Abstract
The association between body mass index (BMI) and chronic obstructive pulmonary disease (COPD) remains controversial. Therefore, a meta-analysis was conducted to further evaluate the relationship. A comprehensive literature search was performed in PubMed, Web of Science, EMBASE, China National Knowledge Infrastructure (CNKI), and Wanfang databases to identify eligible articles until July 15, 2020. Random effect model (REM) was used to compute the pooled results with 95% confidence intervals (CIs). We conducted meta-regression and subgroup analysis to explore potential sources of heterogeneity. Publication bias was evaluated by funnel plots and Egger’s test. Thirty articles with 1,578,449 participants were included in the meta-analysis. The pooled OR of COPD was 1.96 (95% CI: 1.78–2.17) for the underweight group, 0.80 (95% CI: 0.73–0.87) for overweight group, and 0.86 (95% CI: 0.73–1.02) for obesity group. After further excluding 5 studies of high between-study heterogeneity in sensitivity analysis, the pooled OR of COPD was 0.77 (95% CI: 0.68–0.86) for the obesity group. This meta-analysis indicated that BMI was associated with COPD. Specifically, underweight might increase the risk of COPD; overweight and obesity might reduce the risk of COPD.
Introduction
Chronic obstructive pulmonary disease (COPD), one of the most common respiratory diseases, is characterized by irreversible airflow limitation and respiratory obstruction [Citation1]. Airflow limitation has a profound effect on gas exchange and heart function, and leads to comorbidities such as skeletal muscle atrophy and heart failure [Citation2]. Comorbid diseases increase the morbidity and mortality of COPD. In 2015, 3 million people worldwide died of COPD, accounting for 5% of the all deaths. Compared with 1990, the number of deaths from COPD in 2015 increased by 10% and the prevalence increased by 44% [Citation3]. It is estimated that there were 13 million (6.5%) patients with COPD in the United States in 2011 [Citation4]. And direct costs of COPD treatment accounts for $18 billion a year, posing a major challenge to the health care system [Citation5].
Recent years, available evidences found that dietary intake, life habits, and physical conditions could affect the prevalence and development of COPD [Citation6]. Some modifiable dietary intake factors may decrease the risk of COPD such as high fiber [Citation7], vitamin C [Citation8] and fatty acids [Citation9]. In life habits, using biomass fuels [Citation10] and smoking [Citation11] could increase the risk of COPD. As physical status indicators, a history of allergies [Citation12] and high body mass index (BMI) were protective factors to the development of COPD [Citation13].
Over the past 40 years, the global prevalence of underweight was more than double that of obesity. The prevalence of obesity exceeded that of underweight in 2004 among women and in 2011 among men. In 2014, the global age-standardized prevalence of underweight (BMI < 18.5 kg/m2) was 8.8% (7.4%–10.3%) among men and 9.7% (8.3%–11.1%) among women [Citation14]. Underweight might have significant association with osteoporosis, puberty retardation, mental disorders, and respiratory parameters [Citation15]. In 2014, the global age-standardized prevalence of obesity was 10.8% (9.7%–12.0%) among men and 24.0% (18.9%–29.3%) among women [Citation14]. It is estimated that 1.9 billion and 609 million adults were overweight and obesity in 2015, accounting for approximately 39% of the world’s population [Citation16]. Obesity plays a key role in the pathogenesis of multiple system and organ diseases, such as cardiovascular disease, diabetes mellitus, cancers, respiratory disease and so on [Citation17].
The relationship between overweight/obesity and COPD is being increasingly explored. However, there are inconsistencies in the current data. A cohort study by Merja Kanervisto [Citation13] found that overweight and obesity could reduce the risk of COPD. Other studies also suggested that overweight and obesity were protective factors for COPD [Citation11, Citation18, Citation19]. And a large cross-sectional, population-based study conducted in America concluded that overweight and obesity were risk factors for COPD [Citation20]. In addition, some studies showed that there was not statistical significance between overweight/obesity and COPD [Citation21–23].
Given these inconsistencies, we conducted this meta-analysis to further elucidate the relationship between BMI and COPD.
Methods
Search strategy
A literature search was performed to identify available articles up to July 15, 2020 from PubMed, Web of Science, EMBASE, China National Knowledge Infrastructure (CNKI) and Wanfang, Search terms included the following search terms: (BMI or ‘body mass index’ or obesity or overweight or ‘surplus weight’ or waist or hipline or ‘waist hip rate’ or WHR or ‘abdominal obesity’ or ‘visceral adiposity’) and (‘chronic obstructive pulmonary disease’ or COPD). The language was restricted to English and Chinese. Moreover, the reference lists of retrieved articles were reviewed to identify further undetected relevant articles.
Inclusion criteria
Two investigators independently reviewed all identified studies. The inclusion criteria were as follows: (1) observational study designs (cohort, case-control, cross-sectional); (2) the exposure of interest were BMI, underweight, normal weight (reference group), overweight, and obesity; (3) the outcome of interest was COPD; (4) odds ratio (OR), risk ratio (RR) or hazard ratio (HR) with 95% confidence interval (CI) were provided; (5) studies were reported in English or Chinese; (6) the most recent and complete article was chosen if data from the same population had been published more than once.
Studies were excluded according to the following criteria: (1) were not original study or full texts; (2) did not report OR, RR or HR with 95% CI (or no data available to calculate them); (3) outcomes were associated with mortality of COPD.
Data extraction
The following information was extracted from every study: (1) name of first author; (2) publication year; (3) country and continent; (4) study type; (5) age range of cases/controls; (6) number of cases; (7) sample size; (8) BMI category; (9) BMI criteria; (10) assessment of COPD; (11) reported form of COPD; (12) OR, RR or HR with 95% CI, and (13) adjusted covariates.
BMI is now used to define a person as underweight, normal weight, overweight or obesity. Currently, there are several different criteria for BMI, including WHO criteria, Chinese criteria, Asian criteria and so on. Furthermore, there are two primary measurements of COPD, including GOLD (Global Initiative for Chronic Obstructive Lung Disease) criteria which is defined as post-bronchodilator FEV1/FVC (FVC: Forced vital capacity) < 70%, and LLN (Lower Limit of Normal) criteria which is defined as post-bronchodilator FEV1/FVC < cutoff based on lower limit of normal [Citation24].
Statistical analysis
The study-specific log ORs were weighted by the inverse of their variance to calculate pooled ORs with corresponding 95% CIs of the association between BMI and COPD. The I2 was used to assess heterogeneity (I2 values of 0, 25, 50, and 75% represent no, low, moderate and high heterogeneity respectively) [Citation25]. We used random-effect model (REM) if high heterogeneity (I2 >50%) was found; otherwise, the fixed-effect model (FEM) was adopted. Meta-regression was performed to explore the potentially important covariates that might exert substantial impacts on between-study heterogeneity. Influence analysis was used to assess whether the results could be significantly affected by a single study which removed at a time. Leave-one-out sensitivity analysis was carried out to evaluate the key studies that have substantial impact on between-study heterogeneity. Subgroup analysis was performed by year, gender, study type, continent, assessment forms of COPD, reported forms of COPD, BMI criteria, and controls. Publication bias was estimated using Egger’s test, trim and fill method, and visualization of funnel plots.
Statistical analyses were conducted using STATA 15.0 software (Stata Corporation, College Station, TX, USA). All reported P values were two-sided, and p < 0.05 was regarded as statistically significant.
Results
Literature search and study characteristics
The search strategy identified 4,748 articles from PubMed, 4,237 articles from Web of Science, 3,989 articles from EMBASE, 2,452 articles from CNKI, 173 articles from Wan Fang. Six additional articles were found through the reference lists of included articles. After duplicates being removed, 10,512 articles were excluded on screening of titles and/or abstract. After reading full text, 37 articles were excluded for the following reasons: duplicated reports from the same study population (n = 3); lacking OR, RR or HR and 95% CI (n = 31); review (n = 3). Finally, 30 articles were included in this meta-analysis [Citation6, Citation10–13, Citation18–23, Citation26–44]. The detailed steps of our literature search are shown in .
Thirty articles (22 cross-sectional articles, 7 cohort articles and 1 case-control article) were included, involving 1,578,449 participants. In thirty articles, seven articles were conducted in North America (NA), 18 in Asia, four in Europe, and one in Africa. As for assessment of COPD, 13 articles used GOLD, one article used LLN, one article used both GOLD and LLN. As for reported form of COPD, 19 articles were based on spirometry, six articles on questionnaires, one on face-to-face interviews and one on database. The detailed characteristics of included articles are shown in Supplementary Table S1.
Table 1. Subgroup analysis of underweight and risk of COPD.
Quantitative synthesis
For underweight group, the pooled OR of 24 articles [Citation6, Citation10–13, Citation18–23, Citation27, Citation28, Citation30–34, Citation38, Citation39, Citation41–44] was 1.96 (95% CI: 1.78–2.17; I2=67.3%, Pheterogeneity <0.01; ). For overweight group, the pooled OR of 30 articles [Citation6, Citation10–13, Citation18–23, Citation26–44] was 0.80 (95% CI: 0.73–0.87; I2=83.8%, Pheterogeneity <0.01; ). For obesity group, the pooled OR of 23 articles [Citation10, Citation12, Citation13, Citation18, Citation19, Citation22, Citation23, Citation26–34, Citation36–39, Citation42–44] was 0.86 (95% CI: 0.73–1.02; I2=89.4%, Pheterogeneity <0.01; ).
Figure 2. Forest plot of the odds ratio (ORs) with corresponding 95% confidence intervals (CIs) of the association between underweight and risk of COPD.
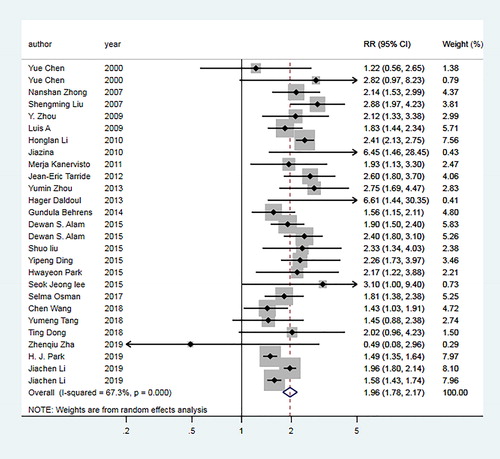
Subgroup analysis
In the underweight group, subgroup analysis was performed by the year (≤2013; >2013), study type (cross-section; cohort), gender (female; male), smoking status (never smoked; smoked), assessment form of COPD (GOLD; LLN), reported form of COPD (spirometry; questionnaires; others), continent (NA; Asia; Europe), and BMI criteria (China; WHO; Asia; others), the results indicated that underweight was a risk factor for COPD. The details of results are summarized in .
In the overweight group, subgroup analysis was performed by year, gender, study type, continent, smoking status, assessment forms of COPD, reported forms of COPD, BMI criteria, and controls. The results showed a significant inverse association in the subgroup analysis by year (≤2013; >2013) and study type (cross-section; cohort). In stratified analysis by assessment and reported forms of COPD, the findings showed a significant inverse association in GOLD method (OR = 0.65, 95% CI: 0.54–0.78) and spirometry method (OR = 0.68, 95% CI: 0.59–0.78). For the subgroup analysis by the continent, a significant inverse association was found for Asia (OR = 0.76, 95% CI: 0.68–0.85) and Europe (OR = 0.58, 95% CI: 0.38–0.88). The details of results are summarized in .
Table 2. Subgroup analysis of overweight and risk of COPD.
In the obesity group, subgroup analysis was performed by year, gender, study type, continent, reported forms of COPD, BMI criteria, and controls. According to the continent, a statistically significant association was found in Asia (OR = 0.74, 95% CI: 0.62–0.89), but not in those conducted in the other continents. With regard to the selection of controls, the association between obesity and COPD was significant (OR = 0.83, 95% CI: 0.70–0.99) in normal weight controls, but not in non-overweight controls (OR = 1.06, 95% CI: 0.44–2.57). The details of results are summarized in .
Table 3. Subgroup analysis of obesity and risk of COPD.
Meta‑regression
To explore the sources of heterogeneity, meta-regression with the covariates of year, study type, gender, assessment form of COPD, reported form of COPD, continent, and BMI criteria were performed. The P value of these covariates in underweight group was 0.002, 0.094, 0.453, 0.170, 0.041, 0.131, and 0.674, respectively, which suggested that year and reported form of COPD were the important sources of heterogeneity. The P value in overweight group was 0.759, 0.541, 0.028, 0.000, 0.011, 0.039, and 0.841, respectively, which suggested that gender, assessment form of COPD, reported form of COPD, and continent were the important sources of heterogeneity. The P value in obesity group was 0.396, 0.815, 0.032, 0.002, 0.011, 0.074, and 0.069, respectively, which suggested that gender, assessment form of COPD and reported form of COPD were the important sources of heterogeneity.
Sensitivity analyses
By using the leave-one-out sensitivity analysis, two studies contributed to high between-study heterogeneity in underweight group. After further excluding these two studies, low heterogeneity (I2= 40.7%) was found and the pooled OR was 2.00 (95% CI: 1.80–2.22). In overweight group, three studies contributed to high between-study heterogeneity. After further excluding these three studies, low heterogeneity (I2 = 39.3%) was found and the pooled OR was 0.81 (95% CI: 0.77–0.87). In obesity group, five studies contributed to high between-study heterogeneity. After further excluding these five studies, low heterogeneity (I2 = 45.4%) was found and the pooled OR was 0.77 (95% CI: 0.68–0.86).
Influence analysis and publication bias
Influence analysis showed that no studies had an excessive influence on the pooled results in three groups (). For the association of underweight and COPD, Egger test showed that there was publication bias (p = 0.037). Seven articles were trimmed after running trim and fill technique. The pooled OR was 1.86 (95% CI: 1.73–1.88) after trimming. In the overweight and obesity groups, Egger test showed that the P values were 0.013 and 0.041, indicating there were publication biases. However, no articles were trimmed after running trim and fill technique. In three groups, the funnel plots appeared to be asymmetrical ().
Discussion
In this meta-analysis, we evaluated the intricate relationship between BMI and COPD. Thirty articles with 1,578,449 participants were included in the meta-analysis. The pooled results indicated that BMI is associated with COPD. Specifically, underweight might increase the risk of COPD. In addition, overweight and obesity could reduce the risk of COPD.
The mechanism of the relationship between BMI and COPD is unclear, but several possible mechanisms have been summarized as follows. Underweight partially mediates the effect on lung function through its impact on respiratory muscles [Citation26]. The increased risk of COPD in underweight individuals may be associated with reduced skeletal muscles mass, which affects both respiratory and non-respiratory muscles [Citation45]. Furthermore, underweight is related to malnutrition or low socioeconomic status. Malnutrition is one of the causes of respiratory muscle dysfunction that often occurs in patients with COPD [Citation46]. In addition, malnutrition increases respiratory muscular atrophy and susceptibility to infection, subsequently impairs lung function [Citation33].
The reduced risk of COPD in overweight/obesity individuals could be explained from the following aspects. First of all, BMI includes not only fat mass but also muscle mass [Citation47]. As a substitute for skeletal muscle mass, fat-free mass (FFM) is positively correlated with cardiorespiratory fitness (CRF) and respiratory muscle strength [Citation48–50]. The lower risk of COPD in obese people may be partly due to the protective effect of higher FFM [Citation51]. Secondly, lung hyperinflation increases the volume of the lungs, shortens and flattens the diaphragm. Therefore, the diaphragm loses the ability to produce contractile force [Citation52]. Compared with people of normal or underweight, obese people have smaller expiratory reserve volume and end-expiratory lung volume, breathe shallower and faster, and have less lung hyperinflation [Citation49]. In addition, lower BMI was related to faster FEV1(Forced expiratory volume in 1 s) decline; higher BMI was related to slower FEV1 decline [Citation47].
Some studies have found that obesity can also affect the prognosis of chronic diseases [Citation53–56]. The “obesity paradox” is that overweight or obesity is associated with a better prognosis in subjects with chronic diseases [Citation57]. Some studies showed that overweight or obesity had a protective effect on the mortality of COPD patients [Citation53, Citation58]. This is because COPD patients need high respiratory muscle mass to cope with increased airway resistance and airflow obstruction [Citation52]. FFM and respiratory muscle mass of overweight/obesity COPD patients are higher than those of normal or underweight COPD patients [Citation59, Citation60]. The obesity paradox not only occurs in COPD, but also in some chronic diseases such as pulmonary embolism [Citation56], cancer [Citation54], and cardiovascular disease [Citation55]. A study on the survival benefits of obese patients with pulmonary embolism showed that the survival rate of pulmonary embolism patients in obesityIand II was higher [Citation56]. Prerana Chittal et al. found that CRF might also be an important factor leading to the obesity paradox in patients with cardiovascular disease. Higher CRF is believed to change the association between obesity and outcome [Citation60]. In addition, obesity, as a nutritional reserve, is particularly important for older, weaker, and comorbid patients. This can also explain why some obese patients with chronic diseases have greater survival benefits [Citation56]. Therefore, the protective effect of higher BMI on the prognosis of certain chronic diseases in the obesity paradox is consistent with the conclusion in our study that overweight or obesity may reduce the risk of COPD.
Heterogeneity between studies is common in meta-analysis, and mainly includes clinical heterogeneity (differences in participants, interventions, and outcomes between studies), methodological heterogeneity and statistical heterogeneity [Citation61]. Due to the high heterogeneity of this study, we used the random effects model (REM) to calculate the pooled results with 95% CIs. To explore the potential sources of heterogeneity, meta-regression and subgroup analysis were carried out. The results indicated that gender, assessment form of COPD and reported form of COPD could be the important sources of heterogeneity. Two, three, and five studies were found to contribute to high heterogeneity for underweight, overweight, and obesity groups by sensitivity analysis, respectively. After excluding these studies, the results did not change in underweight and overweight group, indicating our results were stable and reliable. However, in obesity group, the result changed from insignificance into significance after excluding five studies which contributed to between-study heterogeneity. The results of these excluded studies indicated that obesity was a risk factor for COPD. Compared with other studies, the BMI classification criteria of three excluded studies [Citation12, Citation27, Citation44] were not used common criteria. The OR of an excluded study [Citation42] was only for men. And the outcome of another excluded study [Citation30] was emphysema or COPD.
After analysis, reported form of COPD could be critical sources of heterogeneity in this study. On the one hand, although spirometry is recommended for the diagnosis of COPD, there is considerable challenge based on spirometry in implementing public screening programs. Spirometry is not yet widely available and requires a major investment to healthcare system [Citation42]. Therefore, it is common that the diagnosis of COPD without spirometry [Citation62]. On the other hand, patients might misjudge themselves as normal person in the early stage of COPD because their symptoms are not obvious enough. A study has shown that the prevalence of measured chronic diseases (41.9%) is higher than that of self-reported (24.1%) [Citation63]. Moreover, other forms of COPD reported may have some subjective intention and recall bias, they are not accurate enough relative to the spirometry.
Another possible source of heterogeneity in the study might be the assessment form of COPD. At present, the Global Initiative for Chronic Obstructive Lung Disease tends to use fixed ratio rather than LLN because simplicity and consistency in diagnosis are critical for busy clinicians [Citation24]. GOLD criterion is now widely accepted, mainly because of its practicality. Using the GOLD criterion as a public lung function screening program, although it may be an effective and simple method, seems to overestimate COPD in the elderly [Citation64]. This could be due to the fact that FEV1 value increases with age faster than FVC declines [Citation65]. According to the normal distribution, LLN criterion classifies the bottom 5% of the healthy people as abnormal [Citation66]. The use of the LLN criteria instead of the fixed ratio could reduce age bias and preferably reflect clinically irreversible airflow limitation [Citation21]. However, a study conducted by Surya and colleagues [Citation67] found that LLN criterion failed to identify some patients with important lung pathology and respiratory diseases.
There is growing evidence that BMI and body fat distribution differ among different populations. Compared with Europeans and Americans, Asians have a lower average BMI value and a higher body fat percentage [Citation68]. The cutoff points of WHO, Asian and Chinese criteria for obesity are 30, 25, 28, respectively. In this meta-analysis, included studies used different BMI criteria to define obesity and different BMI classifications as references, which might also be another source of heterogeneity.
This meta-analysis has some advantages. First, plenty of studies and participants were included, increasing the power of the analyze. Second, we roundly analyzed the relationship between BMI and COPD from three aspects of underweight, overweight, and obesity. Third, after sensitivity analysis and influence analysis, the results of underweight group and overweight group did not change, indicating that the results were stable. Fourth, all articles were adjusted for most confounding factors.
Similarly, the present study also has several limitations. First, there was a high heterogeneity in this meta-analysis, but the heterogeneity was greatly reduced after sensitivity analysis. Second, the articles included in this meta-analysis have multiple non-uniform methods to classify BMI, which could affect the results to certain extent. Third, for COPD diagnosed by questionnaires or self-reported, it may be misdiagnosed due to potential measurement errors and recall bias. Fourth, the adjusted confounding factors were different in each study, which may affect the observed association.
Conclusions
In summary, this meta-analysis indicated that underweight might increase the risk of COPD; overweight and obesity might reduce the risk of COPD. It deepens our understanding and insight into the intricate relationship between BMI and COPD. Further in-depth researches are needed to investigate the real cause and effect relationship between them.
Declaration of interest statement
The authors declare that they have no competing interests.
Supplemental Material
Download PDF (473.4 KB)Additional information
Funding
References
- Cho WK, Lee CG, Kim LK. COPD as a disease of immunosenescence. Yonsei Med J. 2019;60(5):407–413. DOI:10.3349/ymj.2019.60.5.407
- Barnes PJ, Celli BR. Systemic manifestations and comorbidities of COPD. Eur Respir J. 2009;33(5):1165–1185. DOI:10.1183/09031936.00128008
- Criner RN, Han MK. COPD care in the 21st century: a public health priority. Respir Care. 2018;63(5):591–600. DOI:10.4187/respcare.06276
- Ford ES, Croft JB, Mannino DM, et al. COPD surveillance–United States, 1999-2011. Chest. 2013;144(1):284–305. DOI:10.1378/chest.13-0809
- López-Campos JL, Tan W, Soriano JB. Global burden of COPD. Respirology. 2016;21(1):14–23. DOI:10.1111/resp.12660
- Behrens G, Matthews CE, Moore SC, et al. Body size and physical activity in relation to incidence of chronic obstructive pulmonary disease. CMAJ. 2014;186(12):E457–E469. DOI:10.1503/cmaj.140025
- Szmidt MK, Kaluza J, Harris HR, et al. Long-term dietary fiber intake and risk of chronic obstructive pulmonary disease: a prospective cohort study of women. Eur J Nutr. 2020;59(5):1869–1879. DOI:10.1007/s00394-019-02038-w
- Park HJ, Byun MK, Kim HJ, et al. Dietary vitamin C intake protects against COPD: the Korea National Health and Nutrition Examination Survey in 2012. COPD. 2016;11:2721–2728. DOI:10.2147/COPD.S119448
- Jiménez-Cepeda A, Dávila-Said G, Orea-Tejeda A, et al. Dietary intake of fatty acids and its relationship with FEV1/FVC in patients with chronic obstructive pulmonary disease. Clin Nutr Espen. 2019;29:92–96. DOI:10.1016/j.clnesp.2018.11.015
- Liu SM, Zhou YM, Wang XP, et al. Biomass fuels are the probable risk factor for chronic obstructive pulmonary disease in rural South China. Thorax. 2007;62(10):889–897. DOI:10.1136/thx.2006.061457
- Zhong N, Wang C, Yao W, et al. Prevalence of chronic obstructive pulmonary disease in China: a large, population-based survey. Am J Respir Crit Care Med. 2007;176(8):753–760. DOI:10.1164/rccm.200612-1749OC
- Chen Y, Breithaupt K, Muhajarine N. Occurrence of chronic obstructive pulmonary disease among Canadians and sex-related risk factors. J Clin Epidemiol. 2000;53(7):755–761. DOI:10.1016/S0895-4356(99)00211-5
- Kanervisto M, Vasankari T, Laitinen T, et al. Low socioeconomic status is associated with chronic obstructive airway diseases. Respir Med. 2011;105(8):1140–1146. DOI:10.1016/j.rmed.2011.03.008
- NCD Risk Factor Collaboration (NCD-RisC). Trends in adult body-mass index in 200 countries from 1975 to 2014: a pooled analysis of 1698 population-based measurement studies with 19·2 million participants. Lancet. 2016;387(10026):1377–1396.
- Mak K-K, Tan SH. Underweight problems in Asian children and adolescents. Eur J Pediatr. 2012;171(5):779–785. DOI:10.1007/s00431-012-1685-9
- Chooi YC, Ding C, Magkos F. The epidemiology of obesity. Metabolism. 2019;92:6–10. DOI:10.1016/j.metabol.2018.09.005
- Dixon AE, Peters U. The effect of obesity on lung function. Expert Rev Respir Med. 2018;12(9):755–767. DOI:10.1080/17476348.2018.1506331
- Li H, Xu B, Zheng W. The prevalence of obesity in middle-aged and old men and its relationship with chronic diseases. Chinese J Epidemiol. 2010;31(4):370–374.
- Zhou Y, Wang D, Liu S, et al. The association between BMI and COPD: the results of two population-based studies in Guangzhou, China. COPD. 2013;10(5):567–572. DOI:10.3109/15412555.2013.781579
- Fuller-Thomson E, Howden KEN, Fuller-Thomson LR, et al. A strong graded relationship between level of obesity and COPD: findings from a national population-based study of lifelong nonsmokers. J Obes. 2018;2018:1–9. DOI:10.1155/2018/6149263
- Daldoul H, Denguezli M, Jithoo A, et al. Prevalence of COPD and tobacco smoking in Tunisia–results from the BOLD study. Int J Environ Res Public Health. 2013;10(12):7257–7271. DOI:10.3390/ijerph10127257
- Park H, Jung SY, Lee K, et al. Prevalence of chronic obstructive lung disease in Korea using data from the fifth Korea national health and nutrition examination survey. Korean J Fam Med. 2015;36(3):128–134. DOI:10.4082/kjfm.2015.36.3.128
- Zhou Y, Wang C, Yao W, et al. COPD in Chinese nonsmokers. Eur Respir J. 2009;33(3):509–518. DOI:10.1183/09031936.00084408
- Vogelmeier CF, Criner GJ, Martinez FJ, et al. Global strategy for the diagnosis, management, and prevention of chronic obstructive lung disease 2017 report. GOLD executive summary. Am J Respir Crit Care Med. 2017;195(5):557–582. DOI:10.1164/rccm.201701-0218PP
- Higgins JP, Thompson SG, Deeks JJ, et al. Measuring inconsistency in meta-analyses. BMJ. 2003;327(7414):557–560. DOI:10.1136/bmj.327.7414.557
- Harik-Khan RI, Fleg JL, Wise RA. Body mass index and the risk of COPD. Chest. 2002;121(2):370–376. DOI:10.1378/chest.121.2.370
- García Rodríguez LA, Wallander MA, Tolosa LB, et al. Chronic obstructive pulmonary disease in UK primary care: incidence and risk factors. Copd. 2009;6(5):369–379. DOI:10.1080/15412550903156325
- Na J, Hu T, Zhao S, et al. Epidemiological investigation of chronic obstructive pulmonary disease in Uighur population over 60 years old in rural Hotan, Xinjiang. J XinJiang Med Univ. 2010;33(09):1017–1020.
- Tran HN, Siu S, Iribarren C, et al. Ethnicity and risk of hospitalization for asthma and chronic obstructive pulmonary disease. Ann Epidemiol. 2011;21(8):615–622. DOI:10.1016/j.annepidem.2010.10.015
- Tarride JE, Haq M, Taylor VH, et al. Health status, hospitalizations, day procedures, and physician costs associated with body mass index (BMI) levels in Ontario, Canada. Clinicoecon Outcomes Res. 2012;4:21–30. DOI:10.2147/CEOR.S24192
- Alam DS, Chowdhury MA, Siddiquee AT, et al. Prevalence and determinants of chronic obstructive pulmonary disease (COPD) in Bangladesh. COPD. 2015;12(6):658–667.
- Ding YP, Xu JX, Yao JJ, et al. The analyses of risk factors for COPD in the Li ethnic group in Hainan, People's Republic of China. Int J Chron Obstruct Pulmon Dis. 2015;10:2593–2599.
- Lee SJ, Kim SW, Kong KA, et al. Risk factors for chronic obstructive pulmonary disease among never-smokers in Korea. Int J Chron Obstruct Pulmon Dis. 2015;10:497–506.
- Liu S, Ren Y, Wen D, et al. Prevalence and risk factors for COPD in greenhouse farmers: a large, cross-sectional survey of 5,880 farmers from northeast China. Int J Chron Obstruct Pulmon Dis. 2015;10:2097–2108. DOI:10.2147/COPD.S79264
- Martin-Rodriguez E, Guillen-Grima F, Marti A, et al. Comorbidity associated with obesity in a large population: the APNA study. Obes Res Clin Pract. 2015;9(5):435–447. DOI:10.1016/j.orcp.2015.04.003
- Wang C, Xu J, Yang L, et al. Prevalence and risk factors of chronic obstructive pulmonary disease in China (the China Pulmonary Health [CPH] study): a national cross-sectional study. Lancet. 2018;391(10131):1706–1717. DOI:10.1016/S0140-6736(18)30841-9
- Zhao C, Wong L, Zhu Q, et al. Prevalence and correlates of chronic diseases in an elderly population: a community-based survey in Haikou. PLoS One. 2018;13(6):e0199006. DOI:10.1371/journal.pone.0199006
- Dong T, Xu X, Chen X, et al. Study on the risk factors of COPD in non-smokers in Sichuan Province. J Prev Med Inform. 2018;34(5):582–585.
- Tang Y, Li Q, Zhang L, et al. Epidemiological investigation and influencing factors of chronic obstructive pulmonary disease in Hubei Province. Chinese J Dis Control. 2018;22(7):721–725.
- Katsimigas A, Tupper OD, Ulrik CS. Opportunistic screening for COPD in primary care: a pooled analysis of 6,710 symptomatic smokers and ex-smokers. COPD. 2019;14:1633–1638. DOI:10.2147/COPD.S204190
- Park HJ, Cho JH, Kim HJ, et al. The effect of low body mass index on the development of chronic obstructive pulmonary disease and mortality. J Intern Med. 2019;286(5):573–582. DOI:10.1111/joim.12949
- Li J, Lv J, Gao M, et al. The relationship between body mass index and waist circumference and the risk of major chronic diseases in Chinese adults. Chinese J Epidemiol. 2019;40(12):1541–1547.
- Zha Z, Leng R, Xu W, et al. Prevalence and risk factors of chronic obstructive pulmonary disease in Anhui Province, China: a population-based survey. BMC Pulm Med. 2019;19(1):102. DOI:10.1186/s12890-019-0864-0
- Osman S, Ziegler C, Gibson R, et al. The association between risk factors and chronic obstructive pulmonary disease in Canada: a cross-sectional study using the 2014 Canadian Community Health Survey. Int J Prev Med. 2017;8(1):86. DOI:10.4103/ijpvm.IJPVM_330_17
- Li J, Zhu L, Wei Y, et al. Association between adiposity measures and COPD risk in Chinese adults. Eur Respir J. 2020;55(4):1901899. DOI:10.1183/13993003.01899-2019
- Charususin N, Dacha S, Gosselink R, et al. Respiratory muscle function and exercise limitation in patients with chronic obstructive pulmonary disease: a review. Expert Rev Respir Med. 2018;12(1):67–79. DOI:10.1080/17476348.2018.1398084
- Sun Y, Milne S, Jaw JE, et al. BMI is associated with FEV(1) decline in chronic obstructive pulmonary disease: a meta-analysis of clinical trials. Respir Res. 2019;20(1):236. DOI:10.1186/s12931-019-1209-5
- Wannamethee SG, Shaper AG, Whincup PH. Body fat distribution, body composition, and respiratory function in elderly men. Am J Clin Nutr. 2005;82(5):996–1003. DOI:10.1093/ajcn/82.5.996
- Guenette JA, Jensen D, O’donnell DE. Respiratory function and the obesity paradox. Curr Opin Clin Nutr Metab Care. 2010;13(6):618–624. DOI:10.1097/MCO.0b013e32833e3453
- Carbone S, Billingsley HE, Rodriguez-Miguelez P, et al. Lean mass abnormalities in heart failure: the role of sarcopenia, sarcopenic obesity, and cachexia. Curr Probl Cardiol. 2020;45(11):100417. DOI:10.1016/j.cpcardiol.2019.03.006
- Park CH, Yi Y, Do JG, et al. Relationship between skeletal muscle mass and lung function in Korean adults without clinically apparent lung disease. Medicine (Baltimore). 2018;97(37):e12281.
- Gea J, Pascual S, Casadevall C, et al. Muscle dysfunction in chronic obstructive pulmonary disease: update on causes and biological findings. J Thorac Dis. 2015;7(10):E418–E438. DOI:10.3978/j.issn.2072-1439.2015.08.04
- Schembri S, Anderson W, Morant S, et al. A predictive model of hospitalisation and death from chronic obstructive pulmonary disease. Respir Med. 2009;103(10):1461–1467. DOI:10.1016/j.rmed.2009.04.021
- Lennon H, Sperrin M, Badrick E, et al. The obesity paradox in cancer: a review. Curr Oncol Rep. 2016;18(9):56. DOI:10.1007/s11912-016-0539-4
- Elagizi A, Kachur S, Lavie CJ, et al. An overview and update on obesity and the obesity paradox in cardiovascular diseases. Prog Cardiovasc Dis. 2018;61(2):142–150. DOI:10.1016/j.pcad.2018.07.003
- Keller K, Hobohm L, Münzel T, et al. Survival benefit of obese patients with pulmonary embolism. Mayo Clin Proc. 2019;94(10):1960–1973. DOI:10.1016/j.mayocp.2019.04.035
- Spelta F, Fratta Pasini AM, Cazzoletti L, et al. Body weight and mortality in COPD: focus on the obesity paradox. Eat Weight Disord. 2018;23(1):15–22. DOI:10.1007/s40519-017-0456-z
- Chailleux E, Laaban JP, Veale D. Prognostic value of nutritional depletion in patients with COPD treated by long-term oxygen therapy: data from the ANTADIR observatory. Chest. 2003;123(5):1460–1466. DOI:10.1378/chest.123.5.1460
- Sabino PG, Silva BM, Brunetto AF. Nutritional status is related to fat-free mass, exercise capacity and inspiratory strength in severe chronic obstructive pulmonary disease patients. Clinics (Sao Paulo, Brazil). 2010;65(6):599–605. DOI:10.1590/S1807-59322010000600007
- Chittal P, Babu AS, Lavie CJ. Obesity paradox: does fat alter outcomes in chronic obstructive pulmonary disease? COPD. 2015;12(1):14–18. DOI:10.3109/15412555.2014.915934
- Reito A, Launonen A, Paloneva J. Factors explaining heterogeneity in studies comparing surgical and nonsurgical treatment of midshaft clavicle fractures: a meta-regression analysis of randomized controlled trials and high-quality observational studies. J Shoulder Elbow Surg. 2020;29(8):1726–1734. DOI:10.1016/j.jse.2020.02.011
- Ho T, Cusack RP, Chaudhary N, et al. Under- and over-diagnosis of COPD: a global perspective. Breathe (Sheff). 2019;15(1):24–35. DOI:10.1183/20734735.0346-2018
- Patel S, Ram F, Patel SK, et al. Association of behavioral risk factors with self-reported and symptom or measured chronic diseases among adult population (18-69 years) in India: evidence from SAGE study. BMC Public Health. 2019;19(1):560. DOI:10.1186/s12889-019-6953-4
- Vollmer WM, Gíslason T, Burney P, et al. Comparison of spirometry criteria for the diagnosis of COPD: results from the BOLD study. Eur Respir J. 2009;34(3):588–597. DOI:10.1183/09031936.00164608
- Wang S, Gong W, Tian Y, et al. Voluntary pulmonary function screening with GOLD standard: an effective and simple approach to detect lung obstruction. J Thorac Dis. 2015;7(11):1970–1977. DOI:10.3978/j.issn.2072-1439.2015.11.20
- Pellegrino R, Viegi G, Brusasco V, et al. Interpretative strategies for lung function tests. Eur Respir J. 2005;26(5):948–968. DOI:10.1183/09031936.05.00035205
- Miller MR. Comparison of spirometric thresholds in diagnosing smoking-related airflow obstruction. Thorax. 2014;69(12):1145–1146. DOI:10.1136/thoraxjnl-2014-205960
- Oh SW, Shin SA, Yun YH, et al. Cut-off point of BMI and obesity-related comorbidities and mortality in middle-aged Koreans. Obes Res. 2004;12(12):2031–2040. DOI:10.1038/oby.2004.254