Okeke, and Obiora (Citation2021) identified the presence of volcanic rocks and notable geothermal manifestations in the Sokoto basin in the Northwestern part of Nigeria. Numerous factors influence the process, development, and utilisation of geothermal resources, all of which must be considered when forecasting potential geothermal locations (Meng et al. Citation2021). Several criteria and conditions must be met for geothermal energy to exist and be exploited in a region (Wang et al. Citation2018). Recent research has demonstrated that geothermal energy sources are possible in regions underlain by basement rocks, including metamorphic and igneous rocks formed in the earth’s interior (Usman et al. Citation2019). Additionally, the discovery and use of Nigeria’s geothermal resources are possible due to the prevalence of their potential, particularly in the Cretaceous sedimentary basins and certain sections of the basement complex terrains. For example, the deduced geothermal gradient within the Niger Delta basin shows a considerable geothermal potential (Tende, Aminu, and Gajere Citation2021). A comprehensive geothermal survey must be carried out to identify prospective well locations for geothermal energy optimisation in areas where geothermal hot springs lack a natural outflow or are absent (Jiang et al. Citation2022). Exploration for these resources must be successful in making a switch to geothermal energy effectively. From existing and operational geothermal wells around the globe, the installed capacity of geothermal electricity worldwide in 2014 was 12,635 MW, with the United States leading with a capacity of 3450 MW and an operating capacity of 2542 MW (Amoo Citation2019). Geothermal energy is a clean and renewable energy source produced from the earth’s internal heat content, which is around 1031 Joules higher than the world’s energy usage.
Geothermal energy is a form of thermal energy produced and preserved in the earth due to radioactive decay and constant heat dissipation during the earth’s formation. The temperature variation between the earth’s core and its surface causes a continual movement of heat from the centre to the earth’s surface. The temperature at the core-mantle border is as high as 4000oC, which can heat rocks and water in the crust to about 370oC (Kehinde et al. Citation2018). The earth’s surface water circulates to the subsurface, where heating takes place, before returning to the earth’s surface through an underground ‘plumb system’ of clustered cracks or permeable rock zones, and this increase in the earth’s heat is usually moved to the earth’s surface by advection of water from the ground to form hydrothermal systems. The physics of this process is vital and can be resolved using the downward terrestrial radiation from the Surface Energy Balanced (SEB) model. This is the main idea of this work: to accurately predict prospective geothermal locations using remote sensing techniques. One of the advantages of the remote sensing techniques is their ability to sense the geothermal energy system, which is composed of three basic parts: a heat source, a reservoir, and a working liquid or gas that transfers heat from the source to the geothermal feature (Jiang et al. Citation2022). When rising fluids with elevated temperatures reach the surface, geothermal manifestations including hot springs, fumaroles, geysers, and mud spots can be visible (Keam, Katherine, and Leonie Citation2005). The idea of downward terrestrial radiation, which is not expected to be seasonal as the rising fluids are initiated from the earth’s crust and not the sun.
Aided methods that are used to enhance remote sensing techniques are the basis for drawing certain conclusions. For example, Fanao et al. (Citation2021) used an analytical method and the entropy weight method to map each criterion and calculate each weight, and the geothermal potential area was determined using a multi-criteria decision analysis method that depends on a geographic information system. Suleiman, Okeke, and Obiora (Citation2021) postulated that Curie point depth, geothermal gradient, and heat flow calculations give added information to geothermal exploration. For example, they reported that the Sokoto basin’s northeast (Gandi and Rabah) and northwest (Argungu) have enormous geothermal potential due to deeper Curie points, steep geothermal gradients, and significant heat flow. According to Emujakporue and Ekine (Citation2014), the computed geothermal gradient is from 17.30.14 to 24.330.70°C/km, with a mean of 22.240.57°C/km, while the heat flow ranges from 38.060.31 to 56.392.41 Wm2, with an average of 50.151.25 Wm2. Geological structures such as growth faults and rollover anticlines and fluid movement in the subsurface are all plausible sources of geothermal anomalies. Low sedimentary thickness was linked to locations with high heat flow, while high sedimentary thickness was linked to areas with low heat flow. Abuzied et al. (Citation2020) also postulated the use of a heat flow map for determining geothermal sites in the Gulf of Suez coastal area, which had heat flow zones of 67–200 Wm−2.
Remote sensing gathers information about a specific area using satellites and/or airborne sensors that are not in direct contact with any item. Both passive and active methods are possible. Various sensors are employed in passive approaches to detect natural radiation emitted or reflected by the object or area being examined. Energy is emitted in active remote sensing, and the signal that is reflected back by the object is measured (Vaughen et al. Citation2010). Remote sensing is utilised in oil and gas exploration as well as the discovery of geothermal resources. Remote sensing is used to conduct a preliminary investigation of geothermal potential, such as hydrothermally changed minerals like sinter and tuff, in potentially geothermal locations (Calvin, Littlefield, and Kratt Citation2015). Photographic and thermal infrared sensors can only measure depths of a few microns to millimetres below the surface (Manan et al. Citation2015). Thermal infrared remote sensing (RS) and the resultant land surface temperature can be used to map thermal anomalies and calculate geothermal heat flux in geothermal potential areas (Haselwimmer, Prakash, and Holdmann Citation2013). Over the last two decades, significant improvements in wavelength coverage, spectral resolution, and image quality have led to the development of near-infrared and thermal-infrared imagery tools such as the Advanced Spaceborne Thermal Emission and Reflection Radiometer and the Moderate-resolution Imaging Spectroradiometer (Manan et al. Citation2015).
The geological structure of Nigeria is comprised of Proterozoic to Lower Palaeozoic metamorphic and igneous rocks; the tertiary to recent volcanic rocks make up the Basement Complex. In the southwestern part of the country, schist belts (metasediments and metavolcanics) dominate the basement. The Benue Trough, which is part of a mega-rift system often called the West and Central Africa Rift System, is made up of 6000 metres of Cretaceous sediments coupled with some volcanic activity (Obaje Citation2009). The Santonian tectonic episode of compressional folding had an impact on the Benue Trough and was fairly severe, resulting in approximately 100 synclinal folds and anticlinal folds. The Afikpo synclinal fold and the Abakaliki anticlinal fold are two distinct deformations in the southern Benue Trough. In terms of geography, the Benue Trough is split into three: lower (southern), middle (central), and upper (northern). The southern Benue Trough comprises the Niger Delta-Benin Embayment, the Anambra basin, the Abakaliki fold (Abakaliki Anticlinorium), the Afikpo syncline, and the Calabar flank, which are all parts of the Sedimentary basin in southern parts of Nigeria. The region has a variety of fault patterns, with the NE–SW direction being the most prominent. The southern Benue Trough and the surrounding Cameroonian volcanics contain Upper Cretaceous to Tertiary volcanics (Obaje Citation2009).
Due to the geology of Nigeria and its reduced environmental disasters such as earthquakes, tremors, etc., there is a call for the adaptation of geothermal energy as the current realities of climate change are affecting its main source of energy generation (hydrothermal energy). These issues underline the need to examine all conceivable energy resources to create a new energy future for Nigeria. Most research conducted on geothermal energy prospects in Nigeria considered specific geographical sections, which may already be known by common geological features such as warm springs. Due to the geological features of Nigeria and the direct and indirect tectonic activities, there is a need to map the thermal parameters over Nigeria to determine likely geothermal wells and ground heat catchment locations in Nigeria. This research aims to investigate potential locations of geothermal wells and ground heat catchment over Nigeria with the specific objectives being to: evaluate the thermal parameters of the study location using remote sensing; estimate the terrestrial radiation over the study area using spatial maps; evaluate the statistical significance of the thermal parameters; and validate the emerging theories obtained via remote sensing measurements for ground measurements at selected locations.
2. Theory of study
The SEB model is somewhat interesting as it is employed to introduce an ideal assimilating atmosphere because the earth’s atmosphere assimilates the majority of terrestrial radiation released from the earth’s surface (Pierrehumbert Citation2011; Trenberth, Fasullo, and Kiehl Citation2009). The energy equilibrium of a surface layer with a unit horizontal area and a finite depth (the surface energy balance) can be expressed as
(1)
(1) Q is the total amount of heat energy that is held in the surface layer, Rn is the net radiation from the surface, and G is the ground heat flux. This is the energy lost via the lower boundary due to heat conduction. When it is directed into the ground and away from the surface, it is a positive number. Sensible Heat Flux is denoted by H. It symbolises the energy that is lost from the surface via heat movement to the atmosphere. When it is propelled upward and away from the surface, it is positive. λE is the flux of latent heat. It depicts the evaporation-induced energy lost at the surface.
The net radiation for land contains a mixture of shortwave and longwave fluxes (Arizona edu Citation2022), Radiation Fluxes
(2)
(2) where
is the surface shortwave radiative flux (positive), Rd downward longwave flux (positive), and
is the surface upward longwave flux L0 (negative),
is the shortwave albedo, and
is the surface longwave emissivity (directly proportional to the absorption), that is the percentage of incoming long-wave radiation that the surface is able to absorb. If the surface acts as a black body, then
= 1. The radiation flux emitted by a black body is given by the Stefan Boltzmann law as σT4 where σ = 5.67 × 10−8 W m−2 K−4 is the Stefan–Boltzmann constant. Almost all natural surfaces are grey than black, they radiate a longwave radiative flux of magnitude
T4 where
is less than unity.
The phenomena of terrestrial radiation validate the Stefan–Boltzmann equation, which asserts that the total energy flow F in units of watts per square metre (W m−2) emitted from an item/object is directly proportional to the absolute temperature T in units of Kelvin (K) to the power four of the object
(3)
(3) The Stefan Boltzmann constant is σ = 5.67 × 10−8 W m−2 K−4, ϵ = the emissivity (0 < ϵ < 1), a characteristic constant for the material that permits variations from the ideal blackbody behaviour (for which ϵ = 1). For ϵ = 1, F = the area under the Planck curve.
In calculating the geothermal gradient, Fourier’s law is an essential element in its estimation. Fourier’s law gives the essential relationship for conductive heat transport. (Tanaka, Okubo, and Matsubayashi Citation1999). It states that a temperature differential (ΔT) between various portions of a geologic system causes heat flux (Watt per unit area of cross-section) and that it is directly related to a material quality termed thermal conductivity (J s1 m1 K1).
The capability of rocks to carry heat is depicted by their thermal conductivity. It varies greatly depending on the type of rock. Crystalline basement rocks like granites and gneisses conduct heat two to three times more conductive than the unconsolidated material (gravel, sand). Under the premise that the thermal variation is vertical and the thermal gradient dT/dz is constant in a one-dimensional situation, Fourier’s law is given by:
(4)
(4) where q is the heat flux, and k is the coefficient of thermal conductivity.
Tanaka, Okubo, and Matsubayashi (Citation1999) found that the following equation could be used to get the Curie temperature (θ) given the Curie point depth zb and the thermal gradient dT/dz using the following equation:
(5)
(5) The dT/dz is constant.
(6)
(6) The ‘Bare Rock’ Energy Balance Model is employed to introduce an ideal assimilating atmosphere because the Earth’s atmosphere assimilates the majority of terrestrial radiation released from the Earth’s surface (Pierrehumbert Citation2011). Absorbed Solar radiation is the energy input while the output is the emitted terrestrial radiation (ETR). The emission temperature of the planet is determined by energy equilibrium at the top of the atmosphere in the ‘Bare Rock’ model, which is currently understood to be the atmospheric temperature Ta = 255 K. Since one ETRa travels downward and one upward atmosphere (two times 2ETRa = 2σTa4), the theory underlying this model implies that, to attain balance, the summation of terrestrial radiation emitted from the atmosphere is directly proportional the radiation assimilated from the surface (ETRs = σTs4) (Trenberth, Fasullo, and Kiehl Citation2009).
(7)
(7)
(8)
(8) where Stefan Boltzmann constant is σ is 5.67 × 10−8 W m−2 K−4, absorbed surface radiation temperature Ts is 21/4, emitted terrestrial radiation Ta is 303 K, total emitted terrestrial radiation from the atmosphere ETRa absorbed radiation coming from the surface ETRs.
3. Methodology
This study used four main methods as presented in the flowchart. The methods include data acquisition via remote sensing, ground measurement, spatial analysis, statistical investigation, and the estimation of thermal terrestrial radiation using existing models ((a)). The materials used in this study are based on their mode of exploration. A computer system was used to download and process the data. The MATrix LABoratory (MATLAB), Statistical Package for Social Sciences (SPSS), and Quantum Geographic Information System (QGIS) were the softwares used for the study. The evaluation of the terrestrial radiation was carried out using Equations (1)–(6) to fulfill objective one of the research works. The spatial analysis of the terrestrial radiation over the research area was performed to fulfill the second objective of the research work. The statistical significance was ascertained to fulfill the third objective while the ground measurement and remote sensing of selected locations were carried out to fulfill the fourth objective of the research work.
MATLAB was used for data analysis of over 150,240 files, developing algorithms, and estimating parameters from existing models. SPSS was used to statistically analyse different types of thermal parameters with sectionalised regions of the study location. QGIS was used to perform construct, export graphical maps, analyse, and generate geospatial information. The materials that were used for the ground measurement include global positioning systems device, thermal logger, and image logger. The thermal logger collects information about the temperature, humidity, pressure, gas concentration, and depth of selected boreholes. This device was used in the study area to take the ground measurement of thirteen wells.
The thermal parameters of 40 years between 1980 and 2019 for 302 locations across Nigeria ((b)) used for this research were obtained MERRA-2. The thermal parameters from the satellite measurement include; Ground heat land, latent heat flux land, sensible heat flux land, net longwave land, net shortwave land, rate of change of total land energy, soil temperature layer 1, soil temperature layer 2, soil temperature layer 3, soil temperature layer 4, soil temperature layer 5, soil temperature layer 6, the surface temperature of saturated zone, surface temperature of unsaturated zone, total energy storage land.
The various tests carried out for the statistical analysis are the normality test, univariate outliner test, and correlation test. The normality test is performed to ascertain if the data is a norm and subsequently to ascertain whether the average is a representational number of the data. This is the precondition for statistical tests because normalised data is an integrative theory in parametric testing. Some of the most popular techniques for examining the norm of continual data include the Shapiro–Wilk test, Kolmogorov–Smirnov test, skewness, kurtosis, histogram, box plot, P–P plot, Q–Q plot, and mean with standard deviation. The two procedures that are most frequently used to check the normality of the data are the Kolmogorov–Smirnov test and the Shapiro–Wilk test. Kolmogorov–Smirnov test is used for n ≥ 50 sample sizes, whereas Shapiro–Wilk test is used for small sample sizes <50 (Gupta et al. Citation2019).
4. Results and discussion
The highest parameter of the terrestrial radiation for DJF (December, January, and February), MAM (March, April, and May), JJA (June, July, and August), SON (September, October, and November), and Year are documented in . Also, the highest value of the geothermal gradient was discussed in the listed table.
In , the notable heat catchment in the Niger Delta basin is Okirika, Yenagoa, and Nembe as corroborated by the isothermal map presented by Onuoha and Ekine (Citation1999) which indicates that the thermal property increases towards the coastal swamp of the basin. In , the heat catchment area of the Anambra basin is in the Ozubulu, Oguta, and Obolo areas of the basin which corroborates with the temperature variation of wells in the southwestern part of this basin presented by Onuoha and Ekine (Citation1999). In , the notable heat catchment in the Niger Delta basin is Okitipupa and Atijere as corroborated by the isothermal map presented. In , the heat catchment area of the Bida basin is in the Eban and Mokwa areas of the basin which corroborates with the subsurface thermal flow in the basin that trends in the NE–SW trending direction with values above 94 m W m−2 similar to the faults system in the basin as reported by Nwankwo and Sunday (Citation2017). In , the heat catchment area of the Chad basin is in the Kumaganum, Gashua, and Geidam areas of the basin which corroborates with the highest heat variations in the south of Bama and Gashua areas as reported by Kwaya, Kurowska, and Arabi (Citation2016). In , the heat catchment area of the Sokoto basin is in the Kuchi, Gwandi, and Gwadabawa areas of the basin which corroborates with the subsurface thermal flow in the NE–SW direction and also, the increase from the south towards the north with values above 100 m W m−2 observed in the north-central portion because of its deep magmatic mass that has not completed its cooling in association with young volcanism and faulted structure as reported by Nwankwo.
Table 1. Thermal property over Niger Delta basin.
Table 2. Thermal property over Anambra basin.
Table 3. Thermal property over Dahomey basin.
Table 4. Thermal property over Bida basin.
Table 5. Thermal property over Chad basin.
Table 6. Thermal property over Sokoto basin.
In , the heat catchment area of the Upper-Benue basin is in the Potiskum, Gombe, and Kafarati areas of the basin which corroborates with the highest heat flow variations in these areas as reported by Kwaya (2016). In , the heat catchment area of the Mid-Benue basin is in the Lokoja and Otukpa areas of the basin which corroborates with the subsurface heat flow in the basin which host undifferentiated old granites as reported by Adetona, Salako, and Rafiu (Citation2017). In , the heat catchment area of the Basement Complex is in the Malumfashi and Kano areas of the basin due to the heat flow diffusing from the basement.
Table 7. Thermal property over Upper-Benue basin.
Table 8. Thermal property over Mid-Benue basin.
Table 9. Thermal property over Basement Complex.
The spatial map in represents the terrestrial radiation of the year from January to December (Terr_Year). This is the mean of the terrestrial radiation throughout the year clearly shows heat catchment areas, low, medium, and high geothermal well locations. The increase in the temperature of the basin compared to other basin is attributed to high tectonic activity in Chad basin and the basin is considered unstable (Adedapo, Kurowska, and Ikpokonte Citation2013). However, there are some assumptions based on the geology of the area.
The sedimentary basins are linked to the formation of the Benue trough and were created by the separation of the South American and African plates in the Early Cretaceous. Shortly after that deposition or sedimentation began with magma emplacement resulting in igneous intrusions in the upper area of the Benue trough, and the Asu River Group the oldest sediment followed by Ezeaku Group, and Awgu Group being the youngest (Nwajide Citation1990). These geological features explain the clustering of heat catchment and geothermal wells from the Mid-Benue trough to the Anambra basin.
The composition of the youngest formation in the sedimentary basin is composed mostly of fine to coarse sand and some intercalations of shale. These minerals possess a thermal conductivity that ranges from high to low. The thermal conductivity of these minerals was determined by Robertson (Citation1988) with the highest Quartz at about 7.8 W/m K, Calcite at 3.4 W/m K, K-felspar 2.3 W/m K, etc., while the basement complex is made of Migmatic Gneiss, Quartzite, cal-silicate, biotite-hornblenbe schist, and amphibolites. Because of the thermal conductivity of the minerals in the formation, they are able to absorb the radiation coming from the sun. Formations having a lot of minerals with high conductivity absorb more radiation thereby producing high terrestrial radiation in these areas while formations having mineral composition having low thermal conductivity, reflect low terrestrial radiation values despite the season. This discovery makes the heat catchment areas more prone to mineral deposits.
The area covered by the southern basement complex of Nigeria the predominantly composed of amphibolites, migmatite gneisses, granites, pegmatites, and schists which is made up of biotite schist, quartzite schist talk-tremolite schist, and the muscovite schists with a crystalline intrusion. The intermediate and mafic rocks in southwestern areas of Nigeria are the hornblende gneiss and the amphibolite. These amphibolites are found in riverbeds and outcrops in valley-like areas in the Ilesha and Ife regions. The enormous dark-green, fine-grained melanocratic amphibolite. Commonly hornblende gneiss outcrops are bordered with the large foliated melanocratic amphibolite. This rock (hornblende gneiss) crops out in Igangan, Aiyetoro, and Ifewara, along Ile-Ife road in southern Nigeria. The hornblende gneiss is folded, faulted with strong foilations.
More than 75% of the earth’s surface in the basement complex of southwestern Nigeria is made up of the magmatite-gnessic complex, which is thought to have evolved during three significant geotectonic events: Early Proterozoic (2000 Ma) granite emplacement, the Pan African events, and the Ibadan (Southwestern Nigeria) grey gneisses are all examples of the beginning of the crust-forming process. Based on geological field mapping, Rahaman and Ocan (Citation1978) documented over 10 evolutionary processes of the basement complex, with the intrusion of dolerite dykes being the most recent. These geological facts and terrestrial radiation output in the Southwest Nigeria make Ijebu-Ode, Ijebu-Igbo, and Shagamu areas of interest. Though the convergences of these facts extend to Ikogosi which is already known by the presence of warm spring, there is the need to perform field measurement in Ijebu-Ode, Ijebu-Igbo, and Shagamu.
The spatial map in shows the Geothermal gradient throughout the year from January to December. The geothermal gradient <0.5 reveals locations that may not have any geothermal potentials. Locations with geothermal gradient within the range of 0.5–1 have low terrestrial radiation ≥702 W m−2 are potential locations for deep geothermal wells. Locations with geothermal gradients >1 are likely regions where low and medium geothermal wells can be found. This observation corroborates the seasonal terrestrial radiation.
According to Adedapo, Kurowska, and Ikpokonte (Citation2013), a high geothermal gradient value is 7.62°C/100 m gotten from the northeastern region of the Chad basin. This work corroborates that the existence of Ikogosi warm spring in the Ado areas of Ekiti and this latest finding suggests that local abnormalities can cause the distribution of geothermal heat inside Nigeria’s Precambrian basement rock to vary. The Irrua, Ekpoma, Kwale, Ubiaja, Agbor, Edo, Yenagoa, Elele, Degema, areas of the Niger Delta basin. Adedapo, Kurowska, and Ikpokonte (Citation2013) observed in their work that the highest geothermal values were recorded very close to the Cretaceous rocks outcropping in the North of Niger Delta while the minimum geothermal gradient obtained across the sedimentary basin is 1.2°C/100 m which is found at the centre of the mid-Benue trough, the Anambra basin, and the Chad basin. Kwaya, Kurowska, and Arabi (Citation2016) geothermal gradients of 4°C/100 m were seen in Gulumba, Kukawa, and Dikwa, 5.84°C/100 m were recorded in the southern part of Bida, and lesser values of 2.85°C/100 m were seen at Bama and Kala Balge. This work further provides the geothermal gradient for Kaura-namoda, kotorkoshi, Gora, Birnin-gwari, Lere, Kajuro of the basement complex of the Northwest, Bokkos area of the Jos-Pleteau complex, Kanti, Eban, Bida areas of Bida basin, the Lafia, Wikki, Gombe Numan and Suwa areas in the upper Benue basin, Dan-sade, Argungu, Birnin-kebbi, Bagudo which is the SW-NE of Sokoto basin. However, this supports the finding that the Sokoto basin’s zone of maximum gradients is stretched in an SW-NE direction, parallel to the general strike of the region’s major sedimentary formations, which are very thin.
Statistical analysis of terrestial radiation for December, January, and February (Terr_DJF), March, April, and May (Terr_MAM), June, July, August (Terr_JJA), September, October, November (Terr_SON), January to December (Terr_Year) and geothermal gradient of January to December (GG_Year) across Nigeria were analysed within geological description based on the different sedimentary basins and basement complexes in Nigeria. They are the Anambra Basin, Bida Basin, Sokoto Basin, Chad Basin, Niger Delta Basin, Dahomey Basin, Upper Benue Basin, Mid-Benue Basin, Basement Complex.
shows the results from the Kolmogorov–Smirnov Test and the Shapiro–Wilk Test normality test for Anambra basin. This shows the significant value for Terr_DJF, Terr_MAM, Terr_JJA, Terr_SON, and Terr_Year to be 0.000. This indicates that the normality in the data is significant because it is less than 0.05 and it is not normally distributed. Also, the normality test of GG_year has a slightly higher significant value of .006 for the Kolmogorov–Smirnov Test and 0.002, and for the Shapiro–Wilk Test which indicates that the data is significant and not normally distributed.
Table 10. The Normality test of Anambra basin.
shows the results from the Kolmogorov–Smirnov Test and the Shapiro–Wilk normality Test of Bida basin. This shows inconsistency in the significant value Terr_DJF, Terr_MAM, Terr_JJA, Terr_SON, and Terr_Year of both tests. Also, the normality test of GG_year has a higher significant value of 0.200 for Kolmogorov–Smirnov Test and 0.163 for the Shapiro–Wilk Test which indicates that the data is not significant and normally distributed. From correlation table in the Appendix, each individual variables (Terr_DJF, Terr_MAM, Terr_JJA, Terr_SON, Terr_Year, and GG_Year) correlate to one another, which is indicative of a positive correlation. Kendal Tan and Spearman’s rho shows a significant correlation with the terrestrial radiation data interval of 40 years. However, there was no correlation between seasonal terrestrial radiation to the geothermal gradient. This observation gives credence to the assertion made in spatial analysis.
Table 11. The normality test of Bida basin.
shows the results from the Kolmogorov–Smirnov and the Shapiro–Wilk normality test of the Basement complex. This shows the significant value for Terr_DJF, Terr_MAM, Terr_JJA, Terr_SON, Terr_Year, and GG_Year to be 0.000. This indicates that the normality in the data is significant because it is less than 0.05 but not normally distributed.
Table 12. The normality test of basement complex.
The Univariate outliners test was carried out on Terr_DJF, Terr_MAM, Terr_JJA, Terr_SON, Terr_Year, and GG_Year of Basement complex which showed three outliners at Gaya, Kano, Moriki, and Sabon birnin gwari. shows the results from the Kolmogorov–Smirnov and the Shapiro–Wilk normality test of Dahomey basin. This shows the significant value for Terr_DJF, Terr_MAM, Terr_JJA, Terr_SON, and Terr_Year to be 0.000. This indicates that the normality in the data is significant and not normally distributed. Also, the normality test of GG_year has an increase in the significant value with 0.144 for Kolmogorov–Smirnov Test and 0.359 for the Shapiro–Wilk Test which indicates that the data is significant and not normally distributed.
Table 13. The normality test of Dahomey basin.
The Univariate outliners test was carried out on Terr_DJF, Terr_MAM, Terr_JJA, Terr_SON, Terr_Year, and GG_Year of the Dahomey basin which showed three outliners at Owode, Okitipupa, and Sango-Ota. shows the results from the Kolmogorov–Smirnov Test and the Shapiro–Wilk normality test of Mid-Benue basin. This shows the significant value for Terr_DJF, Terr_MAM, Terr_JJA, Terr_SON, and Terr_Year to be 0.000. This indicates that the normality in the data is significant because it is less than 0.05 and it is not normally distributed. Also, the normality test of GG_year has an increase in the significant value with 0.200 for Kolmogorov–Smirnov Test and 0.199 for the Shapiro–Wilk Test which indicates that the data is significant and not normally distributed. The Univariate outliners test was carried out on Terr_DJF, Terr_MAM, Terr_JJA, Terr_SON, Terr_Year, and GG_Year of the Mid-Benue basin which shows an outliner at Egume. shows the results from the Kolmogorov–Smirnov and the Shapiro–Wilk normality test of Niger Delta basin. This shows the significant value for Terr_DJF, Terr_MAM, Terr_JJA, Terr_SON, and Terr_Year to be 0.000. This indicates that the normality in the data is significant because it is less than 0.05 and it is not normally distributed. Also, the normality test of GG_year has an increase in the significant value with 0.039 for Kolmogorov–Smirnov Test and 0.275 for the Shapiro–Wilk Test which indicates that the data is significant and not normally distributed.
Table 14. The normality test of Mid-Benue basin.
Table 15. The normality test of Niger Delta basin.
The Univariate outliners test was carried out on Terr_DJF, Terr_MAM, Terr_JJA, Terr_SON, Terr_Year and GG_Year of Basement complex of the North West which showed three extreme outliners in Ekpoma, Kwale, and Ubiaja. shows the results from the Kolmogorov–Smirnov and the Shapiro–Wilk normality test of the Sokoto basin. This shows inconsistency in the significant value Terr_DJF, Terr_MAM, Terr_JJA, Terr_SON, and Terr_Year of both tests. Also, the normality test of GG_year has a higher significant value of 0.200 for Kolmogorov–Smirnov Test and 0.747 for the Shapiro–Wilk Test which indicates that the data is not significant and normally distributed.
Table 16. The normality test of Sokoto basin.
presents the results from the Kolmogorov–Smirnov and the Shapiro–Wilk normality test of Upper Benue basin. This shows inconsistency in the significant value Terr_DJF, Terr_MAM, Terr_JJA, Terr_SON, and Terr_Year of both tests. Also, the normality test of GG_year has a higher significant value of 0.200 for Kolmogorov–Smirnov Test and 0.867 for the Shapiro–Wilk Test which indicates that the data is not significant and normally distributed. The Univariate outliners test was carried out on Terr_DJF, Terr_MAM, Terr_JJA, Terr_SON, Terr_Year and GG_Year of Upper Benue basin.in Potiskum. shows the results from the Kolmogorov–Smirnov and the Shapiro–Wilk normality test of Chad basin. This shows the significant value for Terr_DJF, Terr_MAM, Terr_JJA, Terr_SON, and Terr_Year to be 0.000 which indicates that the data are significant because it is less than 0.05 and it is not normally distributed. GG_year has an increase in the significant value with 0.200 for Kolmogorov–Smirnov Test and 0.696 for the Shapiro–Wilk Test which indicates that the data is significant and not normally distributed.
Table 17. The normality test of Upper Benue basin.
Table 18. The normality test of Chad basin.
From the spatial and statistical analysis, it is postulated that the remote sensing technique is most accurate within a geothermal mechanism i.e. convection and counter flow in a multi faulted zones. The thermal reversal depth (TRD) was postulated that as the best means of classifying geothermal wells. TRD is the depth below the earth crust where the temperature reverses from negative to positive trend. In other to validate the TRD concept two known geothermal wells located in Dixie Valley, USA and Efeler, Turkey were used. The graph () shows the temperature from 10 am to 3 pm against soil depth of Cerro Prieto, Mexico. The pattern shows a parabolic shape. The points of reversal are very essential in classifying deep, medium and shallow geothermal wells. In this case, the temperature reverses at depth 1.45 m and the pattern is being duplicated at varying magnitude throughout the day at varying magnitude as seen in .
The graph () shows the temperature from 10 am to 3 pm against soil depth of Cesano, Italy. The pattern shows a parabolic shape. These patterns are known patterns for heat catchment or geothermal stations. The points of reverse are very essential in classifying deep, medium and shallow geothermal wells. In this case, the temperature reverses at depth of 0.7 m and the pattern is being duplicated at varying magnitude throughout the day at varying magnitude as seen in . The graph () shows the temperature from 10 am to 3 pm against soil depth of Shagamu. In this case, there were no TRD that was observed. However, due to the trend and feature dataset which benchmarked with known geothermal wells across the globe, there was the need to further the research by field measurement as a way of validating the already established postulate. The ground measurement temperature against depth of seven borehole wells in Shagamu is presented in . The pattern shows a parabolic shape at varying depth at different locations. For example, thermal reversal at depth 0.6 m for location 1; 5 m for locations 4 and 5; 6 m for locations 4,6, and 7; 12 m for location 2.
The graph shows the temperature from 10 am to 3 pm against soil depth of Ijebu Igbo (). In this case, there were no TRD that was observed. However, due to the trend and feature dataset which benchmarked with known geothermal wells across the globe, there was the need to further the research by field measurement as a way of validating the already established postulate. Since the positive thermal reversal is a salient feature for detecting geothermal well location. Due to the depth of thermal reversal, it can be inferred that Shagamu has a middle geothermal well. The graph () shows the temperature of satellite data for 10 am–12 pm and ground measurement temperature against depth of six borehole in Ijebu Igbo. The pattern shows a parabolic shape at varying depth at different locations. For example, reverses at depth 5 m for location 1; 6 m for location 2, and 4; 9 m for location 4,5, and 6; and 10 m for location 3. The thermal reversal is an indication that it is a heat catchment or geothermal well location. However, the temperature reversal depth makes it a middle geothermal well.
The validation of the field can be seen in the work existing works (Adetona, Salako, and Rafiu Citation2017; Ademila Citation2018; Okeifufe, Izuwa, and Nwogu Citation2020).
5. Conclusion
The exploration of potential geothermal wells and heat catchment areas using the aided methodology of remote sensing data has shown huge success. The Surface Energy Balance model was used to estimate seasonal and non-seasonal terrestrial radiation features which are essential for the highlighted objectives of this study. The seasonal terrestrial radiation is an indication of mineral deposits or heat catchment zones while the non-seasonal features are most likely geothermal locations as the heat is constantly coming from the molten magma. The normality of the terrestrial radiation in each geological classification gives credience to the variability within each geological zone. The spatial analysis reveals that there are more geothermal wells in mid and southern Nigeria, though most of these geothermal wells are mid-depth wells. The geothermal gradient for Kaura-namoda, kotorkoshi, Gora, Birnin-gwari, Lere, Kajuro of the basement complex of the Northwest; Bokkos area of the Jos-Pleteau complex; Kanti, Eban, Bida areas of Bida basin; Lafia, Wikki, Gombe Numan and Suwa areas in the upper Benue basin; Dan-sade, Argungu, Birnin-kebbi, Bagudo which is the SW–NE of Sokoto basin; are indication that the geothermal wells located in the northern parts of Nigeria are mainly deep geothermal wells. Also, the heat catchment area of the Sokoto basin is in the Kuchi, Gwandi, and Gwadabawa areas of the basin which corroborates with the subsurface thermal flow in the NE–SW direction and also, the increase from the south towards the north with values above 100 W m−2 that has been associated with young volcanism and faulted structure. The geothermal gradient >0.5 reveals locations that may not have any geothermal potential. Locations with geothermal gradient within the range of 0.5–1 have low terrestrial radiation ≥702 W m−2 are potential locations for deep geothermal wells. Locations with geothermal gradients >1 are likely regions where low and medium geothermal wells can be found. This observation corroborates the seasonal terrestrial radiation. The thermal reversal depth (TRD) was proposed which has been validated by existing geothermal wells around the globe and field dataset. Based on the TRD, it is easy to determine geothermal wells in multi-faulted zones. The findings in this research contributed the following to knowledge: produced baseline geothermal energy prospective locations across Nigeria; created a near-surface heat analogue for determining heat catchment areas; and introduced the thermal reversal concept for classifying heat catchment and geothermal wells. From the above study, it is recommended that further ground studies be carried out in the basement complexes of Nigeria. This is because there is little or no information on the temperature at depth due to the formation in those areas. The strength of this work is the adoption of the model and satellite dataset for the determination of heat catchments, shallow geothermal wells, and deep geothermal wells. This protocol has been reported to be >72% accurate. The limitation of the research is the inability to clearly differentiate the difference between middle and deep geothermal wells. It is therefore recommended that a rock thermal model be incorporated into the model to compare theoretical and experimental trends.
Disclosure statement
No potential conflict of interest was reported by the author(s).
References
- Abuzied, S. M., M. F. Kaiser, E.-A. H. Shendi, and M. I. Abdel-Fattah. 2020. “Multi-Criteria Decision Support for Geothermal Resources Exploration Based on Remote Sensing, GIS and Geophysical Techniques Along the Gulf of Suez Coastal Area, Egypt.” Geothermics 88 (101893): 101893. doi:10.1016/j.geothermics.2020.101893.
- Adedapo, J. O., E. Kurowska, and A. Ikpokonte. 2013. “Geothermal Gradient of the Niger Delta From Recent Studies.” International Journal of Science Engineering Research 2: 11–20.
- Adedayo, H. B., S. A. Adio, and B. O. Oboirien. 2021. “Energy Research in Nigeria: A Bibliometric Analysis.” Energy Strategy Reviews 34 (100629): 100629. doi:10.1016/j.esr.2021.100629.
- Ademila, A. O. 2018. “Radiometric Survey in Geological Mapping of Basement Complex Area of Parts of Southwestern Nigeria.” Tạp chí khoa học về trái đất 40 (3): 288–298. doi:10.15625/0866-7187/40/3/12619.
- Adetona, A. A., K. A. Salako, and A. A. Rafiu. 2017. “Curie Depth and Geothermal Gradient from Spectral Analysis of Aeromagnetic Data Over Upper Anambra and Lower Benue Basin, Nigeria.” Nigerian Journal of Technological Research 12 (2): 20. doi:10.4314/njtr.v12i2.4.
- Amoo, L. M. 2019. “Low-Enthalpy Geothermal Springs for Power Generation – An Alternative Approach.” OAlib 06 (11): 1–14. doi:10.4236/oalib.1105866.
- Arizona. 2022. http://www.atmo.arizona.edu/students/courselinks/fall10/atmo579/ATMO579_files/ClassNotes9.pdf): Retrieved June 14, 2022, from Arizona.edu website (Arizona edu, 2022).
- Calvin, W. M., E. Littlefield, and C. Kratt. 2015. “Remote Sensing of Geothermal-Related Minerals for Resource Exploration in Nevada.” Geothermics 53: 517–526. doi:10.1016/j.geothermics.2014.09.002
- Elehinafe, F. B., O. A. Odunlami, A. O. Mamudu, and O. O. Akinsanya. 2021a. “Investigation of the Potentials of Southwest Nigerian Napier Grass as an Energy Source to Replace Fossils Used in Firing Thermal Power Plants for Air Emissions Control.” Results in Engineering 11 (100259): 100259. doi:10.1016/j.rineng.2021.100259.
- Ema, A., I. Onyekachi, C. Chibuzo, and R. Onwe. 2019. “Geothermal Energy Reconnaissance of Southeastern Nigeria From Analysis of Aeromagnetic and Gravity Data.” Analysis of Aeromagnetic and Gravity Data; Pure and Applied Geophysics 176: 1615–1638.
- Emetere, M. E., U. Okoro, B. Etete, and G. Okunbor. 2016. “Free Energy Option and Its Relevance to Improve Domestic Energy Demands in Southern Nigeria.” Energy Reports 2: 229–236. doi:10.1016/j.egyr.2016.09.001.
- Emujakporue, G. O., and A. S. Ekine. 2014. “Determination of Geothermal Gradient in the Eastern Niger Delta Sedimentary Basin From Bottom Hole Temperatures.” Journal of Earth Science and Geotechnical Engineering 4: 109–114.
- Gupta, A., P. Mishra, C. Pandey, U. Singh, C. Sahu, and A. Keshri. 2019. “Descriptive Statistics and Normality Tests for Statistical Data.” Annals of Cardiac Anaesthesia 22 (1): 67. doi:10.4103/aca.aca_157_18.
- Haselwimmer, C., A. Prakash, and G. Holdmann. 2013. “Quantifying the Heat Flux and Outflow Rate of Hot Springs Using Airborne Thermal Imagery: Case Study from Pilgrim Hot Springs.” Alaska. Remote Sensing of Environment 136: 37–46.
- Jiang, C., X. Wang, F. Zhang, K. Deng, and Q. Lei. 2022. “Fracture Activation and Induced Seismicity During Long-Term Heat Production in Fractured Geothermal Reservoirs.” Rock Mechanics and Rock Engineering, 55: 5235–5258. doi:10.1007/s00603-022-02882-z.
- Keam, R. F., M. L. Katherine, and Z. P. Leonie. 2005. “Definition and Listing of Significant Geothermal Feature Types in the Waikato Region.” Proceedings World Geothermal Congress Antalya, pp. 24–29.
- Kehinde, O., K. Babaremu, K. Akpanyung, E. Remilekun, S. Oyedele, and J. Oluwafemi. 2018. “Renewable Energy in Nigeria – A Review.” International Journal of Mechanical Engineering and Technology 10: 1085–1094.
- Kwaya, M. Y., E. Kurowska, and A. S. Arabi. 2016. “Geothermal Gradient and Heat Flow in the Nigeria Sector of the Chad Basin, Nigeria.” Computational Water Energy and Environmental Engineering 05 (02): 70–78. doi:10.4236/cweee.2016.52007.
- Manan, S., S. Anirbid, V. Dwijen, S. Shreya, C. Anjali, and D. Shubhra. 2015. “Overview of Geothermal Surface Exploration Methods.” Overview of Geothermal Surface Exploration Methods 1.
- Meng, F., X. Liang, C. Xiao, and G. Wang. 2021. “Geothermal Resource Potential Assessment Utilizing GIS – Based Multi Criteria Decision Analysis method.” Geothermics 89 (101969): 101969. doi:10.1016/j.geothermics.2020.101969.
- Nwajide, C. S. 1990. Cretaceous Sedimentation and Palaeogeography of the Central Benue Trough. International Monograph Series, Braunschweig, 19-38.
- Nwankwo, L. I., and A. J. Sunday. 2017. “Regional Estimation of Curie-Point Depths and Succeeding Geothermal Parameters From Recently Acquired High-Resolution Aeromagnetic Data of the Entire Bida Basin, North-Central Nigeria.” Geothermal Energy Science 5 (1): 1–9. doi:10.5194/gtes-5-1-2017.
- Obaje, N. G. 2009. “Geology and Mineral Resources of Nigeria.” Lecture Notes in Earth Sciences.
- Okeifufe, N. F., N. C. Izuwa, and N. Nwogu. 2020. “Estimating the Quantity of Recoverable Heat in a Geothermal Reservoir in Nigeria,” in Day 1 Tue, August 11, 2020. SPE.
- Oludamilare, B. A., K. K. Mark, F. A. Ayodeji, A. Theophilus, I. A. Oluwatobi, and S. Tomonobu. 2020. “Challenges and Prospects of Nigeria’s Sustainable Energy Transition With Lessons From Other Countries’ Experiences.” Energy Reports 6: 993–1009. doi:10.1016/j.egyr.2020.04.022
- Onuoha, K. M., and A. S. Ekine. 1999. “Subsurface Temperature Variation and Heat Flow in the Anambra Basin, Nigeria.” Journal of African Earth Sciences 28 (3): 641–652. doi:10.1016/S0899-5362(99)00036-6
- Pierrehumbert, R. T. 2011. “Infrared Radiation and Planetary Temperature.” Physics Today 64 (1): 33. doi:10.1063/1.3541943.
- Rahaman, M. A., and O. O. Ocan. 1978. “On Relationship in the Precambrian Migmatite Gneiss of Nigeria.” Journal of Mining and Geology 15: 23–30.
- Robertson, E. C. 1988. Thermal Properties of Rocks, United States Department of the Interior Geological Survey, Open-File Report 88–441.
- Suleiman, T., F. N. Okeke, and N. D. Obiora. 2021. “Spectral Analysis of High-Resolution Aeromagnetic Data for Geothermal Energy Reconnaissance Across Sokoto Basin, Northwest, Nigeria.” Journal of the Earth and Space Physics 46 (4): 147–158.
- Tanaka, A., Y. Okubo, and O. Matsubayashi. 1999. “Curie Point Depth Based on Spectrum Analysis of the Magnetic Anomaly Data in East and Southeast Asia.” Tectonophysics 306 (3–4): 461–470. doi:10.1016/s0040-1951(99)00072-4.
- Tende, A. W., M. D. Aminu, and J. N. Gajere. 2021. “A Spatial Analysis for Geothermal Energy Exploration Using Bivariate Predictive Modelling.” Scientific Reports 11 (1): 19755. doi:10.1038/s41598-021-99244-6.
- Trenberth, K. E., J. T. Fasullo, and J. Kiehl. 2009. “Earth’s Global Energy Budget.” Bulletin of the American Meteorological Society 90 (3): 311–324. doi:10.1175/2008bams2634.1.
- Usman, O., C. C. Ezeh, A. O. Omali, and A. I. Chinwuko. 2019. “Integration of Aeromagnetic Interpretation and Induced Polarization Methods in Delineating Mineral Deposits and Basement Configuration Within Southern Bida Basin, North-West Nigeria.” In On Significant Applications of Geophysical Methods, 69–71. Cham: Springer International.
- Vaughan, R. G., L. P. Keszthelyi, A. G. Davies, D. J. Schneider, C. Jaworowski, and H. Heasler. 2010. “Exploring the Limits of Identifying Sub-Pixel Thermal Features Using ASTER TIR Data.” Journal of Volcanology and Geothermal Research 189 (3-4): 225–237.
- Wang, K., B. Yuan, G. Ji, and X. Wu. 2018. “A Comprehensive Review of Geothermal Energy Extraction and Utilization in Oilfields.” Journal of Petroleum Science & Engineering 168: 465–477. doi:10.1016/j.petrol.2018.05.012.