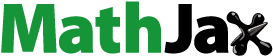
Abstract
Precipitation has a dominant role in agriculture, and a regular rain pattern is usually vital to agriculture; excessive or inadequate rainfall can be harmful. In this paper, an agricultural drought index is utilized to study the agricultural drought periods and analyze them with their intensities at various locations. Some nonhomogeneous Poisson models are also used to calculate the probability of agricultural droughts (number of times occurred) in a time interval of interest. It is to be assumed that the number of agricultural drought event occurrences is a Nonhomogeneous Poisson Process (NHPP) has a rate function, which depends on some parameters that must be estimated. Two cases of these functions are considered: the Weibull and linear intensity function. The Bayesian approach with Gibbs sampling under the Markov Chain Monte Carlo (MCMC) algorithm is used to estimate the parameters of these functions. The most appropriate fitted model is selected by using Deviance Information Criteria (DIC) and use that appropriately fitted model to calculate the accumulated events of agricultural drought in a time interval of interest at each location. Ordinary Kriging (OK) is used to regionalize the parameters and present its spatial behavior. The results based on the DIC indicate that the Power Law Process (PLP) performs better than the linear intensity function, NHPP model. The interpolated parameter values for the appropriate model, their patterns, and fluctuations for the study area are efficiently presented using contour maps. It is a novel and straightforward approach to assess the selected model parameter values used to predict the accumulated drought events at un-sampled locations. The proposed framework might also help to analyze other spatial variables of interest and can be used for climate-change study, ecosystem modeling, etc. The findings can also help to make decisions for sustainable environmental management in Pakistan.
1. Introduction
In recent years, it has been observed that the climate changes on the planet, causing the irregularity in the behavior of rainfall or precipitation. Often with little rain in some areas and excessive rain in other regions, which adversely affects agriculture, forests, water reservoirs, and biodiversity. Over the past several decades, extensive and frequent drought events had disastrous effects on the environment, economy, and society (Mishra and Singh, Citation2010). In Pakistan, this abnormal behavior has been accounted for; some parts of Pakistan face water supply shrinkage. Therefore, rarely observed a shortage of water in water reservoirs that supply water to the agricultural areas.
Low precipitation and unpredictable weather patterns are the leading causes of drought in an area. According to Bayissa et al. (Citation2015), drought is a natural hazard indicated by a remarkable reduction in water availability during an extended period over a large area. Drought frequently occurs because it is a part of the climate, but timely action (i.e., before, during, and after it) could reduce the risk. Precipitation plays a vital role in managing water resources because it is the primary parameter to identify some natural hazards, like drought and floods (Zambrano et al., Citation2017). In each discipline, drought has its meaning and classifications, which leads to inventing some particular and detailed methodologies or frameworks to analyze the drought events. Some of these methodologies are the Precipitation Data Analysis (PDA) method, Water Balance (WB) method, Stream Flow Analysis (SFA) methods, and methods of using geographical and historical data. The frequently used drought analysis methods are based on the drought indices (Ellahi et al., Citation2020). Several drought indices have been recommended and proposed by various researchers to analyze drought events. A comprehensive discussion on few drought indices is given in (Mishra and Singh, Citation2010).
In drought monitoring and analysis, is an essential tool developed by (McKee et al., Citation1993).
Zhao et al. (Citation2018) utilized for the Spatio-temporal evaluation of drought in Loess Plateau. Nasrollahi et al. (Citation2018) used SPI-3 and SPI-12 at all stations to study the Spatio-temporal pattern of drought hazards in Semnan province (Iran) and constructed the Drought Hazard Index map. SPI-3 was also used by Gidey et al. (Citation2018) to assess the meteorological drought duration, onset, offset, magnitude, severity, frequency, and spatial extent in Raya, including its environs (Northern Ethiopia), and evaluate seasonal rainfall deficit. Mishra and Singh (Citation2010) also computed SPI-3 to analyze and discuss the agricultural droughts. The 3 and 12 months SPI was also calculated and utilized by Merabti et al. (Citation2018) to analyze the agricultural and hydrological drought.
According to the McKee et al. (Citation1993) classification system, the value of SPI less than or equal to −1.0 indicates the occurrence of drought events and ends when the value becomes positive. So, this limit (of SPI less than or equal to −1.0) was considered a threshold by Achcar et al. (Citation2016) to enumerate and analyze the drought periods using the NHPP model. NHPP models are commonly used to analyze the exceedances events like noise pollutions (Guarnaccia et al., Citation2015), the earthquake ground-motion intensities (Iervolino et al., Citation2014), and the air quality standards (Achcar et al., Citation2016; Achcar et al., Citation2008; Rodrigues et al., Citation2015). It provides us a way towards the point pattern of exceedance events (Cressie, Citation1992; Diggle, Citation2013). The major problem in the NHPP models is to determine and estimate a suitable intensity function for appropriately representing the expected number of failures experienced up to a particular time. The NHPP has various functional forms of intensity functions under different assumptions. So, to obtain the results from NHPP models, the existing parameters of the models should be determined. For the NHPP models used for the count data analysis, the Bayesian approach is considered with various prior distributions to estimate the model parameters, commonly illustrated in previous literature (Guarnaccia et al., Citation2015; Leininger and Gelfand, Citation2017). The researcher commonly used the Bayesian approach for the estimation of parameters in various applications of different areas, i.e., in the analysis of air pollution data, in the software reliability analysis, in the drought analysis, and air quality standards (Bar-Lev et al., Citation1992; Kuo and Yang, Citation1996; Cid and Achcar, Citation1999; Achcar et al., Citation2008, Citation2011, Citation2016; Rodrigues et al., Citation2015) .
Geostatistical techniques are often used to illustrate spatial patterns of the random field (Entin et al., Citation2000; Mohanty et al., Citation2000). In geostatistics, a key concept is a variogram, which is used to calculate the local variability of the random field and interpolate the input parameters used for spatial interpolation through kriging (Webster and Oliver, Citation2007; Chen and Guo, Citation2017). In the modeling of variogram, the fundamental structural parameters are the sill, range, and nugget. In addition, the variogram models might contain simple models, i.e., Linear, Nugget, Spherical, Exponential, Power, and Gaussian, or the sum of one or more variogram models (Pebesma, Citation2004).
Here, we provide an efficient, effective, and novel framework for analyzing the drought periods to evaluate the aggregated number of drought events at unsampled locations. In the previous literature for the drought events or period analysis like Ellahi et al. (Citation2020) utilize only the NHPP based on a single intensity function only. But the comparative study of models improves the results significantly in applied statistics. Therefore, our study is based on a comparative assessment of two NHPP models to select the appropriate model for the drought events analysis. Further, it also provides a novel way for the assessment of appropriate model parameters at unsampled locations for the analysis of accumulated drought events at that locations. The current study will support agricultural and irrigation authorities to improve the drought mitigation policies and planning. That will enhance the development of adaptation strategies for long-term drought based on the current limited resources.
The outline of our proposed study is as follows: Section 2 introduced data structure and explanations of methods utilized in our framework. Section 3 presents the descriptive structure of the agricultural drought index at observed locations, the comparison of two different models, and the presentation of the estimated accumulated number of drought events based on the most appropriately selected model. It also consists of the spatial interpolation results for the parameters of the selected model. Section 4 discussed these results, and at the end, conclusions and some future directions are discussed in Section 5.
2. Agricultural drought index formulation
SPI was originally proposed by McKee et al. (Citation1993) and was further modified by Edwards et al. (Citation1997). This index provides detailed information about the relative precipitation variation from normality. The 3-months is commonly used to analyze agricultural droughts; therefore, the current study is mainly concerned with analyzing the
month time scale
The computation of SPI-3 is a multi-step procedure.
For the calculation of SPI-3 used in the analysis of agricultural drought, in the initial step, summed the monthly precipitation data from month i-2 to month i. The summation value is then allocated to month i (where i = 1,2,3, …. the number of months). Therefore, the values for the first two months will be missed in the case of SPI-3.
The second step involves fitting appropriate distributions to the long-term aggregated precipitation for every considered station of our study region. The R-GUI software is used to determine the appropriately fitted distributions based on multiple goodnesses of fit criteria. The parameters of selected distributions are then estimated by using best-unbiased estimations techniques.
The cumulative probabilities for aggregated monthly average precipitation are then calculated in the next step, using the appropriately fitted distributions and their resulting parameters. The selected distributions with their parameters and estimation methods are given in .
Table 1. Fitted distributions and methods of parameters estimations.
For SPI calculation, the standardization is done using the method proposed by Abramowitz and Stegun (Citation1965), detailed described in Zaho et al. (Citation2018).
The negative values of SPI-3 indicates the occurrence of drought for that time and region. On the other hand, the positive value indicates the wetness dominance for that time and region. Normal, moderate, severe, and extreme, respectively, rank the severity of wetness and drought events. This rank classification is dependent on the negative and positive values of SPI-3, which are presented in .
Table 2. Classification of droughts based on SPI.
2.1. Mathematical description of NHPP models
This section describes the NHPP models mathematically with their intensity and mean value functions. The data of precipitation used in the calculation of SPI-3 are collected from N meteorological stations. These are the areas where we should assess the probability that the severe events of drought occur (i.e., ) a particular number of times, in a specific time interval, along with the total average number of drought events. For the time interval
>
let
denotes the occurrence of the total number of drought events at the location
of interest. Based on Ki, the set of observed months of drought are denoted by
=
The value of Ti depends on the ith station; on the other hand, in our case, we consider the same value of Ti, i.e., for every
The values of
and the sets
were calculated easily from the considered stations' information where the measurements are taken. So, in our present research, we collected the set of observed data at sampled locations, denoted by
It is to be assumed that for the given time interval
the number of occurrences of drought events follows NHPP with an intensity (rate) and mean value function
>
and
The cumulative drought event occurrences for a particular time interval
at a location
are denoted by
Let,
is a NHPP with
and
as intensity and mean value functions, respectively. So, the Poisson model becomes
(2)
(2)
where
and
>
For the prediction and interpretations of the behavior of
the behavior of the
should be understood. So our problem reduced to studying
to understand and analyze the behavior of
that can be assumed in several ways. Here, we used the comparative study of two well-known cases of NHPP for the analysis of agricultural drought periods at 52 locations of Pakistan. These two special cases of NHPP models are usually applied in operating safety policy and reliability. These are the linear intensity function and the power-law process, also known as the Weibull process. The mean value functions are,
(3)
(3)
(4)
(4)
respectively, where,
=(
), for
is the vector of parameters that rule the behavior of the mean function. the intensity functions related to Equationequations (3)
(3)
(3) and Equation(4)
(4)
(4) are given by
(5)
(5)
(6)
(6)
In both models, the parameters vary or depend on each station individually, but the rate functions themselves remain constant. The =(
),
represents the rate functions parameters that are related to N meteorological stations.
In the current study, we also want to investigate the number of drought events and their behavior in Pakistan for the fixed time interval of interest. Therefore, in the temporal framework for studying the behavior of drought in Pakistan, the rate functions are considered here for a suitable comparative study. So, are the vectors of Poisson rate function parameters of each ith sampled location. The statisticians and mathematicians have introduced several parametric and nonparametric estimation methods to estimate the parameters. Still, here we use the Bayesian point of view for the estimation of rate function parameters. For
the location,
=(
) is an unknown vector of parameters (in both cases of rate functions), estimated under some uncertainties, described by assigning the prior distributions in the Bayesian approach. So our problem is further reduced to estimate
=(
), for every
These estimated parameters represent the most appropriate behavior to present each station's drought measurements.
2.2. Bayesian formulation
This section presents the general mathematical framework of the Bayesian approach to analyze the drought periods. The joint posterior distribution of the vector is the distribution of interest for each station. This joint probability distribution is obtained by utilizing the likelihood function of the NHPP model and the prior distribution of
(i.e., the prior joint distribution of the parameters
and
). The posterior distribution is
Where is the likelihood function and
is the prior distribution of
So, according to our hypothesis, the NHPP models are as follow;
(7)
(7)
here, we used
and
to make it unequivocal the reliance of rate and mean function on the corresponding parameters
(Cox and Lewis, 1966).
The MCMC methods are used to generate the posterior summaries of interest. In the Bayesian approach for the above-discussed models, it is considered that the priors for parameters and
are uniform distributions, i.e.,
and
The hyperparameter
values for prior distributions can be considered by the personal judgment of the analyst. Those values of hyperpriors should be considered for which the prior distributions have minimum variance. Here we assume the prior independence between the parameters. The simulated samples of the joint posterior distribution
are obtained by using the MCMC methods. For this purpose, we used the library "R2jags" under R software originally introduced by (Su and Yajima, Citation2012).
In this work, the prior distributions of hyperparameters are supposed to be known that will be described later. The most appropriate model is selected using the existing Bayesian adequacy measures called DIC; the smaller the DIC, the better the models will be. The performance of the model is observed by comparing the graphs of estimated and actual mean values of the cumulated number of drought events versus the month of occurrence. After selecting the most appropriate model, each station's estimated parameters are regionalized to observe the spatial behavior of the parameters. For this purpose, we used Ordinary Kriging (OK) (see, Malvic, 2008, which is considered as the Best Linear Unbiased Predictor (BLUP).
3. Estimation results
Here, the results of the above-discussed models are presented in this section. The methodology provided in the previous sections is implemented on the average monthly precipitation data of 52 observed locations of Pakistan. Since the objective of our paper is to estimate and investigate agricultural drought events therefore precipitation data is used to calculate month
values at each sampled location. presents the time series plots of SPI-3 from 1968 to 2016.
Fig. 1. The 3-Month standardized precipitation index (SPI −3) versus time (date) (a) Kotli, (b) Dir, (c) Kalat and (d) Lasbella.

3.1. Agricultural drought
The atypical behavior of SPI-3 (i.e., values of SPI-3 is less than or equal to −2.0) in indicates the severely dry periods for Dir and Kotli. In Dir, the longest seasonal or agricultural drought period was from August 1969 to July 1971. That was 23 months drought period with −2.3, the peak value. Other severe droughts of 1, 2, 3, and 4 months with peak values ranging from to
are also observed in Dir. shows the values of SPI-3 of Kotli with temporal variations. It indicates 2, 3, 5, and 7 months of severe agricultural drought periods with different peak values ranging from −2.04 to −3.56. indicates that no single month of severe drought exists from 1968 to 2016 for Kalat and Lasbella.
The cumulative number of drought events in each time versus month of occurrence is presented in for the SPI-3 series() of Kotli, Dir, Kalat, and Lasbella. The moderate drought periods, other than the severe drought periods, at each station can also be identified from with various duration and peak values. The moderate and severe drought events classification is provided in . The summaries of these drought periods are given in .
Fig. 2. Accumulated numbers of SPI − 3 ≤ −1.0 in each time versus month of © (a) Kotli, (b) Dir, (c) Kalat and (d) Lasbella.

Table 3. Drought periods of each station for 1968-2016 by SPI −3(≤ −1.0).
We could consider the Stochastic Volatility(SV) models (see, Ghysels et al., Citation1996; Meyer and Yu, Citation2000) under the Bayesian hierarchical approach to model time series SPI-3 data. The drought events can occur any time the SPI-3 continuously negative and can reach an intensity of −1.0 or less. In the present paper, we considered the time(months) of droughts identified by the threshold for all stations from January 1968 to December 2016.
The models discussed in the previous sections (2.2 and 2.3) are applied to the cumulative number of drought events concerning months at each station. For this model approximation, the Bayesian framework is used to estimate parameters for studied models at each station for our study region. We know, according to the classification of SPI values, the drought event occurs if Therefore we use the threshold
in our study. Many researchers in their research studies recently considered similar threshold values (e.g., see, Achcar et al., Citation2016). The aggregated drought events at each station for the period 1968–2016 are given in . For those stations, these aggregated values correspond to the values of
respectively. The study period corresponds to a total number of months,
for (
In the case of the power-law process, the uniform non-informative priors are assumed for
and
i.e.,
and
The uniform non-informative priors are also assumed for
and
i.e.,
and
when the linear intensity model is considered. We used those hyperparameter values for which the prior distributions have minimum variance. There is not any hard and fast rule to define hyperparameter's values; these values can be considered based on personal judgment. For the joint posterior distribution of interest, we produced 31,000 Gibbs samples from which the first 1000 were discarded to eliminate or reduce the effect of initial values used in the iteration process in the simulation sample.
Table 4. List of the total number of agricultural droughts at each station (1968-2016).
3.2. Model comparison results
It might be at some individual stations that the linear intensity function performs better than the power-law process, but, collectively the power law process model performs better than the linear intensity function. This is confirmed by Monte Carlo estimates for the DIC of each model. The DIC values for the power-law process and linear intensity function models are given in . As the DIC value of the linear intensity function is greater than the value of the power-law process so, in our case, the power-law process is better than the linear intensity function. Therefore, the estimated parameters of the power-law process are considered to estimate the accumulated number of agricultural droughts at each observed location and time point. The selected model parameters are also estimated at unobserved locations to observe their spatial behavior by using spatial interpolation tools. presents the observed accumulated number of drought events and the estimated mean values of accumulated droughts versus four representative stations using the power law process model.
Fig. 3. Plots of Accumulated numbers of SPI −3 ≤ −1.0 in each time with the estimated mean value function versus month of ©. (a) Kotli, (b) Jiwani, (c) Mianwali, and (d) Barkhan.

Table 5. Values of DIC for power-law process and linear intensity function models.
3.3. Estimation results
The estimates of the parameters and
for each location and their 95% credible intervals (the posterior summaries) provide some useful information about the behavior of each parameter and its effect on the power-law model. It has been observed that for the power-law process, the intensity function
has flexible behavior due to the value of
For
the intensity function is increasing, and for
the intensity function is decreasing and constant for the value of
=1, i.e., the NHPP transformed to HPP. In our study area, It has been observed that
have similar behavior for the stations of the same geographic region. Moreover, it is to be noted that the stations in the different regions may also have similar behavior. The station of Jewani
has a maximum value that is 1.704
with a standard deviation of 0.01163, and the 95% credible interval is [1.68, 1.726]. On the other hand, Kalat has a minimum value of
which is 0.5853
have respective standard deviation is 0.004609 and the 95% credible interval is [0.5792, 0.597]. It is observed that the larger the value of the parameter
the larger will be the standard deviation, and the wider will be the credible interval. Likewise, the smaller value of
has a smaller standard deviation and a little credible interval. It is more important to be noted that the posterior mean of the parameter
for any station implies that the rate at which the drought event occurs is decreasing with respect to time. Therefore, the drought occurrence at the threshold less than or equal to −1.0 is becoming less frequent.
For some stations, the values of the parameter may vary significantly even though the stations or locations exist in the same geographical region. The rate function of some stations has similar behavior, which has a similar value of
Jewani station also has a maximum value for parameter
with 0.4641 standard deviations and a 95% credible interval is
However, Mianwali and Bahawalpur both have a minimum (and same) value of
equals to 1.004 and have the same 95% credible interval
with their respective standard deviations 0.003669 and 0.003884. The
values indicate that if the drought occurrence is increasing or decreasing, they increase or decrease in different ways. If larger (smaller) the value of
smaller(larger) will be the value of
for the fixed value of
and
So, the considered appropriate model captured the different behavior of the drought occurrence rate at different locations.
The estimated and observed accumulated means of drought occurrence for four stations are presented in . Because for all the stations, these graphs are categorized in three types, i.e., good, medium, and worse estimated, based on estimated accumulated means of drought events. Those estimated means are calculated at each point when the drought events occurred for each station. The categories of those fitted graphs are decided based on their graphical representation and RMSEs. To display all these three categories of stations for our fitted models, we selected four stations, i.e., Kotli, Dir, Lasbella, and Kalat. The observed and estimated accumulated means of these selected stations are presented in . Here, the accumulated mean stand for the mean value function either observed or estimated, calculated at each time where the drought event occurred.
The accumulated mean of drought events at Kotli shown in is a good fit to estimate the observed mean with RMSE 2.13576. shows the poor fit of the estimated accumulated mean to the empirical average for Dir, with RMSE 6.56612. The accumulated means of drought events for Kalat and Lasbella are shown in , which is the average fit of estimated to the observed means with RMSEs 3.470529 and 3.450207. Like Kalat, It may be observed that a model taking into consideration can capture the changepoint, or any alternate rate function could be considered. Similarly, for stations Dir and Lasbella, maybe a model either with an alternate rate function or with the presence of more than one changepoints could give a superior fit. presented the RMSEs at each study region.
Table 6. RMSEs of each station for observed vs estimated accumulated drought events.
3.4. Regionalization and interpretation results
In this section, we implemented the above-discussed models for the regionalization or spatial distribution of power-law process model parameters ( and
) by using the estimated posterior mean values of these parameters for 52 locations in Pakistan. These model parameters were used to fit the accumulated number of drought events evaluated by using
In the next sub-sections, we deal with the parameters
and
results individually.
3.4.1. For parameter 

In the case of parameter "λ" for normalization, the Box-Cox transformation technique with an estimated transformation parameter is considered for our data. The variogram model can be calculated with either modulus or classical procedure. We calculated spatial variogram of
by the method of the modulus. shows the graphical assessment of the empirical variogram for ordinary kriging calculated for the
parameter. Before applying the spatial interpolation technique, it should be noted that the spatial correlation must exist in the data. Suppose the spatial correlation does not exist in sample point data. In that case, we cannot use any of the spatial interpolation techniques and need to search for another interpolation method for this data.
Various techniques have been introduced in the literature to investigate the spatial correlation of sampled point data. Here we used the variogram envelope technique to check the spatial correlation in the data. represents the graphical representation of the variogram envelope. Dashed lines are the 95% pointwise variogram envelope, based on the and
percentile of semi-variance estimates. The graph clearly shows that most of the variogram points lie in the envelope and four points lying on the envelope lines; to some extent, these points are also considered in the variogram envelope range. So, it indicates that there is a strong spatial correlation in the sampled points.
For spatial interpolation and structural analysis, variogram modeling and estimation methods used to estimate variogram model parameters are essential (Burrough and McDonnell, 1998). So, for this purpose, various estimation techniques are available in the literature. In this study, the Ordinary Least Square (OLS) estimation, Maximum Likelihood (ML) estimation, Weighted Least Squares (WLS) estimation, and Restricted Maximum Likelihood (REML) estimation are utilized for fitting the variogram model. Various analytical functions (models) are used to fit the variogram model to the experimental variogram, parameters estimation techniques (ML, REML, OLS, and WLS), and their Root Mean Squared Errors (RMSE's) are given in . The main aim of using various analytical functions (models) and parameter estimation methods is to find a suitable model and appropriate estimate of parameters for that model.
The model estimated results were validated by using the leave-one-out cross-validation technique. In , the minimum RMSE= 0.19201 is for the wave model, and the estimated method used is REML. The plot of an appropriate fitted model is shown in . So, we used this model and parameter estimation technique for fitting the empirical variogram and used that fitted model in OK for spatial interpolation of parameter values.
Table 7. Parameters and RMSE's of variogram models in case of
Finally, efforts are made to predict the parameter at un-sampled locations by using the above appropriate selected variogram model and estimation technique in OK that provides the map of Pakistan for the spatial interpolation results with their associated prediction errors. These maps are shown in . The contour map of spatial prediction of parameter
posterior mean values is presented in , and shows the spatial interpolation errors map for the parameter
These maps only show an understanding of the behavior or, to some extent, the confidence intervals of predicted values and their prediction errors in our study area. Using the primary and prominent colors in the construction of contour maps, we can figure out the estimated results for specific points or locations because color fluctuates at every degree. From , the contour intervals for the estimated or predicted
with their prediction or estimation errors are figured out with color keys. Contour intervals for predicting
and prediction errors are approximately [0.7, 1.4] and [0.11, 0.37] i.e., the interval lengths are 0.7 and 0.26. The predicted mean values of the parameter
and their standard deviations have minimum values in the area under latitude [26.5, 28.5] and longitude [69.5, 71.8].
Similarly, The predicted mean values of the parameter and their standard deviations have their maximum values in the area under latitude [25, 26.2] and longitude [61.8, 62.8]. In both cases, i.e., predicted
parameters and their standard error contour maps, the green color represents the minimum values, and the red color shows the maximum values. The prominent green, blue, yellow, and red-colored areas have
values 0.7, 0.95, 1.15, and 1.35 with standard deviations of 0.12, 0.20, 0.28, and 0.36, approximately.
3.4.2. Regionalization and interpretation of parameter 

Before applying the spatial interpolation technique to examine the spatial correlation of sampled point data, several techniques have been introduced in the literature. The spatial correlation is checked by using the variogram envelope technique. provides the graphical presentation of the variogram envelope. The dashed lines are 95% pointwise variogram envelope, based on the and
percentile of semi-variance estimates. From the figure, it has been observed that a maximum number of variogram points lying in the envelope and two points lying on the envelope lines; to some extent, these points are also considered in the range of variogram envelope. So, it is an indication of the existence of a strong spatial correlation in sampled points. The distribution of the parameter
was non-normal, so we transformed the data parameter
to make its behavior normal. For normalization, the Box-Cox transformation technique with an estimated transformation parameter
is considered in our data.
Here, the modulus method is used for the calculation of empirical variogram for " ". shows the visual assessment of the empirical variogram calculated from the
parameter values. After plotting the experimental variogram of the data, different variogram models were fitted to the experimental variogram as the experimental variogram model does not give us better structural analysis and spatial interpolation of the study variable at un-sampled locations. In this study, the parameters of variogram models were estimated by using ML, REML, OLS, and WLS. The fitted variogram model having the smallest RMSE was used to interpolate the parameter
at unobserved locations. Different analytical function models fitted to the experimental variogram, their estimated parameters, parameter estimation techniques, and their Root Mean Squared Errors (RMSE's) are given in . The main aim of using various analytical functions (models) and parameter estimation methods is to find the appropriate variogram model and the appropriate estimate of parameters for that model.
Table 8. Parameters and RMSE's of variogram models in case of
The leave-one-out cross-validation technique was used to validate the estimated model results. The criteria for selecting an optimal model is set by RMSE, i.e., the smaller the RMSE better will be the interpolated results. In , the minimum is for the Matern model, and the most appropriate estimation method used is OLS. shows the plot of the suitable fitted variogram model. So, we used this model and param eter estimation technique for fitting the experimental variogram and used that fitted model in OK for spatial interpolation of
parameter values at unobserved locations.
Finally, the above appropriate selected variogram model and estimation technique were used in OK to predict the parameter at unobserved locations for the distribution maps of the spatial interpolation results and associated prediction error. shows the spatial distribution of the parameter
and their prediction errors, i.e., standard deviations. The contour map of spatial prediction of parameter
posterior mean values is presented in , and shows the spatial interpolation errors map of the parameter
Prominent colors, i.e., green, blue, yellow, and red, were used to construct contour maps, which helps to figure out the estimated results for specific points or locations because color fluctuates at every degree.
Contour intervals of predicted and prediction errors are approximately [0, 145] and [0, 90], i.e., the interval lengths are 145 and 90. The predicted mean values of the parameter
and their standard deviations have minimum values for the maximum study area, i.e., the area under latitude [27, 31] and longitude [68, 74] with their surroundings. Similarly, The predicted mean values of the parameter
and their standard deviations have their maximum values in the area under latitude [25, 30] and longitude [61.5, 62.5]. In both cases, i.e., predicted
parameters and their standard error contour maps, the green color represents the minimum values, and the red color shows the maximum values. The prominent green, blue, yellow, and red-colored areas have
values 5, 50, 95, and 140 with standard deviations 0, 30, 60, and 90, approximately.
4. Discussion
Drought is a natural disaster, and it differs from other natural disasters like floods, earthquakes, and tornadoes, etc. Almost every region of Pakistan has a history of facing severe drought, which substantially damaged the industrial, agricultural, and economic sectors (Hussain and Mumtaz, 2014). The paper provides an assessment of agricultural drought, as categorized by the The advantage in consideration of SPI for drought monitoring is that it can easily estimate the precipitation deficiency at multiple time scales. These time scales measure the consequences of drought events on different water resources. Therefore, in various countries around the world, SPI is now used in multiple operational monitoring systems. It is also recognized by the World Health Organization (WHO). Therefore, this research study may provide a better understanding for policymakers, which helps construct effective drought mitigation and adaptation policies.
In our study, the time series plots of (see, ) presents the descriptive structure of agricultural drought indices. provides the complete and detailed list of start and end dates of agricultural drought periods with their durations (magnitudes), peak and average intensities of agricultural droughts that occurred at Kotli, Dir, Lasbella, and Kalat. Whereas has the list of aggregated agricultural drought events at each region or station under study from 1968 to 2016. The results of these tables indicate that every location or region of our study area has a history of severe agricultural drought events. Achcar et al. (Citation2016) used the NHPP for the drought periods analysis at a single station by using the power law process. But here in our study, we compare the two different NHPP approaches, i.e., the Power-law process and the Linear intensity function, and select the appropriate model to analyze the agricultural drought events at 52 meteorological stations. For this purpose, The appropriate model is selected using DIC, which indicates that power-law process performs better than the linear intensity function (as the DIC value of the power-law process model is less than the linear intensity function model) to evaluate the cumulative drought events in the study area(see, ). The parameters were estimated using the joint posterior distribution of
under MCMC simulation. The means of marginal posterior distributions for each parameter of the power-law process at each station are used to estimate and predict the agricultural drought events at that station. Here, we presented and discussed the observed and estimated four stations' drought events at each time point by the power-law process model. The RMSEs and graphical presentation in provide a clear image of the best, average, and worse estimated results of the accumulated number of drought events models. presented the RMSEs of the fitted model for all 52 regions. The presented results showed that approximately for maximum stations, the model performed significantly well. The worse performance of the model is due to the abrupt changes in the behavior of drought occurrence events at some locations. At some regions where the performance of the model was not as satisfactory is due to the abrupt changes in drought occurrences could be improved, which may be considered in our future project. The novelty in this research work is the use of the geostatistical interpolation method to interpolate the appropriate model (i.e., power-law process) parameters for the study area. The estimation of variogram models, including their parameters, is essential for spatial prediction and structural analysis. Different variogram models were estimated and fitted to the experimental variogram by using ML, REML, OLS, and WLS. The variogram model estimated results were validated by using the Leave-one-out cross-validation technique. The RMSE is used as a measure of selecting the optimal model. The wave model with the REML estimation method has minimum RMSE (i.e., 0.19201, see and ).
On the other hand, in the case of the parameter the Matern model with the OLS estimation method has minimum RMSE (i.e., 8.639952, see and ). These estimated variogram models are then used in OK to predict the parameters
and
at un-sampled locations that provide the detailed structures or spatial variation mirrors (contour maps) of these parameters by generating an accurate and reliable spatial distribution. From these maps, we can observe a clear view of the shape and scale of the selected model over the study area (see and ). The rate of change of parameter
which is the shape parameter of selected models, is very high in the Western Balochistan region. On the other hand, the Eastern Balochistan, the Western highlands, the sub-Montane, the greater Himalayas, and the lower Indus areas have lower rates of variation of the shape parameter
whereas the remaining study area, which is the central and Southern Punjab, has a nominal rate of spatial variation with minimum values of the parameter
The contour maps of their standard deviation also present approximately the same spatial behavior.
Similarly, the rate of variation of parameter which is the scale parameter of models, has the highest rate of variation in the area of the Western Balochistan plateau. In other regions, the variation rate of scale parameters is very low, which is negligible and has minimum values of the parameter
The contour maps of their prediction errors also have the same spatial behavior.
5. Conclusion
Natural disasters cannot be stopped; every disaster provides information to ensure well-planned management and useful information for preventive measures. This paper mainly analyzed the agricultural drought periods and regionalized the appropriately selected model parameters. It delivers a better research framework or structure in understanding the agricultural drought with various intensities at multiple locations. It also provides a framework for the analysis and estimation of cumulative agricultural drought events at each location by an appropriately selected model and regionalizes the selected model parameters for the study area. For this purpose, our research work is divided into three essential phases; each phase has its concept. In the first phase, the concept of month time scale
is used to calculate and exploratory analyses the agricultural droughts with their intensities at each location. That performs well in presenting and estimating the agricultural drought events with their intensities. At the second phase, the concept of point processes is utilized, i.e., NHPP with linear intensity function and Weibull process. The most appropriate model, i.e., the Weibull process model, is selected using DIC to analyze and estimate the accumulated drought events at each time point for each station, which is obtained by introducing a threshold in drought intensities. In the end, the geostatistical interpolation technique (OK) is used to regionalize the appropriate model parameters which are very useful to estimate the parameters at unsampled locations. The spatial variations of the estimated parameters and their respective errors are also presented in contour maps.
We have identified the fruitful directions for the associated future research built on the work developed in this paper. There are no criteria for comparing the drought indices because every drought index is based on a different conceptual framework. However, more climatic variables in calculating the drought index can increase the drought index accuracy to perform better in drought prediction and monitoring. A class of geostatistical models can be used to determine the comparative study to analyze the spatial behavior of model parameters, which will improve the efficiency and accuracy of the proposed research.
Competing interests
The authors declare there are no competing interests.
Consent to participate
All authors are voluntarily agreed to participate in this research study.
Ethical statement
All procedures followed were in accordance with the ethical standards with the Helsinki Declaration of 1975, as revised in 2000.
Consent to publish
All authors are agreed for publication; there is no legal constraint in publishing the data used in the manuscript.
Availability of data and materials
The data used for the preparation of the manuscript is available with the corresponding author and can be provided upon request.
Disclosure statement
No potential conflict of interest was reported by the authors.
Additional information
Funding
References
- Abramowitz, M. and Stegun, I. A. 1965. Handbook of mathematical functions: with formulas, graphs, and mathematical tables. Courier Corporation.
- Achcar, J. A., Coelho-Barros, E. A. and de Souza, R. M. 2016. Use of nonhomogeneous Poisson process (NHPP) in presence of change-points to analyze drought periods: a case study in Brazil. Environ. Ecol. Stat. 23, 405–419. doi:https://doi.org/10.1007/s10651-016-0345-z
- Achcar, J. A., Fernández‐Bremauntz, A. A., Rodrigues, E. R. and Tzintzun, G. 2008. Estimating the number of ozone peaks in Mexico City using a nonhomogeneous Poisson model. Environmetrics 19, 469–485. doi:https://doi.org/10.1002/env.890
- Achcar, J. A., Rodrigues, E. R. and Tzintzun, G. 2011. Using nonhomogeneous Poisson models with multiple change‐points to estimate the number of ozone exceedances in Mexico City. Environmetrics 22, 1–12. doi:https://doi.org/10.1002/env.1029
- Bar-Lev, S. K., Lavi, I., and Reiser, B. 1992. Bayesian inference for the power law process. Ann. Inst. Stat. Math. 44, 623–639
- Bayissa, Y. A., Moges, S. A., Xuan, Y., Van Andel, S. J., Maskey, S. and co-authors. 2015. Spatio-temporal assessment of meteorological drought under the influence of varying record length: The case of Upper Blue Nile Basin, Ethiopia. Hydrolog. Sci. J. 60, 1927–1942.
- Burrough, P. A. and McDonnell, R. 1998. Principles of GIS: Oxford University Press New York. NY, USA. ·
- Cid, J. E. R. and Achcar, J. A. 1999. Bayesian inference for nonhomogeneous Poisson processes in software reliability models assuming nonmonotonic intensity functions. Comput. Stat. Data Anal. 32, 147–159. doi:https://doi.org/10.1016/S0167-9473(99)00028-6
- Chen, S. and Guo, J. 2017. Spatial interpolation techniques: their applications in regionalizing climate-change series and associated accuracy evaluation in Northeast China. Geomatics, Nat. Hazards Risk. 8, 689–705. doi:https://doi.org/10.1080/19475705.2016.1255669
- Cox, D. and Lewis, P. 1966. The statistical analysis of series of events.
- Cressie, N. 1992. Statistics for spatial data. Terra Nova 4, 613–617. doi:https://doi.org/10.1111/j.1365-3121.1992.tb00605.x
- Diggle, P. J. 2013. Statistical Analysis of Spatial and Spatio-Temporal Point Patterns. Boca Raton, Florida: Chapman and Hall/CRC.
- Edwards, C., McKee, T., Doesken, N., and Kleist, J. 1997. Historical analysis of drought in the United States. In: 7th conference on climate variations, 77th AMS annual meeting, Vol. 27.
- Ellahi, A., Almanjahie, I. M., Hussain, T., Hashmi, M. Z., Faisal, S. and co-authors. 2020. Analysis of agricultural and hydrological drought periods by using nonhomogeneous Poisson models: Linear intensity function. J. Atmos. Sol. Terr. Phys. 198, 105190. doi:https://doi.org/10.1016/j.jastp.2020.105190
- Entin, J. K., Robock, A., Vinnikov, K. Y., Hollinger, S. E., Liu, S. and co-authors. 2000. Temporal and spatial scales of observed soil moisture variations in the extratropics. J. Geophys. Res. 105, 11865–11877. doi:https://doi.org/10.1029/2000JD900051
- Ghysels, E., Harvey, A. C. and Renault, E. 1996. 5 Stochastic volatility. Handbook of Statistics 14, 119–191. doi:https://doi.org/10.1016/S0169-7161(96)14007-4
- Gidey, E., Dikinya, O., Sebego, R., Segosebe, E., Zenebe, A. and co-authors. 2018. Modeling the spatio-temporal meteorological drought characteristics using the standardized precipitation index (SPI) in Raya and its environs. Earth Syst. Environ. 2, 281–292. doi:https://doi.org/10.1007/s41748-018-0057-7
- Guarnaccia, C., Quartieri, J., Tepedino, C. and Rodrigues, E. R. 2015. An analysis of airport noise data using a nonhomogeneous Poisson model with a change-point. Appl. Acoust. 91, 33–39. doi:https://doi.org/10.1016/j.apacoust.2014.12.002
- Hussain, M. and Mumtaz, S. 2014. Climate change and managing water crisis: Pakistan’s perspective. Rev. Environ. Health 29, 71–77.
- Iervolino, I., Giorgio, M. and Polidoro, B. 2014. Sequence‐based probabilistic seismic hazard analysis. Bull. Seismol. Soc. Am. 104, 1006–1012. doi:https://doi.org/10.1785/0120130207
- Kuo, L. and Yang, T. Y. 1996. Bayesian computation for nonhomogeneous Poisson processes in software reliability. J. Am. Stat. Assoc. 91, 763–773. doi:https://doi.org/10.1080/01621459.1996.10476944
- Leininger, T. J. and Gelfand, A. E. 2017. Bayesian inference and model assessment for spatial point patterns using posterior predictive samples. Bayesian Anal. 12, 1–30.
- Malvić, T. 2008. Kriging, cokriging or stochastical simulations, and the choice between deterministic or sequential approaches. Geol. Croat. 61, 37–47.
- Meyer, R. and Yu, J. 2000. BUGS for a Bayesian analysis of stochastic volatility models. J. Econom. 3, 198–215. doi:https://doi.org/10.1111/1368-423X.00046
- McKee, T. B., Doesken, N. J. and Kleist, J. 1993. The relationship of drought frequency and duration to time scales. In: Proceedings of the 8th Conference on Applied Climatology, Vol. 17, Boston, MA, American Meteorological Society, pp. 179–183.
- Merabti, A., Martins, D. S., Meddi, M. and Pereira, L. S. 2018. Spatial and time variability of drought based on SPI and RDI with various time scales. Water Resour. Manage. 32, 1087–1100. doi:https://doi.org/10.1007/s11269-017-1856-6
- Mishra, A. K. and Singh, V. P. 2010. A review of drought concepts. J. Hydrol. 391, 202–216. doi:https://doi.org/10.1016/j.jhydrol.2010.07.012
- Mohanty, B. P., Famiglietti, J. S. and Skaggs, T. H. 2000. Evolution of soil moisture spatial structure in a mixed vegetation pixel during the Southern Great Plains 1997 (SGP97) Hydrology Experiment. Water Resour. Res. 36, 3675–3686. doi:https://doi.org/10.1029/2000WR900258
- Nasrollahi, M., Khosravi, H., Moghaddamnia, A., Malekian, A., Shahid, S. and co-authors. 2018. Assessment of drought risk index using drought hazard and vulnerability indices. Arab. J. Geosci. 11, 606. doi:https://doi.org/10.1007/s12517-018-3971-y
- Pebesma, E. J. 2004. Multivariable geostatistics in S: the gstat package. Comput. Geosci. 30, 683–691. doi:https://doi.org/10.1016/j.cageo.2004.03.012
- Rodrigues, E. R., Gamerman, D., Tarumoto, M. H. and Tzintzun, G. 2015. A nonhomogeneous Poisson model with spatial anisotropy applied to ozone data from Mexico City. Environ. Ecol. Stat. 22, 393–422. doi:https://doi.org/10.1007/s10651-014-0303-6
- Su, Y. S. and Yajima, M. 2012. R2jags: A Package for Running jags from R. R package version 0.03-08, URL http://CRAN.R-project.org/package=R2jags.
- Webster, R. and Oliver, M. A. 2007. Geostatistics for environmental scientists. John Wiley & Sons.
- Zambrano, F., Wardlow, B., Tadesse, T., Lillo-Saavedra, M., Lagos, O. and co-authors. 2017. Evaluating satellite-derived long-term historical precipitation datasets for drought monitoring in Chile. Atmos. Res. 186, 26–42. doi:https://doi.org/10.1016/j.atmosres.2016.11.006
- Zhao, Q., Chen, Q., Jiao, M., Wu, P., Gao, X., Ma, M. and Hong, Y. 2018. The temporal-spatial characteristics of drought in the Loess Plateau using the remote-sensed TRMM precipitation data from 1998 to 2014. Remote Sensing 10, 838. doi:https://doi.org/10.3390/rs10060838
- Zhao, Q., Chen, Q., Jiao, M., Wu, P., Gao, X. et al. 2018. The temporal-spatial characteristics of drought in the Loess Plateau using the remote-sensed TRMM precipitation data from 1998 to 2014. Remote Sensing 10, 838. doi:https://doi.org/10.3390/rs10060838