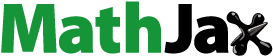
Abstract
An optimal network design was carried out to prioritise the installation or refurbishment of greenhouse gas (GHG) monitoring stations around Africa. The network was optimised to reduce the uncertainty in emissions across three of the most important GHGs: CO2, CH4, and N2O. Optimal networks were derived using incremental optimisation of the percentage uncertainty reduction achieved by a Gaussian Bayesian atmospheric inversion. The solution for CO2 was driven by seasonality in net primary productivity. The solution for N2O was driven by activity in a small number of soil flux hotspots. The optimal solution for CH4 was consistent over different seasons. All solutions for CO2 and N2O placed sites in central Africa at places such as Kisangani, Kinshasa and Bunia (Democratic Republic of Congo), Dundo and Lubango (Angola), Zoétélé (Cameroon), Am Timan (Chad), and En Nahud (Sudan). Many of these sites appeared in the CH4 solutions, but with a few sites in southern Africa as well, such as Amersfoort (South Africa). The multi-species optimal network design solutions tended to have sites more evenly spread-out, but concentrated the placement of new tall-tower stations in Africa between 10ºN and 25ºS. The uncertainty reduction achieved by the multi-species network of twelve stations reached 47.8% for CO2, 34.3% for CH4, and 32.5% for N2O. The gains in uncertainty reduction diminished as stations were added to the solution, with an expected maximum of less than 60%. A reduction in the absolute uncertainty in African GHG emissions requires these additional measurement stations, as well as additional constraint from an integrated GHG observatory and a reduction in uncertainty in the prior biogenic fluxes in tropical Africa.
1. Introduction
Population growth in Africa is expected to double from 1.3 billion to 2.5 billion by the year 2050 (United Nations, Citation2019), with a consequent increase in demand for energy and natural resources (Cerutti et al., 2015; López-Ballesteros et al., Citation2018). In contrast to Europe, America and Australia, where over 70% of people live in urban areas, only 40% of the African population currently lives in urban areas, but this is set to increase to 50% by 2050 (United Nations, Citation2019). Therefore, while African emissions from fossil fuel use and cement production are still small – only 3.6% of the global total (Boden et al., Citation2017) – this is set to increase in the future. Emissions from land use, land use change and forestry (LULUCF) make up over a third of the continent’s total carbon emissions – a relatively large proportion compared with other regions – and globally the absolute LULUCF emissions from tropical Africa are the third largest (Li et al., Citation2017). While African anthropogenic greenhouse gas (GHG) emissions may have been considered small in the past, the role of Africa in global GHG emission budgets is growing. In addition, the role of the terrestrial biosphere in sources and sinks of GHGs across Africa are not well understood or quantified, with resulting large uncertainties in the African terrestrial biosphere GHG budget. This means that it is becoming increasingly important to constrain the uncertainty in the emissions from all sources in Africa.
To achieve this, more measurements of GHG concentrations in the atmosphere are needed in Africa. Large uncertainties still exist on the global, continental and regional emission budgets of the most dominant GHGs – CO2, CH4, and N2O. Accurate evaluation of the major GHG budgets of countries and regions is essential if a global mitigation regime is to be effective, and are a requirement of the 2018 Paris Agreement. A key element of the agreement is a global stocktake of emission budgets, which quantify the amount of GHG produced by different sectors and by different activities, such as power generation. The first of these stocktakes is set to take place in 2023 (Northrop et al., Citation2018). Budgets, or inventories, are commonly developed at the national level using bottom-up techniques which consider activity data from individual processes, e.g. levels of traffic or coal consumption at power plants, to estimate the emissions at the sectoral level, e.g. emissions from a country’s manufacturing sector, from aviation, or road transport. Regularly updating budgets is important for assessing progress towards climate change mitigation goals.
Inverse modelling is a top-down approach that can be used to further constrain bottom-up estimates of sources and sinks that are provided by GHG inventories and models of biospheric processes, and which are aggregated to build GHG budgets at the national and regional levels. These budgets describe the amount of GHG that is produced (released into the atmosphere) or captured (removed from the atmosphere) by anthropogenic and natural processes. These budgets can be expressed as fluxes of a GHG, which describe the rate at which the GHG is emitted or absorbed by a particular process or set of processes. Inverse modelling makes use of atmospheric observations of GHG concentrations that are independent of these bottom-up estimates (Enting and Mansbridge, Citation1989; Rayner et al., Citation1999; Rödenbeck et al., Citation2003; Chevallier et al., Citation2010). This technique is based on the premise that a GHG concentration measured at a particular time and location, can be modelled by combining information on atmospheric transport, fluxes of these GHGs, and boundary concentrations (in the case of a limited domain, or regional, inversion). The fluxes can be determined from the information available in the bottom-up inventories, together with the uncertainties associated with each process. Standard atmospheric inversion techniques typically rely on precise – continuous or flask – in situ measurements of atmospheric GHG concentrations (expressed as mole fractions), normally from tall towers, which are used to refine the prior estimates of the GHG emissions and uptake. Tall towers allow the sampling inlets for the GHG measurement instruments to be placed at heights tall enough to be representative of the GHG concentrations in the planetary boundary layer without undue local influence from the surface (Haszpra et al., Citation2015). The appropriate height required is site-specific and depends on the surrounding topography. It can range from 10 m above ground level (magl) (e.g. for a coastal site like Mace Head Atmospheric Research Station) up to 300 m for an inland continental tower (e.g. Boulder Atmospheric Observatory). The measurements used for the inverse modelling approach are further filtered based on a variety of approaches to ensure measurements are chosen that can be resolved by the atmospheric transport model. The GHG measurement instruments at these sites measure mole fractions extremely precisely: better than 10 ppb for CO2, 0.3 ppb for CH4, and 0.2 ppb for N2O. Measurements can be of the GHGs themselves, and of other co-tracers, like carbon monoxide or ethane, which can be used to further constrain sectoral emissions of these GHGs.
Radiative forcing is a measure of the balance in incoming and outgoing energy from the Earth-atmosphere system. Increasing GHGs in the atmosphere leads to a more positive radiative forcing, which in turn leads to global warming. N2O and CH4 are both potent GHGs, with global warming potentials of 265 and 28 over a 100 year period compared with CO2 (Myhre et al., 2013), but because of the abundance of CO2 in the atmosphere, it has the greatest human-induced radiative forcing effect (0.18 (N2O) and 0.62 (CH4) versus 1.95 (CO2) Wm−2, respectively) (Etminan et al., Citation2016). While atmospheric CO2 concentrations are increasing, mainly due to burning of fossil fuels, increases in CH4 and N2O have also been noted in the atmosphere. Taken together, these constituent GHGs have a large effect on human-induced radiative forcing in the atmosphere (Rigby et al., Citation2017; Nisbet et al., Citation2019; Thompson et al., Citation2019). A definitive conclusion on what is causing these increases in atmospheric CH4 and N2O is prevented by a lack of measurements of concentrations and fluxes of these GHGs from processes suspected of contributing to these GHGs around the globe, which is likely due to a combination of factors such as the global increase in shale gas and oil extraction (Howarth, Citation2019) and the impact of climate change on microbial activity (Nisbet et al., Citation2016). The increase in the growth rate of atmospheric CH4 is large enough to challenge commitments to the Paris Agreement, and therefore it is essential to find out what these sources are, and from where they are coming (Nisbet et al., Citation2019). The degree to which these processes impact the African CH4 budget is uncertain. Increases in anthropogenic N2O are mainly due to N-fertilizer use in the agricultural sector (Thompson et al., Citation2019), which is highly variable and uncertain across Africa. Therefore, it is important to monitor all three GHGs.
To meet this objective the ‘Supporting EU-African Co-operation on Research Infrastructures for Food Security and Greenhouse Gas Observations’ (SEACRIFOG) project (www.seacrifog.eu) was established. The goal of this project was to lay the groundwork for a network of environmental research infrastructures for the systematic long-term observation of key variables relevant to climate forcing across Africa and the surrounding oceans (Beck et al., Citation2019). The intention of this project was to design this network tailored to the African context by considering those processes which contribute most to the continent’s radiative forcing and those that propagate the greatest uncertainty in continental-scale GHG reporting. This network should allow for the quantification of the continent’s anthropogenic and natural contributions to global GHG budgets at least at the same level of accuracy as is available for the rest of the world. In accordance with global estimates for the main GHGs (Saunois et al., Citation2016; Le Quéré et al., 2018), the corresponding target uncertainty for the overall African radiative forcing estimate is set to 15% at a 1 sigma (68%) confidence level. In addition, this network would have African ownership and benefit African researchers.
This paper presents the results of a network design exercise for the placement of tall tower in situ GHG measurement sites on the African continent with the objective of providing additional constraint for bottom-up estimates of both anthropogenic and biospheric components of the African GHG budgets. The analysis is based on the technique of atmospheric inversion, which relies on measurements from what are referred to as ‘atmospheric’ monitoring stations in the ICOS (Integrated Carbon Observing System) Handbook on GHG measurement techniques and standards (ICOS ERIC, Citation2020), which measure concentrations of GHGs in the planetary boundary layer and are the type of monitoring stations envisioned for this component of an integrated observation network.
These types of measurements of GHGs over Africa are sparse compared to other regions of the world, and where measurements are available, these tend to be short-term project-based measurement campaigns (less than 5 years). Studies which have aimed at optimising a global observation network have high-lighted Africa as a region associated with large uncertainty in its terrestrial GHG fluxes, urgently requiring further constraint by in situ measurements (Patra and Maksyutov, Citation2002; Borges et al., Citation2015). In particular, long-term, high precision continuous measurements are needed. On the African continent few stations have records spanning longer than a decade. The longest and most complete record belongs to Cape Point World Meteorological Organization Global Atmospheric Watch (GAW) station, which has continuous measurements of CO2 mole fractions since 1993 and of CH4 and N2O since 1983 (Labuschagne et al., Citation2018). This facility is located at Cape Point at the south-western tip of Africa (34:35 S, 18:49 E), predominantly to record baseline measurements of well-mixed, clean air originating over the Southern Ocean. This station is important for constraining Southern Hemisphere background levels of these and other tracers in the atmosphere, but it is been shown that it is difficult to improve top-down estimates of terrestrial GHG fluxes for southern Africa, relying only on a single Cape Point station (Whittlestone et al., Citation2009). Other atmospheric observatory sites in Africa or on islands near Africa measuring GHGs include a site near the Gobabeb Training and Research Centre, operational since 2012, on the west coast of Namibia (22:55 S, 15:03 E), which continuously measures trace gases, including CO2 (Morgan et al., Citation2015); Assekren in Algeria, located at an elevation of 2710 m above sea level (masl), measuring since 1996; Cape Verde Atmospheric Observatory, continuously measuring GHGs since 2008 (Carpenter et al., Citation2010); Izana, on the Canary Islands 2367 masl, continuously measuring the main GHGs since 2015 (Gomez-Pelaez et al., Citation2019); Amsterdam Island measuring GHGs since 1980 (Gaudry et al., Citation1991); and Mt. Mugogo, Rwanda, 2640 masl, recently established as part of the AGAGE (Advanced Global Atmospheric Gases Experiment) network in 2017. The primary purpose of these sites is to sample atmospheric baseline concentrations of GHGs and other tracers, and it is not possible to adequately constrain the GHG budgets for Africa through inverse modelling by only using measurements from these in situ sites.
Other types of GHG measurements in Africa have come from what are referred to as ‘ecosystem’ measurement sites and make use of the eddy-covariance measurement technique (ICOS ERIC, Citation2020), with measurements available since the early 2000s. This technique provides a direct measurement of fluxes and relies on high frequency measurements of GHGs, where the accuracy of the difference between measurements is of primary importance, while the accuracy of absolute measurements is of secondary importance. For example, in South Africa GHG measurements have been taken using the eddy-covariance technique at the Skukuza flux tower site since 2000, and recently at fourteen other sites around the country (https://efteon.saeon.ac.za/). There are a number of long-term eddy-covariance measurement records in Africa available through the network called FLUXNET (https://fluxnet.org/). There are upwards of 30 decommissioned sites that operated for campaigns of varying durations in Botswana, Sudan, Burkina Faso, Ghana and others (López-Ballesteros et al., Citation2018; Beck et al., Citation2019). Eddy-covariance sites are useful for directly characterising atmosphere-surface GHG fluxes for areas (or foot-prints) of 1 km2 or less (Aubinet, Citation2000; Vesala et al., Citation2008). The location of the sites and the height of the the measurements need to be suitable so that the assumptions regarding this method are valid, which are dependent on vegetation height, surface roughness and characteristics of the boundary layer. The sites are usually located in areas selected as representative of a larger ecosystem so that the flux estimates can be upscaled to the whole region or ecosystem. In contrast, tall-tower in situ measurements are representative of a much larger area – typically hundreds to thousands of km2 around the measurement site – providing a top-down measurement-based method to constrain GHG budgets at regional, continental and global scales. Up-scaled flux measurements from eddy-covariance sites are useful for informing biospheric flux models and can be used for comparison with flux estimates from atmospheric inversions (Kuppel et al., Citation2012; Broquet et al., Citation2013; Leip et al., Citation2018), but the measurements of GHG concentrations at these sites are usually not at an adequate height or accurate enough to be useful in atmospheric inversions. This analysis is not intended to provide information on where ecosystem measurements sites should be placed.
Measurements of column concentrations of CO2 and CH4 are available from several satellite products, with more missions set for the future. For example, column CO2 (XCO2) and XCH4 measurements are available from SCIAMACHY/Envisat (sensor/satellite), TANSO-FTS/GOSAT, and TROPOMI/Sentinel-5 Precursor, and OCO-2 provides XCO2 measurements (Kong et al., Citation2019; Reuter et al., Citation2019; Schneising et al., Citation2019). Satellite measurements of N2O are not currently available. The advantage of satellite products derived from orbiting satellites is that they provide information for a large surface area of the Earth, but the return time to a particular location can be infrequent, and measurements do not provide the temporal information on the diurnal cycle of these GHGs. Although techniques to constrain estimates of GHG budgets with these measurements are developing fast, there are still large uncertainties and biases associated with the satellite column measurements of GHGs and large discrepancies in flux estimates of these GHGs obtained using different products (Wang et al., Citation2019). There are also large inconsistencies between GHG flux estimates using in situ measurements and satellite observations (Wang et al., Citation2018). Although we expect satellite missions for GHG measurements to continue into the future, a single mission usually only lasts a finite amount of time before the instrument fails, therefore consistent long-term timeseries satellite observations are rare. We expect that tall-tower in situ measurements of GHGs will form the backbone of an integrated observation network that will include strategically placed ecosystem measurement stations and make use of satellite observations of GHG where appropriate. The network design in this paper is not intended to provide information on in situ measurements required to ground truth satellite column measurements of GHGs.
A way of designing a network for new tall-tower in situ measurement sites is to optimise the reduction in uncertainty of GHG fluxes from subregions within the domain of interest that would result from measurements at these new stations. This is based on the prior assessment of uncertainty in the flux estimates, and on how much the observational footprint of a site overlaps with regions of high flux uncertainty. We can make use of the flux uncertainty estimates produced from an atmospheric inversion to provide this information (Patra and Maksyutov, Citation2002; Tarantola, Citation2005; Ziehn et al., Citation2014; Nickless et al., Citation2015; Kaminski and Rayner, Citation2017).
In this study we aimed to determine the optimal locations to place new tall-tower observatories to reduce the uncertainty in the fluxes of CO2, CH4, and N2O for the continent of Africa. We limit the number of new stations to be between ten and twelve so that the network remains financially feasible and the optimisation calculations can be performed in a reasonable amount of time. In addition to presenting the rationale and outcome of this network design, we also investigate the amount of uncertainty reduction we can realistically expect from this network, in order to inform what improvements are needed in other components of an integrated observatory system to reach the level of 15% uncertainty that we wish to attain for estimates of the GHG budgets in Africa.
In Section 2, we introduce the model framework, the candidate sites, and data products used to provide the prior fluxes, and we provide the method used to derive the flux uncertainty using the inverse modelling approach and describe the optimisation method. The results of the network design are presented in Section 3, and discussion and concluding remarks are provided in Sections 4 and 5.
2. Methods
2.1. General overview
In order to design a network of sites that can provide the optimal amount of information on terrestrial fluxes across Africa, we need spatial information on where each candidate site is obtaining information from, and where the largest information gains are to be had. We can do this by combining the candidate site footprints, expressed as a sensitivity matrix, and the uncertainty we expect in the surface fluxes, expressed as a spatio-temporal covariance matrix describing flux uncertainties. Using a fishing boat as an analogy, the footprint would describe the fishing boat’s location and size of the net, and the uncertainty matrix would describe the amount of fish in each zone. Combining these two pieces of information will provide an estimate of the total catch from a fishing boat (in real terms, the reduction in flux uncertainty across the African continent). The goal is to find the best locations for a fleet of fishing boats so that the total catch is maximal.
The atmospheric inversion approach provides a metric for this in the form of the solution to the posterior covariance matrix, which expresses the amount of uncertainty that is left in the system after assimilating the observations. To calculate the posterior covariance matrix we do not require the observations, only the model-measurement errors. We can compare this to the prior flux covariance matrix to determine the reduction in uncertainty achieved by the atmospheric inversion.
Additional information we can take into account at each site is the discrepancy between modelled and measured concentrations we would expect at a particular site. This is mainly explained by error in the atmospheric transport model, which in the absence of site-specific data, we can assume to be similar to what is assumed for other measurement sites around the world, particularly if a standardised measurement protocol is followed at new sites (ICOS ERIC, Citation2020). In the analogy, this could be compared with the size of the holes in the fishing net, which results in a loss to the total catch. In this study we allow for site-specific model-measurement error by taking into account the sites exposure to local influence of biomass-burning, which we know to be difficult for an atmospheric transport model to replicate.
To determine which set of sites will result in the greatest reduction in flux uncertainty across Africa, we use the Incremental Optimisation approach. The metric that needs to be maximised by this approach must depend on the subset of sites considered. We use the reduction in the total flux uncertainty that we expect from the atmospheric inversion to supply this metric. The optimisation algorithm selects the site with the greatest flux uncertainty reduction to add as the first site in the network, and then proceeds to add sites in this way, taking into account the sites that have been placed in the network in the previous iterations.
In the following subsections, we describe how candidate sites were selected in Section 2.2, which is based on existing infrastructure. We provide the details on how the posterior covariance matrix was calculated, based on the solution to the Bayesian inverse modelling approach which is described in Section 2.3. The individual terms, such as the sensitivity matrix and the prior flux uncertainty matrices for each GHG, that are needed in this solution are detailed in Sections 2.4 to 2.8. Section 2.9 describes the optimisation algorithm, and 2.10 describe our novel approaches to jointly optimising the network design for three GHGs.
2.2. Candidate sites
A list of existing African sites that are, or were, part of the GAW, TCCON (Total Column Carbon Observing Network), GCOS (Global Climate Observing System), GUAN (GCOS Upper Air Network), or Fluxnet observation networks was compiled in line with the SEACRIFOG (Supporting EU-African Cooperation on Research Infrastructures for Food Security and Greenhouse Gas Observations) project (López-Ballesteros et al., Citation2018). The sites were mapped over a regular grid with extent 22ºW to 60ºE, and 40ºN to 35ºS, and grid size 8º by 8º, which corresponds roughly to the average land area of an African country. The grid size was made as fine as computationally practicable, due to a limitation on the number of candidate sites for which footprints could be generated and which the optimisation algorithm could handle in a feasible amount of time. One site was selected within each grid cell with priority given to sites that were within the GAW or TCCON networks, and to sites that were operational. If no site existed within a grid cell, then a hypothetical site was created at the centre of the grid cell. Focusing on sites where atmospheric measurements were already taking place ensured that at least some infrastructure and human resource support is available for each site, which reduces the costs of starting GHG measurements at these locations.
The resulting list of candidate sites consisted of 51 locations (). The optimisation aimed to select between ten and twelve of these sites that would bring about the highest uncertainty reduction. Ultimately, the exact location of future stations will be largely determined by practical considerations, such as topography, the presence of existing infrastructure – such as communication towers and meteorological stations – available manpower, the relative security of the instruments, and the accessibility of the sites. Eight established sites for measuring CO2, CH4 and N2O (five outside of Africa and three within Africa), in either the GAW or ICOS networks, were included as sites in the base network. The footprints describing the atmospheric transport at the European sites were retrieved primarily to test the output of the atmospheric transport model against well-established sites. These European sites could potentially be used to inform boundary conditions in the northern part of an African domain in a full regional inversion, but we had to limit the number of these sites due to computational resource constraints (). The number of sites in the solution was guided by a realistic assessment of what might be feasible and fundable over the next decade. Not all of the operating observational towers, such as Gobabeb, were included as existing stations due to previous discontinuities in the records at these stations. Sensitivity tests and previous studies (Nickless et al., Citation2015) confirmed that including these as candidate sites or existing sites did not influence the final network design solution.
Fig. 1. The 51 potential locations of the new stations in the optimal network design and the mean fossil fuel emissions from the ODIAC product regridded onto the in g C m−2 month−1 for the year 2012. Uncertainty estimates were set at 100% of the estimated net primary productivity flux estimates. The candidate site locations were based on existing infrastructure where possible. The existing Cape Point (South Africa), Cabauw20 (The Netherlands), Cabauw200, Mace Head (Ireland), OPE (France), Hungary, Lamto (Ivory Coast) and Ifrane (Morocco) stations are listed as 59, 1, 2, 3, 4, 5, 32, and 54. These were included in the base network.

Table 1. A table of 51 candidate sites selected from existing observation networks and 8 established European (*) or African sites (**), making up the base network to which new sites will be added. Two imaginary sites were created in grid cells where no candidate sites were available (sites 38 (Algeria) and 48 (Somalia)).
Table 2. Ranking of the new stations added to the base network for 4 three-month periods. The station with rank 1 achieved the highest uncertainty reduction in the total CO2 flux for Africa. The cumulative reduction in uncertainty relative to the base uncertainty is provided in brackets.
2.3. Bayesian inversion method
Modelling the concentration of a GHG at a site using a large number of regional fluxes as inputs must contend with the problem that several configurations of the regional fluxes could produce the same modelled concentration mole fraction value. The problem is said to be underdetermined or ill-conditioned. Bayesian inverse modelling is often used to solve for the fluxes in an atmospheric inversion (Rayner et al., Citation1996; Bousquet et al., Citation1999; Kaminski et al., Citation1999; Rayner et al., Citation1999; Enting, Citation2002; Gurney et al., 2002; Peylin et al., Citation2002; Gurney et al., Citation2003; Law et al., 2003; Baker et al., 2006; Ciais et al., Citation2010), as this approach limits the solution space for the fluxes. This method provides a way of ‘regularising’ the estimates of the fluxes by including prior information on the fluxes, which constrains the posterior solution. We use the framework of a regional inversion approach which aims to solve for surface fluxes in a gridded domain. The solution to this Bayesian inverse modelling problem provides the posterior covariance matrix of the fluxes, which provides the information on how much the uncertainty in the fluxes has been reduced by the atmospheric mole fraction observations.
The observed mole fractions (c) at a measurement site at a given time can be expressed as the sum of contributions (in mole fraction units) from the surface fluxes and from the boundaries of the spatial domain of the inversion. These contributions added together will give an estimate of the atmospheric mole fraction of the GHG at the site. Ziehn et al. (Citation2014) and Nickless et al. (Citation2015) showed that the changes to the posterior uncertainties in the boundary mole fractions compared to the prior uncertainties were very small, and therefore had little impact on the uncertainty reduction. For the purposed of the network design, we can therefore only consider the contributions to c which are attributable to the surface fluxes within the inversion domain. Moreover, we are interested in designing a network that constrains surface flux emissions in the African domain, and therefore focus on reducing the uncertainties in these fluxes. For convenience, we refer to the the modelled mole fraction contributions from the surface fluxes as cmod. The relationship between these mole fraction contributions and the sources, s, can be modelled using the following linear equation:
(1)
(1)
where cmod are the modelled mole fractions, s are the surface fluxes, and H is the sensitivity matrix, which projects the fluxes into the mole fraction space and provides the contribution from each spatio-temporal gridded surface flux to the total mole fraction contribution at the measurement site. In the full inversion setting the vector s would be composed of surface fluxes and boundary concentrations (Lauvaux et al., Citation2012; Ziehn et al., Citation2014; Nickless et al., Citation2015, Citation2018a).
The inversion framework that we used solved for gridded surface fluxes over a three-month period. The flux in a grid cell represented the total flux, calculated as the sum of the biogenic fluxes and fossil fuel fluxes. We solved for three-monthly fluxes as this would allow us to investigate the effect of seasonal variation in the fluxes on the network design. Solving for a fluxes with the same mean over a three-month period allowed for seasonal variation, but ensured that the posterior uncertainty covariance matrix was not too large for computational purposes. For the purpose of the network design we solved for the total flux, but a real-world inversion, aimed at modelling the observed GHG mole fractions at a site, would most likely solve for the component fluxes separately (e.g. fossil fuel and biogenic fluxes) (Chevallier et al., Citation2014; Kaminski and Rayner, Citation2017; Nickless et al., Citation2018a, Citation2019b; White et al., Citation2019). We solve for the uncertainty reduction of gridded fluxes at a relatively high resolution in order to allow the network design to take into account nuances in atmospheric transport that are due to large scale topography, and information on localised emissions.
Biomass burning can potentially impact on the observed mole fractions of GHGs at a site, but these emissions are not ideally observed using tower-based in situ atmospheric measurements. This is because some biomass burning emissions would exit the surface layer before being observed at the site, and the way these emissions rise out of the surface layer is difficult to model.
The solution to the Bayesian inversion posterior flux error covariance matrix, Cs, can be calculated as follows (Tarantola, Citation2005):
(2)
(2)
(3)
(3)
where Cc is the covariance matrix of the model-measurement errors, and
is the prior uncertainty covariance matrix of the surface fluxes. The subsequent sections provide details on how each of these matrices are produced.
2.4. Lagrangian particle dispersion model (LPDM)
The sensitivity matrix, H, was derived by running a Lagrangian particle dispersion model (LPDM) in backward mode. LPDMs are stochastic models that simulate the trajectories of a large number of air parcels (referred to as particles). The particle histories represent transport due to mean ambient flow, turbulence, and horizontal and vertical diffusive transport (Pisso et al., Citation2019). The LPDM is an offline model driven by three-dimensional meteorological fields. The particles were released from the candidate measurement locations and travelled to the surface and the boundaries (Seibert and Frank, Citation2004). The surface is taken to be the layer of air between the ground surface and 50 magl. The boundaries are the curtains of this box. We used FLEXPART version 10.3 (Pisso et al., Citation2019). The location of each particle at discrete time steps from the release time were recorded by the LPDM, and this information was accumulated into a spatio-temporal matrix of particle counts. Seibert and Frank (Citation2004) provides details on how these particle counts can be transformed into the sensitivity matrix, H, which projects the surface fluxes into the concentration space of the selected GHG.
FLEXPART was driven by meteorological fields in gridded format generated by the European Centre for Medium-Range Weather Forecasts ERA-Interim meteorological analyses for the year 2012, and particle counts were calculated at three-hourly intervals at each of the candidate sites. A total of 60,000 particles were released over the course of each three-hour period, and these remained live for 10 days (Pisso et al., Citation2019). The choice of 60,000 particles ensured a stable solution for the sensitivity matrix, so that each region was representatively sampled. The lifespan of 10 days for each particle ensured that the regions to which the mole fraction observation at the measurement site were most sensitive were well sampled sufficiently back in time. Surface fluxes from regions where older particles would have reached had negligible impact on the mole fraction observation at the site.
In order to generate the sensitivity matrix, particle counts were aggregated to a gridded domain with a spatial resolution of 0.4º by 0.4º (238,238 pixels for the African domain under consideration). The height of each surface grid cell was set at 50 m. Each row in the sensitivity matrix, H, represented a three-hourly mean mole fraction. Each column represented a surface grid cell flux within the domain. The particle counts back in time were integrated over a three-month period within each grid cell, but night and daytime periods were separated. H had separate sensitivities for night and day due to the strong diurnal cycle in biogenic CO2 fluxes. Therefore the H provided sensitivities of each three-hourly mean mole fraction to three-monthly fluxes, which assumed that biogenic and anthropogenic fluxes remained relatively homogeneous over a season. This was to ensure that the prior flux uncertainty covariance matrices, discussed in Sections 2.6, 2.7 and 2.8, were of a manageable size to allow for the computation of the posterior flux covariance matrices to take place in a reasonable amount of time with the available computational memory resources, while still allowing some seasonality in the fluxes.
The LPDM model was run for a full year. Although it would be ideal to have the sensitivity matrix for several years, it is not feasible to run an LPDM model for a large number of candidate sites at the spatial resolution that we carried out for the network design over multiple years. Therefore, network designs are often performed over a limited time period (Ziehn et al., Citation2014; Nickless et al., Citation2015; Lopez-Coto et al., Citation2017). In this case we ran the LPDM model for the full year of 2012, for which we had well-validated meteorological data driving the LPDM model and reliable prior information.
2.5. Model-measurement error covariance matrix
The diagonal elements of the model-measurement error covariance matrix, Cc, contain the measurement error as well as the error in modelling the mole fractions, which occurs due to an imperfect representation of atmospheric transport. The measurement error is assumed to be much smaller than the error in modelling the mole fractions. In a typical inversion the measurement error can be diagnosed using the observed mole fractions and performance of the instrument when measuring calibration or reference gases (Thompson and Stohl, Citation2014). Although we do not have these observation data available, we can assume that the measurement errors, in the case of GHG measurements that follow the GAW or ICOS recommendations, will be at least an order of magnitude smaller than the transport modelling errors (Bergamaschi et al., Citation2015). The errors in modelling the observations are assigned based on assumptions of how well the transport model can recreate the observed mole fractions, if the true source fluxes were known. Overall transport model errors can be thought to consist of two main parts, ‘representation’ and ‘aggregation’ errors. Representation errors occur as a result of the discrepancy between the modelled mole fraction that is representative of a volume, which we get from the LPDM model, and the measurement which is taken at a point in this volume. This discrepancy should be mitigated by the use of averaged measurements of the mole fraction.
Aggregation errors – from both temporal and spatial aggregation – are as a result of the discretisation of fluxes in space and time. When the fluxes are aggregated over space and time, these fluxes are homogenised, losing the spatial and temporal heterogeneity in the flux field within each grid cell. The air parcel which reaches the measurement site may have passed over only a small feature within the grid cell, but it carries the information of the homogenised grid cell. A full description of these types of model-measurement errors can be found in Ciais et al. (Citation2010) and Kaminski and Rayner (Citation2017). The model-measurement errors lead to a reduction in the information that a measured mole-fraction can supply to the estimate of the fluxes. The larger these errors are assumed to be, the more dependent the solution of the posterior flux covariance matrix is on the prior flux covariance matrix.
A model-measurement error of 2 ppm was assigned to each three-hourly CO2 mole fractions, similar to previous network designs for South Africa (Nickless et al., Citation2015, Citation2018b) and Australia (Ziehn et al., Citation2014), and used in previous regional CO2 inversions (Nathan et al., 2018). Following Ganesan et al. (Citation2015), we assigned model-measurement error uncertainties to each three-hourly CH4 and N2O mole fraction observation of 10 ppb and 0.4 ppb, respectively.
Site-specific adjustments for each candidate site were made to the model-measurement errors, based on the influence of biomass burning on the candidate site. Biomass burning episodes are expected to be poorly represented by atmospheric transport models and measurements made at tower sites will only partially capture emissions from these episodes. Therefore, it would be a disadvantage to establish a site strongly influenced by local biomass burning, as it would be challenging to model the mole fraction concentrations at this site and attribute surface fluxes of the GHGs to these measurements. To account for this additional source of modelling error, the contributions of biomass burning emissions to three-hourly mole fraction concentrations during each three-month period were calculated for each candidate site. This was achieved by multiplying the sensitivity matrix, H, for each candidate site with the vector of biomass burning emissions. This was carried out for all three gas species. These emissions were estimated from the Global Fire Emissions Database version 4 (GFED4) product (van der Werf et al., Citation2017). The largest modelled contribution to a single three-hourly averaged measurement within each three-month period was set as the biomass burning error and represented the maximum potential influence of biomass burning on a three-hourly measurement taken at the candidate site. The total measurement-model error for each candidate site (represented by diagonal variance terms in the model-measurement error covariance matrix) was taken as the quadratic sum of the GHG-specific base model-measurement error and the site-specific biomass burning model error. The quadratic sum is taken as it is assumed that the sources of model-measurement error are independent. Therefore, each site had a customised model-measurement error covariance matrix, where sites that had strong potential influence from biomass burning were assigned larger errors. As the temporal and spatial correlation terms of the model-measurement covariance matrix are difficult to model in the absence of observations, and since these errors should contribute negligibly to posterior uncertainty covariance matrix, for the purposes of this network design, we assume the elements of the model-measurement covariance matrix are independent.
2.6. Prior CO2 flux uncertainty covariance matrix
To derive the diagonal elements of the prior flux uncertainty covariance matrix, we summed the prior uncertainty variances in the fossil fuel and biogenic fluxes, assuming these fluxes were independent. We used the fossil fuel emissions for the year 2012 from the ODIAC (Open-source Data Inventory for Anthropogenic CO2) product (https://odiac.org/index.html), and set the uncertainty at 100% of the estimate for each grid cell (Oda and Maksyutov, Citation2011; Lauvaux et al., Citation2016; Oda et al., Citation2017, Citation2018) (). This product makes use of global energy consumption statistics and distributes the emissions from these activities based on known point source emitters, such as power plants, and on a global nightlight distribution satellite product. Emissions from point and diffuse sources are estimated separately. The uncertainties in the biogenic fluxes were set to the same magnitude as the net primary productivity estimated by the digital global vegetation model LPJ (Lund-Potsdam-Jena) S2 (CO2 and climate [time-invariant present-day land use mask]) run from the TRENDY version 1 database (Trends in net land-atmosphere carbon exchange over the period 1980–2010 project) (http://dgvm.ceh.ac.uk/node/21/) (Sitch et al., Citation2015) (). LPJ simulates energy, water and carbon fluxes in a modular framework based on plant functional types (Sitch et al., Citation2003). Using the net primary productivity as a surrogate for the uncertainty in the net biospheric flux meant that the error assigned to these fluxes was large. This approach was used because small net ecosystem exchange fluxes could result from large gross primary productivity and ecosystem respiration fluxes, and therefore a proportional uncertainty allocation based on the net flux could be misleading. Large proportional errors were assigned as models for CO2 biospheric fluxes have been shown to be highly sensitive to parameter uncertainty, such as those associated with soil temperature and moisture (Exbrayat et al., Citation2013).
Fig. 2. The mean net primary productivity (NPP) for the periods (a) January to March, (b) April to June, (c) July to August, and (d) September to December 2012. The uncertainty in net ecosystem exchange was set at 100% of the absolute NPP fluxes at the resolution of 0.2º expressed in g C m−2 month−1. NPP as estimated by the digital global vegetation model LPJ (Lund-Potsdam-Jena) S2 (CO2 and climate [time-invariant present-day land use mask]).
![Fig. 2. The mean net primary productivity (NPP) for the periods (a) January to March, (b) April to June, (c) July to August, and (d) September to December 2012. The uncertainty in net ecosystem exchange was set at 100% of the absolute NPP fluxes at the resolution of 0.2º expressed in g C m−2 month−1. NPP as estimated by the digital global vegetation model LPJ (Lund-Potsdam-Jena) S2 (CO2 and climate [time-invariant present-day land use mask]).](/cms/asset/5b24e71b-5b5a-418d-8618-ec2345cc7dda/zelb_a_1824486_f0002_c.jpg)
We did not assign an uncertainty to the ocean fluxes, as the sensitivity of the candidate sites to these fluxes should be small relative to those from the terrestrial sources, and at a pixel level, these fluxes should be relatively small. This way we ensured that the network deliberately aimed to reduce uncertainty in the terrestrial fluxes, rather than reducing the overall uncertainty by reducing oceanic flux uncertainty, which we assumed would be better left to oceanic sites or shipping vessel observations. Similarly, in order to model the observed mole fraction at the site, the contribution from the boundaries would be required. Although these boundary mole fraction estimates and their uncertainties are important in the context of a real-world inversion, we ignored the uncertainty in the boundary mole fractions so that sites were not selected to reduce these uncertainties.
We set the off-diagonal elements of the prior flux uncertainty covariance matrix to zero. In an inversion using these measurements to solve for fluxes, we would account for spatial uncertainty correlations in the fluxes. An observation network that optimised the uncertainty reduction in the most uncertain pixels would also be optimised to reduce uncertainty if these flux uncertainties were spatially correlated as well (however, the magnitude and spatial distribution of the uncertainty reduction would be modified if off-diagonal elements were included). Setting the flux uncertainty covariance matrix as diagonal for the purposes of the optimal network design is advantageous computationally, as the flux covariance matrices are large and a new posterior flux covariance matrix needed to be calculated every time the set of candidate sites changed.
2.7. Prior CH4 flux uncertainty covariance matrix
Estimates of CH4 fluxes were obtained from several sources, following the approach of Palmer et al. (Citation2018). Anthropogenic emissions included emissions from waste, energy, industry, and agriculture, and fugitive emissions from gas flaring and venting during oil and gas production. These were obtained from the Emission Database for Global Atmospheric Research (EDGAR) v4.3.2 (Janssens-Maenhout et al., Citation2012).
Wetland and rice emissions were taken from Bloom et al. (Citation2012). Other natural emissions, including the soil sink negative flux, volcanoes and emissions from termites, were taken from Fung et al. (Citation1991). The total prior CH4 fluxes are presented in . The uncertainties were set at 100% of the prior estimate.
Fig. 3. The prior CH4 fluxes at the resolution of 0.4º expressed in g CH4 m−2 month−1 for periods January to March, April to May, June to August, and September to December 2012. Anthropogenic emissions from EDGAR v4.3.2 (Janssens-Maenhout et al., Citation2012), wetland and rice emissions from Bloom et al. (Citation2012), and other natural emissions, including the soil sink negative flux, volcanoes and emissions from termites from Fung et al. (Citation1991).

In this network optimisation exercise we did not account for the tropospheric OH sink. In a global inversion, this term represents a substantial loss of CH4 (Palmer et al., Citation2018). However, given the long lifetime of CH4, OH uncertainties have little influence on regional flux inversions (lifetime is approximately ten years compared to the transport model simulation timescale of ten days).
2.8. Prior N2O flux uncertainty covariance matrix
As for the CH4 fluxes, uncertainties in N2O fluxes were assigned at 100% of the prior estimate. The prior estimates for anthropogenic emissions were derived from EDGAR v4.3.2, as were used in Ganesan et al. (Citation2015). Natural emissions from soils, mostly due to bacterial processes of nitrification and denitrification, were derived from emissions reported for 2008 in Saikawa et al. (Citation2013) following Ganesan et al. (Citation2015).
2.9. Optimisation
Three optimisation routines have been most commonly used for optimal network design of atmospheric greenhouse gas observations in the literature: namely incremental optimisation (IO) (Patra and Maksyutov, Citation2002; Ziehn et al., Citation2014; Nickless et al., Citation2015, Citation2018b), genetic algorithm (Rayner, Citation2004), and simulated annealing (Rayner et al., Citation1996). The IO procedure has been shown to work well for design of an observation network in comparison with more computationally demanding procedures, like simulated annealing and the genetic algorithm methods (Patra and Maksyutov, Citation2002; Nickless et al., Citation2015, Citation2018b).
To implement the IO procedure, we added one station at a time from the candidate site list to our base network of eight stations and calculated Cs. We chose the station that resulted in the largest uncertainty reduction and added it to the network, simultaneously removed it from the candidate list. We then repeated the process until we reached a network size of ten to twelve new stations. The solution proceeded to ten stations for the individual GHGs as the additional uncertainty reduction achieved by adding beyond ten stations was close to zero for all three species. The IO procedure provides us with a stepwise progression of the optimal network.
The overall uncertainty in fluxes can be expressed as the sum over all the elements of Cs (JCs):
where n is the number of elements in the diagonal of Cs. We evaluated the different networks in terms of their uncertainty reduction:
where UR is the uncertainty reduction, JCs is the optimised uncertainty metric value and JCs base the value of the uncertainty metric calculated from the posterior error covariance matrix of the fluxes if only the base stations are included.
2.10. Multi-species network design
Two approaches were used to create a single solution for the location of new stations, both aimed to optimise the overall uncertainty reduction in all three GHGs. The first approach made use of the separate solutions across different GHGs and seasons. As the largest uncertainty reductions were achieved during the Northern and Southern Hemisphere summer periods in the single species cases for CO2 () and N2O (), the multispecies solution was limited to selecting sites from January to March and July to August. In this approach, we ensured that the top sites from all three species were included in the solution. The four sites that achieved the highest uncertainty reduction in the Northern and Southern Hemisphere summer CO2 solutions were added to the solution, followed by the top four summer sites from the CH4 and then from the N2O solutions; a total of twelve stations. If a site already existed in the combined solution, then the next highest ranked site was selected.
Table 4. Ranking of the new stations added to the base network for 4 three-month periods. The station with rank 1 achieved the highest uncertainty reduction in the total N2O flux for Africa. The cumulative reduction in uncertainty relative to the base un-certainty is provided in brackets.
The second approach solved for the uncertainty reduction across all three gases simultaneously, and weighted the three GHGs equally. The term optimised by the IO procedure was the combined uncertainty reduction, calculated as the average percentage uncertainty reduction over all three gases. The posterior flux uncertainty covariance matrix needed to be calculated for each gas every time a site was added to the network. To do this calculation in a feasible amount of time, H was reduced in dimensions so that it provided the sensitivity to daily measurements rather than three-hourly measurements. The code, sensitivity matrices, and prior flux uncertainty matrices used to calculate the overall uncertainty reduction are provided in Nickless et al. (Citation2019a). The single-site uncertainty reduction for each candidate site calculated using this combined approach across the three GHGs is provided in the supplementary material.
3. Results
3.1. Biomass burning error
The potential contribution to an observed CO2 mole fraction measurement from biomass burning emissions was largest for those sites situated near the woodland and shrubland regions of Africa. These regions are located predominantly between 10ºN and 25ºS ( and ).
Fig. 4. The prior N2O fluxes at the resolution of 0.4º expressed in mg N2O m−2 month−1 for periods (a) January to March, (b) April to May, (c) June to August, and (d) September to December 2012. Anthropogenic emissions from EDGAR v4.3.2 and natural emissions from soils were derived from emissions reported for 2008 in Saikawa et al. (Citation2013).

Fig. 5. The potential biomass burning error in modelled CO2 mole fraction (in units of ppm) at each candidate site for the (a) January to March, (b) April to May, (c) June to August, and (d) September to December 2012 periods.

This is expected as most of the fuel load would be located in these regions. The potential error here was as high as 20 ppm during the January to March 2012 period, ten times larger than the minimum model-measurement error assigned to each site accounting for errors due to representation and aggregation error. The biomass burning contributions reduced in the April to June period, and were at a minimum during the July to August period. This corresponds to the Southern Hemisphere – where most of the sites with high biomass burning contributions are located – winter period, when biogenic activity is at the lowest for the year. Similar patterns in the distribution of biomass burning emissions for CH4 and N2O were observed (see supplementary material).
3.2. Network design to constrain CO2 fluxes
The spatial distribution of sites for limiting the uncertainty in CO2 fluxes in the optimal network was different for each season; but the selected sites were always concentrated in tropical Africa, with no sites located in Northern Africa, and only one site in southern Africa (). During the periods April to June and July to September (Northern Hemisphere summer period), optimal sites were located in the northern tropical regions of Africa, whereas during the periods October to December and January to March (Southern Hemisphere summer period), optimal sites were located predominantly south of the equator.
Fig. 6. Optimal locations to situate new atmospheric monitoring sites to an existing network to reduce the overall uncertainty of CO2 fluxes from terrestrial Africa for the periods (a) January to March, (b) April to June, (c) July to August, and (d) September to December 2012. Sites are coloured according to the rank in the optimal design, with light green sites representing the site with the largest uncertainty reduction and which is the first site added to the network.

The only site listed as an optimal location for all four seasons was Kisangani in the Democratic Republic of Congo. This was followed by Bunia, also in the Democratic Republic of Congo, which appeared in the solution for three of the seasons ().
The solutions were dominated by those sites sensitive to regions with high natural biogenic productivity.
The season for which the network was optimised had a large influence on the amount of uncertainty reduction achievable by a ten member network. The greatest uncertainty reduction from one site was by Maun, Botswana during the January to March period (Southern Hemisphere summer), reducing the uncertainty by 18.7%. The network was also able to achieve the highest uncertainty reduction during this period, reaching 54% with a total of ten sites. Over 40% reduction could be achieved by adding just four sites to the existing network. The network for the period from July to September (Northern Hemisphere summer) was able to achieve over 50% uncertainty reduction with ten sites. For this period Kassala, in Sudan, achieved the highest uncertainty reduction by a single site with 17.2% reduction.
We found diminishing returns to the total flux uncertainty reduction as we added more sites to the network. If we extrapolate the amount of uncertainty reduction with a simple three-parameter curve, even for the seasons with largest uncertainty reduction, it is difficult for the network to reach higher than 60% uncertainty reduction (). Therefore, for posterior uncertainty estimates to be small in absolute terms the prior uncertainty needs to be small enough so that a 40 or 50% reduction in this uncertainty will suffice.
3.3. Network design to constrain CH4 fluxes
The solutions for CH4 showed far less dependency on season than the solutions for CO2, with more common stations between network solutions for the four separate periods compared with the CO2 network (). There were four stations common among all network solutions. Dundo, Angola, achieving the top uncertainty reduction of 10.9% and 9.8% for the July-September and October-December periods, respectively, and located towards the middle of the solutions for the January-March and April-June periods (). Kisangani appeared in the top-half for the January-March and April-June periods and bottom-half for the remaining two periods. Amersfoort and Lubango appeared in all four solutions, but generally towards the bottom. Zoétélé, Kinshasa, and Bunia appeared in three of the four network solutions.
Fig. 8. Optimal locations to situate new atmospheric monitoring sites to an existing network to reduce the overall uncertainty of CH4 fluxes from terrestrial Africa for the periods (a) January to March, (b) April to June, (c) July to August, and (d) September to December 2012. Sites are coloured according to the rank in the optimal design, with light green sites representing the site with the largest uncertainty reduction and which is the first site added to the network.

Table 3. Ranking of the new stations added to the base network for 4 three-month periods. The station with rank 1 achieved the highest uncertainty reduction in the total CH4 flux for Africa. The cumulative reduction in uncertainty relative to the base uncertainty is provided in brackets.
The network solution was dominated by sites which showed sensitivity to sources of CH4 in the African tropics and in southern Africa. There was less variation in the total uncertainty reduction achieved by a ten station network across different seasons. The uncertainty reduction achieved by a ten station network reached up to 37.8% for the July to September period, and achieved the lowest uncertainty reduction of 31.5% the October to December period.
3.4. Network design to constrain N2O fluxes
Like the CO2 network solutions, the network solutions for constraining N2O fluxes showed a strong dependency on the time of year. During the January to March period, Southern Hemisphere summer, the network was strongly influenced by the natural soil fluxes expected over Madagascar (). The soil N2O fluxes for each season are provided in the supplementary material. The station that achieved the greatest uncertainty reduction for this period was Antananarivo, Madagascar with an uncertainty reduction of over 17%. This station did not appear in the solution for any other period ().
Fig. 9. Optimal locations to situate new atmospheric monitoring sites to an existing network to reduce the overall uncertainty of N2O fluxes from terrestrial Africa for the periods (a) January to March, (b) April to June, (c) July to August, and (d) September to December 2012. Sites are coloured according to the rank in the optimal design, with light green sites representing the site with the largest uncertainty reduction and which is the first site added to the network.

Kisangani, although it never achieved the top uncertainty reduction, was in the optimal network for all four periods. Ngaoundere, Cameroon; Dundo, Angola; and Am-Timan, Chad, were listed in three of the four optimal solutions.
The maximum uncertainty reduction was 37.1% achieved for the January-March period – Southern Hemisphere summer – followed by 35.6% achieved for the July-September period – Northern Hemisphere summer. The uncertainty reduction for October-December period reached 18.2%, but only 10.1% for the April-June period.
The solution was largely dictated by the prior estimates of the natural soil fluxes (see supplementary material). The anthropogenic emissions per pixel were much smaller compared to those from natural soils, and showed relatively little seasonality.
3.5. Multi-species network design
The result of the multi-species optimal network design using approach 1, where sites were selected from the individual network design solutions, is presented in and the uncertainty reductions achieved for each GHG and season are given in . The spatial location of sites in the solution was expected, given the individual network design solutions, with most sites concentrated between 10ºN and 20ºS, with the most extreme northerly site located in Sudan and the most extreme southerly site in Botswana. This network provided good coverage across highly productive regions, in terms of biogenic fluxes, during all parts of the year ().
Fig. 10. Optimal locations to situate new atmospheric monitoring sites to an existing network to reduce the overall uncertainty of CO2, CH4, and N2O fluxes from terrestrial Africa, where 2012 has been taken as a representative year, obtained using the solutions from the individual gas network designs. Sites are coloured according to the order in which they were added to the solution, where sites were first selected from the CO2 solution, followed by CH4, and N2O.

Table 5. Uncertainty reductions achieved by the combined network solution aimed at reducing the uncertainty in fluxes across CO2, CH4 and N2O fluxes and across both Northern and Southern Hemisphere summers for approach 1, which uses the separate network design solutions (12 sites), and approach 2, which optimises over all three gases simultaneously (10 sites and 12 sites).
The optimised network solution using approach 1, with twelve stations, achieved lower uncertainty reductions per species compared with the individual network solutions with ten stations, which were optimised for a particular GHG and season. For CO2 the highest uncertainty reduction was achieved for the period January to March at 47.8% compared with the 54.4% from the individual solution. The difference was more extreme during the Northern Hemisphere summer period, where the uncertainty reduction achieved was only 40.4% compared with the 51.8% of the individual solution. Similar losses in uncertainty reduction were obtained for CH4 and N2O.
Using the second optimisation approach (), solving across all three gases simultaneously, gave a very similar spatial distribution of optimal sites, sharing six of the twelve stations, and with the remaining stations being in similar locations. Maun, Botswana, and Kassala, Sudan, came out as top sites in both approaches. The sites from the second approach were slightly more spread out, with the most extreme northerly site in Mauritania, and most southerly in South Africa.
Fig. 11. Optimal locations to situate new atmospheric monitoring sites to an existing network to reduce the overall uncertainty of CO2, CH4, and N2O fluxes from terrestrial Africa, where 2012 has been taken as a representative year, following approach 2, which optimised over the three gases simultaneously. Sites are coloured according to the rank in the optimal design, with light green sites representing the site with the largest uncertainty reduction and which is the first site added to the network.

The uncertainty reductions achieved across the different gases and seasons were similar to the first approach. One notable difference is the much poorer uncertainty reduction achieved by the second approach, even with twelve sites, during the January to March period for N2O. This is due to the same weighting assigned to a unit percentage uncertainty reduction for any gas. The network was able to achieve uncertainty reduction for CO2 much more easily than for N2O, and so the solution for the second approach was dominated by uncertainty reduction in CO2.
The combined solution demonstrates that significant compromises are needed if one observation network is required for all three GHGs. The uncertainty reduction requirement for each gas needs to be reduced, or the number of towers installed increased (). Increasing the number of sites to as many as thirty would still see the average uncertainty reduction across the three gases stay below 50%.
4. Discussion
4.1. Individual GHGs
The network design results show that to reduce uncertainties in total African GHG emissions, an observation network would ideally be dynamic to allow sampling over different high-productivity regions dependent on the season. For a tall-tower network the sites are fixed, so this is not feasible, and so compromises will need to be made in the uncertainty reduction achievable in each season by the final network design.
CO2 emission uncertainty, which we assume to scale with net primary productivity, was very strongly concentrated between between 10ºN and 25ºS. This region contains the tropical rain forests and Miombo woodlands. The tropical rainforest activity dominates during the Northern Hemisphere summer, whereas the Miombo woodland and savanna ecosystems are most active during the Southern Hemisphere summer.
Anthropogenic emissions of CO2 are concentrated in a few regions in Africa, particularly in South Africa. In a previous network design study for South Africa (Nickless et al., Citation2015), the network design favoured sites closer to these anthropogenic sources during the winter period, when the majority of the natural regions in South Africa were less productive. For the entire African region there is no period when all natural productivity is low, and therefore the uncertainty in these biogenic fluxes drives the location of sites to reduce the overall uncertainty. North Africa, which has relatively low biogenic productivity, has regions with large anthropogenic emissions, but the uncertainty in these emissions compared with those from biogenic fluxes elsewhere in Africa is small, and therefore no sites are located here for any of the periods.
Compared to CO2 and N2O, there is much less seasonality in the spatial distribution and size of prior CH4 fluxes. This was translated into the network solutions for CH4, where the same sites appeared in the network solution across different periods. Unlike CO2 and N2O, uncertainty in anthropogenic emissions of CH4 made up a larger proportion of the overall uncertainty than biogenic fluxes. These characteristics suggest that there is some justification for having a separate observation network for CH4.
The uncertainty in N2O fluxes is driven by the natural soil fluxes, largely driven my climatic effects and biogenic productivity on soil microbial activity, which show strong seasonality. These fluxes, according to the Saikawa et al. (Citation2013) inventory, are strongly seasonal with different hotspots in each season, such as one seen over Madagascar during the January-March period. These areas of highly concentrated fluxes of N2O coincide with the soil fluxes, which are correlated with climatic variables like soil temperature and moisture. The N2O hotspot in Madagascar led to the selection of the Antananarivo site as the top uncertainty reduction location during this period. Similar hotspots for each period dictated the optimal solution, leading to optimal networks that differed between seasons more so than for CO2 or CH4.
4.2. Multi-species network design
We used two separate approaches to solve for a multi-species observation network. These provided network solutions with some differences. These differences are due to different priorities of the optimisation approaches. The first approach aimed to ensure that all three species had substantial uncertainty reductions across the whole year. Therefore, the top four sites for each GHG were selected, which provided the bulk of the uncertainty reduction for the individual GHG solutions. The second approach weighted a unit of percentage uncertainty reduction the same across all three species and aimed to maximise the sum of these percentage uncertainties. The individual GHG solutions showed that the percentage uncertainty reduction achieved for CO2 was much higher compared to the other two GHGs. Therefore, as the sum of the percentage uncertainty reduction was dominated by CO2, this led to a network that covered as much of the high CO2 activity regions as possible, and therefore the network solution was dominated by uncertainty reduction in the CO2 fluxes.
Ignoring the nuances of where sites are to be placed, the solutions for the optimal network across different seasons and different GHGs clearly show that new tall-tower stations in Africa should be concentrated between 10ºN and 25ºS. If all the new sites are concentrated here, concerns may be raised about observation of areas outside this region. For example, new sources of emissions may be identified which are not included in the current prior information. Fortunately, satellite observation by missions, such as GOSAT, have good coverage of these regions (). Most of North Africa and southern Africa are well viewed by GOSAT () (Wang et al., Citation2018), whereas the areas identified as best locations for a tall-tower network are poorly viewed due to cloud interference (but still better compared with many parts of Europe, for example).
Fig. 13. Number of valid retrievals from the GOSAT satellite product (https://earth.esa.int/web/guest/ missions/3rd-party-missions/current-missions/gosat) for CO2 for the year 2012.

Large uncertainties in GHG emissions from wetlands in tropical Africa have been identified (Lunt et al., Citation2019). It is estimated that the CH4 emissions from African wetlands makes up between 7 to 23% of global wetland emissions estimated from an ensemble of models, indicating the large degree of uncertainty in this component of the African CH4 budget (Bloom et al., Citation2017). Lunt et al. (Citation2019) showed that the emissions from the Sudd wetlands in south Sudan increased by 3 Tg yr−1 during the period 2010 to 2016, but this was found to be transient, and isotopic measurements have indicated that the budget was moving towards dominance by microbial activity during this period (Nisbet et al., Citation2016; Lunt et al., Citation2019). The network design identifies sites near these regions, such as Kassala, Sennar and En Nahud, which could play an important role in identifying specific high-emission wetland locations and reducing the uncertainty in these emissions.
4.3. Limitations and future work
This study used an uncertainty-minimisation focused approach to solving for an optimal network, and although attempts were made to include sites that would be less costly, the total cost of establishing and maintaining a site at a particular location was not explicitly factored into the optimisation. The considerations of what a new site would cost in general terms can be easily obtained (ICOS ERIC, Citation2020), but the site-specific costs will be highly variable among sites and over time (e.g. due to foreign exchange variability), and will depend on many factors (López-Ballesteros et al., Citation2018). Approaches are available for optimising on both uncertainty reduction and cost reduction simultaneously (Kaminski and Rayner, Citation2017). However, we propose that the incremental optimisation approach used here could now be used together with a thorough economic analysis of a much smaller subset of sites, to determine what the most cost effective network will look like.
Running atmospheric dispersion models, like FLEXPART, for multiple sites can take a large amount of computing resources and time. To make this problem manageable, one year was chosen for the network design as the typical synoptic patterns are expected to be similar from year to year. Although El Ninõ and La Ninã events can influence interannual variability in climate and whether a region is a carbon source or sink (Abdi et al., Citation2016), this study was concerned with the uncertainty and not the absolute estimates. The uncertainties related to the variability in the biogenic fluxes from year to year were incorporated into the uncertainty estimates. The year 2012 was a moderate La Ninã year, and therefore expected to represent average, rather than extreme, climatic conditions. The optimal network solution was determined by the aggregated sensitivity of sites to the largest sources, rather than by single synoptic events.
The assumption used here, and in many atmospheric inversion studies, was that uncertainty scales with the size of the flux. This assumption follows when proportional uncertainty is applied to the fluxes, which is often the case for atmospheric inversion studies, and this means that as the absolute value of a flux increases, so will the uncertainty value. Therefore, because regions with higher productivity have larger fluxes, it was assumed that more productive systems are more uncertain systems. Although this is representative of current uncertainty estimates of biogenic fluxes, it would be good to distinguish the uncertainty estimates for well known large sources from poorly known large sources. This is more relevant to Boreal systems, which generally have more detailed inventory data available than systems in Africa. Remotely sensed products could potentially be used to provide information about spatial and temporal changes in coverage and solar-induced chlorophyll fluorescence (SIF), which is related to the net biogenic CO2 flux, could be used to refine uncertainty estimates as well.
We have discussed the use of satellite observations as part of an integrated network of GHG observatories. This study provides some evidence that tall-tower network and satellite measurements could complement each other. Further analysis is required in order to establish the joint constraint on estimates of GHG sources and sinks that could be achieved with the combination of satellite and in situ observations. Further study is also required on where towers could be placed to aid in validation of flux estimates from satellite observations (e.g. from a particular point source) and atmospheric mole fraction estimates from satellite products (most likely at sites in arid regions, which would not provide much constraint of total GHG budgets). These are two very different objectives, and would likely require completely separate sets of measurements to perform these two types of validation.
LPJ models net primary productivity based on plant functional types. But it does not have information on the exact timing of land use and land use changes which occurred during the study period. There is not a great deal of information available on the rate of response or timing of photosynthesis or net ecosystem exchange after a disturbance, such as clearcut deforestation or fire, and these events are not explicitly included as part of the available information when modelling net primary productivity. These types of disturbances can cause a region to move from a carbon sink to a carbon source. Lack of information on land use change in earth system models is listed as one of the major contributors to model-data disagreement (McGuire et al., Citation2001; Wu et al., Citation2019). This network design has attempted to incorporate some of the uncertainty attributed to biomass burning into the design of the study, but little information is available on other disturbances, such as land use change, for Africa. In order for atmospheric observations of GHGs to be used to inform on emissions from disturbances through inverse modelling, a way needs to be developed to explicitly incorporate information about land use change into the prior information of the inversion.
5. Conclusions
An optimal network design solution for the observation of GHGs by tall towers is dominated by sites in the mid tropics, particularly requiring sites in countries like Angola, the DRC, and Botswana, which have high levels of vegetation cover. Networks aimed at reducing uncertainties for a targeted GHG are substantively different when compared between CO2, CH4 and N2O, leading to diminished uncertainty reductions for the non-targeted GHGs. Approaches that aimed to solve across all three gases were able to find reasonable solutions that could reduce uncertainty across the board in a commensurate way.
The largest reduction in uncertainty for a single GHG was achieved for total African CO2 fluxes, at 54.4%. Therefore, even at the best estimate, more uncertainty reduction is required through integration of tall-tower network observations with other types of GHG observations, and improvements in inventories and process models. Adding more and more sites to the network is not sufficient to achieve uncertainties in GHG emissions across all of Africa that reaches the desired level of 15%, so that uncertainties can be comparable to those required for reporting of anthropogenic emissions. In parallel to enhancing measurement capacity, improved prior information is be required. In particular, the size of prior biogenic flux uncertainties need to be reduced and decoupled from the size of the flux.
We provide a plan for where GHG atmospheric monitoring sites should be prioritised in Africa. A roll-out of this network, fully or partially, would contribute towards a reduction in the uncertainty of emissions over Africa and improve GHG budgets at global and continental scales.
Data and code availability
The prior flux products used in this study are publicly available through the citations to the original publications. The FLEXPART model is available through https://flexpart.eu. The LPJ model is available through https://web.archive.org/web/20101213071217/http://www.pik-potsdam.de/research/cooperations/lpjweb. The sensitivity matrices, prior flux uncertainties and model-measurement errors used to calculate the overall uncertainty reduction for adding a new site to the observation network are provide at https://doi.org/10.17605/OSF.IO/K7PR2 (Nickless et al., Citation2019a).
Acknowledgements
The authors would like to thank Alistair Manning for useful tea-time discussions on biomass burning emissions.
Disclosure statement
The authors declare that no competing interests are present.
Supplemental data
Supplemental data for this article can be accessed here.
Additional information
Funding
Notes on contributors
Alecia Nickless
AN wrote and implemented the code for calculating the posterior covariance matrices and performing the incremental optimisation procedure in Python, reshaped and processed the prior fluxes in Python and R, produced all figures using R, interpreted the results, and was responsible for the development of the manuscript. All co-authors contributed towards and reviewed the manuscript. RJS conceived the original concept and interpreted results. AV produced the FLEXPART footprints. JB and AB contributed to site identification and creation of the candidate site list. JA provided the LPJ output. UK, VK, and KP contributed to the FLEXPART runs and data management. MR processed the CH4 and N2O emission datasets and contributed towards interpretation of the results. VJ contributed towards project management. WK contributed towards the initial concept and interpretation of the results.
Robert J. Scholes
AN wrote and implemented the code for calculating the posterior covariance matrices and performing the incremental optimisation procedure in Python, reshaped and processed the prior fluxes in Python and R, produced all figures using R, interpreted the results, and was responsible for the development of the manuscript. All co-authors contributed towards and reviewed the manuscript. RJS conceived the original concept and interpreted results. AV produced the FLEXPART footprints. JB and AB contributed to site identification and creation of the candidate site list. JA provided the LPJ output. UK, VK, and KP contributed to the FLEXPART runs and data management. MR processed the CH4 and N2O emission datasets and contributed towards interpretation of the results. VJ contributed towards project management. WK contributed towards the initial concept and interpretation of the results.
Alex Vermeulen
AN wrote and implemented the code for calculating the posterior covariance matrices and performing the incremental optimisation procedure in Python, reshaped and processed the prior fluxes in Python and R, produced all figures using R, interpreted the results, and was responsible for the development of the manuscript. All co-authors contributed towards and reviewed the manuscript. RJS conceived the original concept and interpreted results. AV produced the FLEXPART footprints. JB and AB contributed to site identification and creation of the candidate site list. JA provided the LPJ output. UK, VK, and KP contributed to the FLEXPART runs and data management. MR processed the CH4 and N2O emission datasets and contributed towards interpretation of the results. VJ contributed towards project management. WK contributed towards the initial concept and interpretation of the results.
Johannes Beck
AN wrote and implemented the code for calculating the posterior covariance matrices and performing the incremental optimisation procedure in Python, reshaped and processed the prior fluxes in Python and R, produced all figures using R, interpreted the results, and was responsible for the development of the manuscript. All co-authors contributed towards and reviewed the manuscript. RJS conceived the original concept and interpreted results. AV produced the FLEXPART footprints. JB and AB contributed to site identification and creation of the candidate site list. JA provided the LPJ output. UK, VK, and KP contributed to the FLEXPART runs and data management. MR processed the CH4 and N2O emission datasets and contributed towards interpretation of the results. VJ contributed towards project management. WK contributed towards the initial concept and interpretation of the results.
Ana López-Ballesteros
AN wrote and implemented the code for calculating the posterior covariance matrices and performing the incremental optimisation procedure in Python, reshaped and processed the prior fluxes in Python and R, produced all figures using R, interpreted the results, and was responsible for the development of the manuscript. All co-authors contributed towards and reviewed the manuscript. RJS conceived the original concept and interpreted results. AV produced the FLEXPART footprints. JB and AB contributed to site identification and creation of the candidate site list. JA provided the LPJ output. UK, VK, and KP contributed to the FLEXPART runs and data management. MR processed the CH4 and N2O emission datasets and contributed towards interpretation of the results. VJ contributed towards project management. WK contributed towards the initial concept and interpretation of the results.
Jonas Ardö
AN wrote and implemented the code for calculating the posterior covariance matrices and performing the incremental optimisation procedure in Python, reshaped and processed the prior fluxes in Python and R, produced all figures using R, interpreted the results, and was responsible for the development of the manuscript. All co-authors contributed towards and reviewed the manuscript. RJS conceived the original concept and interpreted results. AV produced the FLEXPART footprints. JB and AB contributed to site identification and creation of the candidate site list. JA provided the LPJ output. UK, VK, and KP contributed to the FLEXPART runs and data management. MR processed the CH4 and N2O emission datasets and contributed towards interpretation of the results. VJ contributed towards project management. WK contributed towards the initial concept and interpretation of the results.
Ute Karstens
AN wrote and implemented the code for calculating the posterior covariance matrices and performing the incremental optimisation procedure in Python, reshaped and processed the prior fluxes in Python and R, produced all figures using R, interpreted the results, and was responsible for the development of the manuscript. All co-authors contributed towards and reviewed the manuscript. RJS conceived the original concept and interpreted results. AV produced the FLEXPART footprints. JB and AB contributed to site identification and creation of the candidate site list. JA provided the LPJ output. UK, VK, and KP contributed to the FLEXPART runs and data management. MR processed the CH4 and N2O emission datasets and contributed towards interpretation of the results. VJ contributed towards project management. WK contributed towards the initial concept and interpretation of the results.
Matthew Rigby
AN wrote and implemented the code for calculating the posterior covariance matrices and performing the incremental optimisation procedure in Python, reshaped and processed the prior fluxes in Python and R, produced all figures using R, interpreted the results, and was responsible for the development of the manuscript. All co-authors contributed towards and reviewed the manuscript. RJS conceived the original concept and interpreted results. AV produced the FLEXPART footprints. JB and AB contributed to site identification and creation of the candidate site list. JA provided the LPJ output. UK, VK, and KP contributed to the FLEXPART runs and data management. MR processed the CH4 and N2O emission datasets and contributed towards interpretation of the results. VJ contributed towards project management. WK contributed towards the initial concept and interpretation of the results.
Ville Kasurinen
AN wrote and implemented the code for calculating the posterior covariance matrices and performing the incremental optimisation procedure in Python, reshaped and processed the prior fluxes in Python and R, produced all figures using R, interpreted the results, and was responsible for the development of the manuscript. All co-authors contributed towards and reviewed the manuscript. RJS conceived the original concept and interpreted results. AV produced the FLEXPART footprints. JB and AB contributed to site identification and creation of the candidate site list. JA provided the LPJ output. UK, VK, and KP contributed to the FLEXPART runs and data management. MR processed the CH4 and N2O emission datasets and contributed towards interpretation of the results. VJ contributed towards project management. WK contributed towards the initial concept and interpretation of the results.
Karolina Pantazatou
AN wrote and implemented the code for calculating the posterior covariance matrices and performing the incremental optimisation procedure in Python, reshaped and processed the prior fluxes in Python and R, produced all figures using R, interpreted the results, and was responsible for the development of the manuscript. All co-authors contributed towards and reviewed the manuscript. RJS conceived the original concept and interpreted results. AV produced the FLEXPART footprints. JB and AB contributed to site identification and creation of the candidate site list. JA provided the LPJ output. UK, VK, and KP contributed to the FLEXPART runs and data management. MR processed the CH4 and N2O emission datasets and contributed towards interpretation of the results. VJ contributed towards project management. WK contributed towards the initial concept and interpretation of the results.
Veronika Jorch
AN wrote and implemented the code for calculating the posterior covariance matrices and performing the incremental optimisation procedure in Python, reshaped and processed the prior fluxes in Python and R, produced all figures using R, interpreted the results, and was responsible for the development of the manuscript. All co-authors contributed towards and reviewed the manuscript. RJS conceived the original concept and interpreted results. AV produced the FLEXPART footprints. JB and AB contributed to site identification and creation of the candidate site list. JA provided the LPJ output. UK, VK, and KP contributed to the FLEXPART runs and data management. MR processed the CH4 and N2O emission datasets and contributed towards interpretation of the results. VJ contributed towards project management. WK contributed towards the initial concept and interpretation of the results.
Werner Kutsch
AN wrote and implemented the code for calculating the posterior covariance matrices and performing the incremental optimisation procedure in Python, reshaped and processed the prior fluxes in Python and R, produced all figures using R, interpreted the results, and was responsible for the development of the manuscript. All co-authors contributed towards and reviewed the manuscript. RJS conceived the original concept and interpreted results. AV produced the FLEXPART footprints. JB and AB contributed to site identification and creation of the candidate site list. JA provided the LPJ output. UK, VK, and KP contributed to the FLEXPART runs and data management. MR processed the CH4 and N2O emission datasets and contributed towards interpretation of the results. VJ contributed towards project management. WK contributed towards the initial concept and interpretation of the results.
References
- Abdi, A. M., Vrieling, A., Yengoh, G. T., Anyamba, A., Seaquist, J. W. and co-authors. 2016. The El Niño - La Niña cycle and recent trends in supple and demand of net primary productivity in African drylands. Clim. Change 138, 111–125. doi:10.1007/s10584-016-1730-1
- Aubinet, M. 2000. Estimates of the annual net carbon and water exchange of forests: the EUROFLUX methodology. Adv. Ecol. Res. 30, 113–175.
- Baker, D. F., Law, R. M., Gurney, K. R., Rayner, P., Peylin, P. and co-authors. 2006. TransCom 3 inversion intercomparison: impact of transport model errors on the interannual variability of regional CO2 fluxes, 1988. Global Biogeochem. Cy. 20, 2003.
- Beck, J., López-Ballesteros, A., Hugo, W., Scholes, R., Saunders, M. and co-authors. 2019. Development of a climate forcing observation system for Africa: data-related considerations. Codata. 18, 42. doi:10.5334/dsj-2019-042
- Bergamaschi, P., Corazza, M., Karstens, U., Athanassiadou, M., Thompson, R. L. and co-authors. 2015. Top-down estimates of European CH4 and N2O emissions based on four different inverse models. Atmos. Chem. Phys. 15, 715–736. doi:10.5194/acp-15-715-2015
- Bloom, A. A., Bowman, K. W., Lee, M., Turner, A. J., Schroeder, R. and co-authors. 2017. A global wetland methane emissions and uncertainty dataset for atmospheric chemical transport models (WetCHARTs version 1.0). Geosci. Model Dev. 10, 2141–2156. doi:10.5194/gmd-10-2141-2017
- Bloom, A. A., Palmer, P. I., Fraser, A. and Reay, D. S. 2012. Seasonal variability of tropical wetland CH4 emissions: the role of the methanogen-available carbon pool. Biogeosciences 9, 2821–2830. doi:10.5194/bg-9-2821-2012
- Boden, T. A., Marland, G. and Andres, R. J. 2017. Global, regional, and national fossil – fuel CO2 emissions, Carbon Dioxide Information Analysis Center (CDIAC). Oak Ridge National Laboratory, US Department of Energy, Oak Ridge, TN, USA. Online at: http://cdiac.essdive.lbl.gov/trends/emis/methreg.html V2017.
- Borges, A. V., Darchambeau, F., Teodoru, C. R., Marwick, T. R., Tamooh, F. and co-authors. 2015. Globally significant greenhouse-gas emissions from African inland waters. Nat. Geosci. 8, 637–642. doi:10.1038/ngeo2486
- Bousquet, P., Ciais, P., Peylin, P., Ramonet, M. and Monfray, P. 1999. Inverse modeling of annual atmospheric CO2 sources and sinks: 1. Method and control inversion. J. Geophys. Res. 104, 26161–26178. doi:10.1029/1999JD900342
- Broquet, G., Chevallier, F., Bréon, F.-M., Kadygrov, N., Alemanno, M. and co-authors. 2013. Regional inversion of CO2 ecosystem fluxes from atmospheric measurements: reliability of the uncertainty estimates. Atmos. Chem. Phys. 13, 9039–9056. doi:10.5194/acp-13-9039-2013
- Carpenter, L. J., Fleming, Z. L., Read, K. A., Lee, J. D., Moller, S. J. and co-authors. 2010. Seasonal characteristics of tropical marine boundary layer air measured at the Cape Verde atmospheric observatory. J. Atmos. Chem. 67, 87–140. doi:10.1007/s10874-011-9206-1
- Cerutti, P. O., Sola, P., Chenevoy, A., Iiyama, M., Yila, J. and co-authors. 2015. The socioeconomic and environmental impacts of wood energy value chains in Sub-Saharan Africa: a systematic map protocol. Environ. Evid. 4, 12. doi:10.1186/s13750-015-0038-3
- Chevallier, F., Ciais, P., Conway, T. J., Aalto, T., Anderson, B. E. and co-authors. 2010. CO2 surface fluxes at grid point scale estimated from a global 21 year reanalysis of atmospheric measurements. J. Geophys. Res. 115, D21307. doi:10.1029/2010JD013887
- Chevallier, F., Palmer, P. I., Feng, L., Boesch, H., O'Dell, C. W. and co-authors. 2014. Toward robust and consistent regional CO2 flux estimates from in situ and spaceborne measurements of atmospheric CO2. Geophys. Res. Lett. 41, 1065–1070. doi:10.1002/2013GL058772
- Ciais, P., Rayner, P., Chevallier, F., Bousquet, P., Logan, M. and co-authors. 2010. Atmospheric inversions for estimating CO2 fluxes: methods and perspectives. Clim. Change 103, 69–92. doi:10.1007/s10584-010-9909-3
- Enting, I. G. 2002. Inverse Problems in Atmospheric Constituent Transport. Cambridge University Press, New York.
- Enting, I. G. and Mansbridge, J. V. 1989. Seasonal sources and sinks of atmospheric CO2: direct inversion of filtered data. Tellus B 41, 111–126. doi:10.3402/tellusb.v41i2.15056
- Etminan, M., Myhre, G., Highwood, E. J. and Shine, K. P. 2016. Radiative forcing of carbon dioxide, methane, and nitrous oxide: a significant revision of the methan radiative forcing. Geophys. Res. Lett. 43, 12614–12623. doi:10.1002/2016GL071930
- Exbrayat, J.-F., Pitman, A. J., Abramowitz, G. and Wang, Y.-P. 2013. Sensitivity of net ecosystem exchange and heterotrophic respiration to parameterization uncertainty. J. Geophys. Res. Atmos. 118, 1640–1651. doi:10.1029/2012JD018122
- Fung, I., John, J., Lerner, J., Matthews, E., Prather, M. and co-authors. 1991. Three-dimensional model synthesis of the global methane cycle. J. Geophys. Res. 96, 13033–13065. doi:10.1029/91JD01247
- Ganesan, A. L., Manning, A. J., Grant, A., Young, D., Oram, D. E. and co-authors. 2015. Quantifying methane and nitrous oxide emissions from the UK and Ireland using a national-scale monitoring network. Atmos. Chem. Phys. 15, 6393–6406. doi:10.5194/acp-15-6393-2015
- Gaudry, A., Monfray, P., Polian, G., Bonsang, G., Ardouin, B. and co-authors. 1991. Non-seasonal variations of atmospheric CO2 concentrations at Amsterdam Island. Tellus B 43, 136–143. doi:10.3402/tellusb.v43i2.15258
- Gomez-Pelaez, A. J., Ramos, R., Cuevas, E., Gomez-Trueba, V. and Reyes, E. 2019. Atmospheric CO2, CH4, and CO with the CRDS technique at the Izanã Global GAW station: instrumental tests, developments, and first measurement results. Atmos. Meas. Tech. 12, 2043–2066. doi:10.5194/amt-12-2043-2019
- Gurney, K. R., Law, R. M., Denning, A. S., Rayner, P. J., Baker, D. and co-authors. 2002. Towards robust regional estimates of CO2 sources and sinks using atmospheric transport models. Nature 405, 626–630.
- Gurney, K. R., Law, R. M., Denning, A. S., Rayner, P. J., Baker, D. and co-authors. 2003. TransCom 3 CO2 inversion intercomparison: 1. Annual mean control results and sensitivity to transport and prior flux information. Tellus B 55, 555–579. doi:10.3402/tellusb.v55i2.16728
- Haszpra, L., Barcza, Z., Haszpra, T., Pátkai, Z. and Davis, K. J. 2015. How well do tall-tower measurements characterize the CO2 mole fraction distribution in the planetary boundary layer? Atmos. Meas. Tech. 8, 1657–1671. doi:10.5194/amt-8-1657-2015
- Howarth, R. W. 2019. Ideas and perspectives: is shale gas a major driver of recent increase in global atmospheric methane? Biogeosciences 16, 3033–3046. doi:10.5194/bg-16-3033-2019
- ICOS ERIC. 2020. ICOS Handbook (K. Ahlgren & M. Keski-Nisula (eds.); 2020th–2nd ed. ICOS ERIC, Helsinki.
- Janssens-Maenhout, G., Pagliari, V., Guizzardi, D. and Muntean, M. 2012. Global Emission Inventories in the Emission Database for Global Atmospheric Research (EDGAR) — Manual (I), Gridding: EDGAR Emissions Distribution on Global Gridmaps. Luxembourg: European Union, Joint Research Centre, 33 pp.
- Kaminski, T., Heimann, M. and Giering, R. 1999. A coarse grid three dimensional global inverse model of the atmospheric transport, 2. Inversion of the transport of CO2 in the 1980s. J. Geophys. Res. 104, 18555–18581. doi:10.1029/1999JD900146
- Kaminski, T. and Rayner, P. J. 2017. Reviews and syntheses: guiding the evolution of the observing system for the carbon cycle through quantitative network design. Biogeosciences 14, 4755–4766. doi:10.5194/bg-14-4755-2017
- Kong, Y., Chen, B. and Measho, S. 2019. Spatio-temporal consistency evaluation of XCO2 retrievals from GOSAT and OCO-2 based on TCCON and model data for joint utilization in carbon cycle research. Atmosphere 10, 354. doi:10.3390/atmos10070354
- Kuppel, S., Peylin, P., Chevallier, F., Bacour, C., Maignan, F. and co-authors. 2012. Constraining a global ecosystem model with multisite eddy-covariance data. Biogeosciences 9, 3757–3776. doi:10.5194/bg-9-3757-2012
- Labuschagne, C., Kuyper, B., Brunke, E.-G., Mokolo, T., van der Spuy, D. and co-authors. 2018. A review of four decades of atmospheric trace gas measurements at Cape Point, South Africa. Trans. R. Soc. S. Afr. 73, 113–132. doi:10.1080/0035919X.2018.1477854
- Lauvaux, T., Miles, N. L., Deng, A., Richardson, S. J., Cambaliza, M. O. and co-authors. 2016. High-resolution atmospheric inversion of urban CO2 emissions during the dormant season of the Indianapolis Flux Experiment (INFLUX). J. Geophys. Res. Atmos. 121, 5213–5236. doi:10.1002/2015JD024473
- Lauvaux, T., Schuh, A. E., Uliasz, M., Richardson, S., Miles, N. and co-authors. 2012. Constraining the CO2 budget of the corn belt: exploring uncertainties from the assumptions in a mesoscale inverse system. Atmos. Chem. Phys. 12, 337–354. doi:10.5194/acp-12-337-2012
- Law, R. M., Chen, Y., Gurney, K. R. and Transcom 3 Modellers. 2003. TransCom 3 CO2 inversion intercomparison: 2. Sensitivity of annual mean results to data choices. Tellus B 55, 580–595. doi:10.3402/tellusb.v55i2.16736
- Le Quéré, C., Andrew, R. M., Friedlingstein, P., Sitch, S., Hauck, J. and co-authors. 2018. Global carbon budget 2018. Earth Syst. Sci. Data 10, 2141–2194. doi:10.5194/essd-10-2141-2018
- Leip, A., Skiba, U., Vermeulen, A. and Thompson, R. 2018. A complete rethink is needed on how greenhouse gas emissions are quantified for national reporting. Atmos. Environ. 174, 237–240. doi:10.1016/j.atmosenv.2017.12.006
- Li, W., Ciais, P., Peng, S., Yue, C., Wang, Y. and co-authors. 2017. Landuse and land-cover change carbon emissions between 1901 and 2012 constrained by biomass observations. Biogeosciences 14, 5053–5067. doi:10.5194/bg-14-5053-2017
- López-Ballesteros, A., Beck, J., Bombelli, A., Grieco, E., Lorencová, E. K. and co-authors. 2018. Towards a feasible and representative pan-African Research Infrastructure network for GHG observations. Environ. Res. Lett. 13, 085003. doi:10.1088/1748-9326/aad66c
- Lopez-Coto, I., Ghosh, S., Prasad, K. and Whetstone, J. 2017. Tower-based greenhouse gas measurement network design—The National Institute of Standards and Technology North East Corridor Testbed. Adv. Atmos. Sci. 34, 1095–1105. doi:10.1007/s00376-017-6094-6
- Lunt, M. F., Palmer, P. I., Feng, L., Taylor, C. M., Boesch, H. and co-authors. 2019. An increase in methane emissions from tropical Africa between 2010 and 2016 inferred from satellite data. Atmos. Chem. Phys. 19, 14721–14740. doi:10.5194/acp-19-14721-2019
- McGuire, A. D., Sitch, S., Clein, J. S., Dargaville, R., Esser, G. and co-authors. 2001. Carbon balance of the terrestrial biosphere in the twentieth century: analyses of CO2, climate and land use effects with four process-based ecosystem models. Global Biogeochem. Cy. 15, 183–206. doi:10.1029/2000GB001298
- Morgan, E. J., Lavrič, J. V., Seifert, T., Chicoine, T., Day, A. and co-authors. 2015. Continuous measurements of greenhouse gases and atmospheric oxygen at the Namib Desert Atmospheric Observatory. Atmos. Meas. Tech. 8, 2233–2250. doi:10.5194/amt-8-2233-2015
- Myhre, G., Shindell, D., Bréon, F.-M., Collins, W., Fuglestvedt, J. and co-authors 2013. Anthropogenic and natural radiative forcing, in climate change 2013: the physical science basis. In: Contribution of Working Group I to the Fifth Assessment Report of the Intergovernmental Panel on Climate Change (eds. T. F. Stocker, D. Qin, G.-K. Plattner, M. Tignor, S. K. Allen, J. Doschung, A. Nauels, Y. Xia, V. Bex, and P. M. Midgley). Cambridge University Press, Cambridge University Press, Cambridge, United Kingdom and New York, NY, USA, pp. 659–740.
- Nathan, B. J., Lauvaux, T., Turnbull, J. C., Richardson, S. J., Niles, N. L. and co-authors. 2018. Source sector attribution of CO2 emissions using an urban CO/CO2 Bayesian inversion system. J. Geophys. Res. Atmos. 123, 13611–13621.
- Nickless, A., Rayner, P. J., Engelbrecht, F., Brunke, E.-G., Erni, B. and co-authors. 2018a. Estimates of CO2 fluxes over the city of Cape Town, South Africa, through Bayesian inverse modelling. Atmos. Chem. Phys. 18, 4765–4801. doi:10.5194/acp-18-4765-2018
- Nickless, A., Rayner, P. J., Erni, B. and Scholes, R. J. 2018b. Comparison of the genetic algorithm and incremental optimisation routines for a Bayesian inverse modelling based network design. Inverse Prob. 34, 055006. doi:10.1088/1361-6420/aab46c
- Nickless, A., Rayner, P. J., Scholes, R. J., Engelbrecht, F. and Erni, B. 2019b. An atmospheric inversion over the city of Cape Town: sensitivity analyses. Atmos. Chem. Phys. 19, 7789–7816. doi:10.5194/acp-19-7789-2019
- Nickless, A., Scholes, R. J., Vermeulen, A., Beck, J., Lopez´-Ballesteros, A. and co-authors. 2019a. Greenhouse gas observation network design for Africa. OSF. doi:https://doi.org/10.17605/OSF.
- Nickless, A., Ziehn, T., Rayner, P. J., Scholes, R. J. and Engelbrecht, F. 2015. Greenhouse gas network design using backward Lagrangian particle dispersion modelling – Part 2: sensitivity analyses and South African test case. Atmos. Chem. Phys. 15, 2051–2069. doi:10.5194/acp-15-2051-2015
- Nisbet, E. G., Dlugokencky, E. J., Manning, M. R., Lowry, D., Fisher, R. E. and co-authors. 2016. Rising atmospheric methane: 2007–2014 growth and isotopic shift. Global Biogeochem. Cy. 30, 1356–1370. doi:10.1002/2016GB005406
- Nisbet, E. G., Manning, M. R., Dlugokencky, E. J., Fisher, R. E., Lowry, D. and co-authors. 2019. Very strong atmospheric methane growth in the 4 years 2014–2017: Implications for the Paris Agreement. Global Biogeochem. Cy. 33, 318–342. doi:10.1029/2018GB006009
- Northrop, E., Dagnet, Y., Höhne, N., Thwaites, J. and Mogelgaard, K. 2018. Achieving the ambition of Paris: designing the global stocktake. Working Paper. Project for Advancing Climate Transparency (PACT), Washington, DC. Online at: http://www.wri.org/publication/pact-global-stocktakedesign
- Oda, T., Lauvaux, T., Lu, D., Rao, P., Miles, N. L. and co-authors. 2017. On the impact of granularity of space-based urban CO2 emissions in urban atmospheric inversions: a case study for Indianapolis, IN. Elementa 5, 28.
- Oda, T. and Maksyutov, S. 2011. A very high-resolution (1 km × 1 km) global fossil fuel CO2 emission inventory derived using a point source database and satellite observations of nighttime lights. Atmos. Chem. Phys. 11, 543–556. doi:10.5194/acp-11-543-2011
- Oda, T., Maksyutov, S. and Andres, R. J. 2018. The open-source data inventory for anthropogenic carbon dioxide (CO2), version 2016 (ODIAC2016): a global, monthly fossil-fuel CO2 gridded emission data product for tracer transport simulations and surface flux inversions. Earth Syst. Sci. Data 10, 87–107. doi:10.5194/essd-10-87-2018
- Palmer, P. I., O'Doherty, S., Allen, G., Bower, K., Bösch, H. and co-authors. 2018. A measurement-based verification framework for UK greenhouse gas emissions: an overview of the Greenhouse gAs UK and global emissions (GAUGE) project. Atmos. Chem. Phys. 18, 11753–11777. doi:10.5194/acp-18-11753-2018
- Patra, P. K., Houweling, S., Krol, M., Bousquet, P., Belikov, D. and co-authors. 2011. TransCom model simulations of CH4 and related species: linking transport, surface flux and chemical loss with CH4 variability in the troposphere and lower stratosphere. Atmos. Chem. Phys. 11, 12813–12837. doi:10.5194/acp-11-12813-2011
- Patra, P. K. and Maksyutov, S. 2002. Incremental approach to the optimal network design for CO2 surface source inversion. Geophys. Res. Lett. 29, 97–1–97-4.
- Peylin, P., Baker, D., Sarmiento, J., Ciais, P. and Bousquet, P. 2002. Influence of transport uncertainty on annual mean and seasonal inversions of atmospheric CO2 data. J. Geophys. Res. 107, 4385. doi:10.1029/2001JD000857
- Pisso, I., Sollum, E., Grythe, H., Kristiansen, N. I., Cassiani, M. and co-authors. 2019. The Lagrangian particle dispersion model FLEXPART version 10.4. Geosci. Model Dev. 12, 4955–4997. doi:10.5194/gmd-12-4955-2019
- Rayner, P. J. 2004. Optimizing CO2 observing networks in the presence of model error: results from TransCom 3. Atmos. Chem. Phys. 4, 413–421. doi:10.5194/acp-4-413-2004
- Rayner, P. J., Enting, I. G., Francey, R. J. and Langenfelds, R. L. 1999. Reconstructing the recent carbon cycle from atmospheric CO2, 13C and O2/N2 observations. Tellus B 51, 213–232. doi:10.3402/tellusb.v51i2.16273
- Rayner, P. J., Enting, I. G. and Trudinger, C. M. 1996. Optimizing the CO2 observing network for constraining sources and sinks. Tellus B 48, 433–444. doi:10.3402/tellusb.v48i4.15924
- Reuter, M., Buchwitz, M., Schneising, O., Krautwurst, S., O'Dell, C. W. and co-authors. 2019. Towards monitoring localized CO2 emissions from space: co-located regional CO2 and NO2 enhancements observed by the OCO-2 and S5P satellites. Atmos. Chem. Phys. 19, 9371–9383. doi:10.5194/acp-19-9371-2019
- Rigby, M., Montzka, S. A., Prinn, R. G., White, J. W. C., Young, D. and co-authors. 2017. Role of atmospheric oxidation in recent methane growth. Proc. Natl. Acad. Sci. USA. 114, 5373–5377. doi:10.1073/pnas.1616426114
- Rödenbeck, C., Houweling, S., Gloor, M. and Heimann, M. 2003. CO2 flux history 1982–2001 inferred from atmospheric data using a global inversion of atmospheric transport. Atmos. Chem. Phys. 3, 1919–1964. doi:10.5194/acp-3-1919-2003
- Saikawa, E., Schlosser, C. A. and Prinn, R. G. 2013. Global modeling of soil nitrous oxide emissions from natural processes. Global Biogeochem. Cy. 27, 972–989. doi:10.1002/gbc.20087
- Saunois, M., Bousquet, P., Poulter, B., Peregon, A., Ciais, P. and co-authors. 2016. The global methane budget 2000–2012. Earth Syst. Sci. Data 8, 697–751. doi:10.5194/essd-8-697-2016
- Schneising, O., Buchwitz, M., Reuter, M., Bovensmann, H., Burrows, J. P. and co-authors. 2019. A scientific algorithm to simultane-ously retrieve carbon monoxide and methane from TROPOMI on-board Sentinel-5 Precursor. Atmos. Meas. Tech. 12, 6771–6802. doi:10.5194/amt-12-6771-2019
- Seibert, P. and Frank, A. 2004. Source-receptor matrix calculation with a Lagrangian particle dispersion model in backward mode. Atmos. Chem. Phys. 4, 51–63. doi:10.5194/acp-4-51-2004
- Sitch, S., Friedlingstein, P., Gruber, N., Jones, S. D., Murray-Tortarolo, G. and co-authors. 2015. Recent trends and drivers of regional sources and sinks of carbon dioxide. Biogeosciences 12, 653–679. doi:10.5194/bg-12-653-2015
- Sitch, S., Smith, B., Prentice, I. C., Arneth, A., Bondeau, A. and co-authors. 2003. Evaluation of ecosystem dynamics, plant geography and terrestrial carbon cycling in the LPJ dynamic global vegetation model. Global Change Biol. 9, 161–185. doi:10.1046/j.1365-2486.2003.00569.x
- Tarantola, A. 2005. Inverse problem theory and methods for model parameter estimation. Society for Industrial and Applied Mathematics, Philadelphia.
- Thompson, R. L., Lassaletta, L., Patra, P. K., Wilson, C., Wells, K. C. and co-authors. 2019. Acceleration of global N2O emissions seen from two decades of atmospheric inversion. Nat. Clim. Change 9, 993–998. doi:10.1038/s41558-019-0613-7
- Thompson, R. L. and Stohl, A. 2014. FLEXINVERT: an atmospheric Bayesian inversion framework for determining surface fluxes of trace species using an optimized grid. Geosci. Model Dev. 7, 2223–2242. doi:10.5194/gmd-7-2223-2014
- United Nations. 2019. World population prospects: the 2018 revision, Department of Economic and Social Affairs, Population Division. Online at: https://population.un.org/wup/Publications/Files/WUP2018-Report.pdf
- van der Werf, G. R., Randerson, J. T., Giglio, L., van Leeuwen, T. T., Chen, Y. and co-authors. 2017. Global fire emissions estimates during 1997–2016. Earth Syst. Sci. Data 9, 697–720. doi:10.5194/essd-9-697-2017
- Vesala, T., Kljun, N., Rannik, U., Rinne, J., Sogachev, A. and co-authors. 2008. Flux and concentration footprint modelling: State of the art. Environ. Pollut. 152, 653–666. doi:10.1016/j.envpol.2007.06.070
- Wang, H., Jiang, F., Wang, J., Ju, W. and Chen, J. M. 2019. Terrestrial ecosystem carbon flux estimated using GOSAT and OCO-2 XCO2 retrievals. Atmos. Chem. Phys. 19, 12067–12082. doi:10.5194/acp-19-12067-2019
- Wang, J. S., Kawa, S. R., Collatz, G. J., Sasakawa, M., Gatti, L. V. and co-authors. 2018. A global synthesis inversion analysis of recent variability in CO2 fluxes using GOSAT and in situ observations. Atmos. Chem. Phys. 18, 11097–11124.
- White, E. D., Rigby, M., Lunt, M. F., Smallman, T. L., Comyn-Platt, E. and co-authors. 2019. Quantifying the UK’s carbon diox-ide flux: an atmospheric inverse modelling approach using a regional measurement network. Atmos. Chem. Phys. 19, 4345–4365. doi:10.5194/acp-19-4345-2019.
- Whittlestone, S., Kowalczyk, E., Brunke, E. G. and Labuschagne, C. 2009. Source Regions for CO2 at Cape Point Assessed by Modelling 222Rn and Meteorological Data. Technical Report for the South African Weather Service, Pretoria, South Africa.
- Wu, Z., Hugelius, G., Luo, Y., Smith, B., Xia, J. and co-authors. 2019. Approaching the potential of model-data comparisons of global land carbon storage. Sci. Rep. 9, 3367. doi:10.1038/s41598-019-38976-y
- Ziehn, T., Nickless, A., Rayner, P. J., Law, R. M., Roff, G. and co-authors. 2014. Greenhouse gas network design using backward La-grangian particle dispersion modelling - Part 1: methodology and Australian test case. Atmos. Chem. Phys. 14, 9363–9378. doi:10.5194/acp-14-9363-2014