Abstract
Objectives
Schizophrenia genetics is intricate, with common and rare variants’ contributions not fully understood. Certain copy number variations (CNVs) elevate risk, pivotal for understanding mental disorder models. Despite CNVs’ genome-wide distribution and variable gene and protein effects, we must explore beyond affected genes to interaction partners and molecular pathways.
Methods
In this study, we developed machine-readable interactive pathways to enable analysis of functional effects of genes within CNV loci and identify ten common pathways across CNVs with high schizophrenia risk using the WikiPathways database, schizophrenia risk gene collections from GWAS studies, and a gene-disease association database.
Results
For CNVs that are pathogenic for schizophrenia, we found overlapping pathways, including BDNF signalling, cytoskeleton, and inflammation. Common schizophrenia risk genes identified by different studies are found in all CNV pathways, but not enriched.
Conclusions
Our findings suggest that specific pathways - BDNF signalling - are critical contributors to schizophrenia risk conferred by rare CNVs. Our approach highlights the importance of not only investigating deleted or duplicated genes within pathogenic CNV loci, but also study their direct interaction partners, which may explain pleiotropic effects of CNVs on schizophrenia risk and offer a broader field for interventions.
Introduction
The identification of rare and common genetic risk variants of schizophrenia has been one of the recent success stories of biological psychiatry. However, the understanding of the underlying molecular mechanisms that is shared among all these different genes is lagging behind. Genome Wide Association Studies (GWAS) identified several hundred common risk variants, but each locus contributes only a small amount to the total risk (Schizophrenia Working Group of the Psychiatric Genomics C Citation2014; Zhuo et al. Citation2019). Furthermore, many of the implicated genes were also identified as risk genes for other mental disorders with complex, multifactorial genetics and additional environmental risks factors like autism spectrum disorder, attention deficit/hyperactivity disorder, bipolar disorder and depression (Prata et al. Citation2019; Zhuo et al. Citation2019). On a molecular level, the risk genes are clustered around certain biological processes and pathways that can interact with environmental events. In systems biology, a pathway is defined as a series of interactions between genes, gene products or metabolites that lead to a product or change in a cell. In its most recent study, the Schizophrenia Working Group of the Psychiatric Genomics Consortium (PGC), identified 270 distinct genetic loci, enriched around pathways of neuronal excitability, development and synaptic structure (Trubetskoy et al. Citation2022).
In addition to these associations in common, low risk variants, which usually are single nucleotide polymorphisms (SNPs), a number of rare copy number variants (CNVs) are recognised as highly penetrant risk factors for schizophrenia (Walsh et al. Citation2008; Kirov et al. Citation2014; Marshall et al. Citation2017). How a CNV causes the disturbances in the metabolic and signalling network of the organism can be explained by several mechanisms. 1) Direct gene dosage effects, 2) unmasking of recessive variants on the unaffected chromosome (in the case of deletions) (Pardiñas et al. Citation2018; Cleynen et al. Citation2020), and 3) functional effects on interaction partners of the affected genes, respectively, their proteins, including those caused by nuclear reorganisation. Analysis of this last group of effects may give rise to the identification of affected functional pathways, some of which may overlap across CNVs.
Although these highly penetrant rare genetic variants can provide powerful biological models for schizophrenia and other neurodevelopmental disorders, their explanatory power and ability to yield models with high construct validity has so far been limited by a number of factors. Almost all of these CNVs include several genes, and most of these genes can be plausibly implicated in the pathophysiology of the neurodevelopmental changes (Magrinelli et al. Citation2021). There is considerable locus heterogeneity - different groups of deleted or duplicated genes leading to very similar psychiatric phenotypes. The question here is what these CNVs have in common to increase so consistently the risk of developing schizophrenia? There is also considerable phenotypic heterogeneity and variable penetrance - suggesting an important role for genetic and environmental modifiers (Cleynen et al. Citation2020).
Biological pathway schemes are an intuitive way to capture, understand and study such complex interactions and compare the functional effects of different CNVs. Pathway databases like WikiPathways (Martens et al. Citation2021) enable the creation and modification of pathways and make them openly available for the research community. With machine-readable identifier annotations for biological entities (genes, proteins, RNA, metabolites), interactions and literature references it is possible to use these pathways for visualisation and automated analysis, integration and comparison with other data resources.
In this study, we created such machine-readable pathway models that capture the genes of the schizophrenia risk CNVs and their direct interaction partners in order to find overlapping pathways or processes that might explain their increased risk and their convergence onto a unitary psychiatric phenotype. We furthermore investigated if these pathways host more known schizophrenia risk genes than expected by average distribution across the genome.
Materials and methods
CNV selection
The CNV loci with an increased risk of psychiatric disorders (through deletion, duplication or both) were selected as proposed by Kirov et al. (Citation2014) and Marshall et al. (Citation2017) include 1q21.1, 3q29, 7q11.23, 8q11.23, 15q11.2, 15q13.3, Prader Willi and Angelman syndrome region (15q11-q13), 16p11.2, and 22q11.2. The selection was based on the increased risk association done by these two publications individually. The CNVs listed above include the CNVs with the highest risks on developing a psychiatric disorder.
Pathway construction
To construct the pathways, PathVisio pathway drawing software was used (Kutmon et al. Citation2015) including gene product and metabolite mapping databases from BridgeDb (van Iersel et al. Citation2010). First, for each CNV, a list of genes located in the typically deleted or duplicated region was queried from Ensembl genome browser, Human Genome Build GRCh37/hg19 using BioMart (Yates et al. Citation2016). The exact genome positions are summarised with the respective references in . The genes were imported to PathVisio and different sources, such as scientific literature and databases, were investigated to find information, reactions, interaction partners and downstream pathways for the protein coding genes. A detailed description of the search strategy is given in Supplementary Information 1.
Table 1. CNV pathways created in this study and presentation of schizophrenia risk genes from different sources in those pathways by number and enrichment score (z-score).
The nodes in the pathway, such as genes, proteins, RNA and metabolites, were annotated with Ensembl (genes and gene products) or ChEBI (metabolites) identifiers. Interactions were annotated with MIM identifiers, which indicate the nature of interaction and, if available, with their respective RHEA identifiers [www.rhea-db.org]. The interactions also bear the identifier of the source, usually a PubMed identifier of the study that describes the interaction.
Network construction
Cytoscape (Shannon et al. Citation2003) and associated packages (WikiPathways (Kutmon et al. Citation2014), RCy3 (Gustavsen et al. Citation2019)) were used to import the new CNV pathways to Cytoscape, extend them with pathway information (CyTargetLinker (Kutmon et al. Citation2018)), merge, and analyse them. WikiPathways database was queried August 2023. The WikiPathways linksets wikipathways-hsa-20220511 and wikipathways-hsa-Reactome-20220511 were used.
Schizophrenia risk genes
Lists of known schizophrenia risk genes were acquired from two different resources. First, we used a recent publication with a list of schizophrenia-associated loci, identified by GWAS. 2121 associated genes were extracted from these loci available from the Supplementary Table 3 published by the Schizophrenia working group of the PGC (Trubetskoy et al. Citation2022). 1756 genes could be mapped to an Ensembl gene identifier and are used as ‘PGC-all’ gene set. The same authors filtered and prioritised this initial list to 120 high confidence risk genes (of which 106 were protein coding), these we used as ‘PGC-filtered’ gene set.
Second, we consulted the DisGeNET database (Piñero et al. Citation2017) [Dec 1st, 2020] for gene-disease and variant-disease associations from multiple curated resources. We used the DisGeNET search function to extract genes and genetic variants associated with schizophrenia [CUI: C0036341]. A relatively high gene-disease-association score threshold of 0.3 was chosen which means that the information on the gene-disease association was included from a minimum of one curated source (0.3), or a combination of ‘inferred from animal models’ (0.2 score) plus at least one inferred source (0.1) or number of publications > 9 (0.1). For variant-disease-associations a filter of 0.7 (minimum 1 curated source) was chosen. These scores were selected for a relatively high confidence gene-disease association. For detailed information on thresholds see DisGeNET documentation [https://www.disgenet.org/dbinfo]. The variants were mapped to genes before further use. The combination of both the gene and variant derived gene lists was used as ‘merged’, ‘Dis-M’, the intersection of both lists as ‘Dis-I’.
All gene lists are available in Supplementary Table 1.
Overrepresentation analysis
Pathway overrepresentation analysis was done using PathVisio statistics function described in Kutmon et al. (Citation2015). This approach is based on a commonly used overrepresentation statistics, calculating the z-score, which gives an indication if there are more genes from a chosen dataset – in this case, the risk gene lists - found in a specific pathway, compared to the number of genes expected by chance. The background list was a standard list of protein coding genes from Ensembl containing about 20 000 human genes. The pathway collection was downloaded from WikiPathways database (version 20230410), the updated PW/AS and the new 8q21.11 pathway were included manually.
Results
Pathway creation
We created pathway models for the defined CNV syndromes identified by Kirov et al. (Citation2014) and Marshall et al. (Citation2017) in order to provide the available interaction knowledge of the affected genes in a machine-readable way that enables further analysis. These pathways are publicly available on WikiPathways http://wikipathways.org. summarises the pathways and their main characteristics. shows an example of one of these pathways. Note that for about 10% of the protein coding genes in these affected regions, no information on their function or interactions was available at the time of data extraction (August 2023).
Figure 1. 1q21.1. Copy number variation pathway WP4905. The red arrow indicates the position of the deleted genes on the chromosome. Protein coding gene are black boxes, pseudogenes grey, RNA genes purple, metabolites blue and pathways green. The interactions are annotated interactions carrying the information about the nature of interaction for automated analysis. The small numbers indicate the literature references http://www.wikipathways.org/instance/WP4905.
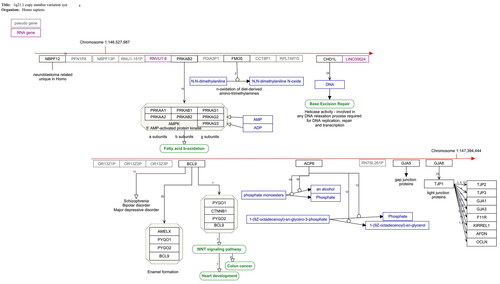
CNV pathway overlap analysis
To identify potential overlap of the different CNV pathway models that might explain common observed symptoms, the pathways were imported to Cytoscape, extended with gene-pathway information from WikiPathways database adding known pathways to a gene, forming a gene-pathway network. Pathways that share genes with more than one CNV were extracted to identify functional links between CNVs. All CNVs shared genes from the ‘Brain-Derived Neurotrophic Factor (BDNF) signaling pathway’. When the smallest pathway (15q11.2, with four protein coding genes) was removed, there were further nine pathways (out of a total of 1453 pathways approved for data analysis in the database) which shared genes with the remaining CNV pathways: ‘Ciliary landscape’, ‘Leptin signalling pathway’, ‘VEGFA-VEGFR2 Signalling Pathway’, ‘Alzheimer’s disease’, ‘Alzheimer’s disease and miRNA effects’, ‘IL-18 signalling pathway’, ‘Focal Adhesion’, ‘Pleural mesothelioma’ and ‘Gastrin Signalling Pathway’ (). Nine of ten CNV pathways (excluding 15q11.2) shared genes with at least one, but usually several, WNT signalling pathways. Moreover, multiple links to different pathways for general signalling hubs like MAPK, JUN, and TP53 were found in almost all pathways.
Table 2. Shared pathways between the different CNVs and their z-scores from schizophrenia risk gene overrepresentation analysis.
In a second step, the extended CNV pathways were merged, and network analysis revealed the most densely connected nodes within the network indicating hubs of importance (Supplementary Table 2). The larger CNV (22q11.2, 7q11.23, 16p11.2 prox, 3q29 and PW/AS) pathways were among the top ten but were removed from the list in order to avoid redundancy. Among the most connected nodes are well known transcription factors like NFKB1, MYC and TP53 and multi-functional kinases, such as MAPK3, JUN, and RAF1. Inflammation mediators AKT1, CTNNB1 and TNF could be linked to the immune system and inflammation pathways connected. The full network is available on Ndex database [https://doi.org/10.18119/N9QC84].
The links between pathways formed by shared genes are defined by prior knowledge from experimental evidence as stated in the literature references of the individual pathways. However, to investigate how likely it is that a random gene set of similar size as the CNV pathways shares genes with the pathways listed in , permutation statistics were done (Supplementary Information 2). In short, it is highly unlikely that the connection patterns we found could have occurred by chance. The number of randomly overlapping genes is, as expected, strongly dependent on the size of the pathways. For example, an average of 4.2 ± 1.3 of ten pathways share a random gene with the BDNF pathway (which has 144 genes) by chance. The actual overlap was much higher also for the other pathways investigated, again depending on their size. The VEGFA-VEGFR2 signalling pathway and the Pleural mesothelioma pathway, the largest pathways in this study with 431, respectively 451, genes, were an exception. They showed a high random overlap with the CNV pathways due to their size.
To investigate the benefit of functional interaction partners to the genes affected by CNVs another network was created using only the genes deleted/duplicated in these CNVs. In this approach, no overlapping pathways between the different CNVs were found (data not shown).
The genes, which form the connections between the CNV pathways and the nine pathways () were isolated and visualised in . As the BDNF pathway is of special interest in brain function and development, the network connecting it with the CNV pathways is shown in detail in . In the BDNF signalling pathway network (), most genes connect to one of the CNV pathways. There are also three genes, which are present in the BDNF pathway and more than one CNV pathway: CTNNB1, which is also one of the most connected hub genes in the network, FYN, and GRB2.
Figure 2. Pathway overlap between the different CNVs. (a) The nine CNV pathways (violet octagons with red borders) (except 15q11.2) share genes (green spheres) with a group of pathways (violet octagons, list in ) that are known to play a role in schizophrenia development. (b) BDNF pathway shares genes (red border) with each of the investigated CNV pathways.
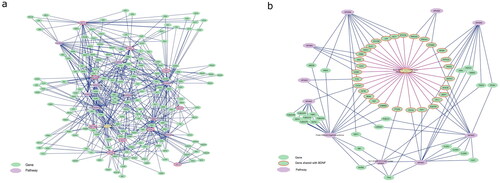
Comparison with known schizophrenia risk genes
There were 2121 genes extracted from the loci identified by the most recent GWAS study of schizophrenia risk genes and 1756 could be mapped to Ensembl gene identifiers (PGC-all). Additionally, we used the filtered and prioritised list with 120 high-confidence risk genes also provided from the PGC study (PGC-filtered).
The DisGeNET variant-disease association list for schizophrenia contained 2897 unique variants. 2330 variants were mapped to 1 392 different protein-coding genes, after filtering for a minimum VDA score of 0.7 to 811 genes. The gene-disease-association list contained 2872 genes, after filtering for a minimum GDA score of 0.3 1026 genes. These two DisGeNET lists share 113 genes (intersection, Dis-I). The merged list of both, gene and variant-disease associations, contains 1725 genes (merged, Dis-M).
There is some overlap between the different gene sets (). DisGeNET includes gene disease and gene variant information, among others, from the NHGRI-EBI GWAS Catalog. The number of genes listed there is lower than given by the original authors because DisGeNET itself filters out variants with p > 1.0 × 10−6 and a pre-defined group of variant consequences.
Figure 3. Overlap in schizophrenia risk genes extracted from GWAS studies and DisGeNET database. PGC-all and PGC-filtered (Trubetskoy et al. Citation2022), Dis-I = intersection/overlap between DisGeNET’s gene-disease and variant-disease associated genes for schizophrenia, Dis-M = merge of DisGeNET’s gene-disease and variant-disease associated genes for schizophrenia.
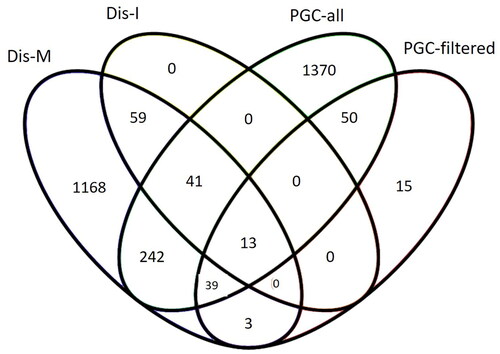
Visualisation and overrepresentation of schizophrenia risk genes in CNV pathways
also shows the number of schizophrenia risk genes in the different CNV pathways. We indicated how many risk genes are located specifically in the affected CNV locus and how many are found in the whole pathway (Supplementary pathway figures). Overrepresentation analysis z-scores above 1.96 show that these pathways host more than the average number of schizophrenia risk genes expected by chance for this dataset.
The PGC datasets identified a part of the 16p11.2 proximal locus as a risk locus. The PGC-all gene set shares 24 genes in the pathway, and after filtering and prioritisation there were still three risk genes. The 8q11.23 pathway also contains a risk gene from the PGC-filtered gene set. Consequently, these pathways got a high enrichment score. Notably, apart from the 8q11.23 and the 16p11.2 proximal pathways, there are no enriched pathways although there were 13 genes of the 22q11.2 and 9 genes in the 7q11.23 pathway found but hardly any risk genes identified by the PGC datasets in the other CNV pathways ().
Schizophrenia-associated genes from the DisGeNET dataset are found in the 22q11.2 (both Dis-I and Dis-M), 15q13.3 and Prader-Willi/Angelman syndrome pathways. As the PGC-all (1756) and the Dis-M (1725), and the PGC-filtered (120) and Dis-I (113) datasets are of comparable size we presume that this is not primarily an effect of dataset size.
Pathway overrepresentation analysis results
To investigate which pathways, other than CNV pathways, contained an increased number of schizophrenia risk genes a pathway overrepresentation analysis was performed using the different risk gene lists and the WikiPathways database (full results in Supplementary Table 3). For all lists, the ‘Nicotine effect on chromaffin cells’, ‘Prion disease pathway’, ‘Rett syndrome causing genes’, ‘Synaptic signaling pathways associated with autism spectrum disorder’ and ‘GPCRs, class C metabotropic glutamate, pheromone’ were enriched. Common in all datasets were further: pathways concerning neurological function (e.g. ‘Nicotine Activity on Dopaminergic Neurons’, ‘PKC-gamma calcium signalling pathway in ataxia’, ‘Disruption of postsynaptic signalling by CNV’), lipid metabolism (‘Lipid homeostasis’, ‘Arachidonate Epoxygenase/Epoxide Hydrolase’), immune system (‘Complement and Coagulation Cascades’), WNT signalling (‘Regulation of Wnt/B-catenin Signalling by Small Molecule Compounds’) and ‘Genes involved in male infertility’. Notably, most of the overlapping pathways between the different CNVs are enriched with at least one schizophrenia risk gene list – mostly the Dis-M (). The only exceptions are the pathways associated with cytoskeleton and cilia function. ‘Ciliary landscape’ has a negative z-score in three of the four gene sets, indicating a smaller number of risk genes than expected by chance. The PGC-filtered gene set results matches with the analysis provided in the original paper (PGC2022), which found the highest z-scores on ‘Synaptic signaling pathways associated with autism spectrum disorder’ and ‘Disruption of postsynaptic signaling by CNV’.
Discussion
Resource creation
In this study, we created pathway models for CNVs with a high penetrance for schizophrenia, which serve as a bioinformatics and data analysis resource to enable pathway and network modelling and analysis of any kind of omics data. The pathways are results of manual literature review, which was translated to fully machine-readable pathways, annotated with unique, persistent identifiers from resources like Ensembl, UniProt or ChEBI, and provide the provenance for every single statement in form of literature references. The pathways are published in the WikiPathways database and will undergo regular curation and updates (Martens et al. Citation2021).
Overlapping pathways analysis
Using the information about functional interaction partners of the deleted/duplicated genes in the different CNVs it was possible to identify pathways on which these CNVs converge (). These shared pathways align with previously known pathways and biological processes affected in schizophrenia:
1) Neuronal development and function, as represented by the BDNF pathway. All investigated CNV pathways have direct links to BDNF-triggered downstream functions () (NetPath et al. Citation2021) but also to other processes that are central to neuronal development. For example, CYFIP1 (located in the 15q11.2 locus, but due to genetic overlap also present in the ‘Prader-Willi/Angelman syndrome’ pathway) is found in the ‘Fragile X syndrome’ pathway, interacts in the wave regulatory complex to regulate cytoskeleton remodelling by regulating the production of f-actin, and is generally important for brain development (De Rubeis et al. Citation2013; Silva et al. Citation2019). Our findings provide explanation and support results previous therapeutic intervention studies that have identified BDNF as a target of schizophrenia treatment by increasing BDNF levels e.g. by exercise (Fisher et al. Citation2020; Tripathi et al. Citation2023).
2) Cytoskeleton and cell-cell connections, including ciliary landscape, gap junction (note that two gap junction proteins (GJA5, GJA8) are located in the 1q21 region and associated with cardiac and ocular phenotypes (Nielsen et al. Citation2003)) and focal adhesion pathways. These contain proteins that are also involved in synaptic structure, e.g. KCTD13 (16p11.2 prox locus) (Golzio et al. Citation2012). The region 8q11.23 hosts one specific gene, RB1CC1, which is an inhibitor of PTK2, focal adhesion kinase, which is responsible for cell mobility (Abbi et al. Citation2002). In addition to its role in axonal growth, CTNNB1, which is part of the 1q21.1 and 7q11.23 pathways, can also be found in the Ciliary landscape pathway (Boldt et al. Citation2016).
3) Immune system pathways are also highly affected and connected within all CNV pathways, specifically, the IL-18 signalling pathway. Lipid metabolism pathways may be included here as metabolic inflammation mediators (Le Hellard et al. Citation2010).
Common signalling hubs, like the different WNT, PI3K-Akt and the MAPK3 pathways, were identified as overlapping between CNVs. MAPK3 is located at 16p11.2 and therefore directly affected by deletion/duplication but due to its broad action range it also affects almost all other CNV pathways (Blizinsky et al. Citation2016). Generally, MAPK3 and several other kinases and transcription factors such as AKT1, NKFB1, JUN and TP53 tend to show up as network hubs because they are involved in so many different processes and intensively studied. Thus, their involvement in the CNV pathways may not be particularly specific, but still contribute to disease development.
CTNNB1 is a highly connected hub gene in the network and it is one of the genes connecting two CNV pathways (1q21.1 and 7q11.23) and the BDNF signalling pathway (Supplementary Table 3, ). In the 1q21.1 pathway it is a binding partner in a complex with BCL9 (whose gene is located in 1q21.1), PYGO1 and PYGO2, contributing to the WNT signalling pathway. In the 7q11.23 CNV pathway it is inhibited by BCL7B (whose gene is located in the 7q11.23 region), which is therefore also an inhibitor of the WNT signalling pathway. WikiPathways hosts several individual WNT signalling pathways that cover different aspects of up- or downstream effects. Most CNVs share a link to at least one of those WNT signalling pathways, which makes it an interesting pathway to study for these disorders.
To conclude, using the CNV pathways, it was possible to identify common processes shared by all these CNVs with high risk of developing schizophrenia, which may lead to new methods for targeting these pathways for a better understanding of disease biology and, ultimately, early diagnosis and treatment.
Investigation of schizophrenia risk gene distribution and identification in the CNV pathways
The identification of these CNVs and their shared pathways invites the question of genetic links between these (relatively) rare forms of schizophrenia and the large number of common variants identified by GWAS. One could hypothesise that the pathways we identified based on convergence of CNV pathways also host a number of schizophrenia risk genes with common risk variants, higher than expected by average. To answer this question we conducted overrepresentation analysis and chose two different collections of schizophrenia risk genes: The first datasets come from the currently largest and most recent GWAS study from the PGC’s study from 2022 (Trubetskoy et al. Citation2022) (PGC-all and PGC-filtered). Integrating more than 300 000 participants makes it the currently largest GWAS study identifying common variants with low risk contribution to schizophrenia. The other datasets are from the gene-disease association database DisGeNET (Piñero et al. Citation2017) (Dis-M, Dis-I). DisGeNET collects a broader spectrum of gene-disease and variant-disease associations by integrating curated databases, animal model data, inferred data and literature (Piñero et al. Citation2017). In contrast to GWAS data, DisGeNET includes both common and rare variants in the gene-disease associations and provides a score indicating amount and quality of references for each.
Although using different overrepresentation analysis tools, the result of the pathway overrepresentation analysis of the GWAS study (‘neuronal function, including synaptic organization, differentiation and transmission’ (Trubetskoy et al. Citation2022)) overlaps with our analysis results showing neuronal function pathways among the highest enriched pathways.
Visualising the schizophrenia risk genes in the CNV pathways showed clearly that all pathways host schizophrenia risk genes from at least two of four gene sets. These risk genes are not necessarily located in the deleted region but there are always some direct interaction partners of the deleted genes, which are suspected to be a risk gene. Given the about 2000 potential schizophrenia risk genes (which is about 10% of the human genome!) this is not surprising.
The question is, whether there is an unusual accumulation of risk genes in these pathways. To summarise the results, overrepresentation analysis showed that, with exceptions (15q13.2, Prader-Willi/Angelman syndrome region, 22q11.2 (rare and common variants from DisGeNET), 8q23.11 and 16p11.2 prox (common variants from GWAS)), the CNV pathways are not hosting more than the expected average number of schizophrenia risk genes. But almost all of the overlapping pathways between the CNVs do – with the risk gene set that includes both, rare and common variants. The more restricted gene lists (PGC-filtered and Dis-I) are found overrepresented in the three neuronal pathways (with one exception). The 22q11.2 CNV pathway is highly enriched for the DisGeNET datasets and is also a CNV with a particularly high schizophrenia risk (Kirov et al. Citation2014; Kendall et al. Citation2017). 16p11.2 was itself identified in the PGC GWAS study as a high-risk locus and is therefore, not surprisingly enriched in this analysis.
The conclusion from this study emphasises that the increased schizophrenia risk in certain CNVs originates from both the quantity of affected risk genes in the CNV region itself (as shown for 16p11.2 and 22q11.2) and the connection to certain biological pathway processes. The effects of CNVs on pathways related to BDNF signalling, cytoskeleton, and immune system could constitute relevant pathophysiological mechanisms.
Strengths and limitations
The focus of this study is not on single genes but on whole pathways, supporting the emerging pathway/network based medicine and creating a systems biology resource for future investigations of the genetic mechanisms of schizophrenia and other neurodevelopmental disorders.
The limitation of this pathway based study is that pathway creation is dependent on literature input (with implicit bias on availability and selection of sources), requires time and experience to map biomedical knowledge properly to machine-readable identifiers and interactions. We cannot claim completeness of the pathway in reference to the known interaction partners of the genes in the CNV loci investigated. Furthermore, not all CNVs identified in patients have been studied so far to evaluate schizophrenia – or other disease – risks on a larger scale. The molecular pathways are created based on molecular function knowledge on a more or less standardised human model. Individual genetic variations that influence gene expression and protein function could at that stage not be taken into account. Future studies could include personalised genetic data for a more precise risk evaluation based on molecular pathways. However, WikiPathways is a community-created, expert-curated pathway database, and thus, future improvements and updates can easily be incorporated. A limitation of our overlap analysis with DisGeNET is that this database incorporates information from CNV studies, but with the low number of genes in the CNV loci compared to the overall number of schizophrenia risk genes listed in DisGeNET the influence of such circularity on our results would have been minimal.
Conclusion and implications
We identified several potential underlying pathways, most importantly BDNF signalling, that connect pathogenic CNVs on a molecular level and might explain their shared high penetrance for schizophrenia. A key implication of this work relates to the improvement of our understanding of convergent mechanisms of genetic risk for schizophrenia, and further work should determine whether similar mechanisms could also be identified in patients without high-penetrance variants. Another line of impact is the contribution to drug discovery, based on the identification of drug targets in the nodes of the identified pathways. This study also provides potential functional explanation for previous intervention studies on increasing BDNF levels as schizophrenia treatment and enables target prioritisation based on molecular pathway interactions. Further refinement of this work might concern the separation of developmental stages in the pathways, which may enable a focus on the molecular pathways active in the early and adolescent brain development that is highly relevant for the genesis of schizophrenia.
Statement of interest
None to declare.
Supplemental Material
Download (9.8 MB)Supplemental Material
Download (6.5 MB)Supplementary information 1.docx
Download MS Word (12.3 KB)Supplementary Tables.xlsx
Download MS Excel (596.4 KB)Supplementary information 2.docx
Download MS Word (257.4 KB)Acknowledgements
The authors would like to thank Prof. Dr. Han Brunner for helpful discussions around genetics of neurodevelopmental disorders, and Dr. Martina Kutmon and Dr. Lars Eijssen for helpful discussions around permutation testing statistics.
Additional information
Funding
References
- Abbi S, Ueda H, Zheng C, Cooper LA, Zhao J, Christopher R, Guan JL. 2002. Regulation of focal adhesion kinase by a novel protein inhibitor FIP200. Mol Biol Cell. 13(9):3178–3191. doi: 10.1091/mbc.e02-05-0295.
- Blizinsky KD, Diaz-Castro B, Forrest MP, Schürmann B, Bach AP, Martin-de-Saavedra MD, Wang L, Csernansky JG, Duan J, Penzes P. 2016. Reversal of dendritic phenotypes in 16p11.2 microduplication mouse model neurons by pharmacological targeting of a network hub. Proc Natl Acad Sci U S A. 113(30):8520–8525. doi: 10.1073/pnas.1607014113.
- Boldt K, van Reeuwijk J, Lu Q, Koutroumpas K, Nguyen T-MT, Texier Y, van Beersum SEC, Horn N, Willer JR, Mans DA, et al. 2016. An organelle-specific protein landscape identifies novel diseases and molecular mechanisms. Nat Commun. 7(1):11491. doi: 10.1038/ncomms11491.
- Cleynen I, Engchuan W, Hestand MS, Heung T, Holleman AM, Johnston HR, Monfeuga T, McDonald-McGinn DM, Gur RE, Morrow BE, et al. 2020. Genetic contributors to risk of schizophrenia in the presence of a 22q11.2 deletion. Mol Psychiatry. 26(8):4496–4510. doi: 10.1038/s41380-020-0654-3.
- Cox DM, Butler MG. 2015. A clinical case report and literature review of the 3q29 microdeletion syndrome. Clin Dysmorphol. 24(3):89–94. doi: 10.1097/MCD.0000000000000077.
- De Rubeis S, Pasciuto E, Li KW, Fernández E, Di Marino D, Buzzi A, Ostroff LE, Klann E, Zwartkruis FJT, Komiyama NH, et al. 2013. CYFIP1 coordinates mRNA translation and cytoskeleton remodeling to ensure proper dendritic spine formation. Neuron. 79(6):1169–1182. doi: 10.1016/j.neuron.2013.06.039.
- Degenhardt F, Priebe L, Meier S, Lennertz L, Streit F, Witt SH, Hofmann A, Becker T, Mössner R, Maier W, et al. 2013. Duplications in RB1CC1 are associated with schizophrenia; identification in large European sample sets. Transl Psychiatry. 3(11):e326–e326. doi: 10.1038/tp.2013.101.
- Dell’Edera D, Dilucca C, Allegretti A, Simone F, Lupo MG, Liccese C, Davanzo R. 2018. 16p11.2 microdeletion syndrome: a case report. J Med Case Rep. 12(1):90.
- Fisher E, Wood SJ, Elsworthy RJ, Upthegrove R, Aldred S. 2020. Exercise as a protective mechanism against the negative effects of oxidative stress in first-episode psychosis: a biomarker-led study. Transl Psychiatry. 10(1):254. doi: 10.1038/s41398-020-00927-x.
- Golzio C, Willer J, Talkowski ME, Oh EC, Taniguchi Y, Jacquemont S, Reymond A, Sun M, Sawa A, Gusella JF, et al. 2012. KCTD13 is a major driver of mirrored neuroanatomical phenotypes of the 16p11.2 copy number variant. Nature. 485(7398):363–367. doi: 10.1038/nature11091.
- Gustavsen JA, Pai S, Isserlin R, Demchak B, Pico AR. 2019. RCy3: network biology using cytoscape from within R. F1000Res. 8:1774. doi: 10.12688/f1000research.20887.3.
- Kendall KM, Rees E, Escott-Price V, Einon M, Thomas R, Hewitt J, O'Donovan MC, Owen MJ, Walters JTR, Kirov G. 2017. Cognitive performance among carriers of pathogenic copy number variants: analysis of 152,000 UK biobank subjects. Biol Psychiatry. 82(2):103–110. doi: 10.1016/j.biopsych.2016.08.014.
- Kirov G, Rees E, Walters JT, Escott-Price V, Georgieva L, Richards AL, Chambert KD, Davies G, Legge SE, Moran JL, et al. 2014. The penetrance of copy number variations for schizophrenia and developmental delay. Biol Psychiatry. 75(5):378–385. doi: 10.1016/j.biopsych.2013.07.022.
- Kutmon M, Ehrhart F, Willighagen EL, Evelo CT, Coort SL. 2018. CyTargetLinker app update: a flexible solution for network extension in cytoscape. F1000Res. 7:743. doi: 10.12688/f1000research.14613.1.
- Kutmon M, Lotia S, Evelo CT, Pico AR. 2014. WikiPathways app for cytoscape: making biological pathways amenable to network analysis and visualization. F1000Res. 3:152. doi: 10.12688/f1000research.4254.1.
- Kutmon M, van Iersel MP, Bohler A, Kelder T, Nunes N, Pico AR, Evelo CT. 2015. PathVisio 3: an extendable pathway analysis toolbox. PLoS Comput Biol. 11(2):e1004085. doi: 10.1371/journal.pcbi.1004085.
- Le Hellard S, Mühleisen TW, Djurovic S, Fernø J, Ouriaghi Z, Mattheisen M, Vasilescu C, Raeder MB, Hansen T, Strohmaier J, et al. 2010. Polymorphisms in SREBF1 and SREBF2, two antipsychotic-activated transcription factors controlling cellular lipogenesis, are associated with schizophrenia in German and Scandinavian samples. Mol Psychiatry. 15(5):463–472. doi: 10.1038/mp.2008.110.
- Magrinelli F, Balint B, Bhatia KP. 2021. Challenges in clinicogenetic correlations: one gene - many phenotypes. Mov Disord Clin Pract. 8(3):299–310. doi: 10.1002/mdc3.13165.
- Marshall CR, Howrigan DP, Merico D, Thiruvahindrapuram B, Wu W, Greer DS, Antaki D, Shetty A, Holmans PA, Pinto D, et al. 2017. Contribution of copy number variants to schizophrenia from a genome-wide study of 41,321 subjects. Nat Genet. 49(1):27–35. doi: 10.1038/ng.3725.
- Martens M, Ammar A, Riutta A, Waagmeester A, Slenter DN, Hanspers K, R AM, Digles D, Lopes EN, Ehrhart F, et al. 2021. WikiPathways: connecting communities. Nucleic Acids Res. 49(D1):D613–D621. doi: 10.1093/nar/gkaa1024.
- McDonald-McGinn DM, et al. 1993. 22q11.2. In: Adam MP, Ardinger HH, Pagon RA editors. Seattle (WA): GeneReviews((R)).
- Mervis CB, et al. 1993. 7q11.23 duplication syndrome. In: Adam MP, Ardinger HH, Pagon RA editors. Seattle (WA): GeneReviews((R)).
- NetPath MK, Hanspers K, Roudbari Z, Evelo C, Chichester C, Willighagen E, Weitz E. 2021. Brain-derived neurotrophic factor (BDNF) signaling pathway (Homo sapiens). www.wikipathways.org/instance/WP2380. [accessed 2021].
- Nielsen PA, Baruch A, Shestopalov VI, Giepmans BN, Dunia I, Benedetti EL, Kumar NM. 2003. Lens connexins alpha3Cx46 and alpha8Cx50 interact with zonula occludens protein-1 (ZO-1). Mol Biol Cell. 14(6):2470–2481. doi: 10.1091/mbc.e02-10-0637.
- Pardiñas AF, Holmans P, Pocklington AJ, Escott-Price V, Ripke S, Carrera N, Legge SE, Bishop S, Cameron D, Hamshere ML, et al. 2018. Common schizophrenia alleles are enriched in mutation-intolerant genes and in regions under strong background selection. Nat Genet. 50(3):381–389. doi: 10.1038/s41588-018-0059-2.
- Piñero J, Bravo À, Queralt-Rosinach N, Gutiérrez-Sacristán A, Deu-Pons J, Centeno E, García-García J, Sanz F, Furlong LI. 2017. DisGeNET: a comprehensive platform integrating information on human disease-associated genes and variants. Nucleic Acids Res. 45(D1):D833–D839. doi: 10.1093/nar/gkw943.
- Prata DP, Costa-Neves B, Cosme G, Vassos E. 2019. Unravelling the genetic basis of schizophrenia and bipolar disorder with GWAS: a systematic review. J Psychiatr Res. 114:178–207. doi: 10.1016/j.jpsychires.2019.04.007.
- Schizophrenia Working Group of the Psychiatric Genomics C. 2014. Biological insights from 108 schizophrenia-associated genetic loci. Nature. 511(7510):421–427.
- Shannon P, Markiel A, Ozier O, Baliga NS, Wang JT, Ramage D, Amin N, Schwikowski B, Ideker T. 2003. Cytoscape: a software environment for integrated models of biomolecular interaction networks. Genome Res. 13(11):2498–2504. doi: 10.1101/gr.1239303.
- Silva AI, Haddon JE, Ahmed Syed Y, Trent S, Lin T-CE, Patel Y, Carter J, Haan N, Honey RC, Humby T, et al. 2019. Cyfip1 haploinsufficient rats show white matter changes, myelin thinning, abnormal oligodendrocytes and behavioural inflexibility. Nat Commun. 10(1):3455. doi: 10.1038/s41467-019-11119-7.
- Tripathi A, Nasrallah HA, Pillai A. 2023. Pimavanserin treatment increases plasma brain-derived neurotrophic factor levels in rats. Front Neurosci. 17:1237726. doi: 10.3389/fnins.2023.1237726.
- Trubetskoy V, Pardiñas AF, Qi T, Panagiotaropoulou G, Awasthi S, Bigdeli TB, Bryois J, Chen C-Y, Dennison CA, Hall LS, et al. 2022. Mapping genomic loci implicates genes and synaptic biology in schizophrenia. Nature. 604(7906):502–508. doi: 10.1038/s41586-022-04434-5.
- van Bon BWM, et al. 1993. 15q13.3 microdeletion. In: Adam MP, Ardinger HH, Pagon RA editors. Seattle (WA): GeneReviews((R)).
- van Iersel MP, Pico AR, Kelder T, Gao J, Ho I, Hanspers K, Conklin BR, Evelo CT. 2010. The BridgeDb framework: standardized access to gene, protein and metabolite identifier mapping services. BMC Bioinf. 11(1):5. doi: 10.1186/1471-2105-11-5.
- Walsh T, McClellan JM, McCarthy SE, Addington AM, Pierce SB, Cooper GM, Nord AS, Kusenda M, Malhotra D, Bhandari A, et al. 2008. Rare structural variants disrupt multiple genes in neurodevelopmental pathways in schizophrenia. Science. 320(5875):539–543. doi: 10.1126/science.1155174.
- Yates A, Akanni W, Amode MR, Barrell D, Billis K, Carvalho-Silva D, Cummins C, Clapham P, Fitzgerald S, Gil L, et al. 2016. Ensembl 2016. Nucleic Acids Res. 44(D1):D710–716. doi: 10.1093/nar/gkv1157.
- Zhuo C, Hou W, Li G, Mao F, Li S, Lin X, Jiang D, Xu Y, Tian H, Wang W, et al. 2019. The genomics of schizophrenia: shortcomings and solutions. Prog Neuropsychopharmacol Biol Psychiatry. 93:71–76. doi: 10.1016/j.pnpbp.2019.03.009.