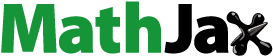
ABSTRACT
The demand response and peak power limiting could potentially reduce the peak power and energy demand. This study examines the effect of rule-based demand response and peak power limiting on the peak power and energy demand of heating. Study was conducted as a co-simulation where buildings and district heating production were simulated separately but both inclusively. Results indicate that demand response provides 2.8–4.7% energy saving and 2.3–3.4% total district heating cost saving potential. Moreover, according to the simulations, demand response provides 32% emission reduction in district heating production in contrast to the reference case. Peak power limiting provides significant reduction in the peak power and district heating base cost. However, its ability to provide additional reduction in the energy demand and emissions is confined compared to the demand response due to the effective time of the limit. Chosen acceptable temperature range can be maintained decently.
Introduction
Buildings are responsible of 35% of the global final energy consumption (Hamilton et al. Citation2020). Buildings’ operation is responsible for 28% of the global greenhouse gas (GHG) emissions, when indirect upstream energy generation emissions are considered (IEA Citation2020). Building sector’s operational emissions reached all time high of 10 GtCO2 in 2019. When building construction industry is accounted the building sectors share of global emissions increases to 38% (Hamilton et al. Citation2020; IEA Citation2020). To adjust with the net zero targets by 2050, EU has set the most recent target of reducing CO2 emissions by 55% by 2030 compared to 1990 (European Commission Citation2021).
Heating is the largest form of energy end use, accounting of over half of the final energy consumption in EU. Space heating accounts for about 27% in European Union (EU28) total energy demand (HRE4 Citation2017). The majority of the heat production relies on the fossil fuel burning. Fossil-fuel-based energy generation is associated with climate change, air pollution, health risks, and cost dependency on hydrocarbon markets. In 2019, about 55% of the people lived in urban areas, and due to the rapid urbanisation share is predicted to rise to 68% by 2050. Thus heat demand in cities is increasing and buildings and cities urgently require alternatives for fossil fuels and other combustion-based energy production to avoid the worst climate concerns to realise (Bertelsen et al. Citation2021). Transition to a sustainable heating sector requires investments in energy efficiency to decrease the total energy demand, and to energy production systems to replace fossil fuels with renewable and non-emitting energy sources (Mathiesen et al. Citation2019).
District heating (DH) networks are utilised to deliver heating to buildings. District heating provides an efficient heating supply system especially in densely populated urban areas in colder climates (Paiho and Saastamoinen Citation2018). It allows an efficient waste heat utilisation among buildings and can provide centralised heat storages. In 2021, about 8% of the total heat consumption was delivered by district heating networks globally, of which Europe, Russia and China together established more than 90% (Delmastro et al. Citation2022; IEA Citation2019). In European Union, district heating’s market share is 13% of the total heat demand of buildings (Connolly et al. Citation2014). Especially countries located in Northern Europe have high penetration of district heating with market shares up to 65% (Kontu et al. Citation2020; Werner Citation2017). In Germany, district heating accounts for about 14% of the total heating market (Moczko Citation2019). In 2016, around 83% of the district heat was produced by combined heat and power (CHP) plants, 15% by heat-only boilers (HOB) and 2% by excess heat from industry in Germany (Popovski et al. Citation2019). Fuel mix in the German district heating production relies mainly to natural gas and coal, which account for 46% and 36% of the production, respectively. Waste fuels represent 13% and biomass 6% of the fuel mix (Popovski et al. Citation2019). Decarbonising the district heating sector is essential to meet the ambitious climate goals in EU.
Emissions can be reduced by deep renovation of the buildings or decarbonising the energy sector. Using less-emitting sources of heat such as heat pumps, biogas or -mass in the CHP plants and HOBs, and maximising the waste heat exploitation are some of the main alternatives of the sustainable district heating production. Electrifying heating and maximising the renewable utilisation in the heat production via heat pumps requires flexibility from the energy system as major renewable electricity sources are intermittent.
Demand side management (DSM) refers to the energy efficiency actions and consumption profile changes taken in the demand side of the energy supply chain (Eissa Citation2011). In general, demand side management includes variety of actions to reduce the energy demand such as energy efficiency, energy storages and demand response (Peltokorpi et al. Citation2019). In scientific literature, demand response (DR) is a subgroup of DSM and it often refers to the changes in consumption profile (Siano Citation2014). The purpose of the demand response is to reduce the energy consumption at peak times and balance the demand profile. Different demand response actions are for example load shifting, valley filling and peak cutting.
Demand response can increase the flexibility of the energy network. Purpose is to match the demand to the supply by changing demand pattern, not by increasing supply (Safdar, Hussain, and Lehtonen Citation2019). There are two main demand response strategies: incentive- and price-based. In incentive-based strategies, utility company pays customer incentives to reducing the supply to the customer when the network load is under threat. Price-based strategies rely on the dynamic pricing of the energy, which is adjusted higher on peak-times and lower on off-peak periods. Customers then adjust their demand curve so they can benefit from the lower prices on off-peak periods (Shan et al. Citation2016). Thus, in the production side, demand response offers a way to mitigate the usage of peak power fuels. It enables consumers to act as a prosumers, active operators in the energy market.
Demand response of electricity networks has been studied widely. DR control is also implemented in electrical heating and direct electric appliances. Yin et al. studied the flexibility of electricity of thermostatically controlled HVAC loads in commercial and residential properties (Yin et al. Citation2016). Ji, Baldick, and Novoselac (Citation2014) studied the real-time price based dynamic demand response control of the electrical heating and air conditioning in residential building. Study conducted by Eseye et al. (Citation2019) studied the building integrated and EV batteries demand response potential. Alimohammadisagvand (Citation2016; Citation2017) and Arabzadeh (Citation2018) studied the thermal comfort and cost saving of demand response in electrically and heat pump heated house. Furthermore, demand response of electricity loads has been studied by Albadi and El-Saadany (Citation2008) and Sun, Li, and Dababneh (Citation2016). Price-based demand response of electricity is easy to apply as the electricity market is open and prices are transparent to everyone.
Similar applications in the district heating are rare and research topic is not as widespread as electricity demand response. However, demand response control of space heating, ventilation and domestic hot water have been studied in for example (Cai, You, and Wu Citation2020; Guelpa and Verda Citation2021; Knudsen and Petersen Citation2017). Moreover, demand response control of space heating in different building types was studied in Suhonen et al. (Citation2020). Yuan et al. (Citation2021) studied district heated swimming hall’s demand response potential. Ju et al. (Citation2021a; Citation2021b) studied the energy and power flexibilities in district heated buildings. These studies propose that demand response can enable moderate cost and energy savings for the building owner as well as increase the flexibility in the district heating network. However, demand response of district heating is not commonly feasible in current district heating market. There is no incentive for the customer to change the consumption pattern as there are many different energy related saving opportunities for building owners.
In district heating, demand response is applied by storing heat to the structures of the building, or to separate thermal storage, when the energy prices are low. Buildings with more thermal mass are more suitable for the structural thermal storage. Case buildings selected for this study represent buildings with brick or concrete structures which tend to have more thermal mass than those of wooden structures for example. To apply the price-based demand response, dynamic pricing of the heat is required. In district heating market, the dynamic pricing is not utilised as in electricity market, and it is known as a rigid business which is facing many challenges in the energy market (Ala-kotila, Vainio, and Heinonen Citation2020). However, dynamic pricing has been studied in multiple papers (Dominković et al. Citation2018; Li et al. Citation2019). Changing pricing mechanisms and transitioning from traditional customers to prosumers, which gives them opportunity to benefit from changing their consumption patterns, can be beneficial for both parties. This substantiates the research attention also in this paper.
Peak power limiting (i.e. demand limiting or peak cutting) is a demand response method where the power demand is decreased by controlling with an algorithm or setting an upper limit for the power demand. Ala-kotila, Vainio, and Heinonen (Citation2020) conducted a demand response case study in district heating network. The study demonstrated the potential of demand response in apartment buildings by using energy and cost saving, emission reductions, and peak power reduction as indicators. In that case, demand response algorithm was applied for peak shaving by preferring domestic hot water heating over space heating. Peak load was reduced by 14–15%. Moreover, results indicated 9% annual reduction in normalised energy consumption, energy cost and greenhouse gas emissions. However, they did not implement strict peak power limits for the heating.
In this study, direct peak power limiting is studied by setting an upper limit for the power demand. The main objectives of the peak power limiting are reducing the peak power demand of the buildings and district heating network. The purpose is to obtain cost savings and reduce CO2 emissions by reducing the operation of the peak power plants, which are often based on fossil fuels burning. According to the best knowledge of the authors, there are no papers which study the peak power limiting as stated.
The novelty of this study lies in the combination of the building-level simulation and district heating production optimisation for demand response and peak power limiting analyses. This enables the study of the effect of the demand response and peak power limiting on the entire district heating system and the benefits of these for both the producer and the consumer. In this investigation, the effects of price-based demand response and peak power limiting are investigated on the energy and cost saving potential, and indoor thermal conditions on the building side. Furthermore, cost saving of the heat producer and CO2-emission saving from the production are investigated. Specific production scenario is adopted to determine the dynamic hourly price of the heat which is used for the demand response algorithm. The district heating network consists of certain amount of three different building types: apartment, cultural centre, and office. The production scenario includes variety of energy production methods, including biomass CHP, natural gas HOB, heat pump, solar thermal, and a storage tank.
Materials and methodology
Entire simulation process
The simulation process of this study consists of two main parts – district heating production and network level calculations and building level simulation. The overall flow chart of the study and complete simulation process are presented in .
Figure 1. Flow chart of the entire simulation process. Modified from Ju et al. (Citation2021).

The simulation process starts with the building simulations of studied buildings: apartment building, cultural centre, and office building. Studied case buildings are first simulated without demand response or peak power limiting. Second, the district heating network is constructed of the number of case buildings, to match it in one of the actual district heating networks in Hamburg. Third, the dynamic district heating price is determined based on the annual heat demand of the whole district heating network with selected production scenario using the heat generation schedule optimiser (HGSO) production optimisation tool.
After the first cycle, the process is repeated but demand response and peak power limiting are applied before the building simulations. The building simulations are conducted with indoor air setpoints defined by control algorithm (see Section 2.4.4) and predefined power limits for space and ventilation heating. Then the annual hourly district level heat demand defined with new building specific demands and the optimisation of the heat production is performed.
Building level simulations
The building level simulation process includes the dynamic building simulation and the determination of the demand response’s control signals and indoor air temperature setpoints by control algorithm.
Description of simulation process
Building simulation process is presented in . Building simulation starts with the defining of the hourly control signals. The control signals for each hour are defined in the Excel-tool based on the district heating price by Behrang–Sirén method (Alimohammadisagvand Citation2018). Then the indoor air temperature setpoints of space heating are calculated by setpoint control algorithm. Setpoint control algorithm receives control signal, acceptable indoor air temperature ranges, limiting outdoor temperature, and moving 24-h average of outdoor temperature. Then the setpoint smoothing is applied on the setpoints to avoid the rebound effect as described in Section 2.2.5. Finally, the dynamic building simulation is performed which outputs the energy consumption and indoor thermal conditions of the building. The output data is fed back to Excel where the results are analysed.
The standard EN 16798 provides input parameters concerning indoor environment (e.g. indoor air quality and thermal environment) for the design and assessment of energy performance of buildings (SFS-EN 16798-1, Citation2019). It was used to define the minimum acceptable indoor air temperature limit used in this study. Standard presents three categories for the indoor climate which apply for different situations. The minimum temperature limit of 20°C used in this study was chosen based on the category II as it represents the normal level of expectation of indoor climate and should be used in new buildings and renovations. Maximum temperature limit in heating season was set based on Suhonen et al. (Citation2020).
Building simulation tool
The dynamic building simulation tool used in this study was IDA Indoor Climate and Energy (IDA ICE) v. 4.8. IDA ICE is a detailed and dynamic multi-zone simulation software which can be used to perform simulations of energy consumption, indoor air quality, and thermal comfort in buildings. The software allows the modelling of buildings with different structural geometries and parameters, HVAC systems, on-site energy generation, and usage profiles. Furthermore, IDA ICE enables detailed modelling of different components and control of the technical systems. The software has been validated against the EN 15265-2007 and the EN 13791 standards (EQUA Citation2010; Kropf and Zweifel Citation2001). In addition, it has been validated in multiple studies (Achermann and Zweifel Citation2003; Bring, Sahlin, and Vuolle Citation1999; EQUA Citation2010; Moinard and Guyon Citation1999) and is widely used in the research and industry, thus it represents a proper simulation software for this study.
Weather data
Outdoor temperature used in this study is based on the actual measured weather data in Hamburg in 2018. Weather data includes hourly outdoor temperature, relative humidity and solar irradiation among other parameters. The measured outdoor temperature of the year is shown in . The minimum and maximum temperatures of the measured time series are −12.9°C and 35°C. The annual average outdoor temperature is 10.8°C and the according heating degree days at 15.5°C indoor temperature are 2147°Cd.
The design outdoor air temperature in Hamburg, where the studied district heating network and case buildings are located, is −12°C. The average outdoor temperature from 2018 is 10.8°C and that of TRY2015 is 9.7°C. TRY 2015 is based on the measured outdoor temperature between 1995 and 2012 provided by German Meteorological Service which represents the average climate conditions (Deutschen Wetterdienst Citation2017b; Citation2017a).
Case buildings description
In this study, three types of buildings were studied. The building types are apartment building, office building, and cultural centre. These types of buildings were selected because they exist in the actual studied district heating network in Hamburg. General building information are shown in .
Table 1. General building information.
The actual apartment building, that the IDA ICE model of this study is based on, is located in Hamburg, Germany. Building has been initially built in 1930s and it consist of four floors. The apartment represents a typical apartment building of era and location. In this study, it was assumed that the heating and natural ventilation system is in original condition.
The cultural centre is based on the actual building located in Hamburg. Building was originally built in early 1980s and it has been renovated since. It has four floors including basement. IDA ICE model was built according to the design documents.
The studied office building consists of four floors and includes spaces such as offices, conference rooms, and hallways. The building model was originally constructed by Martin (Martin et al. Citation2017). Parameters were adjusted so they match those of cultural centre as it better represents the building stock in Hamburg.
The building models have been constructed to IDA ICE based on the real geometries of the buildings, but some simplifications were made. For instance, the floors were expected to be identical and only the top floor was modelled and copied. Building model parameters are shown in . The building parameters were selected so they represent the construction and the latest renovation year of the building.
Table 2. Building model parameters used in the IDA ICE simulations.
In apartment building and cultural centre, space heating also heats the natural ventilation supply air. In cultural centre, the share of space heating power of the total design heating power without domestic hot water is 61% and ventilation heating power 39%. In the office, building corresponding values are 39% and 61% respectively.
District heating substation efficiency was set to 0.97. The control curves of the supply water temperature as function of outdoor temperature are presented in . For the apartment building, the maximum supply water temperature is 80°C and in cultural centre and office building it is 70°C.
presents the main input parameters of the ventilation systems of the studied buildings. Apartment building does not have mechanical ventilation and only utilises natural ventilation. Cultural central has three different ventilation systems depending on the space type: mechanical supply and exhaust, mechanical exhaust, and natural ventilation. Office building is equipped with mechanical supply and exhaust ventilation. None of the buildings is equipped with heat recovery. The mechanical ventilation systems were turned on 2 h before the expected occupied time and off 2 h after the occupancy ends. The air change rate of the natural ventilation was set based on Mikola (Citation2017). Mechanical ventilation airflow rates were set based on REHVA’s health-based ventilation guideline (Citation2012) for Europe (Seppänen et al. Citation2012). Duct pressure losses and fan efficiencies were chosen based on the standard EN 13779 (SFS EN 13779, Citation2007). Duct pressure losses were set to ‘high’ and fan efficiencies to ‘low’ according to the standard as the systems are expected to be old.
Table 3. Ventilation systems’ input parameters.
More detailed case building descriptions are presented in Suhonen et al. (Citation2020).
Demand response control and peak power limiting
In this study, rule-based demand response control is used. Control is based on the future hourly energy price, and it was assumed that future 24-h energy price is known. Demand response control consists of two main parts: control signal and control algorithm. In addition, there is third optional part, setpoint smoothing, which was also implemented in this study. Moreover, peak power limiting is applied to the space and ventilation heating along with the demand response in three different magnitudes: 80%, 70%, and 60%, where the percentage states the available heating power with respect to the realised peak power of the reference case.
Control signal
Control signal calculation is based on the Behrang–Sirén method (Alimohammadisagvand Citation2018) which is a moving average method. This particular control signal method has been developed by Alimohammadisagvand et al. (Martin et al. Citation2017; Muller Citation2018; Vand Citation2020) and has been used in multiple studies. Control signal gets a value based on the future 24-h price trend of the energy. Price trend is either increasing, decreasing, or flat, and the control signal gets a value of +1, −1, or 0, respectively.
Control signal is based on the hourly energy price (HEP), moving average energy price from hour + p to + q , and marginal value. Pseudo code of the control signal is presented in Equation (1)
(1)
(1) where (HEP) is the hourly energy price and
moving average of hourly energy price from hour + p to hour + q, and (CS) is the control signal.
Marginal value defines how sensitive the control signal is to the changes of the energy price. Lower marginal value makes the control signal more sensitive to the changes in price and higher marginal value less sensitive. Higher marginal value results in less positive control signal value, thus less potential heat loading hours and more heating energy savings. This was also one of the results in Ju et al. (Citation2021a) and Suhonen et al. (Citation2020). Marginal value used in this study is 75 €/MWh which was the higher value of two used in Suhonen et al. (Citation2020). Hourly energy prices fed into the control signal calculation in Excel resulted from the production side optimisation (see Section 2.3).
Setpoint control algorithm
presents the control algorithm. Control algorithm was adapted from Martin et al. (Citation2017) and was also implemented in Ju et al. (Citation2021a) and Suhonen et al. (Citation2020). Control algorithm determines the hourly space heating setpoints TSH,set based on the control signal. TSH,min and TSH,max represent the minimum and maximum space heating setpoints which were set to 20°C and 23°C in this study respectively. Tavr,24out is the moving 24-h outdoor temperature. Moreover, Tlim,out is the limiting outdoor temperature which avoids using of maximum setpoint of space heating when the Tavr,24out is higher than Tlim,out. Tlim,out was set to 0°C based on Martin et al. (Citation2017).
Control algorithm sets the space heating setpoint TSH,set to TSH,max if the price trend is increasing and control signal is +1, and Tavr,24out < Tlim,out. In the other hand, if the price trend is decreasing and control signal is −1, control algorithm sets the setpoint to TSH,min. Furthermore, if the price trend is flattening out on control signal is 0, setpoint is set to TSH,norm.
Indoor air temperature setpoint smoothing is applied as presented in Suhonen et al. (Citation2020). Setpoint smoothing adjusts the setpoint evenly and prevents the rebound effect of the demand response which could occur if it was adjusted from minimum to maximum at one time. Setpoint smoothing applies only upwards.
Peak power limiting
In this study, peak power limiting is applied to the space and ventilation heating. Power is limited directly at the substation of the radiator and air handling unit heating network. Domestic hot water’s power demand is not limited as its availability was desired to be secured in all conditions. Limiting peak power affects the inlet water of the heating water at colder conditions. Thus peak power limiting can have negative impact to the thermal comfort of the occupants. One of the objectives is to study the effect of the power limiting to the indoor air temperatures.
The peak power limiting cases studied were with 80%, 70% and 60% limits according to the realised space and ventilation heating peak power in the reference cases. This means that in the particular case, the peak power delivered to the building was reduced to 80%, 70% or 60% respectively, compared to the realised peak power without limiting in the reference case simulation. The simulation cases are described closely in Section 3.1.
District heating production and network level simulations
Description of simulation process
District heating production and network level simulations are performed based on the hourly building heat demand profiles of the year with specific district heating production scenario.
The district heating network level simulation and calculation process is presented in . First, number of each building type is determined according to the actual heat demand in the one of the district heating networks in Hamburg. The simulated buildings were proportioned to match the actual heating demand of the studied district heating network. Total hourly district heating demand in the simulated network is then determined according to the number of the buildings (see Section 2.3.4).
After the district heating network establishment was done the production cost of the district heating was determined by using the HGSO optimisation tool. The dynamic district heating consumer price profile was then determined based on the production cost. The result from the optimisation is the most economical heat generation schedule for district heating production of the modelled plant combination.
The output of the optimisation tool consists of hourly data of total production cost, costs of heat and electricity, CO2 emissions per plant, CO2 emissions costs, generated heat per plant, storage usage, plant fuel consumption, and revenues.
Simulation tool
The district heating production scenario optimisation was executed by using the HGSO optimisation tool. The optimisation tool HGSO is based on the study by Tillman (Citation2017). The optimisation model is a mixed-integer linear program (MILP), which was applied for energy plants generation scheduling (Christidis Citation2019). The objective of the optimisation tool is to find the most economical running schedule for the production units of the heating network. The constraints of the optimisation include technical and economical parameters. The tool has adopted the open-source solver for solving optimisation problem (Forrest and Lougee-Heimer Citation2005).
The HGSO validated using the reference data from the optimisation tool BoFiT. BoFiT is a commercial optimisation platform developed by ProCom GmbH which is used for smart grid energy and operation optimisation (Lu and Shumei Citation2018; ProCom GmbH Citation2021; Vogt et al., Citation2018). BoFit converts the studied plant into an MILP model which is widely used in district heating grid optimisation (Merkert, Haime, and Hohmann Citation2019). The tool has been validated in several studies (Henrikkson Citation2018; ProCom GmbH Citation2020). The validation of the HGSO is presented in study by Ju et al. (Citation2021).
Production scenario
In the study, the district heating production was simulated with specific generation combination. The demand response control utilised in this study reacts to the fluctuation of the district heating price. Thus more fluctuating cost profile results in higher impact on the demand response. The selection of the production scenario was based on the level of fluctuation of the cost profile of different production scenarios.
shows the specifications of the production scenario. The district heating production was based on biogas fuelled combined heat and power (CHP) plant, natural gas heat only boiler (HOB), heat pump, and solar thermal power. Additionally, there is a thermal storage coupled with the network, which all generation units are capable to charge. The total heating power is 5.59 MW.
Table 4. Generation units and their powers in the used production scenario.
The heat and electricity generation power of the CHP plant and gas boiler are based on the specifications of the actual district heating network of Hamburg. Heat pump and solar thermal plant were chosen for the additional heat capacity for this study to increase the share of the renewable production in the scenario. The capacity was chosen so the maximum heat demand was covered. The capacity of the heat pump is based on heat pump models in the market and the COP is 4.0 in all conditions. The variation of COP value depending on the supply water temperature was neglected to simplify the model. Moreover, the energy source of heat pump is not specified as it does not affect the operation order of the production plants in this study. The production power of the solar thermal plant is based on a measured time series of solar heat generation of the local solar thermal unit. Thermal storage is operated daily and is not used for seasonal heat storing.
Heat demand of the network must be covered by the production scenario for every hour of the year. The generation schedule was optimised with the HGSO tool that utilised electricity price, heat load and costs of different energy sources. HGSO calculated the most cost-effective way of producing heat with the specified production combination for each hour. The tool only considered the heat demand, and the supply water temperature was neglected. It was assumed that each generation unit could provide the maximum supply water temperature of 90°C.
The solar thermal plant and the thermal storage are utilised first in the district heat production. If those are insufficient to cover the network demand the second production unit is selected. There was a constrain that storage must be 50% full by the end of the each 24 h period.
The selection is based on the production cost, i.e. electricity and fuel costs. If the profit from the heat pump is higher than that of the CHP plant, i.e. the electricity price is low, the heat pump is utilised first. The boiler is never selected in the first selection step, as the production cost of CHP or heat pump is always lower than that of heat only boiler.
If the first generator selected is insufficient to cover the remaining heat demand the other of the first two option (i.e. CHP or heat pump) or heat only boilers is selected. If the electricity price is high, and the CHP was selected in the first step, the heat boilers are selected before heat pump. If the electricity price is low enough, the heat pump is selected. Finally, if the production is still short from the total heat demand, the remaining heat demand is covered by storage or the remaining production unit. The operation schedule is illustrated more closely by flowchart in Ju et al. (Citation2021).
Based on the optimised combination, a specific hourly dynamic price was obtained. Price was used to calculate the total district heating energy cost for buildings, and input to the demand response control signal determination for building simulations. Moreover, CO2 emissions from the optimised production combination were also calculated which represents another factor evaluated in this study.
District heating network
Temperatures of the district heating network are important parameters in calculating the required heating water volume flow rate. The supply temperature is controlled based on the outdoor temperature and varies in between 90°C and 75°C. The return temperature is affected by the heat demand in the network. Thus return temperature varies from 50°C to 35°C based on the outdoor temperature. Control curve of the supply water temperature and level of the optimal return water temperature are shown in . Figure illustrates that temperature difference reduces during the midseason but is almost constant at 40°C throughout the year. Thus 40°C (ΔT) is utilised in this study.
Figure 6. District heating supply temperature and the optimal maximum return temperature of the district heating network of Hamburg.

The actual energy consumption data utilised in this study was gathered from 2017 to 2018. The average annual heat demand for actual apartment buildings, cultural centres, and office buildings are 3444, 721 and 3735 MWh respectively which totals 7900 MWh for the whole DH network. Simulated annual DH energy demands for single buildings were 480.2, 457 and 280.1 MWh respectively. Based on these, 7 apartment buildings, 2 cultural centres and 13 office buildings were used in this study, to match the simulated network closely to the actual heating consumption of the buildings. The total DH energy demand of the simulated network is 7916.7 MWh, and deviation of the simulated network’s heat demand from that of the actual network is 0.21%.
District heating base and energy costs
Base cost
The price structure of the district heating used in this study is the actual price structure of the district heating in Hamburg. The price structure consists of a construction fee, an annual base fee and energy costs. The price structure is presented in . The construction fee is a one-time payment when a building is connected to the district heating network and is based on the design water volume flow rate. The construction fee is neglected in this study as the buildings are existing buildings. The annual base fee is based on the maximum water volume flow rate, and it includes taxes and emission costs. The energy cost is based on the energy consumption of the building. The energy price is the dynamic district heating price generated by the production optimisation.
Table 5. Price structure of the distritct heat.
The maximum water volume flow rate for the base fee calculation is derived from the actual realised peak power of the simulated building, including domestic hot water heating. The maximum flow rate is calculated by Equation (2)
(2)
(2) where
is the volumetric flow rate
,
is the maximum heating power
,
is the specific heat of water
is the density of water
, and
is the temperature difference of the district heating supply and return water
.
The maximum realised heating power is obtained from the building simulation. The temperature difference
used in the calculation is 40 K as shown in .
Dynamic DH price
Dynamic district heating cost is defined based on the operation schedule of the generation unit for each hour. Consumer district heating price is then defined based on the hourly production cost of the district heat and the real consumer district heating price.
The total production cost was calculated as shown in Equation (3)
(3)
(3) where
is the district heating production cost, €;
is the total expenses of the production (fuels and electricity), €; and
is the revenue from selling the electricity produced by CHP unit, €.
Total expenses, , are calculated as presented in Equation (4)
(4)
(4) where
is the fuel demand for generation, MWh;
is the price of the fuels, €/MWh;
is the consumed grid electricity, MWh; and
is the grid electricity price, €/MWh. The CHP unit is fuelled by biogas and the German government pays subsidies for that. The DH company receives a bonus for using the biogas. Thus the production cost can be negative if the revenue from the electricity sales and the bonuses is higher than the fuel and grid electricity costs.
The fuels demand is calculated as presented in Equation (5)
(5)
(5) where
is the fuel demand for each production unit per hour, MWh;
is the generated heat of each unit per hour, MWh; and
is the efficiency of each unit.
The CO2 emissions from the production are calculated based on the used fuel as in Equation (6)
(6)
(6) where
is the amount of CO2 emissions per hour, t (tonne); and
is the specific emission of the fuels used by each generation unit, t/MWh. For biogas, used in CHP, specific emission was set to 0 t/MWh. Specific emission for the natural gas used in boilers was set to 0.182 t/MWh based on the German law (BMU Citation2020). Electricity used by the heat pumps was assumed to be certified renewable electricity, and the specific emission was set to 0 t/MWh, thus electricity consumption did not produce CO2-emissions.
The revenue from the government bonus for feeding the renewable electricity to the grid is calculated as in Equation (7)
(7)
(7) where
is the revenue per hour for selling electricity including the government subsidy, €;
is the CHP electricity generation per hour, MWh;
is the current electricity market price, €/MWh;
is the subsidy from selling renewable electricity, €; and
is the average electricity price of the previous month, €/MWh.
The district heating production cost is minimised by the HGSO tool. The hourly heat demand of the studied community and revenue from sold electricity was input to the tool. The tool optimises the production cost for 24 h at a time by calculating hourly production cost and summing them as in Equation (8)
(8)
(8) where
is the production cost of particular 24 h, €; G is the used set of generation units; and
is the expense for each generation unit per hour, €; and
is the revenue per hour from selling the CHP electricity production including the government subsidy, €.
Then the HGSO calculated the specific production cost per hour according to the optimised production cost of the 24 h. The hourly production cost is calculated as in Equation (9)
(9)
(9) where
is the hourly specific production cost, €/MWh;
is the total production cost per hour, €; and
is the hourly district heating demand of the community, MWh.
Heat demand was covered every hour by the combination of the generation units, solar thermal energy, and the thermal storage as shown in Equation (10)
(10)
(10) where
is the generated heat by each unit per hour, MWh;
is the charged or discharged heat to/from the storage, MWh; and
is the heat production of the solar thermal unit per hour, MWh.
The thermal storage is charged or discharged every hour. It can only be charged or discharged at the same hour. The heat from storage to use or from production to storage is calculated as in Equation (11)
(11)
(11) where
is the discharge from the storage per hour, MWh;
is the charged heat into the storage per hour, MWh; and
is the charge or discharge efficiency. When storage is discharging
is 0 and vice versa.
The output of the optimisation is the production costs for every hour of the year. To transfer these into dynamic consumer prices, they were normalised to fit the real consumer price which is 91.2 €/MWh .
The total range of specific production costs (R) is defined by Equation (12)
(12)
(12) where is the total cost range of specific production cost in the simulated year, €/MWh;
is the minimum, €/MWh; and
is the maximum of the specific production costs, €/MWh. The price normalisation factor (F), which is used to calculate the specific normalised consumer price, is calculated by Equation (13)
(13)
(13) where
is the price normalisation factor; and
is the real DH price, €/MWh.
Specific normalised consumer price at hour t is then calculated by Equation (14)
(14)
(14) where
is the DH consumer price at hour t, €/MWh.
presents the dynamic DH price used in this study.
Table 6. Main parameters of the dynamic DH consumer price of the studied production scenario.
Results
Simulation cases
Building simulations consist of five different cases for each building type, thus total of 15 different cases. Simulations cases are presented in . Cases are identical in terms of the input settings for each building type. Each has one reference case which was simulated without demand response control and peak power limiting. In addition, one case was simulated with demand response control without peak power limiting. Last three were simulated with demand response and peak power limiting of 80%, 70% and 60% respectively. Percentage describes the level of available heating power whereas 100% would be unlimited and realised power from the reference case.
Table 7. Building simulation cases.
In addition to the building simulations, the district heating network level results were investigated. In network level simulations, the effect of the peak power limiting was compared to the utilisation of demand response only. The network level simulation combinations are shown in . The peak power limiting magnitude was chosen for each building type based on the building simulations, so the DH cost saving is the highest while maintaining acceptable indoor thermal environment.
Table 8. District heating network level simulation combinations. Quantity of each building type, demand response and peak power limiting setup.
Building simulation results
The effect of demand response and peak power limiting to the building’s heating power and energy demand
In this section, the effect of the demand response and peak power limiting to the total annual district heating energy demand and peak power are presented.
illustrate the power duration curves of the space and ventilation air heating of the occupied hours. Domestic hot water is not included in the graphs as only the effect of studied measures, demand response and peak power limiting, is presented.
Tables 12–14 present the peak power of space and ventilation heating, the total district heating peak power and total district heating energy consumption. The demand response and peak power limiting affect the space heating and ventilation air heating only. The total district heating peak power varies of the set peak limit as it includes the domestic hot water. Moreover, the set peak limit is based on the realised peak power of the reference cases. The district heating costs are determined by the total peak power and total energy demand thus those are presented separately along with the peak power limit setting.
Apartment building
The annual space heating power, which includes the natural ventilation’s supply air heating, of each case is shown in . It presents the duration curve of the space heating power of the heating season and shows the effect of the demand response and peak power limiting to the space heating power.
Figure 7. Duration curves of the space heating power in apartment building cases in the heating season.

Peak power limiting is effective more in the apartment than in the other building types as the apartment building is expected to be occupied every hour of the year in the simulations. Thus also nighttime hours are accounted when it is usually colder, and peak power limit comes effective more.
Daily space heating curves of one of the coldest days of apartment building simulations are shown in .
Figure 8. Hourly space heating demand in apartment building on one of the coldest days of the simulation year.

illustrates that peak power limiting is more effective and generates more additional DH energy savings in the apartment building, which is continuously used and where there is no mechanical ventilation which would be operated based on a schedule. Building is expected to be occupied in the nighttime also and natural ventilation is expected to operate with same air flow rate continuously.
The peak power limit, time peak power limiting is effective, total district heating peak power and energy demand including domestic hot water heating of the simulated apartment building cases are shown in .
Table 9. Annual power and energy results of the apartment building cases. Power limit time indicates the occupied hours that the peak limit takes place, and the time percentage indicates the share of the occupied hours.
Demand response has the lowest effect to the total district heating energy consumption in the apartment among the studied buildings. This is mainly due to the stable power demand of the building as the building is in constant use and is not equipped with mechanical ventilation, which would make the ventilation heating demand more variable.
shows that the time that the heat demand is high enough for the peak power limiting to become effective is minor. The results shows that the required peak power reserve is high compared to the actual required peak power and the time maximum power output is utilised. Peak power limiting of 60% reduces the total district heating peak power up to 34.2% whilst total DH energy demand decreases 4.5%.
reveals that when applying the peak power limit of 80% in addition to the demand response, the total DH peak power decreases about fourfold more. However, the total DH energy demand decreases only 0.2% more. This indicates that peaks are short in terms of time, and the peak power limiting of 80% does not generate much more energy savings. Further appliance of peak power limiting, to 70% and 60%, does not increase the total DH energy demand in contrast to the peak power limit. The maximum simulated peak power limit results in more than eightfold decrease in total DH peak power compared to the demand response control. However, the total DH energy saving is less than doubled. Peak power limiting does not increase the annual total DH energy saving significantly in addition to that of demand response. This is due to the limited and short periods of time that the peak power limiting takes place.
Cultural centre
illustrates the duration curves space and ventilation heating power in the cultural centre simulations of the occupied hours in the heating season. It presents the effects of the demand response and peak power limiting to the heating power in the occupied time of the heating season.
Figure 9. Duration curves of the space and ventilation heating power of occupied hours in the cultural centre case in the heating season.

The peak power limit effect is not as clear as in apartment building cases. This is due to the less occupied hours, as the cultural centre is not occupied during the nights when the lowest outdoor temperatures time of the day occur. Thus limit is not effective as many hours as in apartment building.
presents the space and ventilation heating power of one of the coldest days of the cultural centre simulations.
Figure 10. Hourly space and ventilation heating demand in cultural centre on one of the coldest days of the simulation year.

illustrates that the peak power limiting is not as effective as in apartment building as mechanical ventilation is not operated continuously at the same air flow rate. Moreover, heating power curves show that there is a rebound effect in the peak power limiting cases in the nighttime, when the main AHU is shut down, and more power is utilised by the space heating to maintain the indoor air temperature at setpoint.
presents the annual peak power limit, effective time of the limit, total DH peak power and total DH energy consumption of the cultural centre cases.
Table 10. Annual power and energy results of the cultural centre cases. Power limit time indicates the occupied hours that the peak limit takes place, and the time percentage indicates the share of the occupied hours.
shows that the peak power limiting does not affect the cultural centres power demand as much as that of apartment building. The peak power limiting cases with limit of 80%, 70%, and 60% of the reference case peak power effect 0.2%, 0.7%, and 2.7% of the annual occupied hours respectively.
Demand response has less effect on the total DH peak power than in the apartment building case. However, demand response provides more savings in the total DH energy demand than in apartment case. This is due to the more intermittent use of the cultural centre. The changes in internal gains and scheduled operation of the ventilation create more potential time for the demand response to generate the savings. Thus demand response has more effective hours than in the apartment building. However, the demand response in the nighttime does not affect the actual peak power, as the ventilation is not operating.
The results indicate that the peak power limiting has less effect to the total DH peak power than in apartment building. Ventilation is not operated during the night, when the outdoor temperature is coldest. Shutting down the ventilation system for the night reduces the power demand when it would be the highest, thus the potential peak shaving time of power limiting is reduced.
Office building
illustrates the effect of the demand response and peak power limiting to the space and ventilation heating power in the office building during the occupied time of the heating season.
Figure 11. Duration curves of the space and ventilation heating power of occupied hours in the office building cases in the heating season.

In the office building simulations, the peak power effective time is less than in the cultural centre simulations due to the less occupied hours.
presents the annual peak power limits, time that peak power limit is effective, total DH power, and total district heating energy demand of the office building simulations.
Table 11. Annual power and energy results of the office building cases. Power limit time indicates the occupied hours that the peak limit takes place, and the time percentage indicates the share of the occupied hours.
In the office building cases, the demand response decreases the total DH peak power by 1.9% which less than in cultural centre case. Applying the peak power limiting decreases the peak power up to 13-fold compared to the demand response only. However, the energy consumption is not further decreased by the peak power limiting compared to the demand response. Office building is not occupied, and the ventilation is not operated during the nights and the weekends which decreases the effect of the peak power limiting. Moreover, there are no internal heat gains during the weekends thus the space heating consumption is higher during that time.
Office building has a total of 2000 occupied hours annually. Peak power limits of 80%, 70%, and 60% resulted in affecting 0.1%, 0.4%, and 1.2% of occupied hours respectively. The peak power limiting of 70% and 60% slightly decreases the annual total DH energy saving in contrast to the demand response case (OB-DR). This is due to the rebound effect that occur when the ventilation system is turned off after the office hours.
and illustrate the rebound effect which are based on one of the coldest days of the year. presents the hourly space and ventilation heating power of the office building cases. presents the indoor air temperatures of the coldest room in the office building cases.
Figure 12. Hourly space and ventilation heating demand in office building on one of the coldest days of the simulation year.

Figure 13. Hourly indoor air temperature in office building simulation in one of the coldest days of the year.

Peak power limiting affects the space and ventilation heating. When the ventilation heating power drops, the space heating has more power reserve to utilise, and it starts to compensate if the indoor air temperature is below the setpoint. This offsets the saving generated during the day when peak power limiting is effective. shows that after 6 pm when the ventilation system is shut down, the space heating power is highest in the OB-DR-PPL70 and OB-DR-PPL60. However, in OB-DR and OB-DR-PPL80 the heating powers do not have significant difference between each other even though the heating power in those is a little higher than that of in OB-R-21. This is due to the lower indoor air temperature in the OB-DR-PPL70 and OB-DR-PPL60 cases as shown in , and space heating power increases to room temperature to achieve the setpoint. shows that in OB-DR and OB-DR-PPL80 the indoor air temperature does not decrease below the setpoint significantly during the day and space heating has less to compensate thus there is less power rebound.
Comparing and , heating power curves show that rebound effect is not as notable in cultural centre as in office building. The share of ventilation heating power of the total is higher office building thus operation changes of the AHUs have higher impact. Moreover, the internal gains and occupant density are higher in cultural centre than in office building and indoor air temperature does not decrease as much during the day.
Monetary savings in district heating by demand response and peak power limiting
Apartment building
presents the annual DH cost results of the apartment building simulations. The energy and base cost savings are directly proportional to the total DH energy and power reduction achieved by demand response and peak power limiting. In apartment building cases, peak power limiting represents higher share of the saving in each case. Demand response provides 2.8% saving in energy cost and 4.1% saving in base cost, which account for 3.4% saving in total DH cost. Demand response and peak power limiting of 60% generate up to 34.1% saving in the DH base cost but the DH energy cost saving is 4.6%. Total DH cost saving is 16.7% which is about eightfold compared to that of demand response case. The energy cost saving does not increase as significantly due to the peak power limiting’s finite effect on the energy consumption decrease.
Table 12. Annual district heating energy, base and total DH cost savings in apartment building cases.
In peak power limiting cases, the base cost saving clearly presents the higher share of the total achieved saving. The additional energy cost saving compared to the demand response case (AB-DR) is limited as the peak power hours are minor.
Cultural centre
presents the DH cost results of the cultural centre simulations. Demand response case (CC-DR) achieved total DH cost saving of 3.3% of which the energy cost saving represents 4.1% and base cost saving 2.5%. Peak power limiting increases the energy cost saving from 0.1 to 0.4%-p, and base cost saving from 11.3 to 24.8%-p.
Table 13. Annual district heating energy, base and total DH cost savings in cultural centre cases.
In cultural centre cases, the additional DH energy cost saving of the peak power limiting is less than in apartment building simulations. Apartment buildings are expected to be operated similarly through the year, also in the winter nights when the space heating demand is highest and power limiting has more effect. Cultural centre is not occupied in the nighttime and air handling units are not operated thus the energy saving benefit from the peak power limiting is less when the potential is highest. Moreover, the rebound effect generated by the peak power limiting also slightly decreases the potential saving achieved by the power limit as described earlier.
Total cost saving of the peak power limiting cases range from 8.9% to 15.6%. Additional saving to demand response case is mostly due to the base cost reduction. The occupation time of the building affects the effect of the peak power limiting and amount of the energy cost saving.
Office building
presents the DH cost results in office building simulations. Demand response case (OB-DR) resulted in 2.8% total DH cost saving. DH energy cost saving was 4.8% and base cost saving 1.6%. The energy cost saving in the case OB-DR is the highest among the three building types. This results from the lack of occupation and ventilation heating in the weekends. As the demand response affects the space heating only, the relative savings are higher when the ventilation operation time is reduced.
Table 14. Annual district heating energy, base and total DH cost savings in office building cases.
Case OB-DR-PPL80 resulted in 12% total DH cost saving of which energy cost represents 4.8% and base cost 16.4%. Applying more peak power limiting (70% & 60%) the energy cost saving decreases a little more. This is due to the more intermittent usage of the office building and its ventilation system than the other two building types. Rebound effect caused by shutting down of the ventilation system affects the saving of the peak power limiting in contrast to the demand response only. Office building is not occupied during the weekends and the ventilation is not operating thus the effect of the peak power limiting is less.
Overall, demand response has more effect on the energy cost in the cultural centre and office building due to the intermittent use. In the office building, the ventilation is not operated during the weekends thus the demand response has higher relative change on the total heating demand and cost. The peak power limiting has the highest effect on the apartment building total energy cost due to the constant use of the building. Moreover, in the apartment building, demand response has highest base cost reduction as the demand response has more relative effect on the power demand due to the lack of separate ventilation heating.
The effect of the demand response and peak power limiting to the indoor air temperature
Maintaining acceptable indoor climate is the first priority when demand response actions are applied. In this section, the effective time of the peak power limiting of the annual occupied hours, and the demand response’ and peak power limiting’s effect on the indoor air temperature are analysed. Only occupied hours are analysed. Furthermore, the coldest occupied room is investigated for each building.
The demand response used in the simulation cases does not reduce the thermal comfort significantly. The indoor air temperature stays reasonably well above the lower minimum of acceptable indoor temperature range. The indoor air setpoint variations due to the demand response are shown in .
Table 15. Indoor air setpoint variation in hours
Results show that indoor air setpoint is 20°C 64.6% and 21°C 35.4% of the year. There are no charging hours when the setpoint would be 23°C. This is due to the high marginal value (75 €/MWh) of the control signal, which decreases the charging hours and increases the cost savings. Lower marginal value makes the control signal more sensitive to the changes of the hourly energy price and reduces the cost savings.
Apartment building
presents the indoor air results of the apartment building simulations. In the reference case, AB-R-21, the indoor air falls below the setpoint temperature of 21°C for 113 h which accounts for 10°Ch. In the demand response case AB-DR the temperature falls below 21°C for 3275 h and 1512°Ch. However, indoor air temperature stays reasonably well above the lower limit as it falls below 20°C for 8 h and 1°Ch.
Table 16. The effect of demand response and peak power limiting to indoor air temperature in the coldest occupied room in the apartment building simulation.
In the case AB-DR-PPL80, peak power limiting affects for 1.4% of annual occupied hours. This means that for 1.4% of the year the heat demand is higher than the supply from the district heating to the building. This results indoor air temperature to fall below acceptable level (20°C) for 47°Ch. Result indicates that on average, the realised peak power of the reference case is high for the measured outdoor temperatures during the simulation period, as only 1.4% of the year the demand is higher than 80% of that power.
When peak power limiting of 70% and 60% are applied the respective time and degree hours are higher as seen in , but temperature also falls below 18°C. The minimum temperature in AB-DR-PPL60 is below 15°C. This low indoor air temperatures are not considered acceptable as they decrease thermal comfort of the occupants and may cause health symptoms.
Figure 14. Duration curves of the indoor air temperature in the coldest occupied room during the coldest 800 h in the apartment simulation cases.

In the apartment building, the peak power limit is effective for relatively highest time of the occupied hours among the simulated buildings. This is due to the constant use of the building as the space heating demand is highest during the nighttime when the outdoor temperature is coldest.
Cultural centre
presents the indoor air temperature results of the cultural centre cases during the occupied hours of the year. In the reference case (CC-R-21), indoor air temperature always stays above the setpoint. In demand response case CC-DR, temperature falls below 21°C for 1342 h which results in 523°Ch. Temperature stays above the acceptable minimum temperature (20°C) the whole year during the occupied hours.
Table 17. The effect of demand response and peak power limiting to indoor air temperature in the coldest occupied room in the cultural centre simulation.
illustrates the duration of the indoor air temperature during the coldest occupied hours in the coldest room of the cultural centre. That indicates that in the peak power limiting cases the indoor air temperature falls below the acceptable level. However, in the CC-DR-PPL80 the time indoor temperature maintains below 20°C is so short it does not result in degree hours. In the case with peak power limiting of 70% (CC-DR-PPL70) the limit takes place for 0.7% of the annual time. Temperature falls below acceptable level for 3°Ch. Thus 80% and 70% peak power limiting maintain the acceptable indoor air temperature and occupant’ thermal comfort moderately well.
Figure 15. Duration curves of the indoor air temperature in the coldest occupied room during the coldest 100 h in the cultural centre simulation cases.

In the case CC-DR-PPL60, limiting affects for 2.7% of the time. Indoor air temperature falls below 19°C for 3°Ch during the occupied time.
Peak power limiting has the least effect on the indoor air temperature in the cultural centre among the studied buildings regarding the time and degree hours below the acceptable level. This indicates that the thermal mass and structural design is suitable for the peak power limiting. Moreover, the internal gains and the occupant density are higher than in apartment and office buildings. Thus the indoor air temperatures fall slower than in other building types when peak power is limited.
Office building
shows the indoor air temperature results of the coldest room of the office building simulations. Reference case in office building simulations (OB-R-21) has indoor air temperature to fall below 21°C for 20 h and 2°Ch in a year. In the demand response case of the office building simulations (OB-DR), the temperature falls below 21°C for 1005 h and 491°Ch. However, temperature stays above the lower limit of the acceptable indoor air temperature for the occupied hours.
Table 18. The effect of demand response and peak power limiting to indoor air temperature in the coldest room of the office building simulation.
shows the duration of the indoor air temperature in the coldest room of the office building simulations. Results show that the structural design and thermal mass of the office building are less suitable for the peak power limiting than those of cultural centre. Even though the office building has about 50% less affected hours of peak power limiting, the hours below the studied temperatures are more than doubled in each peak power limiting case.
Figure 16. Duration curves of the indoor air temperature in the coldest occupied room during the coldest 100 h in the office building simulation cases.

Demand response maintains the indoor temperature and thermal comfort well in each building type. The indoor temperature does not fall below acceptable level in any building. The effect of peak power limiting varies more among the different building types. In the apartment building, the effect on the thermal comfort is the highest, as the occupied hours include the coldest nighttime. Cultural centre and office building have less effect as the occupied hours are limited. Office building has less internal gains (equipment and lighting) and lower occupant density than cultural centre which makes the indoor air temperature to decrease more during the peak limiting hours than in cultural centre.
The effect of demand response and peak power limiting to the district heating production and network
The effect of the demand response and peak power limiting was studied in the community level as part of the co-simulation process. Annual district heating power demand of the community in different cases is shown in and . illustrates the annual hourly district heating power demand.
Figure 18. Duration curve of the district heating system’s total power demand of the highest demand hours. The effect of the peak power limiting is illustrated by hatched area.

illustrates that the demand response reduces the total peak power of the DH community by 4.9%. Peak power limiting reduces the peak power by additional 17.8%-p which totals in 22.8% reduction in peak power in contrast to the reference case. However, the additional energy savings are neglectable as the affected time is limited.
shows the duration of the total district heating power during the highest demand hours (200 h) of the simulation year. It is seen that the total time that the peak power limiting is effective in the community level is less than 24 h.
presents the annual results of the district heating network level. Total district heating production, total generation cost, total emissions, specific generation cost and average generation price are presented. Average generation price represents the average of the hourly specific costs.
Table 19. Network level demand response and peak power limiting results. Difference is stated relative to the reference case for DR and DR&PPL cases separately.
Demand response control reduces the total district heating production by 3.8% compared to the reference case. This results in 5.9% profit increase for the district heating producer in contrast to the reference case. Profit increases due to the reduced operation hours of less profitable production units. The CO2 emissions from the production are decreased by 32.3% due to the demand response, as higher emitting production units are operated less.
When peak power limiting is applied to the buildings of the community as described in the total district heating generation is decreased by 0.1% in addition to the decrease by demand response. Total generation cost decreases by 0.2%. This accounts in 0.2% more profit to the DH producer. Furthermore, CO2 emissions of the DH production decrease by additional 1.4%. Average generation cost remains same as the production scenario is unchanged.
Peak power limiting does not provide significant additional savings in energy production and CO2 emissions or increment in the producer’s profit in comparison to the demand response. This is due to the limited additional energy savings peak power limiting provides at the building level as the time that limit becomes effective is minor. The benefit of peak power limiting at the community level energy savings is further reduced as most of the buildings in the simulated community are office buildings. In the DR-PPL70 cases, the effect of the peak power limiting to the cultural centre and office building energy demand was neglectable and demand was increased in the case OB-DR-PPL70 which were used for the community analysis.
Discussion
Results indicate that demand response can provide 2.8–4.7% energy savings and 2.8–3.4% total DH cost savings to the customer depending on the building type. Highest relative total DH energy saving is achieved in the office building (4.7%). Office building is suitable for the demand response as the usage is more intermittent than in other building types. Ventilation is not operated during weekends thus demand response has more relative effect as it only applies on the space heating. Moreover, the lack of the internal heat gains during the weekends increases the relative effect of the demand response. Energy saving reflects directly to the DH energy cost saving, which is the highest in the office building, which is 2.0% and 0.7% higher than in apartment building and cultural centre respectively.
However, the total DH cost saving order, including base cost, is vice-verse. Apartment building has the highest, cultural centre second and office building the least saving potential in the total DH cost. This is due to the DH base cost savings. Total DH peak power is decreased the most in the apartment building. The apartment building does not have separate ventilation heating, thus the demand response affects the total heating power excluding domestic hot water. In the office building and cultural centre part of the heating power is reserved for the ventilation heating, which the demand response does not effect, thus the relative total effect is less.
Higher DH peak power reduction by demand response in the apartment building is also due to the continuous usage of the building. As the building is used similarly during the nights when the outdoor temperature is generally lower, the demand response’s reduction to the peak power gets actualised. In the office building and cultural centre, the demand response does not affect the peak power as significantly, as the ventilation is not operated in the nighttime when the highest power demand would otherwise occur.
Peak power limiting provides the highest relative reduction on the total DH energy consumption and the peak power in the apartment building. This is as well due to the continuous usage of the building and lack of the ventilation heating. The lack of ventilation heating in apartment building makes the heating power more stable. In cultural centre and office building, the ventilation is not operated fully in the nighttime, and this already reduces the delivered peak power. Thus the peak power limiting does not have that significant effect.
Study shows that demand response can maintain the indoor air temperature within the acceptable range decently. However, the indoor air temperature falls below the normal setpoint for some time of the year, which was 21°C in this study. Peak power limiting has more effect on the indoor air temperatures, especially in the apartment building which is potentially occupied every hour of the year.
The building massing (Hootman Citation2012, 170–172) affects the heat storing properties and thermal inertia of the building and results of the DR and peak power limiting would vary among different building massing. For example, buildings with more heat transfer surface between indoor air and structures have generally better heat storing capacity, and saving potential of the DR and peak power limiting are higher. Other structural properties, such as structure types, affect the saving potential also.
Demand response provided significant CO2-emission savings in the community level. The emission reduction results from the reduced operation hours of the gas boilers. However, the peak power limiting was not able to reduce the operation hours of the boiler significantly further, thus the emission saving is minor. Emission reductions of the demand response and peak power limiting are dependent on the peak power fuels of the district heating system, which usually are high emitting fossil fuels.
The community level analysis shows that in the studied system, the peak power limiting does not provide significant additional savings to the demand response. The time that the peak power limiting is effective is minor, especially in the selected cultural centre and office building cases for the community level study. The situation might change if the studied community had different building mix, for example if the apartment buildings represented higher share of the total building stock. However, the apartment building’ thermal conditions were the most affected by peak power limiting which is a restrictive factor in the peak power limiting’s benefits.
On community level, the demand response and peak power limiting would become even more profitable for the producers if the peak power was decreased so much that producers could drop the peak power generation units from the system. The marginal cost of those units is usually high, and the annual usage hours are low. Those units require maintenance, and the fuel must be stored for the coldest times of the year, which generate the costs. However, as the usage hours are limited, they are not very profitable. This study investigated only the production cost of the district heating based on the fuel costs and did not address the fixed costs of the producers. Moreover, the issue of the possibility to remove the peak power generation units form the DH system due to the demand response and peak power limiting was not addressed.
Results of this study indicate that peak power limiting can provide significant savings for the customers in the DH base cost. However, applying peak power limiting as in this study is not trivial. In apartment buildings applying centralised peak power limiting to the district heating substation has effect on every occupier of the building. The situation is same in the office building where different tenants are affected. Thus everyone should agree on the lowered indoor air temperatures in the coldest time of the year.
However, this study indicates that the design peak power can be high for the actual outdoor temperatures. The time that the outdoor temperature drops down to the design outdoor temperature is minor. Thus it could be worthwhile to discuss, how much reserve the heating systems shall have. Especially when the annual mean outdoor temperatures are expected to rise during this the twenty-first century. However, the extreme weathers are predicted to become more common too, including cold periods. Still, the time that they take place annually is critical when designing the heating systems.
Demand response can enable novel customer-centric service models for district heating companies which have potential to benefit both heat producer and end-customer. District heating business has been traditional energy business where end-customer has little influence on the product and pricing. By demand response, selling heat could be more of a service of indoor thermal conditions. Customer could set limits of the indoor air temperature which heat producers agrees to maintain. Applied along with dynamic pricing end-user can save from heating costs as well as heat producer from production costs and usage of peak power fuels.
The study was conducted with the energy prices prior to the Russia’s was in Ukraine in 2022. The war has resulted in the rapid increase in the fuel prices, consequently increasing district heating and electricity costs. Demand response and peak power limiting could provide higher savings due to the increased costs in the energy market. The effect of the increased fuel costs on the saving potential could be studied in the further research.
Conclusion
The objective of this study was to investigate the energy and cost savings of the demand response and peak power limiting for the building owner and the district heating producer. Furthermore, the effect on the building occupants’ s thermal conditions and district heating production emissions was studied. The study was conducted as a co-simulation process, where building level simulations and district heating production level optimisation were conducted separately.
Demand response provides 2.8–4.7% energy savings and 2.3–3.4% total DH cost savings for the building owner. Indoor air temperature is kept within the chosen acceptable range despite the demand response. However, occupants should accept slight difference from the normal indoor air temperature if demand response is applied.
Peak power limiting provides additional 0.3–1.7% energy savings and 12.3–15.2% total DH cost savings to that of demand response. Apartment building has the highest energy and energy cost savings due to the peak power limiting due to the stable use and lack of mechanical ventilation system. Office building has the least additional savings due peak power limiting, and it caused the energy demand and cost slightly to increase from the demand response case due to the rebound effect. Major share of the increased savings in the total district heating cost in peak power limiting cases was due to the reduced base cost.
Lower limits for the peak power caused the indoor air temperatures to fall to unacceptable level. In apartment building, the 80% peak power limiting could maintain the indoor air temperature in the chosen acceptable level. In cultural centre and office building, the chosen acceptable level was achieved still by 70% peak power limiting. Result indicate that the design powers were high for the measured outdoor temperatures, and there is significant saving potential in base cost by reducing the peak power, without notably risking the thermal indoor conditions.
In the community level, the demand response provides 3.8% energy savings, which results in 5.9% profit increase with the studied production scenario. The CO2 emissions were reduced by 32.3% by demand response. However, the peak power limiting does not significantly increase the energy or cost savings, nor reduce the energy production emissions with studied limits in contrast to the demand response.
Further research could implement field tests on district heated buildings to verify the results empirically. Demand response’ actual indoor air temperature variations and their perception by the occupants would place interesting research questions. Furthermore, production side effects such as actual peak power and fuel savings could be studied in co-operation with the DH provider. Moreover, the dynamic pricing of the district heating could be studied further.
Acronyms
Nomenclature | ||
= | expenses of each generation unit (€) | |
Cexp. | = | district heating production expenses (€) |
Cp,24h | = | production cost of particular 24 h (€) |
Cprod. | = | district heating production cost (€) |
cp | = | specific heat of water (kJ/kg*K) |
= | fuel demand for each production unit (MWh) | |
del. | = | electricity demand for generation (MWh) |
dfuel | = | fuel demand for generation (MWh) |
= | specific emission of the fuel (t/MWh) | |
F | = | price normalisation factor (-) |
= | CHP electricity generation (MWh) | |
= | efficiency of each unit (%) | |
= | charge or discharge efficiency (%) | |
= | CO2 emissions (t) | |
n50 | = | air leakage rate (1/h) |
Pmax | = | maximum heating power (kW) |
pavr.el. | = | average electricity price of previous month (€/MWh) |
pbonus | = | government subsidy from selling renewable electricity (€) |
pDH | = | DH consumer price (€/MWh) |
pel. | = | price of electricity (€/MWh) |
pfuel | = | price of fuel (€/MWh) |
pprod. | = | specific production cost (€/MWh) |
preal | = | real DH price (€/MWh) |
ρ | = | density of water (kg/m3) |
Q | = | district heating demand of the community (MWh) |
qcharge | = | charge to the storage (MWh) |
qdischarge | = | discharge from the storage (MWh) |
qST | = | heat production of the solar thermal plant (MWh) |
qstorage | = | charged or discharged heat to/from the thermal storage (MWh) |
= | volumetric flow rate (m3/s) | |
= | generated heat of each production unit (MWh) | |
R | = | total cost range of specific production cost (€/MWh) |
Rel. | = | revenue from selling electricity (€) |
ΔT | = | temperature difference (K) |
Tavr,24out | = | moving 24-hour outdoor temperature (°C) |
Tlim,out | = | limiting outdoor temperature (°C) |
TSH,max | = | maximum space heating setpoint (°C) |
TSH,min | = | minimum space heating setpoint (°C) |
TSH,norm | = | normal space heating setpoint (°C) |
TSH,set | = | space heating setpoint (°C) |
Acknowledgement
This study is part of the Smart Pro HeaT – Smart Prosumer Heating Technologies and FINEST Twins projects. Smart Pro Heat project is funded by Business Finland and private companies Caverion Ltd., Fourdeg Ltd., Halton Ltd., and Aalto University as well as the Federal Ministry for Economic Affairs and Energy of Germany in the project; EnEff: Wäarme SmartProHeaT: Smart Prosumer Heating Technologies, Subproject: Integration of smart prosumers into smart thermal grids (Project number: 03ET1598), executed by Hamburg University of Applied Sciences. FINEST Twins project is funded by European Union (Horizon 2020 programme, Grant No. 856602) and the Estonian government.
Disclosure statement
No potential conflict of interest was reported by the author(s).
References
- Achermann, M., and G. Zweifel. 2003. RADTEST – Radiant Heating and Cooling Test Cases. A Report of Task 22. In IEA. IEA. https://task22.iea-shc.org/Data/Sites/1/publications/RADTEST_final.pdf.
- Ala-kotila, P., T. Vainio, and J. Heinonen. 2020. “Demand Response in District Heating Market—Results of the Field Tests in Student Apartment Buildings.” Smart Cities 3 (2): 157–171. https://doi.org/10.3390/smartcities3020009.
- Albadi, M. H., and E. El-Saadany. 2008. “A Summary of Demand Response in Electricity Markets.” Electric Power Systems Research 78 (11): 1989–1996. https://doi.org/10.1016/j.epsr.2008.04.002.
- Alimohammadisagvand, B. 2016. “Cost-optimal Thermal Energy Storage System for a Residential Building with Heat Pump Heating and Demand Response Control.” Applied Energy 174: 275–287. https://doi.org/10.1016/j.apenergy.2016.04.013.
- Alimohammadisagvand, B. 2017. “Influence of Energy Demand Response Actions on Thermal Comfort and Energy Cost in Electrically Heated Residential Houses.” Indoor and Built Environment 26 (3): 298–316. https://doi.org/10.1177/1420326X15608514.
- Alimohammadisagvand, B. 2018. “Comparison of Four Rule-Based Demand Response Control Algorithms in an Electrically and Heat Pump-Heated Residential Building.” Applied Energy 209: 167–179. https://doi.org/10.1016/j.apenergy.2017.10.088.
- Arabzadeh, V. 2018. “A Novel Cost-Optimizing Demand Response Control for a Heat Pump Heated Residential Building.” Building Simulation 11 (3): 533–547. https://doi.org/10.1007/s12273-017-0425-5.
- Bertelsen, N., B. Mathiesen, S. R. Djorup, N. C. A. Schneider, S. Paardekooper, L. Sánchez García, J. Z. Thellufsen, J. Kapatanakis, L. Angelino, and J. Kiruja. 2021. Integrating Low-Temperature Renewables in District Energy Systems: Guidelines for Policy Makers. International Renewable Energy Agency. In Aalborg University. https://vbn.aau.dk/ws/portalfiles/portal/406326231/Integrating_low_temperature_renewables_in_district_energy_systems_Guidelines_for_policymakers_2021.pdf.
- BMU (Federal Ministry for the Environment, N. C. and N. S. 2020. Gesetz zur Einsparung von Energie und zur Nutzung erneuerbarer Energien zur Wärme-und Kälteerzeugung in Gebäuden*(Gebäudeenergiegesetz - GEG)- Anlage 9 (zu § 85 Absatz 6)-Umrechnung in Treibhausgasemissionen. (Act on the Saving of Energy and the Use of Renewable Energies for Heating and Cooling in Buildings* (Building Energy Act - GEG)-Annex 9 (to § 85 18 Science and Technology for the Built Environment paragraph 6)-Conversion into greenhouse gas emissions). In Gebäudeenergiegesetz - GEG (Anlage 9 (zu § 85 Absatz 6)). https://www.gesetze-im-internet.de/geg/anlage_9.html.
- Bring, A., P. Sahlin, and M. Vuolle. 1999. Models for Building Indoor Climate and Energy Simulation. A Report of IEA Task 22. IEA.
- Cai, H., S. You, and J. Wu. 2020. “Agent-based Distributed Demand Response in District Heating Systems.” Applied Energy 262: 114403. https://doi.org/10.1016/j.apenergy.2019.114403.
- Christidis, A. C. 2019. Thermische Speicher zur Optimierung des Betriebs von Heizkraftwerken in der Fernwärmeversorgung [Thermal storage for the Optimisation of The Operation of Combined Heat and Power Plants in District Heating Applications].
- Connolly, D., H. Lund, B. v Mathiesen, S. Werner, B. Möller, U. Persson, T. Boermans, D. Trier, P. A. Østergaard, and S. Nielsen. 2014. “Heat Roadmap Europe: Combining District Heating with Heat Savings to Decarbonise the EU Energy System.” Energy Policy 65: 475–489. https://doi.org/10.1016/j.enpol.2013.10.035.
- Delmastro, C., F. Briens, M. Husek, and R. Martinez-Gordon. 2022. District heating. District Heating. https://www.iea.org/reports/district-heating.
- Deutschen Wetterdienst, (DWD). 2017a. Ortsgenaue Testreferenzjahre von Deutschland für mittlere, extreme und zukünftige Witterungsverhältnisse. Deutschen Wetterdienst (DWD). https://www.bbsr.bund.de/BBSR/DE/forschung/programme/zb/Auftragsforschung/5EnergieKlimaBauen/2013/testreferenzjahre/try-handbuch.pdf?__blob=publicationFile&v=3.
- Deutschen Wetterdienst, (DWD). 2017b. Ortsgenaue Testreferenzjahre von Deutschland für mittlere und extreme Witterungsverhältnisse. In Deutscher Wetterdienst (DWD). Deutscher Wetterdienst (DWD). https://www.bbsr.bund.de/BBSR/DE/forschung/programme/zb/Auftragsforschung/5EnergieKlimaBauen/2013/testreferenzjahre/try-projektbericht.pdf?__blob=publicationFile&v=3.
- Dominković, D. F., M. Wahlroos, S. Syri, and A. S. Pedersen. 2018. “Influence of Different Technologies on Dynamic Pricing in District Heating Systems: Comparative Case Studies.” Energy (Oxford) 153: 136–148. https://doi.org/10.1016/j.energy.2018.04.028.
- Eissa, M. M. 2011. “Demand Side Management Program Evaluation Based on Industrial and Commercial Field Data.” Energy Policy 39 (10): 5961–5969. https://doi.org/10.1016/j.enpol.2011.06.057.
- EQUA. 2010. “Validation of IDA Indoor Climate and Energy 4.0 with Respect to CEN Standards EN 15255-2007 and EN 15265-2007.” In EQUA Simulation AB. EQUa Simulation AB.
- Eseye, A. T., M. Lehtonen, T. Tukia, S. Uimonen, and R. J. Millar. 2019. Exploiting Flexibility of Renewable Energy Integrated Buildings for Optimal Day-ahead and Real-time Power Bidding Considering Batteries and EVs as Demand Response Resources.
- European Commission. 2021. “Fit for 55”: delivering the EU’s 2030 Climate Target on the way to climate neutrality. In European Commission. https://eur-lex.europa.eu/legal-content/EN/TXT/?uri=CELEX%3A52021DC0550.
- Forrest, J., and R. Lougee-Heimer. 2005. CBC User Guide. https://pubsonline.informs.org/doi/10.1287educ.1053.0020.
- Guelpa, E., and V. Verda. 2021. “Demand Response and Other Demand Side Management Techniques for District Heating: A Review.” Energy (Oxford) 219: 119440. https://doi.org/10.1016/j.energy.2020.119440.
- Hamilton, I., H. Kennard, O. Rapf, J. Kockat, and S. Zuhaib. 2020. The Global Status Report for Buildings and Construction. United Nations. https://globalabc.org/sites/default/files/inline-files/2020%20Buildings%20GSR_FULL%20REPORT.pdf.
- Henrikkson, J. 2018. Investigation of the Improvement Potential of Heat Load Forecasts in BoFiT. Stockholm: Royal Institute of Technology.
- Hootman, T. 2012. “Net Zero Energy Design: A Guide for Commercial Architecture. John Wiley & Sons.” Incorporated.
- HRE4. 2017. Heating and Cooling: Facts and Figures. https://www.euroheat.org/wp-content/uploads/2017/07/29882_Brochure_Heating-and-Cooling_web-1.pdf.
- IEA. 2019. How Can District Heating Help Decarbonise the Heat Sector by 2024? https://www.iea.org/articles/how-can-district-heating-help-decarbonise-the-heat-sector-by-2024.
- IEA. 2020. Tracking Buildings 2020. In IEA. https://www.iea.org/reports/tracking-buildings-2020.
- Ji, H. Y., R. Baldick, and A. Novoselac. 2014. “Dynamic Demand Response Controller Based on Real-Time Retail Price for Residential Buildings.” IEEE Transactions on Smart Grid 5 (1): 121–129. https://doi.org/10.1109/TSG.2013.2264970.
- Ju, Y., J. Jokisalo, R. Kosonen, V. Kauppi, and P. Janßen. 2021a. “Analyzing Energy Flexibility by Demand Response in a Finnish District Heated Apartment Building E3S Web of Conferences Cold climate HVAC & Energy 2021. Tallinn, Estonia.18.4.2021.
- Ju, Y., J. Jokisalo, R. Kosonen, V. Kauppi, and P. Janßen. 2021b. “Analyzing Power and Energy Flexibilities by Demand Response in District Heated Buildings in Finland and Germany.” Science & Technology for the Built Environment 27 (10): 1–28. https://doi.org/10.1080/23744731.2021.1950434.
- Ju, Y., J. Lindholm, M. Verbeck, J. Jokisalo, R. Kosonen, P. Janßen, Y. Li, H. Schäfers, and N. Nord. 2021. “Cost Savings and CO2 Emissions Reduction Potential in the German District Heating System with Demand Response.” Science & Technology for the Built Environment 28 (2): 1–27. https://doi.org/10.1080/23744731.2021.2018875.
- Knudsen, M. D., and S. Petersen. 2017. “Model Predictive Control for Demand Response of Domestic hot Water Preparation in Ultra-low Temperature District Heating Systems.” Energy and Buildings 146: 55–64. https://doi.org/10.1016/j.enbuild.2017.04.023.
- Kontu, K., J. Vimpari, P. Penttinen, and S. Junnila. 2020. “Individual Ground Source Heat Pumps : Can District Heating Compete with Real Estate Owners’ Return Expectations?” Sustainable Cities and Society 53, https://doi.org/10.1016/j.scs.2019.101982.
- Kropf, S., and G. Zweifel. 2001. Validation of the Building Simulation Program IDA-ICE According to CEN 13791. In Hochschule Für Technik + Architektur Luzern. http://www.equaonline.com/iceuser/validation/ICE_vs_prEN%2013791.pdf.
- Li, H., J. Song, Q. Sun, F. Wallin, and Q. Zhang. 2019. “A Dynamic Price Model Based on Levelized Cost for District Heating.” Energy, Ecology and Environment 4 (1): 15–25. https://doi.org/10.1007/s40974-019-00109-6.
- Lu, Z., and L. Shumei. 2018. The Status Analysis and Development Outlook of the Regional Multi-Energy System (RMES). https://doi.org/10.1109/CIEEC.2018.8745736.
- Martin, K., J. Jokisalo, I. korkeakoulu, R. Kosonen, and A. Aalto-yliopisto, & University. 2017. Demand Response of Heating and Ventilation within Educational Office Buildings. https://aalto.finna.fi/Record/aaltodoc.123456789_29149.
- Mathiesen, B., N. Bertelsen, N. Schneider, L. Sánchez-García, S. Paardekooper, J. Z. Thellufsen, and S. R. Djorup. 2019. Towards a Decarbonised Heating and Cooling Sector in Europe: Unlocking the potential of energy efficiency and district energy. https://vbn.aau.dk/ws/portalfiles/portal/316535596/Towards_a_decarbonised_H_C_sector_in_EU_Final_Report.pdf.
- Merkert, L., A. Haime, and S. Hohmann. 2019. “Optimal Scheduling of Combined Heat and Power Generation Units Using the Thermal Inertia of the Connected District Heating Grid as Energy Storage.” Energies (Basel) 12 (2): 266. https://doi.org/10.3390/en12020266.
- Mikola, A. 2017. “Performance of Ventilation in Estonian Apartment Buildings.” Energy Procedia 132: 963–968. https://doi.org/10.1016/j.egypro.2017.09.681.
- Moczko, D. 2019. District Energy in Germany. https://www.euroheat.org/knowledge-hub/district-energy-germany/.
- Moinard, S., and G. Guyon. 1999. Empirical Validation of EDF ETNA and GENEC Test-Cell Models. A Report of Task 22. In IEA. IEA. http://www.mojo.iea-shc.org/data/sites/1/publications/Final_report.pdf.
- Muller, T. 2018. “Demand Response Potential: Available When Needed?” Energy Policy 115: 181–198. https://doi.org/10.1016/j.enpol.2017.12.025.
- Paiho, S., and H. Saastamoinen. 2018. “How to Develop District Heating in Finland?” In Energy Policy 122: 668–676. https://doi.org/10.1016/j.enpol.2018.08.025.
- Peltokorpi, A., M. Talmar, K. Castrén, and J. Holmström. 2019. “Designing an Organizational System for Economically Sustainable Demand-Side Management in District Heating and Cooling.” Journal of Cleaner Production 219 (10): 433–442. https://doi.org/10.1016/j.jclepro.2019.02.106.
- Popovski, E., A. Aydemir, T. Fleiter, D. Bellstädt, R. Büchele, and J. Steinbach. 2019. “The Role and Costs of Large-Scale Heat Pumps in Decarbonising Existing District Heating Networks – A Case Study for the City of Herten in Germany.” Energy (Oxford) 180: 918–933. https://doi.org/10.1016/j.energy.2019.05.122.
- ProCom GmbH. 2020. BoFiT. https://procom-energy.de/en/tag/bofit/.
- ProCom GmbH. 2021. BoFiT Optimization. https://procom-energy.de/en/products/bofit-optimization/.
- Safdar, M., G. A. Hussain, and M. Lehtonen. 2019. Costs of Demand Response from Residential Customers’ Perspective.
- Seppänen, O., N. Brelih, G. Goeders, and A. Litiu. 2012. Health Based Ventilation Guidelines for Europe. Existing Buildings, Building Codes, Ventilation Standards and Ventilation in Europe. In REHVA.
- SFS EN 13779. 2007. Ventilation for non-Residential Buildings. Performance Requirements for Ventilation and Room-Conditioning Systems. Helsinki: Finnish Standards Association SFS.
- SFS-EN 16798-1. 2019. Energy performance of buildings. Ventilation for buildings. Part 1: Indoor Environmental Input Parameters for Design and Assessment of Energy Performance of Buildings Addressing Indoor Air Quality, Thermal Environment, Lighting And Acoustics. https://online.sfs.fi/fi/index/tuotteet/SFS/CEN/ID2/1/766211.html.stx.
- Shan, K., S. Wang, C. Yan, and F. Xiao. 2016. “Building Demand Response and Control Methods for Smart Grids: A Review.” Science & Technology for the Built Environment 22 (6): 692–704. https://doi.org/10.1080/23744731.2016.1192878.
- Siano, P. 2014. “Demand Response and Smart Grids—A Survey.” Renewable & Sustainable Energy Reviews 30: 461–478. https://doi.org/10.1016/j.rser.2013.10.022.
- Suhonen, J., J. Jokisalo, R. Kosonen, V. Kauppi, Y. Ju, and P. Janßen. 2020. “Demand Response Control of Space Heating in Three Different Building Types in Finland and Germany.” Energies 13: 23. https://doi.org/10.3390/en13236296.
- Sun, Z., L. Li, and F. Dababneh. 2016. “Plant-level Electricity Demand Response for Combined Manufacturing System and Heating, Venting, and air-Conditioning (HVAC) System.” Journal of Cleaner Production 135: 1650–1657. https://doi.org/10.1016/j.jclepro.2015.12.098.
- Tillman, P. 2017. Entwicklung einer Einsatzoptimierung von W€armeerzeugern zur wirtschaftlichen Bewertung unterschiedlicher Integrationskonzepte tiefer Geothermie in einem Nahw€armenetz [Development of an optimization for the use of heat generation units for the economic evaluation of different integration concepts of deep geothermal energy in a local heating network].
- Vand, B. 2020. “Demand Response Potential of District Heating and Ventilation in an Educational Office Building.” Science and Technology for the Built Environment 26 (3): 304–319. https://doi.org/10.1080/23744731.2019.1693207.
- Vogt, M., F. Marten, and M. Braun. 2018. “A Survey and Statistical Analysis of Smart Grid co-Simulations.” Applied Energy 222: 67–78. https://doi.org/10.1016/j.apenergy.2018.03.123.
- Werner, S. 2017. “District Heating and Cooling in Sweden.” Energy (Oxford) 126: 419–429. https://doi.org/10.1016/j.energy.2017.03.052.
- Yin, R., E. C. Kara, Y. Li, N. DeForest, K. Wang, T. Yong, and M. Stadler. 2016. “Quantifying Flexibility of Commercial and Residential Loads for Demand Response Using Setpoint Changes.” Applied Energy 177: 149–164. https://doi.org/10.1016/j.apenergy.2016.05.090.
- Yuan, X., L. Lindroos, J. Jokisalo, R. Kosonen, Y. Pan, and H. Jin. 2021. “Demand Response Potential of District Heating in a Swimming Hall in Finland [Article].” Energy and Buildings 248: 111149. https://doi.org/10.1016/j.enbuild.2021.111149.