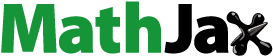
Abstract
Numerous studies have explored associations between bicycle network characteristics and bicycle ridership. However, the majority of these studies have been conducted in inner metropolitan regions and as such, there is limited knowledge on how various characteristics of bicycle networks relate to bicycle trips within and across entire metropolitan regions, and how the size and composition of study regions impact on the association between bicycle network characteristics and bicycle ridership. We conducted a retrospective analysis of household travel survey data and bicycle infrastructure in the Greater Melbourne region, Australia. Seven network metrics were calculated (length of the bicycle network, betweenness centrality, degree centrality, network density, network coverage, intersection density and average weighted slope) and Bayesian spatial models were used to explore associations between these network characteristics and bicycle ridership. We demonstrated that bicycle ridership was associated with several network characteristics, and that these characteristics varied according to the outcome (count of the number of trips made by bike or the proportion of trips made by bike) and the size and characteristics of the study region. These findings challenge the utility of approaches based on spatially modeling network characteristics and bicycle ridership when informing the monitoring and evaluation of bicycle networks. Further efforts are required to be able to quantify network characteristics that reflect the myriad of factors that influence comfort and safety for people of all ages and abilities.
1. Introduction
To advance bicycling as an active and sustainable mode of transport, cities across the world are increasing investment in bicycling infrastructure (Kraus & Koch, Citation2021; UK Department of Transport, Citation2020). The presence and quality of bicycling infrastructure has a significant impact on bicycling (Pucher & Buehler, Citation2017), and there is considerable scope for increases in bicycling participation when high-quality and connected infrastructure is provided (L. Pearson et al., Citation2022).
The length of bicycling infrastructure and the extent of newly implemented bicycling infrastructure are commonly used in planning documents and academic studies to assess a city’s bicycling network (Boisjoly et al., Citation2020; Buehler & Pucher, Citation2012). However, there is growing recognition of the importance of how ‘connected’ the network is; that is, enabling people to use continuous, safe and low-stress routes to access everyday destinations (Boisjoly et al., Citation2020; Lowry & Loh, Citation2017; Winters et al., Citation2013). Fragmentation in the network may force riders into mixed traffic, require lengthy detours, and may discourage bike riding altogether, primarily due to safety concerns (Handy & Xing, Citation2011; Schoner & Levinson, Citation2014). We acknowledge that there are a diversity of factors that influence bike riding that extend beyond infrastructure, including personal factors such as physical fitness, attitudinal factors such as community perceptions of cyclists, and environmental factors (Pearson et al., Citation2023; L. K. Pearson et al., Citation2022). However, consistently across the world, the key barriers to increased bike riding participation relate to riding on the road alongside motor vehicles (Pearson et al., Citation2023; L. K. Pearson et al., Citation2022). Therefore, providing safe and connected networks of bicycling infrastructure is critical to enhance bicycling participation.
Understanding the role that bicycle infrastructure network characteristics have on bicycling is therefore necessary to advance knowledge of how to plan the implementation of safe and connected networks, and to benchmark networks across jurisdictions. Recent developments in measuring bicycle network connection include the use of indicators developed using graph theory, such as density, directness and centrality (Kamel & Sayed, Citation2021; Osama et al., Citation2017; Schoner & Levinson, Citation2014). Such approaches offer systematic methods for measuring network quality for comparison within and between cities. For example, Schoner and Levinson demonstrated that connectivity and directness were important factors in predicting bicycle commuting (Schoner & Levinson, Citation2014), Osama et al. suggested more connected, dense, flat, continuous and off-street bicycle networks yield higher bicycling (Osama et al., Citation2017), and Kamel and Sayed showed that network centrality, assortativity, weighted slope, directness, length, complexity and connectivity were associated with bicycle ridership (Kamel & Sayed, Citation2021). While these studies have been important in advancing knowledge on the role of various network characteristics, they have commonly been conducted in inner metropolitan regions, such as inner Vancouver (Kamel & Sayed, Citation2021; Osama et al., Citation2017) or inner Seattle (Lowry & Loh, Citation2017), or have used single city-wide network measures to contrast these factors between cities (Schoner & Levinson, Citation2014). As such, there is limited knowledge on how various characteristics of bicycle networks relate to bicycle trips within and across entire metropolitan regions, and how the size and composition of study regions impact on the association between bicycle network characteristics and bicycle ridership.
Using population-weighted travel survey data and robust measures of bicycle network characteristics, this study aimed to: (1) quantify the association between bicycle network characteristics and bicycle ridership across the metropolitan region of Greater Melbourne, Australia, using Bayesian spatial modes; and (2) explore whether these associations hold or break down when the size of the study region is changed.
2. Methods
2.1. Study design
We conducted a retrospective analysis of household travel survey data and bicycle infrastructure in the Greater Melbourne region, Australia, using Bayesian spatial models
2.2. Setting
The State of Victoria, Australia, has a population of 6.7 million people of which 67% reside in the Greater Melbourne area.(Australian Bureau of Statistics, Citation2020) The Australian Bureau of Statistics (ABS) define seven hierarchical classifications of functional areas in Australia, from mesh blocks (the smallest unit) to the country level (the largest unit). Within these is a functional area known as Statistical Areas Level 2 (SA2), which are medium-sized general-purpose areas, reflecting a community that interacts together socially and economically. SA2s generally have a population range of 3,000 to 25,000 persons, with an average of approximately 10,000 persons. For this study, analyses were restricted to the 309 SA2 areas within Greater Melbourne, reflecting an area of 9,986 km2 ().
Figure 1. Map of (a) population density (persons per square kilometre); (b) total population per SA2. The borders represent SA2 boundaries in the Greater Melbourne region.

Greater Melbourne is a region with low bicycle mode share (1.7% of all trips) (Beck et al., Citation2021). In 2018, of the 40,564 km of total street/path infrastructure, 1,165 (2.9%) were classified as on-road bike lanes, 2,647 (6.5%) were classified as off-road paths, and 8 km (0.02%) were classified as protected on-road bike lanes.
2.3. Travel survey data
Travel survey data were captured through the Victorian Integrated Survey of Travel & Activity (VISTA), coordinated by the Victorian Department of Transport. We used data from three waves (2012–14, 2014–16, 2016–18) of the VISTA. VISTA is a survey of day-to-day travel conducted in the Greater Melbourne area and in a single regional center in Victoria. Since 2012, 16,000 households and 66,000 people have contributed to the VISTA survey. VISTA randomly selects households to complete the VISTA travel diary for a single specified day. VISTA employs a stratified, clustered sampling methodology, with stratification based on ABS Statistical Areas Level 3 (SA3), which are geographical areas built from whole SA2s. The survey and resulting data are weighted to generate population-representative data at the SA3 level, with data representing an average day across one or more of the three waves (2012–14, 2014–16, 2016–2018). In this study, we employed a set of combined weights that use the full data set from 2012–2018 to produce statistics weighted to the 2017–18 population. The Australian Statistical Geography Standard is used by the ABS for the release and analysis of statistics. Mesh blocks are the smallest geographical areas defined by the ABS, which then make up the increasingly larger statistical areas of SA1s, SA2s, SA3s and SA4s (see Supplementary Material). We selected SA2s as the study unit of area as it represented the best fit for the various forms of data that were used in this study and provided sufficient spatial variation in these characteristics; use of data at a SA3 level would have led to crude estimations of dependent and independent variables, losing the nuances of spatial variation that exists at a SA2 level. As robust weights for bicycling were not available at a SA2 level, SA3 weights were applied to SA2 level (i.e. that data were collapsed to an SA2 level and person-level SA3 weights were applied at an SA2 level). Weights were applied to the SA2 in which the trip originated, and therefore, data reflect where trips commenced and not trip routes or destinations. Unless otherwise specified, data reflect trips made within Greater Melbourne on an average day across the study period. Eligibility for inclusion in this study were participants aged 18 years and older, and trips that had trip origins and destinations within the Greater Melbourne region.
For the purposes of this study, we modeled counts of the number of trips and the proportion of all trips that were made by bike at an SA2 level. As described above, we used combined weights for the full data set from 2012–2018, and therefore the summary measures of bike ridership reflect an average day over the period of 2012–2018.
2.4. Bicycle infrastructure data
In the absence of government data sets of bicycle infrastructure in Victoria, we used Open Street Map (OSM) data to characterize bicycling infrastructure in the study region. We captured infrastructure at a single time-point, which was the final year of the study period (2018). 2018 OSM data was downloaded for the Greater Melbourne region from Geofrabrik (Citation2021). Bicycle infrastructure was coded by OSM contributors according to the OSM Wiki (OpenStreetMap, Citation2021) and stratified into: on-road bike lanes, protected on-road bike lanes, and off-road paths (off-road dedicated bike path, off-road shared path (shared with pedestrians), and footways where bicycling is legal). Further information on this method is described previously (Beck et al., Citation2021). In some cases, the border lines of SA2 areas fell along roads and paths. When classifying network characteristics for each SA2, a road or path that fell along an SA2 border was included in the analysis for both adjacent SA2s. Therefore, in some situations, roads or paths have been double counted as a result of their inclusion in multiple SA2s.
2.5. Network characteristics
To explore characteristics of the network, we calculated seven network metrics that have previous been demonstrated to be associated with bicycle ridership (Kamel & Sayed, Citation2021; Osama et al., Citation2017; Schoner & Levinson, Citation2014). These seven metrics were the length of the bicycle network, betweenness centrality, degree centrality, network density, network coverage, intersection density and average weighted slope. These were grouped under four headings (bicycle network length, network centrality, connectivity and coverage, and topography) and are described in detail below. These are described in detail below.
2.5.1. Bicycle network length
The length of the bicycle network was calculated for each SA2. This was categorized as bicycle infrastructure that was off-road (off-road dedicated bike path and off-road shared path) and on-road (on-road bike lanes and protected on-road bike lanes).
2.5.2. Network centrality
Two measures of centrality were calculated for the bicycle network (combined for both on-road and off-road infrastructure): betweenness centrality and degree centrality. Measures of centrality, such as betweenness and degree centrality, are firstly calculated at a node-level and describe how ‘central’ or important each node or link is within a network (Freeman, Citation1978). Specific definitions of node-level betweenness and degree centrality are provided below. These node-level centrality measures can be aggregated to reflect networks containing multiple nodes and links, such as at a suburb level, city level or any appropriate spatial area (which in our case was at the SA2 level). These area-level centrality measures are known as ‘network centrality’, where network centrality is defined as “the average difference between the relative centrality of the most central point and that of all other points” (Freeman, Citation1978). Therefore, higher values of network centrality reflect a network that has a greater number of streets/paths that serve as the only connection to other streets/paths (Zhang et al., Citation2015). Conversely, a gridded network tends to have a lower value of network centrality because all streets/paths in such a network are equally important and have the same possibility of connecting to others.
Betweenness centrality quantifies the number of times a node acts as a bridge along the shortest path between two other nodes, and is based on the idea that a node is central if it lies between many other nodes. Betweenness centrality, CB, is calculated as:
where
represents the number of node pairs
and
that contain point
on the shortest path connecting them and
represents the number of node pairs
and
Degree centrality measures to what extent a node is connected directly to other nodes, and is based on the idea that important nodes have the largest number of ties to other nodes in the graph. Degree centrality, is calculated as:
where
only if node
and node
are connected by a link or more, and is equal to zero otherwise, and
is the number of nodes in the network.
Because the aim of this study was to explore the association between network characteristics and bicycle trips at an SA2 level, betweenness centrality and degree centrality were aggregated to SA2s, as per Zhang et al. (Citation2015). The following equation was used to calculate aggregate measures of betweenness centrality and degree centrality () at an SA2 level:
where
is the centrality of SA2
and
is the largest possible value of
for the SA2.
2.5.3. Coverage
Three measures of connectivity and coverage were implemented in this study: network coverage, network density and intersection density (Kamel & Sayed, Citation2021; Osama & Sayed, Citation2016). Network coverage was calculated as the ratio of the number of bicycle network links to the number of street network links in the SA2. Network density was calculated as the ratio of the total length of bicycle network links in the SA2 to the SA2 area. Intersection density was calculated as the ratio of the number of intersections to the area of the SA2, including intersections between bicycle network links, and between bicycle network and street network links.
2.5.4. Topography
Topography was measured using the average weighted slope of the bicycle network within the SA2. Elevation data was sourced from the Victorian Government ‘Vicmap Elevation’ product, which includes a Digital Elevation Model (DEM) at 10 m grid resolution.(Victorian Department of Environment Land Water & Planning, Citation2021) The elevation data was applied to the OSM data using the ‘slopes’ package in R (ITS Leeds, Citation2020). The average weighted slope was then calculated by computing the bicycle network link slopes (), multiplying the slope by the length of the link (
), and then applying the following formula:
2.5.5. Calculating network metrics
In order to calculate network metrics, one needs to characterize the bicycle network as a graph. The links represent the bicycle network infrastructure (e.g. off-road path or on-road bike lane) and the nodes represent the intersections between network links (e.g. street and bicycle network links). Street and path networks, such as those represented in OSM data, are commonly represented by points which are effectively arbitrarily located, such as scenarios in which a curved path between two intersections is represented by a series of intermediate points (Gilardi et al., Citation2020). In these scenarios, it is necessary to ‘contract’ the network and remove these artifacts such that the network contains only edges that directly connect junctions. Following the guidance of Gilardi et al. (Citation2020), we used the R package ‘dodgr’ to contract the OSM network data and employed the ‘igraph’ package to calculate the aforementioned network metrics.
Data were prepared using the statistical software package R v4.0.3 (R Core Team, Citation2023) and the integrated development environment RStudio (RStudio 2020, Boston, MA, USA), using the ‘srvyr’, ‘tmap’, ‘survey’, ‘srvyr’, ‘dodgr’, ‘igraph’ and ‘slopes’ libraries (Posit team Citation2023).
2.6. Statistical analyses
We employed Bayesian spatial models to explore the association between network characteristics and bicycle ridership. A variety of methods have previously been used to explore associations between network characteristics and bicycle ridership. Berrigan et al. (Citation2010) employed a multivariate regression model (modeling a binary component of whether a person reported any active transport and a lognormal component for the number of minutes of active transport), Handy and Xing (Citation2011) employed a logistic regression model (modeling a binary outcome of commute mode), Marshall and Garrick (Citation2010) employed a multinomial logistic regression model (modeling four categories of mode choice), Schoner and Levinson (Citation2014) employed a linear regression model (modeling the rate of bicycle commuters per 10,000 commuters), while Osama et al. (Citation2017) and Kamel et al. (Citation2020) employed Bayesian hierarchical statistical models (modeling bike kilometers traveled). Model selection was largely driven by the type of outcome (such as binary, nominal, continuous). We chose to employ Bayesian spatial models as these models borrow strength across neighboring spatial areas, can utilize various distributions according to the outcome being modeled and can provide more accurate measures of uncertainty on the posterior distributions of the parameter estimates (Beck et al., Citation2022; El-Basyouny & Sayed, Citation2009).
We modeled two dependent variables: (1) the count of the number of trips; and (2) the proportion of all trips that were made by bike. These outcomes were spatially modeled according to Besag-York-Mollie (Besag et al., Citation1991), as described elsewhere (Blangiardo & Cameletti, Citation2015; Lawson, Citation2013).
Counts of the number of trips made by bike () were modeled as Poisson distributed with mean
Then, the logarithmic transform of is modeled as:
Where is the main effect,
is a vector of area-level covariates,
is the spatially structured effect (modeled using the intrinsic conditional autoregressive specification), and
is the spatially unstructured effect (modeled as normally distributed with mean 0). Poisson regression is used when the outcome variable represents count data, and the link function is the logarithm, as per Blangiardo and Cameletti (Citation2015).
The proportion of trips that were made by bike were modeled as Binomial distributed (accounting for the ratio of the number of trips made by bike over the total number of trips
). Then, the logistic transformation of
is modeled as:
Where is the main effect,
is a vector of area-level covariates,
is the spatially structured effect (modeled using the intrinsic conditional autoregressive specification), and
is the spatially unstructured effect (modeled as normally distributed with mean 0).
Similar to prior studies (Zhang et al., Citation2015), to inform the selection of covariates in the models, we firstly used correlation analyses to examine whether variables were highly correlated with each other. If two variables were found to be substantially correlated (defined as a Pearson’s product moment correlation coefficient greater than 0.5), they were not used in the same model. This left a total of 33 combinations of eight covariates (see Supplementary Material). All 33 models were run and evaluated and model fit was evaluated using the Deviance Information Criterion (DIC). The model with the lowest DIC was considered to have the best fit and selected as the final model.
Models were fitted using the INLA library (Rue et al., Citation2009) through the statistical software package R v4.0.3 (R Core Team, 2021) and the integrated development environment Rstudio (Rstudio 2020, Boston, MA, USA). The default prior distributions in INLA were employed.
2.7. Exploring the impact of the size of the study area
To explore the impact of the size of the study area on model covariates, we conducted sensitivity analyses by classifying Greater Melbourne into three sub-regions and running the aforementioned analyses in each of these sub-regions. Three sub-regions were defined based on ABS Statistical Areas Level 4 (SA4) areas. Specifically, ‘Inner Melbourne’ was defined as the SA4 areas of ‘Melbourne—Inner’, ‘Melbourne—Inner East’ and ‘Melbourne—Inner South’, ‘North and West Melbourne’ was defined as the SA4 areas of ‘Melbourne—West’, ‘Melbourne—North West’ and ‘Melbourne—North East’, and ‘South and East Melbourne’ was defined as the SA4 areas of ‘Melbourne—Outer East’, ‘Melbourne—South East’ and ‘Mornington Peninsula’. These areas are shown in .
2.8. Ethical approval
Ethical approval for this study was provided by the Monash University Human Research Ethics Committee (Project ID: 29210).
3. Results
On an average day in Greater Melbourne, there were 180,393 trips made by bike, reflecting 1.7% of all trips. The median number of bike trips across SA2s in Greater Melbourne was 222 trips (Q1: 64, Q3: 505) and the median proportion of trips made by bike was 0.8% (Q1: 0.3%, Q3: 1.6%) (). Spatial variation in the number of bike trips is shown in and spatial variation in the proportion of bike trips is shown in . The median length of off-road bicycle infrastructure per SA2 was 6.06 km (Q1: 2.50, Q3: 10.47) and the median length of on-road bicycle infrastructure per SA2 was 2.34 km (Q1: 0.22, Q3: 5.82). A map of bicycle infrastructure is shown in and further summary statistics of network characteristics are provided in . Spatial variation in network characteristics is shown in and . In general, on-road bicycle network length, network density, and intersection density were higher in the inner regions of Greater Melbourne, while off-road bicycle network length, degree centrality and average weighted slope were higher in the outer regions of Greater Melbourne.
Figure 3. Count of the number of bicycle trips (per SA2 area). Data reflect where trips commenced (the origin).

Figure 5. Map of bicycling infrastructure, stratified by infrastructure type. The grey borders reflect SA2 boundaries.

Figure 6. Network characteristics (per SA2). (a) Off-road bicycle network length; (b) on-road bicycle network length; (c) betweenness centrality; (d) degree centrality.

Figure 7. Network characteristics (per SA2). (a) network coverage; (b) network density; (c) intersection density; (d) average weighted slope.

Table 1. Summary statistics of bicycle ridership and network data across SA2s. Data presented for Greater Melbourne and for Inner Melbourne.
For the number of trips made by bike, the model with the lowest DIC included measures of degree centrality, off-road bicycle network length, on-road bicycle network length, and network density (). Off-road bicycle network length (mean: 0.08; 95% credible interval: 0.04, 0, 12) and on-road bicycle network length (mean: 0.14; 95% credible interval: 0.07, 0.22) were positively associated with the number of trips made by bike. Degree centrality (mean: 0.86; 95% credible interval: −3.29, 5.00) and network density (mean: −0.26, 95% credible interval: =0.54, 0.03) were not statistically credible at the 5% level.
Table 2. Summary model outputs for the count of the number of trips take by bike: posterior mean, posterior standard deviation (SD), and posterior 95% credible interval for the fixed effects of the covariates. This model relates to the Greater Melbourne region.
For the proportion of trips made by bike, the model with the lowest DIC included measures of intersection density and averaged weighted slope (). Neither of these measures were statistically credible at the 5% level.
Table 3. Summary model outputs for the model of the proportion of trips taken by bike: posterior mean, posterior standard deviation (SD), and posterior 95% credible interval for the fixed effects of the covariates. This model relates to the Greater Melbourne region.
Model fit parameters for all models are provided in Supplementary Material.
3.1. Sensitivity analysis
We conducted sensitivity analyses to explore the impact of the study area on model selection and model covariates. Comparisons of bicycle ridership and network characteristics between the overall study area of Greater Melbourne and the three sub-regions of Inner Melbourne, North and West Melbourne, and South and East Melbourne are presented in . In summary, median values of the count of the number of trips made by bike, the proportion of trips made by bike, the length of on-road bicycling infrastructure, network density and intersection density were higher in Inner Melbourne relative to North and West Melbourne and South and East Melbourne, while the length of off-road bicycling infrastructure was higher in North and West Melbourne and South and East Melbourne.
For the number of trips made by bike, variation was observed in model covariates, as well as the strength and direction of associations, between the overall study region and the three sub-regions (). For example, differences were observed in the inclusion of measures of bicycle network length, centrality and network coverage across all models. Where covariates were included in multiple models, there was some variation in the direction and strength of associations. For example, degree centrality was included in modes for both Inner Melbourne and North and West Melbourne. However, degree centrality was negatively associated with the number of trips made by bike in Inner Melbourne (mean: −12.34; 95% credible interval: −18.39, −7.04), but positively associated with the number of trips made by bike in North and West Melbourne (mean: 22.14; 95% credible interval: 8.27, 36.49). Additionally, off-road bicycle network length was positively associated with the number of trips made by bike for Greater Melbourne overall (mean: 0.08; 95% credible interval: 0.04, 0.12) and for North and West Melbourne (mean: 0.11; 95% credible interval: 0.05, 0.17), but was not associated with the number of trips made by bike in South and East Melbourne (mean: 0.06; 95% credible interval: −0.02, 0.14) and was not included as a covariate in the Inner Melbourne model.
Table 4. Summary model outputs for the count of the number of trips take by bike: posterior mean, posterior standard deviation (SD), and posterior 95% credible interval for the fixed effects of the covariates. Three separate models are presented for Inner Melbourne, North and West Melbourne, and South and East Melbourne.
For the proportion of trips made by bike, variation was observed in model covariates, as well as the strength and direction of associations, between the overall study region and the three sub-regions (). For example, while measures of off-road bicycle network length and on-road bicycle network length were included in the models for North and West Melbourne and South and East Melbourne, they were not included in the overall model nor the Inner Melbourne model. Further, as an example of differences in the strength and direction of associations, on-road bicycle network length was positively associated with the proportion of trips made by bike in North and West Melbourne (mean: 0.17; 95% credible interval: 0.06, 0.28)), but was not associated with the proportion of trips made by bike in South and East Melbourne (mean: −0.01, 95% credible interval: −0.16, 0.14).
Table 5. Summary model outputs for the model of the proportion of trips taken by bike: posterior mean, posterior standard deviation (SD), and posterior 95% credible interval for the fixed effects of the covariates. Three separate models are presented for Inner Melbourne, North and West Melbourne, and South and East Melbourne.
Model fit parameters for all models are provided in Supplementary Material.
4. Discussion
In this study of the bicycle network characteristics and bicycle ridership, we demonstrated that bicycling rates were associated with several network characteristics, and that these characteristics varied according to the outcome (count of the number of trips made by bike or the proportion of trips made by bike) and the size and characteristics of the study region. Given the sensitivity of these models to model inputs, these findings challenge the utility of approaches based on spatially modeling network characteristics and bicycle ridership when informing the monitoring and evaluation of bicycle networks.
Consistent with prior research, we demonstrated that the length of the bicycle network was positively associated with various measures of bicycle ridership (Kamel & Sayed, Citation2021; Kamel et al., Citation2020; Osama et al., Citation2017). A surprising finding was variation in the direction of association between degree centrality and bicycle ridership. In the inner-city region of Inner Melbourne, the finding of centrality being negatively associated with bicycle ridership is logical; high network centrality indicates low inter-connectivity and accessibility of the network (Zhang et al., Citation2015). However, degree centrality was strongly positively associated with bicycle ridership in the North and West Melbourne region. The association between low connectivity and bicycle ridership may be an artifact of a focus on off-road bicycle infrastructure (particularly rail trails; shared-use paths recycled from abandoned railway corridors) in these regions that, while they are not considered as connected and accessible using graph theory approaches, are supportive of recreational bicycling.
Consistent with previous modeling approaches (Kamel & Sayed, Citation2021; Lowry & Loh, Citation2017; Osama et al., Citation2017; Schoner & Levinson, Citation2014), we calculated network centrality measures using the combination of both on-road and off-road bicycling infrastructure. However, in our study region of Greater Melbourne, 99% of on-road bicycle infrastructure was painted bike lanes (with only 8 km of protected on-road bicycle lanes), which are inadequate in providing safe spaces for people on bikes and are likely only supportive of bicycling for the most confident riders (Beck et al., Citation2019; L. Pearson et al., Citation2022). Therefore, the network metrics used in this study are likely biased toward only the most confident riders, and may not reflect the needs of the diversity of bike riders. Given that prior studies used a similar approach, the influence of different types of bicycling infrastructure on network structure and bicycle ridership is not clear and further research is required to improve the quantification of network characteristics that relate to the needs of a diversity of bike riders. It is also important to consider the size and composition of the spatial area when modeling bicycle ridership. In this study, we modeled two outcomes: the count of the number of trips by bike, and the proportion of all trips made by bike. As depicted in , there is variation in both population density and the total population of each SA2. Modeling the count of the number of trips or the number of bicycle kilometers traveled, both of which are common approaches in the literature (Kamel & Sayed, Citation2021; Osama et al., Citation2017), is potentially confounded by the underlying population of the spatial area. For example, spatial areas with larger population sizes may have a greater number of bike riders, and therefore potentially a greater absolute number of bike trips and/or number of bicycle kilometers traveled. Without accounting for this, one may assume that an area has a greater rate of bike riding participation, when it may simply be an artifact of the underlying population. Therefore, normalizing these measures, either as a proportion of the population or as a proportion of all trips (as we have done), is needed to account for this confounding. Additionally, and similar to prior research (Kamel & Sayed, Citation2021; Osama et al., Citation2017; Schoner & Levinson, Citation2014), we explored the association between bicycle network length and bicycle ridership. Measures of bicycle length suffer from similar issues in that they may be related to the underlying size of the spatial area. To address this limitation, we explored normalized measures of bicycle infrastructure (network density and coverage), but further research is required to combine measures of both network coverage and network connectivity.
An interesting finding of this study was the impact of the size and characteristics of the study area had on model and covariate selection. Specifically, our sensitivity analyses on three sub-regions of the Greater Melbourne metropolitan region demonstrated inconsistencies in model selection and the strength and direction of associations between network characteristics and bicycle ridership. This is, perhaps, unsurprising as bicycle ridership and network characteristics vary vastly across large geographical regions, as we have demonstrated. This issue has been highlighted previously; Gil (Citation2017) demonstrated the impact of varying sizes of study areas on measures of street network centrality. Given that the vast majority of prior research has focused on inner city areas (Kamel & Sayed, Citation2021; Lowry & Loh, Citation2017; Osama et al., Citation2017), the generalizability of such findings, particularly to metropolitan areas outside of the inner city, must be questioned. It also raises the question of how robust such approaches are to defining important characteristics of bicycle networks.
It is well established that connected networks of safe and comfortable bicycling infrastructure, known as ‘All Ages and Abilities (AAA) bicycle networks’, are needed to enhance bike riding participation and safety, and thereby realize the potential for substantial gains in population health, equity and sustainability. Reflecting this, engineering guidance has focussed on providing tools to measure bicycling infrastructure on individual street segments, intersections or corridors. However, the absence of standardized definitions and supporting metrics to measure AAA bicycle networks has hindered the implementation of connected, safe and comfortable bicycle networks that enable people of all ages and abilities to get where they want to go. Certainly, the development of measures of ‘bicycle level of service’ (similar to ‘level of traffic stress’) has enhanced our understanding of how the actual and perceived environment is conducive and safe for bicycling (Kazemzadeh et al., Citation2020; Kellstedt et al., Citation2021). These measures include such factors as the presence, type and quality of bicycle infrastructure, topography, exposure to and speed of traffic, bicycle volumes, lighting, perceived and objective safety, and end of trip facilities (Kazemzadeh et al., Citation2020; Kellstedt et al., Citation2021). However, as previously described, these measures are often applied to individual street/path segments and challenges exist as to how to utilize these measures to defined AAA bicycle networks. Where the connectivity of networks has been considered, the measurement of connectivity has often been limited to the use of graph theory approaches, such as that employed in this study. The challenge with such approaches is that they do not consider the myriad of aforementioned factors that relate to comfort and safety. Our study is an example of this. Measures of connectivity used in this study included on-road bicycling infrastructure; the vast majority of which was on-road painted lanes. During the study period, only 8 km of the 1173 km (0.7%) of the on-road infrastructure were protected bike lanes (Beck et al., Citation2021), which are likely only supportive for the most confident riders (Beck et al., Citation2019; L. Pearson et al., Citation2022). This also reflects current ridership in our region in which the vast majority of people who ride bikes are considered ‘Strong and fearless’ (people who are comfortable riding with traffic in any road environment) and ‘Enthused and confident’ (people who are comfortable riding in traffic, but prefer bike lanes and like using segregated facilities) (L. Pearson et al., Citation2022). There has been some progress toward better characterizing bicycle networks, such as the use of multi-objective methodologies to assess bikeability between origin-destination locations over an entire network (Reggiani et al., Citation2022). However, it is evident that we need to advance the science of measuring AAA bicycle networks to support the implementation of such networks for health, sustainability and equity benefits.
The strengths of this study include the use of population-weighted travel survey data that enables robust evaluation of bike riding across small spatial areas in Greater Melbourne, and the evaluation of the association between network characteristics and bicycling across a large metropolitan area. However, there are a number of limitations to note. Firstly, due to a low number of bicycle trips, robust survey weights were only available at the SA3 level, and these were applied to SA2 areas. As a result, there may be some errors in the weightings applied the travel survey data. Further, survey weights were applied to the SA2 in which the trip originated, and the data presented do not reflect trips that occurred across multiple SA2s. Additionally, due to the relatively low number of trips made by bike, we were unable to stratify by trip purpose; it is likely that network characteristics that are supportive of bicycling may differ between trip types. In the absence of government data sets of bicycling infrastructure in Victoria, we were reliant on Open Street Map (OSM) data to characterize bicycling infrastructure. There is variability in the accuracy of OSM data in international settings (Ferster et al., Citation2020), and the accuracy of OSM data is unknown in our region. Additionally, as described above, network characteristics may not reflect how connected and comfortable a network is for all ages and abilities. Further, we did not consider land use and other parameters shown to be associated with bicycle ridership (Nelson et al., Citation2021; Winters et al., Citation2013), as the primary focus of this study was on the association between network characteristics and bicycle ridership.
5. Conclusion
We have demonstrated that the association between network characteristics and bicycle ridership varies according to the measure of bicycle ridership and the size and characteristics of the study area. These findings challenge the utility of approaches based on spatially modeling network characteristics and bicycle ridership, and highlight the impact of the study area on associations between these characteristics and bicycle ridership. While we employed robust measures of network connectivity, these measures do not consider comfort and safety. There is a need to advance how we characterize the connectivity and comfort of bicycling networks that reflect the needs of people of all ages and abilities.
Supplemental Material
Download PDF (403.6 KB)Acknowledgements
The authors would like to acknowledge the assistance provided by the Victorian Department of Transport, and in particular, Geoffrey Simmons.
Disclosure statement
There are no conflicts of interest to declare.
Additional information
Funding
References
- Australian Bureau of Statistics. (2020). Australian Bureau of Statistics: Data by Region. https://itt.abs.gov.au/itt/r.jsp?databyregion
- Beck, B., Chong, D., Olivier, J., Perkins, M., Tsay, A., Rushford, A., Li, L., Cameron, P., Fry, R., & Johnson, M. (2019). How much space do drivers provide when passing cyclists? Understanding the impact of motor vehicle and infrastructure characteristics on passing distance. Accident; Analysis and Prevention, 128, 253–260. https://doi.org/10.1016/j.aap.2019.03.007
- Beck, B., Winters, M., Thompson, J., Stevenson, M., & Pettit, C. (2021). Spatial variation in bicycling: A retrospective review of travel survey data from Greater. Melbourne, Australia. SocArXiv. https://doi.org/10.31235/osf.io/78qgf
- Beck, B., Zammit-Mangion, A., Fry, R., Smith, K., & Gabbe, B. (2022). Spatiotemporal mapping of major trauma in Victoria, Australia. PloS One, 17(7), e0266521. https://doi.org/10.1371/journal.pone.0266521
- Berrigan, D., Pickle, L. W., & Dill, J. (2010). Associations between street connectivity and active transportation. International Journal of Health Geographics, 9(1), 20. https://doi.org/10.1186/1476-072X-9-20
- Besag, J., York, J., & Mollie, A. (1991). Bayesian image restoration with two applications in spatial statistics. Annals of the Institute of Statistical Mathematics, 43(1), 1–20. https://doi.org/10.1007/BF00116466
- Blangiardo, M., & Cameletti, M. (2015). Spatial and spatio-temporal Bayesian models with R-INLA. John Wiley & Sons.
- Boisjoly, G., Lachapelle, U., & El-Geneidy, A. (2020). Bicycle network performance: Assessing the directness of bicycle facilities through connectivity measures, a Montreal, Canada case study. International Journal of Sustainable Transportation, 14(8), 620–634. https://doi.org/10.1080/15568318.2019.1595791
- Buehler, R., & Pucher, J. (2012). Cycling to work in 90 large American cities: New evidence on the role of bike paths and lanes. Transportation, 39(2), 409–432. https://doi.org/10.1007/s11116-011-9355-8
- El-Basyouny, K., & Sayed, T. (2009). Urban arterial accident prediction models with spatial effects. Transportation Research Record: Journal of the Transportation Research Board, 2102(1), 27–33. https://doi.org/10.3141/2102-04
- Ferster, C., Fischer, J., Manaugh, K., Nelson, T., & Winters, M. (2020). Using OpenStreetMap to inventory bicycle infrastructure: A comparison with open data from cities. International Journal of Sustainable Transportation, 14(1), 64–73. https://doi.org/10.1080/15568318.2018.1519746
- Freeman, L. C. (1978). Centrality in social networks conceptual clarification. Social Networks, 1(3), 215–239. https://doi.org/10.1016/0378-8733(78)90021-7
- Geofrabrik (2021). GEOFABRIK//Downloads. https://www.geofabrik.de/data/download.html
- Gil, J. (2017). Street network analysis “edge effects”: Examining the sensitivity of centrality measures to boundary conditions. Environment and Planning B: Urban Analytics and City Science, 44(5), 819–836. https://doi.org/10.1177/0265813516650678
- Gilardi, A., Lovelace, R., & Padgham, M. (2020). Data structures and methods for reproducible street network analysis: Overview and implementations in R. OSF Preprints. https://doi.org/10.31219/osf.io/78yub
- Handy, S. L., & Xing, Y. (2011). Factors correlated with bicycle commuting: A study in six small US cities. International Journal of Sustainable Transportation, 5(2), 91–110. https://doi.org/10.1080/15568310903514789
- ITS Leeds (2020). Slopes package. https://github.com/ropensci/slopes
- Kamel, M. B., & Sayed, T. (2021). The impact of bike network indicators on bike kilometers traveled and bike safety: A network theory approach. Environment and Planning B: Urban Analytics and City Science, 48(7), 2055–2072.
- Kamel, M. B., Sayed, T., & Bigazzi, A. (2020). A composite zonal index for biking attractiveness and safety. Accident; Analysis and Prevention, 137, 105439. https://doi.org/10.1016/j.aap.2020.105439
- Kazemzadeh, K., Laureshyn, A., Winslott Hiselius, L., & Ronchi, E. (2020). Expanding the scope of the bicycle level-of-service concept: A review of the literature. Sustainability, 12(7), 2944. https://doi.org/10.3390/su12072944
- Kellstedt, D. K., Spengler, J. O., Foster, M., Lee, C., & Maddock, J. E. (2021). A scoping review of bikeability assessment methods. Journal of Community Health, 46(1), 211–224. https://doi.org/10.1007/s10900-020-00846-4
- Kraus, S., & Koch, N. (2021). Provisional COVID-19 infrastructure induces large, rapid increases in cycling. Proceedings of the National Academy of Sciences, 118(15). https://doi.org/10.1073/pnas.2024399118
- Lawson, A. B. (2013). Bayesian disease mapping: Hierarchical modeling in spatial epidemiology. Chapman and Hall/CRC.
- Lowry, M., & Loh, T. H. (2017). Quantifying bicycle network connectivity. Preventive Medicine, 95 Suppl, S134–S140. https://doi.org/10.1016/j.ypmed.2016.12.007
- Marshall, W. E., & Garrick, N. W. (2010). Effect of street network design on walking and biking. Transportation Research Record: Journal of the Transportation Research Board, 2198(1), 103–115. https://doi.org/10.3141/2198-12
- Nelson, T., Roy, A., Ferster, C., Fischer, J., Brum-Bastos, V., Laberee, K., Yu, H., & Winters, M. (2021). Generalized model for mapping bicycle ridership with crowdsourced data. Transportation Research Part C: Emerging Technologies, 125, 102981. https://doi.org/10.1016/j.trc.2021.102981
- OpenStreetMap. (2021). OpenStreetMap Wiki. https://wiki.openstreetmap.org/wiki/Main_Page
- Osama, A., & Sayed, T. (2016). Evaluating the impact of bike network indicators on cyclist safety using macro-level collision prediction models. Accident; Analysis and Prevention, 97, 28–37. https://doi.org/10.1016/j.aap.2016.08.010
- Osama, A., Sayed, T., & Bigazzi, A. Y. (2017). Models for estimating zone-level bike kilometers traveled using bike network, land use, and road facility variables. Transportation Research Part A: Policy and Practice, 96, 14–28. https://doi.org/10.1016/j.tra.2016.11.016
- Pearson, L., Dipnall, J., Gabbe, B., Braaf, S., White, S., Backhouse, M., & Beck, B. (2022). The potential for bike riding across entire cities: Quantifying spatial variation in interest in bike riding. Journal of Transport & Health, 24, 101290. https://doi.org/10.1016/j.jth.2021.101290
- Pearson, L., Gabbe, B., Reeder, S., & Beck, B. (2023). Barriers and enablers of bike riding for transport and recreational purposes in Australia. Journal of Transport & Health, 28, 101538. https://doi.org/10.1016/j.jth.2022.101538
- Pearson, L. K., Berkovic, D., Reeder, S., Gabbe, B. J., & Beck, B. (2022). Adults’ self-reported barriers and enablers to riding a bike for transport: A systematic review. Transport Reviews, 43(3), 356–384. https://doi.org/10.1080/01441647.2022.2113570
- Posit team (2023). RStudio: Integrated Development Environment for R. Posit Software, PBC, Boston, MA. http://www.posit.co/.
- Pucher, J., & Buehler, R. (2017). Cycling towards a more sustainable transport future. Transport Reviews, 37(6), 689–694. https://doi.org/10.1080/01441647.2017.1340234
- R Core Team (2023). R: A language and environment for statistical ## computing. R Foundation for Statistical Computing, Vienna, Austria. https://www.R-project.org/.
- Reggiani, G., van Oijen, T., Hamedmoghadam, H., Daamen, W., Vu, H. L., & Hoogendoorn, S. (2022). Understanding bikeability: A methodology to assess urban networks. Transportation, 49(3), 897–925.
- Rue, H., Martino, S., & Chopin, N. (2009). Approximate Bayesian inference for latent Gaussian models by using integrated nested Laplace approximations. Journal of the Royal Statistical Society Series B: Statistical Methodology, 71(2), 319–392. https://doi.org/10.1111/j.1467-9868.2008.00700.x
- Schoner, J. E., & Levinson, D. M. (2014). The missing link: Bicycle infrastructure networks and ridership in 74 US cities. Transportation, 41(6), 1187–1204. https://doi.org/10.1007/s11116-014-9538-1
- UK Department of Transport. (2020). Gear change: A bold vision for cycling and walking.
- Victorian Department of Environment Land Water and Planning. (2021). Vicmap elevation. https://www.land.vic.gov.au/maps-and-spatial/spatial-data/vicmap-catalogue/vicmap-elevation
- Winters, M., Brauer, M., Setton, E. M., & Teschke, K. (2013). Mapping bikeability: A spatial tool to support sustainable travel. Environment and Planning B: Planning and Design, 40(5), 865–883. https://doi.org/10.1068/b38185
- Zhang, Y., Bigham, J., Ragland, D., & Chen, X. (2015). Investigating the associations between road network structure and non-motorist accidents. Journal of Transport Geography, 42, 34–47. https://doi.org/10.1016/j.jtrangeo.2014.10.010