Abstract
Effective therapies for pathological gambling exist, but their use is limited to about 10% of the target population. In an attempt to lower the barriers for help, Internet-based cognitive behavioural therapy (ICBT) has been shown to be effective when delivered to a non-depressed sample with pathological gambling. This study sought to extend this finding to a larger, more representative population, and also test a model to predict responder status. Following advertisement, a total of 284 participants started an 8-week ICBT programme with minimal therapist contact via e-mail and weekly telephone calls of less than 15 min. The average time spent on each participant, including telephone conversations, e-mail, and administration, was 4 h. In addition to a mixed effects model to evaluate the effectiveness of the treatment, two logistic regression analyses were performed with the following eight pre-defined response predictor variables: work-life satisfaction, primary gambling activity, debts due to gambling, social support, personal yearly salary, alcohol consumption, stage of change, and dissociative gambling. ICBT resulted in statistically significant reductions in the scores of pathological gambling, anxiety, and depression as well as an increase in quality of life compared to pre-treatment levels. Follow-ups carried out in the treatment group at 6, 18, and 36 months indicated that treatment effects were sustained. Using the eight predictor variable model rendered an acceptable predictive ability to identify responders both at post-test (AUC = .72, p < .01) and at 36-month follow-up (AUC = .70, p < .01). We conclude that ICBT for pathological gamblers, even if depressed, can be effective and that outcome can partly be predicted by pre-treatment characteristics.
Keywords:
Introduction
Since pathological gambling was first introduced in the Diagnostic and statistical manual of mental disorders (American Psychiatric Association, Citation1980), there has been a fast development in the availability of games, primarily via the Internet (Hodgins, Stea, & Grant, Citation2011). One example is Sweden which, in 2006, introduced a state-owned Internet poker site which is essentially open all the time. Nowadays you can, in principle, sit in your underwear at home in the middle of the night with a whisky in your hand and gamble away all your savings. Social responsibility is said to be prioritised by the gaming operator, yet no peer-reviewed research has been published evaluating the effects of the preventive steps taken (e.g. warning messages) to ensure responsible gaming. The risks of developing pathological gambling habits are probably increased by the availability of online games, but this increased access has not been mirrored by increased access to psychological help in case gambling progresses into pathological gambling. This is unfortunate, since there are effective psychosocial treatments (Gooding & Tarrier, Citation2009; Pallesen, Mitsem, Kvale, Johnsen, & Molde, Citation2005). However, only about 10% of persons with pathological gambling seek treatment (Ladouceur, Citation2005). Besides a lack of therapists, long waitlists, and costs, it is likely that shame and stigma influence the decision to not seek help (Evans & Delfabbro, Citation2005). Therefore, there is a need to lower the barriers for seeking help. One way of achieving this objective is to offer help online.
However, the progress of online treatments for gambling problems has been slow. For instance, in a recent systematic review of Internet-based therapy studies on addictions, Gainsbury and Blaszczynski (Citation2011) concluded that only one study has been carried out with acceptable methodology on pathological gambling. In that particular study, carried out by our research group, Carlbring and Smit (Citation2008) randomised 66 participants to a treatment group or a waitlist control. The results were generally encouraging, with treatment gains maintained up to 36 months after treatment completion. However, the trial excluded all participants with a depression score exceeding 21 points on the self-rated version of the Montgomery-Asberg Depression Rating Scale (Svanborg &Åsberg, Citation1994). Although the idea was to reduce the risk of including participants with suicidal ideation, since no face-to-face contact was offered, this procedure limits the ability to generalise, especially since more people were excluded (n = 158) than included (n = 66). It is uncertain if the results from that particular study can be generalised to the larger population of pathological gamblers. Consequently, the present study was designed to keep the exclusion frequency to a minimum by, for example, including participants regardless of their depression score.
Not everyone entering treatment for pathological gambling completes it and, of those who do, not everyone reaches high end-state functioning (Carlbring, Jonsson, Josephson, & Forsberg, Citation2010). Attempts have been made to try to predict who will drop out (Dowling, Citation2009) and who will benefit from treatment (Dowling, Citation2009; Dowling & Cosic, Citation2010; Dunn, Delfabbro, & Harvey, Citation2011; Raylu & Oei, Citation2007). In Internet-based programmes this is an appealing idea. If we knew who would not likely benefit from a low-intensity intervention, we could override the stepped care approach in which a person receives the lowest service tier in the first instance, only stepping up to more intensive services as clinically required (Bower & Gilbody, Citation2005). Unfortunately, in order for patients to progress to the next level, a treatment failure needs to be recognised. If we had a way of predicting who would benefit from treatment, then those who would not benefit could skip the Internet-based treatment and go directly to a more intensive intervention.
The results of the pathological gambling treatment prediction studies are mixed. Some studies have suggested that high pre-treatment alcohol use is a negative predictor, while others have failed to replicate that finding (Dowling, Citation2009). Other studies have found that there is a lower likelihood of treatment response if the participant is young, living alone, has a low income and a low readiness to change (Dowling, Citation2009). In a study by Dowling on females presenting with pathological gambling, no differences could be found between dropouts and completers (Dowling, Citation2009). One reason for the inconclusiveness could be that researchers use different definitions of what a dropout is (cf. Eysenbach, Citation2005) and different predictors. Another problem is that groups are generally small, making statistical methods like logistic regression less suitable. Finally, there are reports that there might be different predictors in face-to-face therapy and Internet-based treatment (Andersson, Carlbring, & Grimund, Citation2008; Nordgreen et al., Citation2012).
In this study, we investigated predictors of who will have a successful outcome following ICBT by the use of eight variables collected in the screening phase. Previous studies have reasoned that since pathological gambling and alcohol and substance abuse share many commonalities, it is reasonable to assume that predictors of outcome in those studies also could have some relation to pathological gambling (Dowling, Citation2009). Even if results are inconclusive in the gambling field as to what variables can be used to predict the outcome, we decided to use eight different predictors in different domains that both theoretically and clinically would be plausible predictors of outcome. First, we entered work-life satisfaction as suggested by Sander and Peters (Citation2009). Second, primary gambling activity was used as a predictor variable since severity of gambling and psychosocial problems have been associated with preferred form of gambling activity (Champine & Petry, Citation2010). Third, debts due to gambling were entered, because financial difficulties have been reported to be an important motivator (Suurvali, Hodgins, & Cunningham, Citation2010). The fourth variable was social support, as lower levels have been shown to correlate with therapy dropout (Dunn et al., Citation2012; Melville, Casey, & Kavanagh, Citation2007; Oakes et al., Citation2011). Fifth, personal yearly salary was used as a proxy for socioeconomic status which is a known risk factor for pathological gambling (Welte, Barnes, Wieczorek, Tidwell, & Parker, Citation2004). Sixth, alcohol consumption was included as suggested by Rash, Weinstock, and Petry (Citation2011), since it has been shown that those who drink while gambling tended to gamble in more risky ways and experience more negative consequences of gambling (Cronce & Corbin, Citation2010). Hazardous alcohol use has previously been linked to continued difficulties with gambling over a 7-year period (Abbott, Williams, & Volberg, Citation2004). Seventh, in accordance with the transtheoretical model of behaviour change (Prochaska, DiClemente, & Norcross, Citation1992), the individual's readiness to act on a new healthier behaviour (stage of change) was included as suggested by Wohl and Sztainert (Citation2011). Finally, dissociative gambling was included as suggested by Jacobs (Citation1988). Although pathological gamblers might not experience dissociative symptoms at a higher rate than normal controls (Ledgerwood & Petry, Citation2006), it still can be a possible predictor of treatment outcome as it can differentiate different subtypes (Milosevic & Ledgerwood, Citation2010).
Methods
Design
Since the superiority of the treatment over a waitlist control had previously been demonstrated (Carlbring & Smit, Citation2008), this trial was designed as a non-comparative, single group study with measurements at baseline and at 3, 6, 18, and 36 months. The effect of the intervention was assessed using four criteria: Gambling (NORC DSM-IV Screen for gambling problems [NODS]; Gerstein et al., Citation1999), anxiety, depression (Hospital Anxiety and Depression Scale [HADS]; Zigmond & Snaith, Citation1983), and overall life satisfaction (quality of life inventory [QOLI]; Frisch, Cornell, Villanueva, & Retzlaff, Citation1992). The study protocol was approved by the regional medical ethics committee (Vetting the ethics of research involving humans in Sweden) and written informed consent was obtained from the participants. The trial is registered in Clinical Trials with the identifier NCT01381250.
Participants and recruitment
Advertisements in newspapers with the heading “Do you have trouble controlling your gambling?” and “Do you want to stop gambling?” were used to recruit participants (cf. Doiron & Nicki, Citation2007). Selection took place with a computerised screening interview consisting of the NODS (Gerstein et al., Citation1999), the HADS (Zigmond & Snaith, Citation1983), the QOLI (Frisch et al., Citation1992), and a number of additional questions related to gambling activities and demographics.
To be included in the study, participants had to meet the following criteria: (1) fulfil the DSM-IV-TR (American Psychiatric Association, Citation2000) criteria for pathological gambling according to the 1-month version of NODS, (2) live in Sweden, and (3) have gambled at least once in the past 30 days.
Of the 464 individuals who applied between May 2005 and May 2007, 316 fulfilled the criteria and 284 subsequently started treatments. The reasons for exclusion are specified in the CONSORT flowchart (see Figure ). The mean age of the included 284 participants (81% male) was 32.2 (SD = 8.8; range: 18–62) and the mean age of onset of regular gambling was 23.7 (SD = 9.1). The mean duration of gambling problems was 5.7 years (SD = 5.5). The most prevalent type of game played was poker and video lottery terminals (VLTs). For a more detailed description, see Tables and .
Figure 1 Participant flow, reasons for exclusion and number of participants providing data at different assessment points throughout the trial.
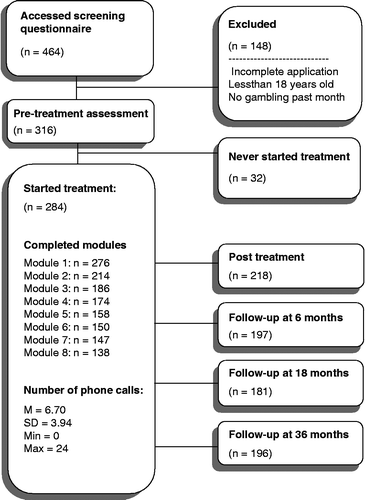
Table 1 Treatment outcome at pre-, post-, 6-, 18-, and 36-month follow-up for the collapsed group (n = 284) as well as the five different subtypes of primary problematic game using mixed effects model
Table 2 Factors associated with responder status at post-treatment (n = 218)
Treatment
The intervention was based on established CBT methods, as described in self-help books (Hodgins, Citation2002; Ladouceur & Lachance, Citation2006) and is described in more detail in Carlbring and Smit (Citation2008). Briefly, the treatment can be described as an eight-chapter book on the Internet, with each of the chapters covering a specific topic. The modules include information and exercises and end with three to eight essay-style questions. Participants were asked to answer the questions and provide worksheets and report on the outcomes of different exercises. For each module they were required to post at least one message in an online discussion group about a predetermined topic. Feedback on homework assignments was usually given via e-mail within 24 h after participants had sent their answers. Once a week telephone calls were made by the therapists to each participant. The purpose was to provide positive feedback and encouragement as well as to answer any questions the subjects had regarding the modules. Each conversation lasted approximately 15 min and a mean total of 6.7 (SD = 3.9) calls were made during the eight weeks.
The therapists were four social workers with an additional 2-year basic training in CBT and motivational interviewing (MI; Miller & Rollnick, Citation2002). The mean total time per week spent on each participant in this study was approximately 20 min, including telephone calls, administration, and responding to e-mails.
Predictor variables
A total of eight predictor variables were selected in order to predict responder status: (1) Work-life satisfaction was defined by the score in the corresponding question in the QOLI (Frisch et al., Citation1992) and subsequently categorised using Swedish norm data (Öst, Breitholtz, & Thulin, Citation1997). (2) Primary gambling activity was categorised by asking the participant (at screening) what game was most accountable for their problems. If two or more gambling types were reported, the one responsible for the majority of the problem was chosen. (3) Debts due to gambling were categorised as yes or no, regardless of the amount. (4) Social support was defined by the participant answering the question “Do you feel that you have someone available who can give you the right type of support to help you cope with life's stresses and problems?” (5) Personal yearly salary was divided into four categories, in an attempt to simulate no income, low income, moderate income, and high income. (6) The number of standard drinks during a typical drinking day was based on the corresponding question in the Alcohol Use Disorders Identification Test (Wennberg, Citation1996) (“How many drinks containing alcohol do you have on a typical day when you are drinking?”) (7) Stage of change was coded by analysing the response to the Swedish version of the Readiness to Change questionnaire. (8) Dissociative gambling was coded by analysing the response to a modified version of Jacobs' question now reading “Have you ever completely lost track of time when gambling?” (Jacobs, Citation1988). Treatment response was defined as having a total of 0 points on the 1-month version of NODS and no incident of gambling over the past 30 days.
Statistical analysis
To test for treatment outcome we used mixed-effects models as suggested by Gueorguieva and Krystal (Citation2004). To investigate possible predictive factors associated with the outcomes, we used logistic regression analysis. Continuous variables were categorised to gain clarity of effect and clinical interpretation. First, we studied crude (unadjusted) association of each factor with the odds of response. Second, to study the adjusted associations for possible confounders, we used multivariable logistic regression models with all factors included. The associations are presented as odds ratios (ORs) with 95% confidence intervals (CIs). There was no transformation or centreing with the pre-intervention measurements.
This analysis was done because in the analysis of longitudinal data, repeated observations for the same individual are correlated. If the repeated measures structure correlation is ignored, this may lead to imprecise variation estimates from the regression models, leading to incorrect statistical conclusions. Such correlation violates the assumption of independence necessary for more traditional, repeated-measures analysis and might lead to bias in regression parameters which could lead to wrong conclusions (Brown & Prescott, Citation1999; Gueorguieva & Krystal, Citation2004). Furthermore, mixed-effect models are more effective in accommodating missing data and the integration of time-varying factors, which are issues in this study.
When comparing the outcome for different subtypes of problematic games we used a covariance pattern model (Brown & Prescott, Citation1999), a special case of mixed-effects models. A separate model was estimated for each of the four outcome measures (HADS Anxiety, HADS Depression, NODS, and QOLI). The variance–covariance matrix was assumed to be block diagonal but unstructured within a block defined by subjects. To study if groups differed across the time points, with respect to the outcome measures, we tested the interaction between time and group. We used the restricted maximum likelihood (REML) as our model estimation method and present estimated means and differences between treatments and their respective standard error (SE) means.
To evaluate the ability of the predictor model to correctly discriminate between responders vs. non-responders, we calculated the area under the receiver operating curve (ROC; trapezoid rule). An area under the curve (AUC) equal to 0.5 suggests no discrimination, 0.7 to < 0.8 is acceptable, 0.8–0.9 is excellent, and >0.9 is outstanding discrimination (Hosmer & Lemenshow, Citation1989). We used the nonparametric method to calculate the SE for the AUC used in the CI and in the comparison of the ROC curves. This gives a conservative estimate of the SE, which implies that the risk of a false difference (type I error) in the evaluation and comparison of the ROC curves is low (Hanley & McNeil, Citation1982). Hosmer and Lemeshow's goodness-of-fit test was used to examine whether the adjusted models adequately fitted the data, and a p value >.05 indicates acceptable fit.
Analyses were done in SPSS 18.0 (SPSS, Inc., Chicago, IL, USA).
Results
Treatment outcome
The effects of the treatment are presented in three ways. First, we examine whether the treatment had any effect if type of gambling problem was not taken into account and the participants were analysed as a whole. Then the participants are divided into five subgroups based on the principal type of gambling problem and the analysis is repeated to see whether the results persist. Finally, we analysed whether there was any interaction between the type of gambling problem and the treatment outcome.
As shown in the first four columns of Table , there were statistically significant main effects of time on all outcome measurements when analysing the whole group, with statistically significant improvements in all four outcomes from pre- to post-intervention. This suggests that the treatment might have a significant positive impact on the participants and that improvements were maintained after 6, 18, and 36 months.
One of the objectives of the present study was to see how results would differ from a previous study (Carlbring & Smit, Citation2008) by including participants with more severe depression (MADRS-S score of 21 or higher). For the participants with more severe depression, the changes in outcome scores (pre-compared to post-intervention) for the gambling-specific scale NODS (n(241) = .93; p = .352) and for the anxiety measure (HADS-A; n(241) = 1.58; p = .115) were similar to those for participants with less severe depression. However, and fully consistent with the regression to the mean effect, the more severely depressed group had a significantly larger change in depression score (HADS-D; n(241) = 3.04; p = .003) compared to the less severely depressed. The more severely depressed group also benefited more in terms of quality of life (QOLI; n(241) = 2.43; p = .016).
Since there was no significant interaction between time and gambling problem, the conclusion drawn is that there is no evidence that the type of gambling activity affects the treatment outcome (see Table ). However, there is a significant main effect with regard to time on NODS. This is explained by the fact that there was a significant difference between those who gambled at VLTs, those who bet money on sports and those who played bingo. This initial difference in the NODS score was then maintained throughout over all measuring points. For those who were primarily casino gamblers, there was a similar trend in depression scores. What was common throughout was that none of these significant differences between the types of gambling are clinically relevant, since all effect sizes were insignificant (Cohen's d < .20).
Prediction
As evident from Table , responder status at post-treatment was significantly associated with the background variables of alcohol consumption and dissociative gambling in the unadjusted analysis. Specifically, consumption of three or more standard drinks during a typical drinking day reduced the ratio of responder status to half compared with those who typically consume two drinks or less. Therefore, low alcohol consumption was associated with a higher response rate. In the adjusted analysis only trends were identified (p = .056 and p = .075, respectively).
As evident from Table , three variables have a significant individual predictive ability: work-life satisfaction, number of standard drinks during a typical drinking day, and dissociative gambling. The type of primary problematic game showed only a trend (p = .054). However, the AUC for each of these individual variables was relatively low (.58–.61).
Table 3 Evaluation of the ability to predict responder status at post-treatment for different predictor variables and models
As evident from Table , the model with all eight variables resulted in an acceptable predictive ability to identify responders at post-treatment (AUC = .72, p < .01). However, similar results were obtained with a model with four variables (work-life satisfaction, primary gambling, dissociative gambling, and number of standard drinks during a typical drinking day; AUC = .69), and a model with only two variables (dissociative gambling and number of standard drinks during a typical drinking day; AUC = .67). Furthermore, there were no significant differences between the three models since the 95% CI for AUC all overlap. In conclusion, the number of standard drinks during a typical drinking day and dissociative gambling have the strongest association with responder status at post-treatment and have a marginal predictive ability in this sample (AUC = .67).
Table shows how responder status was associated with the prediction variables at 36-month follow-up. Only one variable, debts due to gambling, was significantly associated with a positive response. Both the unadjusted and the adjusted analyses showed that the response ratio was about twice as high for individuals with debt compared with the reference category of not having a debt. As shown in Table , the proportion of responders was relatively evenly distributed. No single variable had any significant predictive ability to discriminate between responder vs. non-responder at 36 months—not even debts due to gambling (AUC = .57, p = .129). However, a model with all eight variables together rendered an acceptable predictive ability to identify responders (AUC = .70, p < .01). Table shows that an eight-variable model has a greater chance of identifying a responder.
Table 4 Factors associated with responder status at 36-month follow-up (n = 196)
Table 5 Evaluation of the ability to predict responder status at post-treatment for different predictor variables and models
Discussion
The purpose of this study was twofold—to investigate whether the treatment was effective when participants with symptoms of depression were included and whether treatment response could be predicted by a set of pre-treatment characteristics. First, the intervention showed promise both immediately following the treatment termination and up to the 36-month follow-up. Second, it was possible to predict who will benefit from Internet-based treatment both at post-test and at 36 months by using a model of eight background variables.
While the results point to the value of guided ICBT for pathological gambling, we cannot be certain since no control group was included. This is a weakness in the design as it opens up the possibility that the observed effects are better explained by regression to the mean or spontaneous remission. Indeed, there are reports of significant proportions of participants who naturally recover (Petry, Citation2005; Slutske, Citation2006). On the other hand, when the same treatment was compared to a waitlist control in a similar, controlled study (Carlbring & Smit, Citation2008), there were statistically significant changes as a result of the intervention. The changes observed in the present study are similar in magnitude to those seen previously for all four outcomes (gambling, anxiety, depression, and quality of life), indicating that the effects are probably not due to regression to the mean.
There was an important difference between the population in the previous study, which did not include subjects with a score higher than 21 on the MADRS-S, and the population in this study which did include such subjects. When these populations were analysed separately in the present study, the intervention had a similar effect in more severely depressed participants as for those with less severe depression for gambling and anxiety, but a greater effect for depression and quality of life. These results should be considered in light of the fact that they are not as statistically robust since they were shown in a subgroup of the main study population. Furthermore, the difference in depression score is likely to be an effect of regression to the mean, since these subjects had higher depression scores pre-intervention. Hence, future studies could investigate whether participants meeting DSM criteria for comorbid major depression really benefit more from the intervention.
Interestingly, there were significant differences in depression levels and NODS points between the subgroups of gamblers depending on what game they played, but that did not appear to affect outcome. Since type of game did not interact with treatment outcome, we conclude that no subgroup benefited more from the Internet-based treatment. This is also reflected by the overlapping ORs in the prediction analysis. It seems that other variables are more important for the outcome rather than the type of game played.
The results from the logistic regression suggested that it is possible to predict who will respond to ICBT both at post-intervention and at 36 months using a model with eight variables. The single most important background variables vary over time from alcohol consumption and dissociative gambling at post-intervention to whether one had a gambling debt or not at the 3-year follow-up. While the results are interesting, it is still unclear whether the model is valid for other treatment modalities (e.g. group treatment and individual psychotherapy). The fact that having a gambling debt increased the ratio of later being a treatment responder is not consistent with what has been found previously. However, in a study by Ingle, Marotta, McMillan, and Wisdom (Citation2008), having a small gambling debt was better than not having one. It is possible that the dichotomy in this study can account for the difference, but it is more likely that the two studies used different time frames. Consistent with the Ingle et al's study was that having social support seemed to have a positive influence.
A major issue in prediction analysis is how the outcome variable is defined. In this study, it was defined as having 0 points on the 1-month version of NODS and not having gambled at all over the past 30 days. It is possible that the results would have been different had the response been defined, for example, using the Jacobson and Truax formula for clinical significant improvement (Jacobson & Truax, Citation1991). Also, the results might be specific to different subgroups. This trial grouped together participants regardless whether they, for example, had any comorbid psychiatric disorder. Furthermore, it could be argued that other predictor variables could be important, such as sex, age, whether or not a person is gambling primarily for sensations seeking or to escape from anxiety, and the reason for seeking help (Dowling, Citation2009; Grant, Kim, Hollander, & Potenza, Citation2008; Jamieson, Mazmanian, Penney, Black, & Nguyen, Citation2011).
In summary, the results from this study generally provide evidence for the continued use and development of guided Internet-delivered self-help with telephone support for pathological gamblers. In addition, this study was probably the first to suggest a model for predicting treatment outcomes for pathological gambling by using guided Internet-based treatment. However, the results need to be replicated to ensure that this is not only sample-specific associations. In addition, mechanisms of change needs to be addressed (cf. Andersson, Carlbring, Berger, Almlöv, & Cuijpers, Citation2009).
Acknowledgements
This study was funded by a grant from the Swedish National Institute of Public Health. We would like to thank Ann-Katrin Edlund, Birgitta Enmark, Anna Marklund, and Emma Edlund for serving as therapists and Maria Svensk, Maria Bergström, and Lise Bergman-Nordgren for their help with data collection.
Notes
ClinicalTrials.gov identifier: NCT01381250.
References
- Abbott , M. W. , Williams , M. M. and Volberg , R. A. 2004 . A prospective study of problem and regular nonproblem gamblers living in the community . Substance Use & Misuse , 39 ( 6 ) : 855 – 884 .
- American Psychiatric Association . 1980 . Diagnostic and statistical manual of mental disorders , 3rd ed. , Washington, DC : American Psychiatric Press .
- American Psychiatric Association . 2000 . Diagnostic and statistical manual of mental disorders—Text revision , 4th ed. , Washington, DC : American Psychiatric Press .
- Andersson , G. , Carlbring , P. , Berger , T. , Almlöv , J. and Cuijpers , P. 2009 . What makes Internet therapy work? . Cognitive Behaviour Therapy , 38 ( Suppl. 1 ) : 55 – 60 .
- Andersson , G. , Carlbring , P. and Grimund , A. 2008 . Predicting treatment outcome in Internet versus face to face treatment of panic disorder . Computers in Human Behavior , 24 ( 5 ) : 1790 – 1801 . doi: 10.1016/j.chb.2008.02.003
- Bower , P. and Gilbody , S. 2005 . Stepped care in psychological therapies: Access, effectiveness and efficiency: Narrative literature review . The British Journal of Psychiatry , 186 ( 1 ) : 11 – 17 . doi: 10.1192/bjp.186.1.11
- Brown , H. and Prescott , R. 1999 . Applied mixed models in medicine , New York : Oxford University Press, Inc .
- Carlbring , P. , Jonsson , J. , Josephson , H. and Forsberg , L. 2010 . Motivational interviewing versus cognitive behavioral group therapy in the treatment of problem and pathological gambling: A randomized controlled trial . Cognitive Behaviour Therapy , 39 ( 2 ) : 92 – 103 . doi: 10.1080/16506070903190245, 917437376 [pii]
- Carlbring , P. and Smit , F. 2008 . Randomized trial of Internet-delivered self-help with telephone support for pathological gamblers . Journal of Consulting and Clinical Psychology , 76 ( 6 ) : 1090 – 1094 . doi: 10.1037/a0013603, 2008-16943-010 [pii]
- Champine , R. B. and Petry , N. M. 2010 . Pathological gamblers respond equally well to cognitive-behavioral therapy regardless of other mental health treatment status . American Journal of Addictions , 19 ( 6 ) : 550 – 556 . doi: 10.1111/j.1521-0391.2010.00085.x
- Cronce , J. M. and Corbin , W. R. 2010 . Effects of alcohol and initial gambling outcomes on within-session gambling behavior . Experimental and Clinical Psychopharmacology , 18 ( 2 ) : 145 – 157 . doi: 10.1037/a0019114, 2010-06751-005 [pii]
- Doiron , J. P. and Nicki , R. M. 2007 . Prevention of pathological gambling: A randomized controlled trial . Cognitive Behaviour Therapy , 36 ( 2 ) : 74 – 84 . doi: 10.1080/16506070601092966
- Dowling , N. 2009 . Client characteristics associated with treatment attrition and outcome in female pathological gambling . Addiction Research & Theory , 17 ( 2 ) : 205 – 219 . doi: 10.1080/16066350802346193
- Dowling , N. and Cosic , S. 2010 . Client engagement characteristics associated with problem gambling treatment outcomes . International Journal of Mental Health and Addiction , 9 ( 6 ) : 656 – 671 . doi: 10.1007/s11469-010-9298-x
- Dunn , K. , Delfabbro , P. and Harvey , P. 2012 . A preliminary, qualitative exploration of the influences associated with drop-out from cognitive-behavioural therapy for problem gambling: An Australian perspective . Journal of Gambling Studies , 28 ( 2 ) : 253 – 272 . doi: 10.1007/s10899-011-9257-x
- Evans , L. and Delfabbro , P. H. 2005 . Motivators for change and barriers to help-seeking in Australian problem gamblers . Journal of Gambling Studies , 21 ( 2 ) : 133 – 155 .
- Eysenbach , G. 2005 . The law of attrition . Journal of Medical Internet Research , 7 ( 1 ) : e11 doi: 10.2196/jmir.7.1.e11, v7e11 [pii]
- Frisch , M. B. , Cornell , J. , Villanueva , M. and Retzlaff , P. J. 1992 . Clinical validation of the Quality of Life Inventory. A measure of life satisfaction for use in treatment planning and outcome assessment . Psychological Assessment , 4 ( 1 ) : 92 – 101 .
- Gainsbury , S. and Blaszczynski , A. 2011 . A systematic review of Internet-based therapy for the treatment of addictions [Review] . Clinical Psychology Review , 31 ( 3 ) : 490 – 498 . doi: 10.1016/j.cpr.2010.11.007
- Gerstein , D , Murphy , S , Toce , J. , Hoffmann , A. , Palmer , R. , Johnson , C. , Larison , L. , Chuchro , A. , Bard , L. , Englemann , M. , Hill , T. , Buie , R. , Volberg , H. , Harwood , A. , Tucker , E. , Christiansen , W. , Cummings and Sinclair , S. 1999 . Gambling impact and behaviour , Chicago, IL : National Gambling Impact Study Commission .
- Gooding , P. and Tarrier , N. 2009 . A systematic review and meta-analysis of cognitive-behavioural interventions to reduce problem gambling: Hedging our bets? . Behaviour Research and Therapy , 47 ( 7 ) : 592 – 607 . doi: 10.1016/j.brat.2009.04.002, S0005-7967(09)00087-4 [pii]
- Grant , J. , Kim , S. , Hollander , E. and Potenza , M. 2008 . Predicting response to opiate antagonists and placebo in the treatment of pathological gambling . Psychopharmacology , 200 ( 4 ) : 521 – 527 . doi: 10.1007/s00213-008-1235-3
- Gueorguieva , R. and Krystal , J. H. 2004 . Move over ANOVA: Progress in analyzing repeated-measures data and its reflection in papers published in the archives of general psychiatry . Archives of General Psychiatry , 61 ( 3 ) : 310 – 317 .
- Hanley , J. A. and McNeil , B. J. 1982 . The meaning and use of the area under a receiver operating characteristic (ROC) curve . Radiology , 143 ( 1 ) : 29 – 36 .
- Hodgins , D. C. 2002 . Becoming a winner: Defeating problem gambling: A self-help manual for problem gamblers , Calgary, AB : Addiction Centre, Calgary Regional Health Authority .
- Hodgins , D. C. , Stea , J. N. and Grant , J. E. 2011 . Gambling disorders . Lancet , 378 ( 9806 ) : 1874 – 1884 . doi: 10.1016/S0140-6736(10)62185-X
- Hosmer , D. W. and Lemenshow , S. 1989 . Applied logistic regression , New York : Wiley .
- Ingle , P. , Marotta , J. , McMillan , G. and Wisdom , J. 2008 . Significant others and gambling treatment outcomes . Journal of Gambling Studies , 24 ( 3 ) : 381 – 392 . doi: 10.1007/s10899-008-9092-x
- Jacobs , D. F. 1988 . Evidence for a common dissociative-like reaction among addicts . Journal of Gambling Studies , 4 ( 1 ) : 27 – 37 . doi: 10.1007/bf01043526
- Jacobson , N. S. and Truax , P. 1991 . Clinical significance: A statistical approach to defining meaningful change in psychotherapy research . Journal of Consulting and Clinical Psychology , 59 ( 1 ) : 12 – 19 .
- Jamieson , J. , Mazmanian , D. , Penney , A. , Black , N. and Nguyen , A. 2011 . When problem gambling is the primary reason for seeking addiction treatment . International Journal of Mental Health and Addiction , 9 ( 2 ) : 180 – 192 . doi: 10.1007/s11469-009-9268-3
- Ladouceur , R. 2005 . Controlled gambling for pathological gamblers . Journal of Gambling Studies , 21 ( 1 ) : 49 – 57 .
- Ladouceur , R. and Lachance , S. 2006 . Overcoming your pathological gambling: Workbook , Oxford : Oxford University Press .
- Ledgerwood , D. M. and Petry , N. M. 2006 . Psychological experience of gambling and subtypes of pathological gamblers . Psychiatry Research , 144 ( 1 ) : 17 – 27 . doi: 10.1016/j.psychres.2005.08.017, S0165-1781(05)00281-7 [pii]
- Melville , K. M. , Casey , L. M. and Kavanagh , D. J. 2007 . Psychological treatment dropout among pathological gamblers . Clinical Psychology Review , 27 ( 8 ) : 944 – 958 . doi: 10.1016/j.cpr.2007.02.004, S0272-7358(07)00057-8 [pii]
- Miller , W. R. and Rollnick , S. 2002 . Motivational interviewing: preparing people for change , New York : Guilford Press .
- Milosevic , A. and Ledgerwood , D. M. 2010 . The subtyping of pathological gambling: A comprehensive review . Clinical Psychology Review , 30 ( 8 ) : 988 – 998 . doi: 10.1016/j.cpr.2010.06.013, S0272-7358(10)00107-8 [pii]
- Nordgreen , T. , Havik , O. E. , Ost , L. G. , Furmark , T. , Carlbring , P. and Andersson , G. 2012 . Outcome predictors in guided and unguided self-help for social anxiety disorder [randomized controlled trial] . Behaviour Research and Therapy , 50 ( 1 ) : 13 – 21 . doi: 10.1016/j.brat.2011.10.009
- Oakes , J. , Pols , R. , Battersby , M. , Lawn , S. , Pulvirenti , M. and Smith , D. 2011 . A focus group study of predictors of relapse in electronic gaming machine problem gambling. Part 2: Factors that ‘pull’ the gambler away from relapse . Journal of Gambling Studies , doi: 10.1007/s10899-011-9267-8
- Öst , L. -G. , Breitholtz , E. and Thulin , U. 1997 . Quality of life in anxiety disorder patients Stockholm Unpublished manuscript
- Pallesen , S. , Mitsem , M. , Kvale , G. , Johnsen , B. -H. and Molde , H. 2005 . Outcome of psychological treatments of pathological gambling: A review and meta-analysis . Addiction , 100 ( 10 ) : 1412 – 1422 .
- Petry , N. M. 2005 . Stages of change in treatment-seeking pathological gamblers . Journal of Consulting and Clinical Psychology , 73 ( 2 ) : 312 – 322 .
- Prochaska , J. O. , DiClemente , C. C. and Norcross , J. C. 1992 . In search of how people change: Applications to addictive behaviors . American Psychologist , 47 ( 9 ) : 1102 – 1114 .
- Rash , C. J. , Weinstock , J. and Petry , N. M. 2011 . Drinking patterns of pathological gamblers before, during, and after gambling treatment . Psychology of Addictive Behaviors , 25 ( 4 ) : 664 – 674 . doi: 10.1037/a0025565, 2011-21202-001 [pii]
- Raylu , N. and Oei , T. 2007 . Factors that predict treatment outcomes in a community treatment agency for problem gamblers . International Journal of Mental Health and Addiction , 5 ( 2 ) : 165 – 176 . doi: 10.1007/s11469-007-9056-x
- Sander , W. and Peters , A. 2009 . Pathological gambling: influence of quality of life and psychological distress on abstinence after cognitive-behavioral inpatient treatment . Journal of Gambling Studies , 25 ( 2 ) : 253 – 262 . doi: 10.1007/s10899-009-9128-x
- Slutske , W. S. 2006 . Natural recovery and treatment-seeking in pathological gambling: Results of two U.S. National Surveys . American Journal of Psychiatry , 163 ( 2 ) : 297 – 302 .
- Suurvali , H. , Hodgins , D. C. and Cunningham , J. A. 2010 . Motivators for resolving or seeking help for gambling problems: A review of the empirical literature . Journal of Gambling Studies , 26 ( 1 ) : 1 – 33 . doi: 10.1007/s10899-009-9151-y
- Svanborg , P. and Åsberg , M. 1994 . A new self-rating scale for depression and anxiety states based on the comprehensive psychopathological rating scale . ACTA Psychiatrica Scandinavica , 89 ( 1 ) : 21 – 28 .
- Welte , J. W. , Barnes , G. M. , Wieczorek , W. F. , Tidwell , M. C. and Parker , J. C. 2004 . Risk factors for pathological gambling . Addictive Behaviors , 29 ( 2 ) : 323 – 335 . S0306460303001205 [pii]
- Wennberg, P. (1996). The alcohol use disorders identification test (AUDIT): A psychometric evaluation., Reports from the Department of Psychology, Stockholm University
- Wohl , M. J. and Sztainert , T. 2011 . Where did all the pathological gamblers go? Gambling symptomatology and stage of change predict attrition in longitudinal research . Journal of Gambling Studies , 27 ( 1 ) : 155 – 169 . doi: 10.1007/s10899-010-9186-0
- Zigmond , A. S. and Snaith , R. P. 1983 . The Hospital Anxiety and Depression Scale . Acta Psychiatrica Scandinavica , 67 ( 6 ) : 361 – 370 .