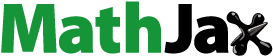
ABSTRACT
Although pneumococcal vaccines are quite effective in reducing disease burden, factors such as imperfect vaccine efficacy and serotype replacement present an important challenge against realizing direct and herd protection benefits of the vaccines. In this study, a novel mathematical model is designed and used to describe the dynamics of two Streptococcus pneumoniae (SP) serotypes, in response to the introduction of a cohort vaccination program which targets one of the two serotypes. The model is fitted to a pediatric SP carriage prevalence data from Atlanta, GA. The model, which is rigorously analysed to investigate the existence and asymptotic stability properties of the associated equilibria (in addition to exploring conditions for competitive exclusion), is simulated to assess the impact of vaccination under different levels of serotype-specific competition and illustrate the phenomenon of serotype replacement. The calibrated model is used to forecast the carriage prevalence in the pediatric cohort over 30 years.
1. Introduction
Nasopharyngial carriage of (or carriage with) Streptococcus pneumoniae (SP) is the primary transmission mechanism and precursor for pneumococcal disease (PD). SP, first isolated in 1881, is a gram-positive diplococcus with over 90 distinct capsular serotypes [Citation32]. With the human nasopharynx as its main reservoir (mostly of young children), SP can spread from person-to-person [Citation5]. Asymptomatic carriers of SP are responsible for most transmission [Citation2, Citation58]. The colonizing bacteria can also spread to the surrounding tissue, or invade the bloodstream of an individual, and can cause mild infection in the upper respiratory tract (acute otitis media and sinusitis) or more severe form of PD (such as pneumonia, meningitis and bacteremia) [Citation56]. PD can be invasive (bacteremia, meningitis and bacteremic pneumonia) when SP infects a normally sterile body site [Citation63] or non-invasive (non-bacteremic pneumonia, mastoiditis, conjuctivitis, acute otitis media or sinusitis).
Despite the high effectiveness of currently available pneumococcal conjugate vaccines (PCVs), pneumococcal carriage continues to cause major public health burden of PD globally. The disease remains a major cause of morbidity and mortality in children under 5 years of age and in older adults (65 and above). In 2016, over 1.2 million deaths resulted from pneumococcal pneumonia [Citation37, Citation57]. In the USA alone, 31,000 cases and 3590 deaths were attributed to invasive PD in 2017 [Citation11].
Since the introduction and widespread use of licensed multi-valent PCVs (dating back to the year 2000), the global burden of PD has steadily decreased dramatically [Citation67]. Unfortunately, however, vaccinated and unvaccinated individuals remain susceptible to disease caused by the non-vaccine SP serotypes (known as non-vaccine-types or, simply, NVT). The first PCV, a heptavalent vaccine offering protection against serotypes 4, 6B, 9V, 14, 18C, 19F, and 23F, was licensed and recommended for use in infants in the USA in 2000 [Citation44]. Two subsequent vaccines, a 10-valent vaccine (by GlaxoSmithKline, covering additionally, serotypes 1, 5, and 7F) and a 13-valent vaccine Prevnar13ő (by Pfizer, targeting serotypes 3, 6A, and 19A in addition to the PCV10 serotypes), are now being used in over 130 countries as a prophylactic measure against PD [Citation44].
The Advisory Committee on Immunization Practices to the Centers for Disease Control and Prevention recommends children to be immunized with four doses of PCV13 at the ages of 2, 4, 6 and 12–15 months. One dose of PPV23 is recommended for adults 65 and above, possibly with a prior dose of PCV13 with shared clinical decision-making [Citation45]. With the exception of 6A, the 23-valent vaccine Pneumovax23ő targets all the serotypes included in Prevnar13ő and additionally protects against serotypes 22F, 33F, 8, 10A, 11A, 12F, 15B, 2, 9N, 17F, and 20. New investigational PCVs with higher valency are under development [Citation46–48]. The reduction in the prevalence of vaccine-types (VT) has been observed to be counteracted by that of the NVT following the PCV vaccination [Citation8, Citation34, Citation35, Citation41, Citation42, Citation61, Citation63, Citation64, Citation68] through the phenomenon of serotype replacement [Citation38]. In the context of nasopharyngial carriage and the invasive pneumococcal disease (IPD), Weinberger et al. [Citation64] defined serotype replacement in carriage as the increase in the prevalence of the NVT after vaccine introduction. Serotype replacement is inevitable if the vaccines used in the community only target a subset of the circulating SP serotypes [Citation4, Citation31]. This dynamic phenomenon undercuts the beneficial effects of vaccines [Citation35], including the herd protection effects of a PCV [Citation41]. Lipsitch [Citation35] urged, however, that it was imperative not to confound serotype replacement with unmasking, a sampling artefact whereby the NVT, even though not more abundant, are more likely to be detected in a vaccinated individual (the VT mask the NVT in unvaccinated individuals, and are difficult to be detected by the existing methods, which have low sensitivity for the minority types).
SP Serotypes compete for carriage where current carriage with a certain serotype reduces the risk to acquire another serotype [Citation14, Citation15, Citation21, Citation34, Citation43, Citation62], and the competition between VT and NVT plays an important role in herd protection. For instance, PCVs reduce the prevalence of VT carriage, thereby providing a competitive advantage to the NVT over the VT [Citation22, Citation32, Citation34, Citation44]. Furthermore, co-colonization with multiple SP serotypes is possible [Citation1, Citation7, Citation29, Citation41, Citation60, Citation66]. In a pre-vaccine era study, Brugger et al. [Citation7] found co-colonization in 90% of carriage-positive samples.
Numerous dynamic models of pneumococcal carriage and disease have been designed and used in the literature to gain insight into the impact of routine vaccination against SP and PD. These models, which typically subdivide the total population into mutually exclusive compartments of individuals who are susceptible (S), vaccinated (V), exposed (E), infectious (I), or recovered (R), range from adopting a deterministic to stochastic to individual-based formalism (see the review article by Lochen and Anderson [Citation37], which also emphasizes that, even within the deterministic modelling paradigm, varying formalisms, such as SIS, SIR, SEI, SI and SIRS have been used to model pneumococcal carriage and disease [Citation13–15, Citation33–35, Citation41, Citation54, Citation58, Citation62] (although the vast majority adopts an SIS structure)). This diversity of modelling paradigms and structures affirm the complexity of the natural history of pneumococcal carriage and scarcity of data on recovery from pneumococcal carriage and disease.
Lipsitch [Citation34] presented an SIS model, with two SP serotypes and co-colonization, for analysing the impact of vaccination on competitive exclusion and coexistence of the serotypes. Their study shows that the presence of an NVT synergizes with the vaccine to reduce VT transmission. The model in [Citation34] was used in a subsequent study to highlight the importance of serotype replacement and the need to further investigate the contrasting role of this phenomenon (where serotype replacement diminishes the impact of vaccines by allowing the NVT related PD to rise as the VT related PD is reduced by the serotype-specific vaccines) [Citation35]. Melegaro et al. [Citation41] developed and used an SIS model to predict the impact of PCV7 on the IPD incidence. In particular, this study showed that although elimination of the VT with PCV7 is achievable, serotype replacement with NVT poses a significant challenge or setback to attaining the optimal benefits of the vaccine. Bottomley et al. [Citation8] presented an SIR model of SP carriage with multiple serotypes to predict the prevalence of VT and NVT following the PCV7 introduction. This model incorporates serotype-specific immunity, where a proportion of infected individuals is assumed to develop lifelong immunity to the serotype after surviving the infection. Sutton et al. [Citation55] developed and analysed an age-structured SEIS model of PD with vaccination. Their model, which assumes a seasonal infection rate, was used to assess the impact of vaccination strategies at a community level.
Generalizing the model of Melegaro et al. [Citation41], Choi et al. [Citation14] proposed a model incorporating carriage with VT, NVT, and a VT-NVT co-colonization to predict the vaccine impact on VT and NVT IPD incidence. The structure of the model does not explicitly incorporate natural immunity. Similarly, the model in Choi et al. [Citation15] splits the serotypes into groups and estimates the parameters governing infection transmission and competition effect between the groups. The effect of switching from PCV7 to PCV13, and that of no vaccination, on IPD cases is assessed. Cobey and Lipsitch [Citation13] used their model to investigate how weak serotype-specific immune response can support coexistence of diverse serotypes.
Gaivao et al. [Citation29] constructed a model to examine competition and facilitation among two co-colonizing serotypes. Global behaviour of the model is mathematically analysed and conditions for endemic persistence are explored. The model does not include vaccination or any other intervention. A two-strain epidemic model was constructed and analysed in [Citation33] incorporating super-infection, where individuals infected with one strain can get infected with the other, replacing the first strain. It was shown, through rigorous global asymptotic stability analysis, that if regulated by super-infection, the disease dynamics can lead to serotype replacement even with a perfect vaccine. Co-colonization is not considered. Vaccination is assumed to protect completely against a target serotype (VT) and partially, fully, or not at all against a second serotype. The model also does not allow for natural immunity after carriage. It establishes, using an individual reproduction number for each serotype, that the presence of an NVT synergizes with the vaccine to reduce the VT transmission. Some modelling studies considered age structure in the dynamics of pneumococcal carriage, disease and vaccination. For instance, Snedecor et al. [Citation54] used an age-structured model to evaluate the herd immunity effects of infant vaccination with PCV7 in the other age groups. Similarly, Wu et al. [Citation65] developed an age-structured model to carry out the long-term cost-effectiveness analysis of PCV13 in Taiwan. Wasserman et al. [Citation68] used an age-structured vaccination model, explicitly incorporating disease compartments, to assess the increase in IPD burden after a change in the dosing schedule in the United Kingdom.
The current study is based on the design, analysis and simulations of a novel dynamic model for gaining realistic insight into pneumococcal carriage and the transmission dynamics of two SP serotypes (one serotype is assumed to be VT and the other is assumed to be NVT) in the presence of a pneumococcal vaccine in a human population. The likelihood of co-colonization with more than two serotypes is insignificant (see [Citation62] and references therein). Most (90%) of the samples detected with carriage in the study reported in Brugger et al. [Citation7] contained two strains. Thus the model to be developed in this study will assume co-colonization with two SP serotypes. Furthermore, in the study reported in [Citation7], half of the co-colonizations were found to be either VT only (20%) or NVT only (30%). Therefore, the proposed model assumes co-colonization with mixed VT-NVT types. That is, it is assumed that the vaccine provides positive efficacy against serotype i (i.e. i is VT) and no protection against serotype j (i.e. j is NVT). Similar co-colonization (between the mixed VT and NVT) is assumed in [Citation41]. This categorization of SP serotypes into VT and NVT i and j respectively is suited to address serotype replacement arising from routine vaccination (the NVT serotype increases in prevalence to fill the niche vacated by the VT following the vaccination). The novel model to be developed also accounts for partial immunity after carriage, in addition to incorporating carriage with an individual serotype.
Being the main reservoir for transmission, the asymptomatic carriage states are the target of the conjugate vaccines [Citation35, Citation59] and are more frequent than disease states [Citation29, Citation35]. Since the contribution of symptomatic individuals to the transmission and overall mortality is negligible, they are not considered in this study. The model to be developed in this study tracks the natural history of carriage. In particular, individuals who had no prior history of carriage will be distinguished from those who have recovered from previous carriage with a certain serotype (or both serotypes), hence acquiring partial natural immunity against such serotype(s). This reduces the risk of subsequent carriage with the same serotype(s). The vaccine protection can wane over time.
2. Model formulation
The multi-serotype vaccination model for SP transmission dynamics is formulated by subdividing the total human population into mutually exclusive compartments based on the natural history of the disease (i.e. non-colonization, carriage with a single SP serotype i or j, co-colonization with both serotypes, recovery from carriage, etc.) and vaccination status (i.e. vaccinated or unvaccinated). Serotype-i is assumed to be VT, while j is NVT. Current carriage with SP serotype is represented using a superscript notation in state variables, vaccine protection is represented using the subscript notation vi, and natural immunity due to exposure history of serotype i and j is represented using the subscript notation ni and nj, respectively. Finally, a subscript v alone (and not followed by i) is used to distinguish the class of individuals who, although were vaccinated in the past, have experienced SP acquisition (consequently, the vaccine is assumed to provide no protection against that particular serotype). It is further assumed that co-colonization is acquired sequentially (and not simultaneously). Elbasha and Galvani [Citation20] made a similar assumption for a model with multiple serotypes of human papillomavirus.
The total human population at time t, , is subdivided into sub-populations of unvaccinated non-colonized (
, colonized with serotype k (
(where
), co-colonized (
, those who recovered from carriage and having immunity against serotype k (
), those who recovered from, and have natural immunity against, both serotypes i and j (
), and those with subsequent carriage with
but having natural immunity against
(
). The compartments representing corresponding vaccinated sub-populations are defined similarly and denoted with the subscript v (instead of u). The equations of the model are given by (EquationA.1
(A.1)
(A.1) ) in Appendix A. The basic qualitative features of the model are rigorously assessed in Appendix B. A schematic diagram of the model is depicted in Figure , and the state variables and parameters of the model are described in Tables and , respectively.
Figure 1. Flow diagram of the model (EquationA.1(A.1)
(A.1) ), showing the transitions of the unvaccinated (left) and vaccinated (right) state variables (or components) of the model.
![Figure 1. Flow diagram of the model (EquationA.1(A.1) (Nu)′=Λ(1−ϕ)+ωNv−(λi+λj+μ)Nu,(Nv)′=Λϕ−[(1−ϵ)λi+λj+ω+μ]Nv,(Cui)′=λiNu+ηiλiRuni−(γi+θiλj+μ)Cui,(Cvi)′=(1−ϵ)λiNv+ηiλiRvni−(θvγi+θiλj+μ)Cvi,(Cuj)′=λjNu+ηjλjRunj+ωCvj−(γj+θjλi+μ)Cuj,(Cvj)′=λjNv+ηjλjRvnj−[γj+θj(1−ϵ)λi+ω+μ]Cvj,(Cuij)′=θiλjCui+θjλiCuj+θjηiλiCunij+θiηjλjCunji−(γij+μ)Cuij,(Cvij)′=θj(1−ϵ)λiCvj+θiλjCvi+θjηiλiCvnij+θiηjλjCvnji−[(θv(1−r2)+r2)γij+μ]Cvij,(Runi)′=γiCui−(ηiλi+λj+μ)Runi,(Rvni)′=θvγiCvi−(ηiλi+λj+μ)Rvni,(Runj)′=γjCuj+ωRvnj−(ηjλj+λi+μ)Runj,(Rvnj)′=γjCvj−[ηjλj+(1−ϵ)λi+ω+μ]Rvnj,(Runinj)′=γiCunji+γjCunij+(1−r1−r2)γijCuij−(ηiλi+ηjλj+μ)Runinj,(Rvninj)′=θvγiCvnji+γjCvnij+θv(1−r2)(1−r1)γijCvij−(ηiλi+ηjλj+μ)Rvninj,(Cunji)′=λiRunj+ηiλiRuninj+r2γijCuij−(γi+θiηjλj+μ)Cunji,(Cvnji)′=(1−ϵ)λiRvnj+ηiλiRvninj+r2γijCvij−(θvγi+θiηjλj+μ)Cvnji,(Cunij)′=λjRuni+ηjλjRuninj+r1γijCuij−(γj+θjηiλi+μ)Cunij,(Cvnij)′=λjRvni+ηjλjRvninj+θv(1−r2)r1γijCvij−(γj+θjηiλi+μ)Cvnij.(A.1) ), showing the transitions of the unvaccinated (left) and vaccinated (right) state variables (or components) of the model.](/cms/asset/bf27669d-d42d-4ff6-b54d-d11cb3235157/tjbd_a_1884760_f0001_oc.jpg)
Table 1. Description of the state variables of the model (EquationA.1(A.1)
(A.1) ).
.
Table 2. Description of parameters of the model (EquationA.1(A.1)
(A.1) ).
The model (EquationA.1(A.1)
(A.1) ) is an extension of numerous models for pneumococcal carriage that incorporate a vaccine, such as those in [Citation8, Citation14, Citation15, Citation33, Citation34, Citation41, Citation54, Citation55, Citation58, Citation62, Citation65, Citation68], by inter alia:
Explicitly tracking the natural history of carriage (the model in [Citation29] considers carriage and co-colonization, but does not incorporate a vaccine)
Allowing for the waning of vaccine-induced immunity over time (this was not accounted for in the aforementioned modelling studies, except in [Citation15, Citation33, Citation41])
Allowing temporary partial natural immunity following carriage (this was not accounted for in the aforementioned modelling studies, except in [Citation58, Citation62, Citation65]).
The model (EquationA.1(A.1)
(A.1) ) is formulated based on the following key underlying assumptions:
The population is well-mixed, so that every member of the cohort can acquire infection from any other member of the cohort (i.e. we assume a homogeneously-mixed population).
The average waiting time in each epidemiological compartment is exponentially distributed.
The vaccine does not provide therapeutic benefit against pneumococcal carriage.
Exposure to carriage with a certain serotype induces partial natural immunity against subsequent carriage acquisitions with the same serotype.
Numerical simulations of the model (EquationA.1(A.1)
(A.1) ) will be carried out using the ranges and baseline parameter values tabulated in Table . The ranges and baseline values of many of the parameters of the model are obtained from the published literature, as described in Section 5.1.
3. Qualitative analysis of the model without vaccination
Before analysing the dynamics of the vaccination model (EquationA.1(A.1)
(A.1) ), it is instructive to study the dynamics of the special case of the model in the absence of vaccination. The aim of this exercise is to determine whether adding vaccination to a base model for the dynamics of two serotypes of SP in a cohort alters its qualitative features. In other words, we seek to answer the question whether there will be dynamical features present in the vaccination model that may not be present in the reduced version of the model that does not include vaccination. This is explored below. It should be noted, first of all, that setting the vaccine-related parameters (ϕ, ε and ω) to zero in the model (EquationA.1
(A.1)
(A.1) ) gives
. This, in turn, yields
The reduced version of the model (EquationA.1
(A.1)
(A.1) ), with these vaccination compartments set to zero, is henceforth called the reduced model.
3.1. Existence and asymptotic stability of equilibria
In this section, the existence and asymptotic stability of the various equilibria (carriage-free, boundary and coexistence) of the reduced model will be explored (theoretically and/or numerically). In this study, the coexistence equilibrium is defined as an equilibrium where some individuals carry serotype-i alone, some other individuals carry serotype-j alone, and some individuals are co-colonized with both serotypes.
3.1.1. Carriage-free equilibrium (CFE)
The model (EquationA.1(A.1)
(A.1) ) without vaccination has a carriage-free equilibrium (denoted by
), given by
It can be shown, using standard linearization around the CFE or the next generation operator method [Citation17, Citation18], that the associated reproduction number of the reduced model is given by
(1)
(1) where,
and
are the constituent basic reproduction numbers for serotype i and j, respectively (with each constituent reproduction number computed using the next generation operator method).
and
represent the average number of secondary carriage cases generated by a typical individual carrying the corresponding serotype(s) during their carriage period, when introduced into a completely non-colonized population in the absence of a pneumococcal vaccine [Citation3, Citation17, Citation18, Citation30]. The result below follows from Theorem 2 in [Citation17].
Theorem 3.1
Consider the special case of the reduced model. The carriage-free equilibrium of this model, given by is locally-asymptotically stable (LAS) if
and unstable if
.
The epidemiological implication of Theorem 3.1 is that, for the reduced model, a small influx of SP-colonized individuals into the population will not generate a large SP outbreak in the population if . In other words, for a small initial number of SP-colonized individuals, the SP serotypes can be effectively controlled in the population if the basic reproduction number (
) can be brought to (and maintained at) a value less than unity. To ensure that such effective control is independent of the initial number of SP-colonized individuals, a global asymptotic stability must be established for the CFE. This is explored below.
Theorem 3.2
The carriage-free equilibrium of the reduced model, is globally-asymptotically stable (GAS) in Ω (defined in (EquationB.2
(B.2)
(B.2) )) whenever
.
The proof of Theorem 3.2, based on using a comparison theorem [Citation50, Citation53], is given in Appendix C. The epidemiological implication of Theorem 3.2 is that, for the case of the reduced model, bringing (and maintaining) the associated reproduction number () to a value less than unity is necessary and sufficient for the effective control (or elimination) of both SP serotypes in the population. The result of Theorem 3.2 is numerically illustrated in Figure (a).
Figure 2. Simulation of the simplified model, showing convergence of the initial solutions (given by ) to carriage-free equilibrium. Parameter values used are:
,
, c = 4508,
,
,
,
,
and (a)
,
(so that,
,
and
), (b)
,
(so that,
,
and
), (c)
,
(so that
,
and
), (d)
,
(so that,
,
,
and
) and (e)
,
(so that,
). All the rate parameters are in years.

It is worth mentioning that heterogeneity exists in the values of based on geographical location. In particular, Gjini et al. [Citation28] estimates
for SP to be approximately 3.42 (95% CI: 2.96,3.96) in a daycare setting in Portugal. In a separate study, Gjini et al. [Citation26] estimated a range of
between 1.23 and 4.77 for SP in a similar setting across different countries. Lipsitch [Citation34] uses a range of
between 1.6 and 4 to analyse different carriage prevalence scenarios.
3.1.2. Boundary equilibria
The reduced model has two boundary equilibria (where only one of the two serotypes (the VT serotypy i or the NVT serotype j) exists at steady-state), as explored below.
(i) Serotype-i-only boundary equilibria
We explore conditions for the existence of the serotype-i-only boundary equilibria based on whether or not is greater, less or equal to unity, as below.
Case 1: . For this case, the reduced model has a unique serotype-i-only boundary equilibrium, given by
(2)
(2) where
(3)
(3) It follows from the expressions in (Equation3
(3)
(3) ) that the serotype-i-only boundary equilibrium (
) exists if
.
Case 2: . In this case, the reduced model has a serotype-i-only boundary equilibrium of the form
(4)
(4) where
(5)
(5) Since
and
, it follows from (Equation5
(5)
(5) ) that
only if
. However, since
is necessarily less than unity (see Section 2), it follows that this boundary equilibrium is not realistic for the reduced model. In other words, the reduced model does not have a serotype-i-only boundary equilibrium whenever
.
Case 3: . For this case, the reduced model has the following serotype-i-only boundary equilibrium:
(6)
(6) where
(7)
(7) It can be seen from (Equation7
(7)
(7) ) that
only if
(which is true only if
). Thus, since
is necessarily less than unity, it follows that the reduced model does not have a serotype-i-only boundary equilibrium when
.
In summary, it follows from the above analyses that the reduced model (noting that ) has a unique serotype-i-only boundary equilibrium (
), which exists only if
.
(ii) Serotype-j-only boundary equilibrium. Using the approach above, it can similarly be shown that the reduced model has the following unique serotype-j-only boundary equilibrium (which exists only when ):
(8)
(8) where
The theoretical results derived in this section are summarized below.
Theorem 3.3
The reduced model has a unique serotype-i-only (serotype-j-only) boundary equilibrium only if and no boundary equilibrium otherwise.
Asymptotic stability of boundary equilibria: special case
The asymptotic stability of the unique serotype-i-only (or serotype-j-only) boundary equilibrium of the reduced model will now be explored for a special case where the following simplifying assumptions hold:
A1: | Co-colonized individuals can only recover from both serotypes (i.e. the co-colonization ij) and not from any of the individual serotypes (i or j). That is, we set | ||||
A2: | No heterogeneity in recovery from single serotype carriage (i.e. | ||||
A3: | No heterogeneity in the modification parameter for the reduction of carriage rate with serotype k due to prior carriage of serotype k ( |
The version of the reduced model with Assumptions A1–A3 is referred to as the simplified model. It can be seen that the reproduction number of the simplified model is given by
Furthermore, the simplified model has a unique serotype-i-only (serotype-j-only) boundary equilibrium, denoted by
(
), given, respectively, by
(9)
(9) where
and
(10)
(10) with
It follows that the boundary equilibrium
(
) exists only if
(
).
To investigate the local asymptotic stability of the two boundary equilibria of the simplified model, we compute the eigenvalues of the associated Jacobian matrix evaluated at each boundary equilibrium. In particular, the eigenvalues of the Jacobian matrix (of the simplified model) evaluated at the serotype-i-only boundary equilibrium () are
from which it follows that, for
(needed for the existence of the boundary equilibrium), the first eight eigenvalues (
) are real and negative. Further,
if and only if
(and
if
. Thus, if
, the Serotype-i-only boundary equilibrium of the simplified model is locally-asymptotically stable whenever
. This boundary equilibrium is unstable if
.
Similarly, it can be shown that the eigenvalues of the Jacobian matrix (of the simplified model) evaluated at the serotype-j-only boundary equilibrium () are
from which it follows that (for
) the serotype-j-only boundary equilibrium is locally-asymptotically stable if
, and is unstable if
. These results are summarized in Theorems 3.4 and 3.5.
Theorem 3.4
The serotype-i-only boundary equilibrium () of the simplified model is LAS if
and
. This boundary equilibrium is unstable if
.
Theorem 3.5
The serotype-j-only boundary equilibrium () of the simplified model is LAS if
and
. This boundary equilibrium is unstable if
.
The theoretical results in Theorems 3.4 and 3.5 establish the conditions for the phenomenon of competitive exclusion in the reduced model. The serotype with the higher reproductive number (greater than unity) drives the other to extinction if the reproduction number of the second serotype is bounded by the threshold given in Theorems 3.4 or 3.5, whichever is applicable. These theoretical results are illustrated in Figure (b) and (c), showing initial solutions of the simplified model converging to the serotype-i-only or serotype-j-only boundary equilibrium (in line with the two theorems). It is worth mentioning that, if and
, a stable boundary equilibrium that contains individual serotype-i and individual serotype-j (without co-colonization) exists. This scenario is depicted in Figure (e).
Figure (b)–(e) show the phenomenon of competitive exclusion when one reproduction number is greater than unity and the other satisfies the inequality given in Theorems 3.4 or 3.5, whichever is applicable. Figure (a) shows the phenomenon of competitive exclusion in the absence of serotype-specific competition. For example, in the absence of competition presented by serotype i, setting in the inequality in Theorem 3.4 yields the strict threshold
, leading to the extinction of serotype j if
. This corresponds to the much narrow stability region of serotype i-only equilibrium in Figure (a). The competitive exclusion of serotype i by j can be described similarly.
Figure 3. Illustration of the results in Theorems 3.4, 3.5 and Conjecture 3.1, showing stability regions of the equilibria of the simplified model in the parameter space for various values of the serotype-specific competition parameters,
and
. The values of
and
used to compute the thresholds given in Theorems 3.4, 3.5 and Conjecture 3.1 are: (a)
, (b)
and
, (c)
and
, (d)
and
, and (e)
. In this case, a boundary equilibrium containing serotype-i and serotype-j (without co-colonization) exists and is stable. The rate parameters are in years.

3.1.3. Coexistence equilibrium of the model without vaccination: numerical illustration
Extensive numerical simulations were carried out to determine whether or not the simplified model has at least one coexistence equilibrium (i.e. an equilibrium where both the VT and NVT and/or their co-colonization exist in the population). These simulations suggest the following conjecture.
Conjecture 3.1
The simplified model has a locally-asymptotically stable coexistence equilibrium whenever the following conditions hold:
,
.
Figure (d) depicts the solution profiles generated using the simplified model showing convergence of prevalence of two hypothetical serotypes, one of which is a VT and the other NVT, and their co-colonization, to a coexistence equilibrium for the case where the hypotheses of Conjecture 3.1 are satisfied. It should be mentioned that the values of the parameters used in generating Figure were arbitrarily chosen solely to illustrate the theoretical results (Theorems 3.4 and 3.5; and Conjecture 3.1), and they may not all be within their realistic (baseline) values. Furthermore, the theoretical results given in Theorems 3.2–3.5 and Conjecture 3.1 are pictorially summarized in Figure . In particular, this figure illustrates the range of values of the constituent reproduction numbers of the simplified model () within which the equilibria of the model (i.e. the carriage-free, boundary and coexistence equilibria) are locally-asymptotically stable for various levels of the serotype-specific competition parameters (
and
). It should be recalled that setting
implies that serotype
poses no competition to the (other) serotype
. This case (with
and
) is depicted in Figure (a). Similarly, the case with
implies that current carriage with serotype
reduces the likelihood of acquiring carriage with the (other) serotype
. Furthermore, this figure shows that when the reproduction number of each of the two serotypes is less than unity, the carriage-free equilibrium becomes locally-asymptotically stable. Competitive exclusion occurs whenever the reproduction number of one serotype exceeds unity, while that of the other is less than the threshold given in Theorems 3.4 or 3.5 (in this case, the former serotype dominates and drives the latter to extinction).
The scenario where the competition parameter is reduced from the value unity to 0.1 (i.e. carriage with serotype i reduces the likelihood of acquiring carriage with serotype j by 10%), while keeping
at unity, is depicted in Figure (b). This figure shows that while the stability region for serotype i (i.e. the stability region for the serotype-i-only boundary equilibrium) increases in size, the stability region of the coexistence equilibrium narrows. Similar dynamics were observed when
is decreased from unity to 0.15, while keeping
at unity (Figure c). When both
and
are decreased, for instance to 0.1 and 0.15 respectively, the window for the stability region of the coexistence equilibrium significantly narrows (Figure d). In other words, there is now significant competition (and less synergy) between the two serotypes. Finally, when
, the coexistence equilibrium does not exist. Furthermore, in this setting, when
, a stable equilibrium that contains serotype-i and serotype-j (without co-colonization) exists when
is on the straight line
in the
-
plane (Figure e).
In summary, this study shows regions, in and
parameter space, for the elimination, or coexistence, or existence of the two individual serotypes only. The size of these regions is greatly affected by the values of the competition parameters,
and
(for serotypes i and j, respectively). In particular, the size of the stability region of the coexistence equilibrium (when each of the reproduction numbers exceed unity) decreases with decreasing values of the competition parameters (below unity).
4. Qualitative analysis of the model with vaccination
The local asymptotic stability of the CFE of the vaccination model (EquationA.1(A.1)
(A.1) ) will now be analysed. The CFE of this model is given by
where
and
. It should be noted that since
, it follows that
and
. It should be observed that
.
It can be shown, using the next generation operator method [Citation17, Citation18], that the reproduction number of the vaccination model, denoted by , is given by
(11)
(11)
The result below follows from Theorem 2 in [Citation17].
Theorem 4.1
The carriage-free equilibrium, of the vaccination model (EquationA.1
(A.1)
(A.1) ), is LAS if
and unstable if
.
The epidemiological implication of Theorem 4.1 is that, for the cohort vaccination model (EquationA.1(A.1)
(A.1) ), a small influx of SP-colonized individuals into the community will not generate a large outbreak of SP in the community if the vaccination program can bring (and maintain) the reproduction
to a value less than unity. Using the parameter values in Table , the value of the basic reproduction number of the model (EquationA.1
(A.1)
(A.1) ) is
.
5. Numerical simulations
The vaccination model (EquationA.1(A.1)
(A.1) ) will now be simulated to assess the community-wide impact of implementing the cohort vaccination strategy (using the PCV13 vaccine), as well as to measure the impact of serotype-specific competition on the burden of the VT and NVT serotypes in the community. The model will be simulated using the parameter values and ranges in Table (which are based on data for SP dynamics in the USA), unless otherwise stated. Further, for all the simulations the PCV13 vaccine will be introduced in the community when the population is already at an endemic steady state for SP. Before discussing the specifics of the two sets of simulations to be carried out, it is important to recall the concepts of cohort vaccination and serotype-specific competition, as briefly described below.
Cohort vaccination is generally defined as the immunization of the birth cohort. In the context of our study, cohort vaccination refers to the implementation of a pediatric vaccination program against SP. In the USA, anti-SP cohort vaccination consists of four PCV13 doses, administered to children at ages 2,4,6 and 12–15 months. In other words, the associated coverage parameter of the cohort vaccination model (EquationA.1(A.1)
(A.1) ), denoted by ϕ, represents the proportion of children within this age cohort (henceforth referred to as the pediatric cohort) that received all four PCV13 doses each year.
The competition parameter measures the assumed reduction in the likelihood of being colonized with serotype
after already been colonized with serotype
. Competition may manifest itself via reduction in the rate at which new serotypes are acquired or through accelerated recovery of co-colonized individuals [Citation5]. Hence, it is instructive to determine the impact of the competition parameters on the burden of the two serotypes in the community, particular as it relates to its important implications in serotype replacement. The modelling study by Bottomley et al. [Citation8] predicts the persistence of low prevalence serotypes facilitated by the vaccine through reduction in serotype-specific competition (such serotypes would otherwise have been driven to extinction). Melegaro et al. [Citation40] noted that serotypes which do not offer strong competition, but either persist longer or have high transmission potential, may replace other serotypes.
5.1. Justification of estimation of baseline values of model parameters
In this section, the estimate of the baseline values of the parameters in Table will be justified, using available data and published literature. Specifically, numerous studies have generated SP carriage prevalence data for specific age groups, communities/regions or for specific serotypes. For example, Desai et al. [Citation16] published carriage data for commonly carried SP serotypes in children in Atlanta, GA. Similarly, Balsells et al. [Citation6] presented a systematic review and meta-analysis of SP carriage data among children under 5. Grant et al. [Citation25] studied carriage prevalence in all ages in American Indians.
The recruitment rate parameter (Λ) is estimated to be per year. This is supported by CDC's provincial data for births (Vital Statistics Rapid Release) for 2018 [Citation9], which showed that there were 3,788,235 births in the USA during 2018. The natural death parameter (μ) is estimated based on the average lifespan (
) of the USA, which is approximately 78.6 years [Citation10]. Thus
per year. The carriage clearance rate parameter
) is estimated based on the data reported by Bottomley et al. [Citation8], Melegaro et al. [Citation41] and Cobey et al. [Citation13]. In particular, Bottomley et al. [Citation8] estimated the per capita carriage clearance rate (with the 95% CI) for the low, medium and high transmission serotypes to be 0.049 (0.035,0.068), 0.039 (0.031,0.049), 0.031 (0.025,0.038) per day, respectively. Since the model in the Bottomley et al. study [Citation8] does not consider co-colonization, the recovery rates are for individual serotypes (hence, the above estimates correspond to a range for
and
to be 11–18 per year).
Melegaro et al. [Citation41] estimated the carriage duration (varies by host age) to be 72 days (for 0–1 year old children), 28 days (for 2–4 years of age), 18 days (for 5–17 years of age), 17 days (for those 18 years of age and older). Thus the Melegaro et al. study suggest a range of and
to lie between 5 and 20 per year. The intrinsic durations of carriage are reported in Cobey et al. [Citation13], quoting some studies, as lying between 25 and 225 days across serotypes. Hence, the Cobey et al. study suggests a range for
and
to lie between 1.6 and 14.6 per year. Considering all these estimates, we set
to be 9.9 per year and
, the clearance rate of the NVT j, to be 8.9 per year (both of which lie within the ranges described above). Furthermore, as noted by Gaivao et al. [Citation29] (citing other studies), the clearance rate of co-colonization is lower than that of a single carriage (especially if co-colonization worsens the health of the host). Thus we estimate
(also considering the range reported in the above mentioned studies) to be 7 per year. The parameters
and
, for the proportion of co-colonized individuals who recovered from carriage with serotype i or j, respectively, are estimated to be 0.4 and 0.3.
The estimate for the contact rate parameter (c) is adapted from Prem et al. [Citation51], which reports the average number of contacts per individual (divided into 5-year age groups) per day to be 12.35, and the base-case probability of transmission of a single SP serotype per contact () is assumed to be (0.0041,0.0034). A hazard ratio of 0.6 (0.39-0.9) (i.e. a colonized individual has an average of 60% chance of getting colonized again with the same serotype) was reported in Granat et al. [Citation27]. Hence, we set the modification parameter for the reduction of carriage with serotype k (
) due to prior recovery from the same serotype to be 0.6 (0.39–0.9). The estimates for the efficacy of the PCV7 vaccine against the acquisition of carriage range from 0.5 in [Citation8] to 0.756 in [Citation41]. Rinta-Kokko et al. [Citation52] reported an efficacy of 0.6 with further serotype-specific values, namely 0.76, 0.60 and 0.39, respectively, against acquisition of the vaccine types 6B, 9V and 23F, and 0.42 (95% CI 0.24−0.56) against all vaccine-type acquisition. Consequently, we set the vaccine efficacy against carriage acquisition (ε) to be 0.7. Sutton et al. [Citation55] estimated the duration for loss of vaccine-induced protection to be 0.015 ( 0–0.03) per year. Similarly, Melegaro et al. [Citation41] estimated the duration of vaccine protection to be 8.3 years (95%CI 5−20)., while Flasche et al. [Citation23] estimated this duration (against carriage and disease) to be 5.8 (2.1–11.2) years. Consequently, following Melegaro et al. [Citation41], we set the value of the vaccine waning rate (ω) to be
per year (which also falls within the range of values in Flasche et al. [Citation23]).
The cohort vaccination coverage parameter (ϕ) is varied across parameter space (for the various scenarios we simulated in this study). Further, since vaccinated colonized individuals are expected transmit carriage at a reduced rate, in comparison to unvaccinated colonized individuals, we set the associated modification parameter () to be 0.5. The modification parameter for the assumed increase in the recovery rate of vaccinated colonized individuals, in relation to unvaccinated colonized individuals (
), is set at 2. Using data on unvaccinated children colonized with SP serotypes, Auranen et al. [Citation5] showed a 10-fold reduction in the probability of acquisition of another SP serotype in the colonized children. Consequently, we set the competition parameter for VT (
) to be 0.1 (i.e. children colonized with the VT are 90% less likely to acquire carriage with the NVT). Similarly, the competition parameter for the NVT (
) is estimated to be 0.15 (within the 95% CI (0.05 –0.15) of the estimate for
). It should be mentioned that, using data for 27 SP serotypes in Kenyan children aged 3–59 months, Lipsitch et al. [Citation36] estimated the competition parameters to be as high as 0.52 (95% CI: 0.37 –0.63).
5.2. Effect of cohort vaccination coverage on dynamics of the SP serotypes
The cohort vaccination model (EquationA.1(A.1)
(A.1) ) will now be simulated to assess the impact of the routine immunization against the vaccine serotype of SP in the cohort group. In particular, simulations will be carried out to assess the impact of variable levels of the cohort vaccination coverage on the dynamics of both the vaccine (VT) and non-vaccine (NVT) SP serotypes in the community. The simulations are aimed at exploring the impact of the cohort vaccination on curtailing the prevalence of the VT serotype (i), as well as in exploring how vaccination indirectly affects the prevalence of the NVT serotype (j). For these simulations, the vaccination model (EquationA.1
(A.1)
(A.1) ) is ran for a period of 100 years, using various values of the cohort vaccination coverage parameter (ϕ). It should be recalled that the cohort vaccination coverage parameter ϕ represents the fraction of the pediatric cohort in the community that is vaccinated. For these simulations, we consider the following three arbitrarily-chosen coverage levels of the cohort vaccination strategy implemented in the community:
Low coverage level of the cohort vaccination program: here, we set
(i.e. 40% of children in the pediatric cohort are vaccinated each year).
High coverage level of the cohort vaccination program: here, we set
(i.e. 80% of children in the pediatric cohort are vaccinated each year). It should be mentioned that this high level of cohort vaccination coverage is representative of the more realistic childhood vaccination in industrialized countries such as the USA, where the pediatric pneumococcal vaccine coverage, using PCV13, for 2017 in some US states (noticeably Arizona, Georgia, Minnesota, New Jersey and New York) was around 80% [Citation12].
Simulations of the model for the worst-case scenario (i.e. with no vaccination, so that ) show that the two streptococcus serotypes (VT and NVT) remain at constant prevalence level at 10.3% and 0.93%, respectively (Figure a), but with the prevalence of the VT exceeding that of the NVT. In this setting, the co-colonization remains constant at 0.036% (Figure a). This is owing to the fact that, for these simulations, the constituent reproduction numbers of the cohort vaccination model, are ordered as
(so that,
). When the cohort vaccination program is implemented at the aforementioned low (40%) coverage level, the simulation results obtained show prevalence in VT (NVT) decreases (increases) from the initial prevalence 10.3% (0.93%) to 1.1% (3.7%) (Figure b). Further, the prevalence of the co-colonization decreases from initial prevalence of 0.036% to 0.015% (Figure b). In this setting, initial prevalence of VT is higher than initial prevalence NVT, however, the prevalence of NVT exceeds the prevalence of VT eventually. This clearly illustrates the presence of the phenomenon of serotype replacement in the cohort vaccination model against the two serotypes of SP (EquationA.1
(A.1)
(A.1) ).
Figure 4. Effect of cohort vaccination coverage (ϕ) on the prevalence of the two SP serotyes and co-colonization. Simulations of the vaccination model (EquationA.1(A.1)
(A.1) ), showing the prevalence of VT, NVT and co-colonization and overall community-wide vaccination coverage, as a function of time for (a) no cohort vaccination(
), (b) low coverage level of cohort vaccination (
) and (c) high coverage level of cohort vaccination (
). Other parameter values used are as given in Table . The constituent reproduction numbers of the vaccination model for each of the settings (a)–(d) above are given by (a)
,
(so that,
), (b)
,
(so that,
), and (c)
,
(so that,
). The rate parameters are in years.
![Figure 4. Effect of cohort vaccination coverage (ϕ) on the prevalence of the two SP serotyes and co-colonization. Simulations of the vaccination model (EquationA.1(A.1) (Nu)′=Λ(1−ϕ)+ωNv−(λi+λj+μ)Nu,(Nv)′=Λϕ−[(1−ϵ)λi+λj+ω+μ]Nv,(Cui)′=λiNu+ηiλiRuni−(γi+θiλj+μ)Cui,(Cvi)′=(1−ϵ)λiNv+ηiλiRvni−(θvγi+θiλj+μ)Cvi,(Cuj)′=λjNu+ηjλjRunj+ωCvj−(γj+θjλi+μ)Cuj,(Cvj)′=λjNv+ηjλjRvnj−[γj+θj(1−ϵ)λi+ω+μ]Cvj,(Cuij)′=θiλjCui+θjλiCuj+θjηiλiCunij+θiηjλjCunji−(γij+μ)Cuij,(Cvij)′=θj(1−ϵ)λiCvj+θiλjCvi+θjηiλiCvnij+θiηjλjCvnji−[(θv(1−r2)+r2)γij+μ]Cvij,(Runi)′=γiCui−(ηiλi+λj+μ)Runi,(Rvni)′=θvγiCvi−(ηiλi+λj+μ)Rvni,(Runj)′=γjCuj+ωRvnj−(ηjλj+λi+μ)Runj,(Rvnj)′=γjCvj−[ηjλj+(1−ϵ)λi+ω+μ]Rvnj,(Runinj)′=γiCunji+γjCunij+(1−r1−r2)γijCuij−(ηiλi+ηjλj+μ)Runinj,(Rvninj)′=θvγiCvnji+γjCvnij+θv(1−r2)(1−r1)γijCvij−(ηiλi+ηjλj+μ)Rvninj,(Cunji)′=λiRunj+ηiλiRuninj+r2γijCuij−(γi+θiηjλj+μ)Cunji,(Cvnji)′=(1−ϵ)λiRvnj+ηiλiRvninj+r2γijCvij−(θvγi+θiηjλj+μ)Cvnji,(Cunij)′=λjRuni+ηjλjRuninj+r1γijCuij−(γj+θjηiλi+μ)Cunij,(Cvnij)′=λjRvni+ηjλjRvninj+θv(1−r2)r1γijCvij−(γj+θjηiλi+μ)Cvnij.(A.1) ), showing the prevalence of VT, NVT and co-colonization and overall community-wide vaccination coverage, as a function of time for (a) no cohort vaccination(ϕ=0), (b) low coverage level of cohort vaccination (ϕ=0.4) and (c) high coverage level of cohort vaccination (ϕ=0.8). Other parameter values used are as given in Table 2. The constituent reproduction numbers of the vaccination model for each of the settings (a)–(d) above are given by (a) Rvi=1.865, Rvj=1.72 (so that, Rv=1.865), (b) Rvi=1.799, Rvj=1.72 (so that, Rv=1.799), and (c) Rvi=1.73, Rvj=1.72 (so that, Rv=1.73). The rate parameters are in years.](/cms/asset/c8fe12c6-479c-4453-90ff-bc7566fc8341/tjbd_a_1884760_f0004_oc.jpg)
When the cohort vaccination coverage is increased to the high coverage level of the cohort vaccination program (i.e. ), the prevalence of the VT (NVT) decreases (increases) from 10.3% (0.93%) to 0.5% (4.04%) and prevalence of co-colonization decreases from 0.036% to 0.0072% (Figure c). In summary, our simulations show that, while the use of cohort vaccination always reduces the prevalence of both the VT serotype (i), such strategy always increases the prevalence of the NVT serotype (j). In this setting, the phenomenon of serotype replacement is observed where the prevalence of NVT exceeds VT.
5.3. Effect of serotype-specific competition on the effectiveness of cohort vaccination
The vaccination model (EquationA.1(A.1)
(A.1) ) is now simulated to assess the impact of the competition parameters,
and
, on the dynamics of the two serotypes in the community. This allows us to measure the impact of such competition on the effectiveness of the cohort vaccination program implemented in the community. The aim is to explore scenarios for serotype dominance and/or co-existence under this serotype competition and cohort vaccination setting. For these simulations, we consider the following three arbitrarily-chosen levels of the serotype-specific competition parameters (it should be mentioned that, in the absence of evidence of stronger or weaker competition offered by VT versus NVT, we choose to set
):
Low serotype-specific competition: here, we set
and consider no (
), low (
) and high (
coverage levels of the cohort vaccination program.
Moderate serotype-specific competition: this is defined by setting
, combined with and no (
), low (
) and high (
coverage levels of the cohort vaccination program.
High serotype-specific competition: in this case, we set
, with no (
), low (
) and high (
coverage levels of the cohort vaccination program.
Simulations for the case when the low serotype-specific competition scenario is combined with a low coverage of cohort vaccination, depicted in Figure (b), show that the prevalence of the VT, NVT and co-colonization decreases (from initial prevalence 15.1%, 11.68%, and 5.94%, respectively) to prevalence to 1.9%, 5.4% and 0.24%, respectively. When the low serotype-specific competition scenario is combined with a high coverage of cohort vaccination, the prevalence of the VT, NVT and co-colonization decrease (from initial prevalence 15.1%, 11.68% and 5.94%, respectively) to prevalence to 0.65%, 4.7% and 0.07%, respectively (Figure c). Furthermore, in this setting, for low and high coverage of cohort vaccination, the prevalence of the NVT is higher than that of the VT (even though initial prevalence of VT is higher than that of NVT), showing a serotype replacement (Figure b and c).
Figure 5. Effect of serotype-specific competition parameters, and
, and cohort vaccination (ϕ) on the prevalence of VT, NVT and co-colonization. Simulations of the vaccination model (EquationA.1
(A.1)
(A.1) ), showing the prevalence of VT, NVT and co-colonization as a function of time for (i) low serotype-specific competition:
and (a) no cohort vaccination (
), (b) low coverage level of cohort vaccination (
), and (c) high coverage level of cohort vaccination (
), (ii) moderate serotype-specific competition:
and (d) no cohort vaccination (
), (e) low coverage level of cohort vaccination (
), and (f) high coverage level of cohort vaccination (
), and (iii) low serotype-specific competition
and (g) no cohort vaccination (
), (h) low coverage level of cohort vaccination (
), (i) high coverage level of cohort vaccination (
). Other parameter values used are as given in Table . The reproduction numbers for these simulations are given as follows: (i) no cohort vaccination (
):
,
(so that,
); (ii) low coverage level of cohort vaccination (
):
,
(so that,
); (iii) high coverage level of cohort vaccination (
):
,
(so that,
). The rate parameters are in years.
![Figure 5. Effect of serotype-specific competition parameters, θi and θj, and cohort vaccination (ϕ) on the prevalence of VT, NVT and co-colonization. Simulations of the vaccination model (EquationA.1(A.1) (Nu)′=Λ(1−ϕ)+ωNv−(λi+λj+μ)Nu,(Nv)′=Λϕ−[(1−ϵ)λi+λj+ω+μ]Nv,(Cui)′=λiNu+ηiλiRuni−(γi+θiλj+μ)Cui,(Cvi)′=(1−ϵ)λiNv+ηiλiRvni−(θvγi+θiλj+μ)Cvi,(Cuj)′=λjNu+ηjλjRunj+ωCvj−(γj+θjλi+μ)Cuj,(Cvj)′=λjNv+ηjλjRvnj−[γj+θj(1−ϵ)λi+ω+μ]Cvj,(Cuij)′=θiλjCui+θjλiCuj+θjηiλiCunij+θiηjλjCunji−(γij+μ)Cuij,(Cvij)′=θj(1−ϵ)λiCvj+θiλjCvi+θjηiλiCvnij+θiηjλjCvnji−[(θv(1−r2)+r2)γij+μ]Cvij,(Runi)′=γiCui−(ηiλi+λj+μ)Runi,(Rvni)′=θvγiCvi−(ηiλi+λj+μ)Rvni,(Runj)′=γjCuj+ωRvnj−(ηjλj+λi+μ)Runj,(Rvnj)′=γjCvj−[ηjλj+(1−ϵ)λi+ω+μ]Rvnj,(Runinj)′=γiCunji+γjCunij+(1−r1−r2)γijCuij−(ηiλi+ηjλj+μ)Runinj,(Rvninj)′=θvγiCvnji+γjCvnij+θv(1−r2)(1−r1)γijCvij−(ηiλi+ηjλj+μ)Rvninj,(Cunji)′=λiRunj+ηiλiRuninj+r2γijCuij−(γi+θiηjλj+μ)Cunji,(Cvnji)′=(1−ϵ)λiRvnj+ηiλiRvninj+r2γijCvij−(θvγi+θiηjλj+μ)Cvnji,(Cunij)′=λjRuni+ηjλjRuninj+r1γijCuij−(γj+θjηiλi+μ)Cunij,(Cvnij)′=λjRvni+ηjλjRvninj+θv(1−r2)r1γijCvij−(γj+θjηiλi+μ)Cvnij.(A.1) ), showing the prevalence of VT, NVT and co-colonization as a function of time for (i) low serotype-specific competition: θi=θj=0.8 and (a) no cohort vaccination (ϕ=0), (b) low coverage level of cohort vaccination (ϕ=0.4), and (c) high coverage level of cohort vaccination (ϕ=0.8), (ii) moderate serotype-specific competition: θi=θj=0.5 and (d) no cohort vaccination (ϕ=0), (e) low coverage level of cohort vaccination (ϕ=0.4), and (f) high coverage level of cohort vaccination (ϕ=0.8), and (iii) low serotype-specific competition θi=θj=0.2 and (g) no cohort vaccination (ϕ=0), (h) low coverage level of cohort vaccination (ϕ=0.4), (i) high coverage level of cohort vaccination (ϕ=0.8). Other parameter values used are as given in Table 2. The reproduction numbers for these simulations are given as follows: (i) no cohort vaccination (ϕ=0): Rvi=1.865, Rvj=1.72 (so that, Rv=1.865); (ii) low coverage level of cohort vaccination (ϕ=0.4): Rvi=1.799, Rvj=1.72 (so that, Rv=1.799); (iii) high coverage level of cohort vaccination (ϕ=0.8): Rvi=1.73, Rvj=1.72 (so that, Rv=1.73). The rate parameters are in years.](/cms/asset/e985c952-b2e7-42aa-9601-04927b576f83/tjbd_a_1884760_f0005_oc.jpg)
When the level of serotype-specific competition is moderate (i.e. we set ) is combined with a low coverage of cohort vaccination, our simulations show that the prevalence of VT, NVT and co-colonization decreases (from initial prevalence 10.91%, 5.3%, 0.96%, respectively, to 1.4%, 4.4% and 0.09%, respectively , Figure e). If high coverage level of the cohort vaccination is used, VT decreases to 0.58%, NVT slightly decreases to 4.3% and co-colonization decreases to 0.036% (Figure f). In this setting, for low and high coverage of cohort vaccination, the prevalence of NVT is higher than that of the VT (showing a low or moderate level of serotype replacement).
It is worth noting from the above simulations for the low and moderate levels of the serotype-specific competition scenarios that the two serotypes (VT and NVT) always coexist. Further, low prevalence levels of co-colonization were observed when no or low coverage level of the cohort vaccination is used (as demonstrated in Figures a and b). It is intuitive to expect the prevalence of co-colonization when low and moderate serotype-specific competition scenarios are used. This is because these scenarios are associated with higher values of the competition parameters ( and
), which, in turn, increase the likelihood of carriage with the other serotype in individuals that were already colonized. In other words, low and moderate levels of serotype-specific competition favours coexistence, rather than serotype removal.
Although a few studies, such as those by Lipsitch et al. [Citation36] (using data from Kenya) and Bottomley et al. [Citation8] (using data from The Gambia), gave high estimates for the competition parameters (0.52 and 0.63 (0.407, 0.975), respectively), majority of the published studies on the dynamics of SP serotypes (particularly those based on using data from European countries) estimated very low values for the competition parameters. For example, using data from a longitudinal study of children in three Danish day-care centers, Auranen et al. [Citation5] estimated the competition parameters to lie in the range 0.05–0.15. Similarly, the study by Gjini et al. [Citation28], based on using data from Portugal, estimated the competition parameters to be in the range 0.04–0.09 (clearly showing a very strong serotype-specific competition in their data set). Numminen et al. [Citation49] used data from Norway to estimate the competition parameters to lie in a range of 0.06–0.14. Finally, Gjini [Citation24] estimated (based on the posterior distributions) ranges of 0.06–0.11 for Portugal, 0.03–0.05 for Norway, and 0.04–0.05 for Greece).
We also simulated the vaccination model (EquationA.1(A.1)
(A.1) ) for the case when the serotype-specific competition is high (i.e. we simulated the model (EquationA.1
(A.1)
(A.1) ) with
). For low coverage of cohort vaccination, the results obtained show that the prevalence of the VT (NVT) decreases (increases) from initial prevalence 10.12% (1.3%) to 1.14% (3.8%) (Figure h). For this setting, the prevalence of the co-colonization decreases from initial prevalence 0.086% to 0.025%. For high coverage level of the cohort vaccination, VT (NVT) decreases (increases) to 0.5% (4.1%) and the co-colonization decreases to 0.012% (Figure i). In this case, the prevalence of NVT much higher than that of the VT (clearly demonstrating serotype replacement).
In summary, the simulations in Figure show the following main results:
When there is no cohort vaccination, the prevalence of co-colonization decreases as the level of the serotype-specific competition increases. Moreover, the prevalence of co-colonization decreases as level of cohort vaccination coverage increases.
The values of the constituent reproduction numbers (
and
) of the vaccination model (EquationA.1
(A.1)
(A.1) ) do not change with changing values of the competition parameters (
and
; since these parameters do not appear in the expressions of the constituent reproduction numbers). This is because the two parameters are associated with co-colonization, which is secondary (and not primary) infection. Hence, they do not appear in any of the next generation matrices (used to compute the reproduction number of the vaccination model (EquationA.1
(A.1)
(A.1) )).
For each level of the cohort vaccination program (low or high), the total prevalence (the sum of the prevalence of VT, NVT and co-colonization) remains the same (at all time t) for all levels of the serotype-specific competition.
The prospect of vaccination-induced serotype replacement (where the NVT replaces the VT, but without causing it to go extinct) is significantly increased with increasing level of both serotype-specific competition and cohort vaccination coverage.
Discussion and conclusion
Pnuemococcal disease (PD), a major respiratory disease caused by nasopharyngial carriage of any of the over 90 serotypes of the bacterium Streptococcus pneumoniae (SP), continues to cause major public health burden globally. The populations at highest risk of PD, namely children under the age of 5 and adults of age 65 or older, continue to suffer high mortality and morbidity annually. Vaccination, using any of the currently available pneumococcal conjugate (PCV) and polysaccharide (PPV) vaccines, is the most effective intervention against PD, and many countries around the world have included pneumococcal vaccine in their regular age-based immunization programs. Each vaccine is serotype specific. That is, each vaccine targets specific SP serotypes, with varying efficacy across the targeted serotypes. The non-vaccine SP serotypes (NVT) have emerged (with increasing prevalence) due to the lack of resource competition with the vaccine serotype (VT), owing to the fact that the incidence and prevalence of the latter have greatly declined following the introduction of the aforementioned vaccine into human populations. This dynamic phenomenon, of the increase in the prevalence of NVT (following the dramatic decline of the VT due to mass immunization), is called serotype replacement.
This study presented a novel mathematical model for gaining insight into the population-level impact of pneumococcal vaccination on the transmission dynamics of SP carriage in a cohort. The resulting dynamic model, which monitors the temporal dynamics of two SP serotypes (VT and NVT), takes the form of a deterministic system of nonlinear differential equations. The model allowed for the assessment of the carriage history of the two SP serotypes in the presence of a vaccine. The SP carriage history was needed to enable the proper accounting of the development of natural immunity against SP serotypes due to past exposure. This immunity impacts subsequent colonizations after recovery. The model allowed for co-colonization with the two serotypes, which was assumed to occur only via the sequential carriage of individuals already colonized with the other serotype (i.e. the model does not allow for simultaneous carriage with the two SP serotypes).
The special case of the model, in the absence of vaccination (termed the reduced model), was rigorously analysed to gain insight into its dynamical features. Our analyses revealed that the carriage-free equilibrium of the reduced model is globally-asymptotically stable whenever the associated basic reproduction number of the model (denoted by ) is less than unity. The epidemiological implication of this result is that the two SP serotypes can be eliminated from the cohort if the reproduction threshold (
) can be brought to (and maintained at) a value less than unity. In other words, this study shows that bringing (and maintaining) the threshold quantity
to a value less than unity is necessary and sufficient for the elimination of the two serotypes in the cohort. To the authors' knowledge, this may be the very first study to establish necessary and sufficient conditions for the theoretical elimination of the two SP serotypes in a cohort, by proving the global asymptotic stability property of the associated carriage-free equilibrium of the model. Some modelling studies (e.g. [Citation28]) have established local stability of the CFE, and, in the case of two serotypes, local stability of the boundary- and coexistence equilibria. It is worth mentioning, however, that the model in [Citation28] also considers the possibility of both serotypes being VT or both NVT.
Conditions for the existence of the boundary equilibria (corresponding to the VT-only or NVT-only) and a coexistence equilibrium (where both the VT and NVT, and/or the co-colonization co-exist) of the reduced model (without vaccination) were theoretically derived. The VT-only and NVT-only boundary equilibria were shown to be locally-asymptotically stable under certain conditions. The implication of this result is that, for the version of the model without vaccination, the epidemiological phenomenon of competitive exclusion can occur if one serotype has a reproduction number greater than unity, and the other less than a certain threshold (in this case, the former drives the latter serotype to extinction). Furthermore, when the two reproduction numbers of the reduced model are both greater than unity, the two serotypes (and their co-colonization) may co-exist. In this case, the serotype with the higher reproduction number dominates the other serotype (but does not drive it to extinction). This gives rise to the phenomenon of serotype replacement.
Our theoretical analysis, coupled with extensive numerical simulations, further showed that the level of serotype-specific competition (measured by the parameters and
) plays an important role in the dynamics of the SP serotypes and the co-colonization in the cohort. Specifically, we showed that the size of the stability regions for the coexistence equilibrium of the reduced model significantly decreases with increasing level of serotype-specific competition. In fact, the stability region disappears for high serotype-specific competition. For such high competition, the reduced model no longer has a coexistence equilibrium (only the carriage-free and the two boundary equilibria exist in this case). Thus this study shows that the mechanism of serotype-specific competition has the dual role of facilitating or impeding serotype coexistence, depending on the strength or level of the competition (lower level of competition supports coexistence, while higher competition level impedes coexistence).
The full model, which incorporates the routine pediatric/cohort vaccination (which entails the administration of four PCV13 doses, given to children in the pediatric cohort), was also rigorously analysed. In particular, it was shown that the carriage-free equilibrium of the full model was also locally-asymptotically stable whenever the associated reproduction number of the full model (denoted by ) is less than unity.
The model developed in this study can be extended in numerous ways. For instance, age structure and pneumococcal disease manifestations, which are essential for analysing the effectiveness of age-based immunization programs and formulating effective policy recommendations to reduce disease burden resulting from SP carriage, can be explicitly incorporated into the model. The model developed in this study is based on assuming a well-mixed population. This simplifying assumption (needed for mathematical tractability) constitutes a limitation of the model, and the results we presented could very well be affected if this assumption is relaxed. Our study can be extended by relaxing the homogenous-mixing assumption, allowing, instead, a heterogenous-mixing (i.e. preferential mixing) framework in a population that is also stratified by age. It is important to optimize the trade-off between vaccine coverage of the number of pathogenic serotypes (hence, maximizing the beneficial effects) and the risk of increase in the pneumococcal disease (PD) burden following the replacement of the VT with the NVT [Citation35]. Hence, there is a need to explicitly include the dynamics of PD in the model (because the impact of vaccination on PD will vary with varying serotype distributions post-vaccination [Citation35]). The dosing schedules of the pneumococcal vaccination program can also be explicitly incorporated in the model, in addition to accounting for the related costs and vaccine coverage/compliance associated with the vaccine dosing schedules (some pneumococcal vaccine modelling studies, such as those in [Citation14, Citation15, Citation19, Citation55, Citation68], have accounted for dosing schedule and its overall impact on the dynamics of the disease). The suggested extensions can further contribute to the efforts to realistically assess the community-wide effectiveness of pneumococcal vaccination programs.
Acknowledgments
One of the authors (ABG) acknowledge the support, in part, of the Simons Foundation (Award 585022) and the National Science Foundation (Award
1917512). The authors are grateful to the anonymous reviewers for their constructive comments.
Disclosure statement
No potential conflict of interest was reported by the author(s).
Additional information
Funding
References
- E.M.C. D'Agata, G.F. Webb, and J. Pressley, Rapid emergence of co-colonization with community-acquired and hospital-acquired methicillin-resistant staphylococcus aureus strains in the hospital setting, Math. Model. Nat. Phenom. 5(3), pp. 76–93.
- R. Austrian, Some aspects of the pnuemococcal carrier state, J. Antimicrob. Chemother. 18(Suppl A) (1986), pp. 35–45.
- R.M. Anderson and R.M. May, Infectious Diseases of Humans, Oxford University Press, New York, 1991.
- K. Atkins, E.I. Lafferty, S.R. Deeny, N.G. Davies, J.V. Robotham, and M. Jit, Use of mathematical modelling to assess the impact of vaccines on antibiotic resistance, Lancet Infect. Dis. 18 (2018), pp. e204–13.
- K. Auranen, J. Mehtala, A. Tanskanen, and M.S. Kaltoft, Between-strain competition in acquisition and clearance of pneumococcal carriage: Epidemiologic evidence from a longitudinal study of day-care children, Am. J. Epidemiol. 171(2) (2010), pp. 169–176.
- E. Balsells, et al. The relative invasive disease potential of Streptococcus pneumoniae among children after PCV introduction: A systematic review and meta-analysis, J. Infect. 77 (2018), pp. 368–378.
- S.D. Brugger, L.J. Hataway, and K. Mühlemann, Detection of streptococcus pneumoniae strain cocolonization in the nasopharynx, J. Clin. Microbiol. 47 (2009), pp. 1750–1756.
- C. Bottomley, A. Roca, P.C. Hill, B. Greenwood, and V. Isham, A mathematical model of serotype replacement in pneumococcal carriage following vacciation, J. R. Soc. Interfact. 10 (2013), pp. 20130786. http://dx.doi.org/10.1098/rsif.2013.0786.
- Centers for Disease Control and Prevention. Vital Statistics Rapid Release: Births: Provisional Data for 2018. Available at https://www.cdc.gov/nchs/data/vsrr/vsrr-007-508.pdf (accessed Jan 3 2020).
- Centers for Disease Control and Prevention. National Center for Health Statistics: Mortality in the United States, preprint (2017). Available at https://www.cdc.gov/nchs/products/databriefs/db328.htm (accessed Jan 2 2020).
- Centers for Disease Control and Prevention. 2017. Active Bacterial Core Surveillance Report, Emerging Infections Program Network, Streptococcus pneumoniae, preprint (2017). Available at via the internet: http://www.cdc.gov/abcs/reportsfindings/survreports/spneu17.pdf (accessed May 14 2019).
- Centers for Disease Control and Prevention. ChildVaxView: 2002 through 2017 Childhood Pneumococcal Conjugate Vaccine (PCV) Coverage Trend Report, Available at https://www.cdc.gov/vaccines/imz-managers/coverage/childvaxview/data-reports/pcv/trend/index.html (accessed Jan 7 2020).
- S. Cobey and M. Lipsitch, Niche and neutral effects of acquired immunity permit coexistence of pneumococcal serotypes, Science 335 (2012), pp. 1376–1380.
- Y.H. Choi, M. Jit, N. Gay, N. Andrews, P.A. Waight, A. Melegaro, R. Georege, and E. Miller, 7-Valent pneumococcal conjugate vaccination in England and Wales: Is it still beneficial despite high levels of serotype replacement?, PLoS One 6(10) (2011), pp. e26190. doi:10.1371/journal.pone.0026190.
- Y.H. Choi, M. Jit, S. Flasche, N. Gay, and E. Miller, Mathematical modelling long-term effects of replacing Prevnar7 with Prevnar13 on invasive pneumococcal diseases in England and Wales, PLoS One 7(7) (2012), pp. e39927. doi:10.1371/journal.pone.0039927..
- A.P. Desai, et al. Decline in pneumococcal nasopharyngeal carriage of vaccine serotypes after the introduction of the 13-valent pneumococcal conjugate vaccine in children in Atlanta Georgia, Pediatr. Infect. Dis. J. 34 (2015), pp. 1168–1174.
- P. Driessche and J. Watmough, Reproduction numbers and sub-threshold endemic equilibria for compartmental models of disease transmission, Math. Biosci. 180 (2002), pp. 29–48.
- O. Diekmann, J.A.P. Heesterbeek, and J.A.J. Metz, On the definition and the computation of the basic reproduction ratio Ro in models for infectious diseases in heterogeneous populations, J. Math. Biol.28 (1990), pp. 365–382.
- E. Delgleize, O. Leeuwenkamp, E. Theodorou, and N. Van de Velde, Cost effectiveness analysis of routine pneumococcal vaccination in the UK: A comparison of the PHiD-CV vaccine and the PCV-13 vaccine using a Markov model, BMJ Open 6 (2016), pp. e010776. doi:10.1136/bmjopen-2015-010776..
- E.H. Elbasha and A. Galvani, Vaccination against multiple HPV types, Math. Biosci. 197 (2005), pp. 88–117.
- S. Flasche, W.J. Edmunds, E. Miller, D. Goldblatt, C. Robertson, and Y.H. Choi, The impact of specific and non-specific immunity on the ecology of Streptococcus pneumoniae and the implications for vaccination, Proc. R. Soc. B 280 (2013), pp. 20131939. http://dx.doi.org/10.1098/rspb.2013.1939.
- G. Falkenhorst, C. Remschmidt, T. Harder, O. Wichmann, S. Glodny, E. Hummers-Pradier, T. Ledig, and C. Bogdan, Background paper to the updated pneumococcal vaccination recommendation for older adults in Germany, Bundesgesundheitsblatt 59 (2016), pp. 1623–1657. DOI 10.1007/s00103-016-2466-9.
- S. Flasche, J. Ojal, O.L.P de Waroux, M. Otiende, K.L. O'Brien, M. Kiti, D.J. Nokes, W.J. Edmunds, and J.A.G. Scott, Assessing the efficiency of catch-up campaigns for the introduction of pneumococcal conjugate vaccine: A modelling study based on data from PCV10 introduction in Kilifi, Kenya, BMC Med. 15(1) (2017), pp. 113.
- E. Gjini, Geographic variation in pneumococcal vaccine efficacy estimated from dynamic modeling of epidemiological data post-PCV7, Sci. Rep. 7 (2017), pp. 3049. DOI:10.1038/s41598-017-02955-y.
- L.R. Grant, et al. Impact of the 13-valent pneumococcal conjugate vaccine on pneumococcal carriage among American indians, Pediatr. Infect. Dis. J. 35(8) (2016), pp. 907–914.
- E. Gjini and M.G.M. Gomes, Expanding vaccine efficacy estimation with dynamic models fitted to cross-sectional prevalence data post-licensure', Epidemics 14 (2016), pp. 71–82.
- S.M. Granat, J. Ollgren, E. Herva, Z. Mia, K. Auranen, and P.H. Mäkelä, Epidemiological evidence for serotype-independent acquired immunity to pneumococcal carriage, J. Infect. Dis. 200 (2009), pp. 99–106.
- E. Gjini, C. Valente, R. Sá-Leão, and M.G.M. Gomes, How direct competition shapes coexistence and vaccine effects in multi-strain pathogen systems, J. Theor. Biol. 388 (2016), pp. 50–60.
- M. Gaivão, F. Dionisio, and E. Gjini, Transmission fitness in co-colonization and the persistence of bacterial pathogens, Bull Math Biol 79 (2017), pp. 2068–2087.
- H.W. Hethcote, The mathematics of infectious diseases, SIAM Rev. 42(4) (2000), pp. 599–653.
- W.P. Hausdorff and W.P. Hanage, Interim results of an ecological experiment – conjugate vaccination against the pneumococcus and serotype replacement, Hum. Vaccin Immunother. 12 (2016), pp. 358–74.
- M. Habib, B.D. Porter, and C. Satzke, Capsular serotyping of streptococcus pneumoniae using the quellung reaction, J. Vis. Exp. JoVE (2014). doi:10.3791/51208.
- M. Iannelli, M. Martcheva, and X. Li, Strain replacement in an epidemic model with super-infection and perfect vaccination, Math. Biosci. 195 (2005), pp. 23–46.
- M. Lipsitch, Vaccination against colonizing bacteria with multiple serotypes, Proc. Natl. Acad. Sci. USA 94 (1997), pp. 6571–6576.
- M. Lipsitch, Bacterial vaccines and serotype replacement: Lessons from Haemophilus influenzae and prospects for Streptococcus pneumoniae, Emerg. Infect. Dis. 5(3) (1999), pp. 336–45.
- M. Lipsitch, et al. Estimating rates of carriage acquisition and clearance and competitive ability for pneumococcal Serotypes in Kenya with a Markov transition model, Epidemiology 23(4) (2012), pp. 510.
- A. Lochen and R.M. Anderson, Dynamic transmission models and economic evaluations of pneumococcal conjugate vaccines: A quality appraisal and limitations, Clin. Microbiol. Inf. (2019). https://doi.org/10.1016/j.cmi.2019.04.026.
- C.D.S. Lefebvre, A. Terlinden, and B. Standaert, Dissecting the indirect effects caused by vaccines into the basic elements, Hum. Vaccin. Immunother. 11(9) (2015), pp. 2142–2157. doi:10.1080/21645515.2015.1052196.
- I.J. Myung, Tutorial on maximum likelihood estimation, J. Math. Psychol. 47 (2003), pp. 90–100.
- A. Melegaro, Y. Choi, R. Pebody, and N. Gay, Pneumococcal carriage in United Kingdom families: Estimating serotype-specific transmission parameters from longitudinal data, Am. J. Epidemiol. 166 (2007), pp. 228–235.
- A. Melegaro, Y.H. Choi, R. George, W.J. Edmunds, E. Miller, and N.J. Gay, Dynamic models of pneumococcal carriage and the impact of the heptavalent pneumococcal conjugate vaccine on invasive pneumococcal disease, BMC Infect. Dis. 10 (2010), pp. 90.
- E. Miller, N.J. Andrews, P.A. Waight, M.P.E. Slack, and R.C. George, Herd immunity and serotype replacement 4 years after seven-valent pneumococcal conjugate vaccination in England and Wales: An observational cohort study, Lancet Infect. Dis. 11 (2011), pp. 760–68. doi:10.1016/S1473-3099(11)70090-1.
- J. Mehtälä, M. Antonio, M.S. Kaltoft, K.L. O'Brien, and K. Auranen, Competition between streptococcus pneumoniae strains: Implications for vaccine-induced replacement in colonization and disease, Epidemiology 24(4) (2013), pp. 522–29.
- G. Masala, M. Lipsitch, C. Bottomley, and S. Flasche, Exploring the role of competition induced by non-vaccine serotypes for herd protection following pneumococcal vaccination, J. R. Soc. Interface 14 (2017), pp. 20170620. http://dx.doi.org/10.1098/rsif.2017.0620.
- A. Matanock, G. Lee, R. Gierke, M. Kobayashi, A. Leidner, and T. Pilishvili, Use of 13-valent pneumococcal conjugate vaccine and 23-valent pneumococcal polysaccharide vaccine among adults aged ≥65 years: Updated recommendations of the advisory committee on immunization practices, Morb. Mortal. Wkly. Rep. 68 (2019), pp. 1069–1075.
- Merck Sharp & Dohme Corp. A Study to Evaluate the Safety, Tolerability, and Immunogenicity of V114 Followed by PNEUMOVAXTM23 in Healthy Adults 50 Years of Age or Older (V114-016/PNEU-PATH), preprint (2018). Available at https://clinicaltrials.gov/ct2/show/NCT03480763?term=NCT03480763&rank=1.
- Merck Sharp & Dohme Corp. A Study to Evaluate the Safety, Tolerability, and Immunogenicity of V114 Followed by PNEUMOVAXTM23 in Adults Infected With Human Immunodeficiency Virus (HIV) (V114-018/PNEU-WAY), preprint (2019). Available at https://clinicaltrials.gov/ct2/show/NCT03480802.
- Pfizer. Trial to Evaluate the Safety and Immunogenicity of a 20-valent Pneumococcal Conjugate Vaccine in Pneumococcal Vaccine-naive Adults, preprint (2019). Available at https://clinicaltrials.gov/ct2/show/NCT03760146?term=NCT03760146&rank=1.
- E. Numminen, L. Cheng, M. Gyllenberg, and J. Corander, Estimating the transmission dynamics of Streptococcus pneumoniae from strain prevalence data, Biometrics 69(3) (2013), pp. 748–57.
- K. Okuneye and A.B. Gumel, Analysis of a temperature and rainfall-dependent model for malaria transmission dynamics, Math. Biosci. 287 (2017), pp. 72–92.
- K. Prem, A.R. Cook, and M. Jit, Projecting social contact matrices in 152 countries using contact surveys and demographic data, PLoS Comput. Biol. 13(9) (2017), pp. e1005697. https://doi.org/10.1371/journal.pcbi.1005697.
- H. Rinta-Kokko, R. Dagan, N. Givon-Lavi, and K. Auranen, Estimation of vaccine efficacy against acquisition of pneumococcal carriage, Vaccine 27 (2009), pp. 3831–3837.
- H.L. Smith, Monotone dynamical systems: An introduction to the theory of competitive and cooperative systems, American Math. Soc., Providence, (1995).
- S.J. Snedecor, D.R. Strutton, V. Ciuryla, E.J. Schwartz, and M.F. Botteman, Transmission-dynamic model to capture the indirect effects of infant vaccination with prevnar (7-valent pneumococcal conjugate vaccine (pCV7) in older populations, Vaccine 27 (2009), pp. 4694–4703.
- K.L. Sutton, H.T. Banks, and C. Castillo-Chavez, Public vaccination policy using an age-structured model of pneumococcal infection dynamics, J. Biol. Dyn. 4(2) (2010), pp. 176–195.
- B. Simell, K. Auranen, H. Käyhty, D. Goldblatt, R. Dagan, and K.L. O'Brien, The fundamental link between pneumococcal carriage and disease, Expert Rev. Vaccines 11(7) (2012), pp. 841–855.doi:10.1586/erv.12.53.
- A.M.C. Sartori, L.M. Rozman, T.C. Decimoni, R. Leandro, H.M.D. Novaes, and P.C. de Soárez, A systematic review of health economic evaluations of vaccines in Brazil, Hum. Vaccines Immunother.13(6) (2017), pp. 1454–1465.
- L. Temime, D. Guillemot, and P.Y. Boëlle, Short- and long-term effects of pneumococcal conjugate vaccination of children penicillin resistance, Antimicrob. Agents Chemother. 48(6) (2004), pp. 2206–2213.
- L. Temime, P.Y. Boëlle, AlJ. Valleron, and D. Guillemot, Penicillin-resistant pneumococcal meningitis: High antibiotic exposure impedes new vaccine protection, Epidemiol. Infect. 133 (2005), pp. 493–501.
- P. Turner, J. Hinds, C. Turner, A. Jankhot, K. Gould, S.D. Bentley, F. Nosten, and D. Goldblatt, Improved detection of nasopharyngeal cocolonization by multiple pneumococcal serotypes by use of latex agglutination or molecular serotyping by microarray, J. Clin. Microbiol. 49 (2011), pp. 1784–1789.
- D. Thorrington, N. Andrews, J. Stowe, E. Miller, and A.J. van Hoek, Elucidating the impact of the pneumococcal conjugate vaccine programme on pneumonia, sepsis and otitis media hospital admissions in England using a composite control, BMC Med. 16 (2018), pp. 13.
- T. van Effelterre, M.R. Moore, F. Fierens, C.G. Whitney, L. White, S.I. Pelton, and W.P. Hausdorff, A dynamic model of pneumococcal infection in the United States: Implications for prevention through vaccination, (2010).
- A.J. van Hoek, N.J. Andrews, P.A. Waight, R.C. George, and E. Miller, Effect of serotype on focus and mortality of invasive pneumococcal disease: Coverage of different vaccines and insight into non-vaccine serotypes, PLoS One 7(7) (2012), pp. e39150. doi:10.1371/journal.pone.0039150.
- D. Weinberger, R. Malley, and M. Lipsitch, Serotype replacement in disease after pneumococcal vaccination, Lancet 378 (2011), pp. 1962–1973. DOI:10.1016/S0140-6736(10)62225-8.
- D.B. Wu, C. Chang, Y. Huang, Y. Wen, C. Wu, and C.S. Fann, Cost-effectiveness analysis of pneumococcal conjugate vaccine in Taiwan: A transmission dynamic modeling approach, Value Health 15 (2012), pp. S15–S19.
- A.L. Wyllie, M.L.J.N. Chu, M.H.B. Schellens, J.V.E. Gastelaars, M.D. Jansen, A. van der Ende, D.Bogaert, E.A.M. Sanders, and K. Trzciński, Streptococcus pneumoniae in saliva of dutch primary school children, PloS One 9(7) (2014), pp. e102045.
- B. Wahl, K.L. O'Brien, A. Greenbaum, A. Majumder, L. Liu, and Y. Chu, et al. Burden of streptococcus pneumoniae and Haemophilus influenzae type b disease in children in the era of conjugate vaccines: Global, regional, and national estimates for 2000-15, Lancet Glob. Health 6 (2018), pp. e744–e757.
- M. Wasserman, M.G. Palacios, A.G. Grajales, F.B. Baez-Revueltas, M. Wilson, C. McDade, and R.Farkouh, Modeling the sustained use of the 13-valent pneumococcal conjugate vaccine compared to switching to the 10-valent vaccine in Mexico, Hum. Vaccines Immunother. 15(3) (2018), pp. 560–569. doi:10.1080/21645515.2018.1516491.
Appendices
Appendix. Model equations
Recall that, in the formulation described in Section 2, the total human population at time t, denoted by , is subdivided into the subpopulations of unvaccinated non-colonized (
, unvaccinated colonized with serotype i (
, unvaccinated colonized with serotype j (
, unvaccinated co-colonized with serotypes i and j (
, unvaccinated who recovered from carriage and having immunity against serotype i (
), unvaccinated recovered from carriage and having immunity against serotype j (
) and unvaccinated who recovered from, and have natural immunity against, serotypes i and j (
), those vaccinated non-colonized (
), vaccinated colonized with serotype i (
, vaccinated colonized with serotype j (
, vaccinated co-colonized with serotypes i and j (
), recovered from (and having natural immunity against) serotype i (
, recovered from serotype j and having vaccine-derived immunity against serotype i (
, recovered from (and having immunity against) serotypes i and j (
, vaccinated and colonized with serotype i with natural immunity against serotype j (
) and vaccinated colonized with serotype j having immunity against serotype i (
).
Individuals acquire carriage with serotype i, j or the co-colonization at the rates and
, respectively given by
where c is the effective contact rate,
(with k = i, j) is the probability of carriage with serotype i or j, respectively, per contact. Similarly, the parameter
accounts for the assumed reduced infectiousness of vaccinated colonized individuals, in comparison to the corresponding unvaccinated colonized individuals.
The model for the dynamics of two SP serotypes, in the presence of a routine cohort vaccination program, is given by the following deterministic system of nonlinear differential equations (where a prime represents differentiation with respect to time t)
(A.1)
(A.1)
The flow diagram of the model (EquationA.1(A.1)
(A.1) ) is depicted in Figure , and its associated state variables and parameters are described in Tables and , respectively.
Appendix. Basic qualitative properties of the model
The basic qualitative features of the model (EquationA.1(A.1)
(A.1) ) will now be explored, aimed at determining whether or not the model is epidemiologically well-posed (so that it can be used to realistically assess the population-wide impact of the intervention strategy considered in the study). First of all, since the model monitors the spread of a disease in human populations, it is necessary that all its state variables are non-negative for all time t. Consequently, it is instructive to determine whether or not the model (EquationA.1
(A.1)
(A.1) ) preserves this (inherent) positivity property of the phenomenon (disease transmission) being modeled. This is explored below, by showing that the model will generate non-negative solutions for all non-negative initial solutions.
It is convenient to define the following feasible region for the model (EquationA.1(A.1)
(A.1) ):
(B.2)
(B.2) We claim the following result.
Theorem B.1
The region Ω is bounded, positively-invariant and attracting for the model system (EquationA.1(A.1)
(A.1) ).
Proof.
Adding all the equations of the model (EquationA.1(A.1)
(A.1) ) gives
from which it follows that,
It should be recalled that
in Ω. Hence, if
, it follows then that
for all
. On the other hand, if
, then
, as
. Thus the bounded set Ω is positively-invariant and attracts all non-negative initial solutions of the model (EquationA.1
(A.1)
(A.1) ). Thus the model is mathematically and epidemiologically well-posed.
Appendix. Proof of theorem 3.2
Proof.
Consider the reduced model with . The proof is based on using comparison theorem [Citation50, Citation53]. Denoting the sum of all the equations of the reduced model by
, it follows that
. Thus
, as
. Consequently, from now on, the limiting system with
, where
, will be used in the analysis.
The equations for the infected components of the reduced model can be written in the form:
(C.3)
(C.3) where the next generation matrices, F and V, and the non-negative matrix, S, are given, respectively, by
and,
with
. Since
, in
, it follows that matrix S is non-negative. Hence, Equation (EquationC.3
(C.3)
(C.3) ) can reduced to the following linear inequality:
(C.4)
(C.4) Since all the eigenvalues of F−V have negative real part for
(from the local asymptotic stability result in Theorem 3.1), it follows that the linearized system of differential inequality (EquationC.4
(C.4)
(C.4) ) is asymptotically stable if
. That is,
Substituting
,
,
,
,
, into the equations of the reduced model gives:
Hence, the carriage-free equilibrium,
, of the reduced model is GAS in
if
.
Appendix. Model fitting to carriage prevalence data
The vaccination model (EquationA.1(A.1)
(A.1) ) will be fitted to the carriage prevalence data of serotypes 19A and 35B, generated from a cohort of 6–59 months children in Atlanta, GA, who were vaccinated with PCV13 (which targets Serotype 19A) during the period 2010–2013 [Citation16]. The Desai et al. study [Citation16] reported the prevalence levels of the two serotypes in six study periods, 6 months apart (from July 2010 to June 2013). It showed a significant decline in the prevalence of the VT 19A (from 7.3% in the first study period where 32 children were identified as carriers of serotype 19A among a total sample of 441 children, to 1.1% in the sixth study period where serotype 19A was present in 3 children among a sample of 282 children). It also showed a noticeable increase in the prevalence of the NVT 35B (from 2.5% in the first study period where 11 of 441 children were colonized with serotype 35B to 8.9% where 25 out of 282 children carried serotype 35B although the rise in the first 5 study periods was more moderate, for example 4.6% in the fifth study period). We chose these two serotypes to illustrate the predictive value of the current model in the context of serotype replacement. It should be emphasized that these two particular serotypes were randomly chosen, not based on any known interactions of the two, so that the increase in the prevalence of serotype 35B may, or may not, be directly linked with the decline in the VT 19A. The model is fitted to the prevalence data (using the maximum likelihood estimation method [Citation39]), for the six study periods, to estimate the parameters
,
,
,
,
and
. For the data fitting, the serotype distribution for the first study period (in [Citation16]) is used as initial condition for the model simulations (to fit the data). Furthermore, the sample size in the first study period is 441 (7.3% are 19A carriers, 2.5% are 35B carriers, and the rest are considered as non-carriers of 19A and 35B). For this cohort, 70% are vaccinated, while the remaining 30% are un-vaccinated [Citation16].
The following set of initial conditions were chosen for the model simulations: ,
,
,
=11,
,
,
,
,
,
,
,
,
,
,
,
,
and
. The end of the first study period was considered as initial time for the start of the simulations. Since the data set is for a closed population, we set the demographic parameters of the model (Λ and
to zero in these simulations. The model is simulated, for a period of 2.5 years, using the parameter values in Table . Figure depicts the prevalence data from the Desai et al. [Citation16] and the data generated from the model (EquationA.1
(A.1)
(A.1) ), from which it follows that the best estimates of the fitted parameters are
,
,
,
,
and
, respectively.
Figure A1. Data fitting of the model (EquationA.1(A.1)
(A.1) ) using the prevalence data for serotypes 19A (VT) and 35B (NVT) generated from a cohort of 6–59 months children in Atlanta, GA, in 6 study periods, 6 months apart, from July 2010 to June 2013 (the end of the first study period is considered as the initial time for the start of the simulations), as reported in [Citation16].
![Figure A1. Data fitting of the model (EquationA.1(A.1) (Nu)′=Λ(1−ϕ)+ωNv−(λi+λj+μ)Nu,(Nv)′=Λϕ−[(1−ϵ)λi+λj+ω+μ]Nv,(Cui)′=λiNu+ηiλiRuni−(γi+θiλj+μ)Cui,(Cvi)′=(1−ϵ)λiNv+ηiλiRvni−(θvγi+θiλj+μ)Cvi,(Cuj)′=λjNu+ηjλjRunj+ωCvj−(γj+θjλi+μ)Cuj,(Cvj)′=λjNv+ηjλjRvnj−[γj+θj(1−ϵ)λi+ω+μ]Cvj,(Cuij)′=θiλjCui+θjλiCuj+θjηiλiCunij+θiηjλjCunji−(γij+μ)Cuij,(Cvij)′=θj(1−ϵ)λiCvj+θiλjCvi+θjηiλiCvnij+θiηjλjCvnji−[(θv(1−r2)+r2)γij+μ]Cvij,(Runi)′=γiCui−(ηiλi+λj+μ)Runi,(Rvni)′=θvγiCvi−(ηiλi+λj+μ)Rvni,(Runj)′=γjCuj+ωRvnj−(ηjλj+λi+μ)Runj,(Rvnj)′=γjCvj−[ηjλj+(1−ϵ)λi+ω+μ]Rvnj,(Runinj)′=γiCunji+γjCunij+(1−r1−r2)γijCuij−(ηiλi+ηjλj+μ)Runinj,(Rvninj)′=θvγiCvnji+γjCvnij+θv(1−r2)(1−r1)γijCvij−(ηiλi+ηjλj+μ)Rvninj,(Cunji)′=λiRunj+ηiλiRuninj+r2γijCuij−(γi+θiηjλj+μ)Cunji,(Cvnji)′=(1−ϵ)λiRvnj+ηiλiRvninj+r2γijCvij−(θvγi+θiηjλj+μ)Cvnji,(Cunij)′=λjRuni+ηjλjRuninj+r1γijCuij−(γj+θjηiλi+μ)Cunij,(Cvnij)′=λjRvni+ηjλjRvninj+θv(1−r2)r1γijCvij−(γj+θjηiλi+μ)Cvnij.(A.1) ) using the prevalence data for serotypes 19A (VT) and 35B (NVT) generated from a cohort of 6–59 months children in Atlanta, GA, in 6 study periods, 6 months apart, from July 2010 to June 2013 (the end of the first study period is considered as the initial time for the start of the simulations), as reported in [Citation16].](/cms/asset/0b869ea2-45d4-4860-8d1d-0be6d43c7206/tjbd_a_1884760_f0006_oc.jpg)
The model (EquationA.1(A.1)
(A.1) ) will be simulated using the baseline and fitted values of the parameters (
,
,
,
,
, and
),
, and other parameter given in Table , to monitor the temporal dynamics of the (prevalence of the) serotypes 19A and 35B in a cohort, under various PCV13 coverage and serotype competition scenarios. It is worth mentioning that although the average lifespan of the US population is 78.6 years, the demographic parameter μ for the implementation of the fitted model to this cohort is fixed at 0.5 per year, because the cohort vaccination of PCV13 in the USA is limited to children under the age of 2 years (i.e.
) [Citation10]. On average there are 3.7 million newborns per year in the USA (i.e.
). The simulations are aimed at exploring the impact of the cohort vaccination on curtailing the prevalence of 19A, as well as in exploring how vaccination indirectly affects the prevalence of 35B. For these simulations, the vaccination model (EquationA.1
(A.1)
(A.1) ) is ran for a period of 30 years, using various values of the low (
) and high (
) cohort vaccination coverages. The serotype distribution is initially set in the cohort group to be 7.3% for 19A and 2.5% for 35B (this assumption is based on the serotype distribution given in [Citation16] for the first study period). Based on this, the initial conditions for each cohort vaccination coverage level is given below:
(i). | No vaccination ( | ||||
(ii). | Low cohort vaccination coverage level ( | ||||
(iii). | High cohort vaccination coverage level ( |
Simulations of the vaccination model (EquationA.1(A.1)
(A.1) ) for the worst-case scenario (i.e. with no vaccination, so that
) show that the prevalence of the streptococcus serotype 19A (35B) increases (decreases) from the initial prevalence 7.3% (2.5%) and reaches 37.93% (0%) at steady state, but the co-colonization remains constant at 0% for the entire 30 years period (Figure a). For these simulations the constituent reproduction numbers of the cohort vaccination model are ordered as
(so that,
). It is worth mentioning that even if both
and
are greater than unity, the solutions converge to serotype 19A-only boundary equilibrium. This suggests that the competitive exclusion results given in Theorems 3.4 and 3.5, for the reduced model discussed in Section 3, also extend to the full vaccination model (EquationA.1
(A.1)
(A.1) ).
Figure A2. Effect of cohort vaccination coverage (ϕ) on the prevalence of the two SP serotyes and co-colonization. Simulations of the vaccination model (EquationA.1(A.1)
(A.1) ), showing the prevalence of 19A, 35B and co-colonization and overall cohort-wide vaccination coverage, as a function of time for (a) no cohort vaccination(
), (b) low coverage level of cohort vaccination (
), (c) high coverage level of cohort vaccination (
). Other parameter values used are as given in Table . The initial condition for no (
), low (
) and high (
) coverage level of cohort vaccination are listed in items (i), (ii) and (iii), respectively, in Section D. The constituent reproduction numbers of the vaccination model for each of the settings (a)–(c) above are given by (a)
,
(so that,
), (b)
,
(so that,
) and (c)
,
(so that,
). The rate parameters are in years.
![Figure A2. Effect of cohort vaccination coverage (ϕ) on the prevalence of the two SP serotyes and co-colonization. Simulations of the vaccination model (EquationA.1(A.1) (Nu)′=Λ(1−ϕ)+ωNv−(λi+λj+μ)Nu,(Nv)′=Λϕ−[(1−ϵ)λi+λj+ω+μ]Nv,(Cui)′=λiNu+ηiλiRuni−(γi+θiλj+μ)Cui,(Cvi)′=(1−ϵ)λiNv+ηiλiRvni−(θvγi+θiλj+μ)Cvi,(Cuj)′=λjNu+ηjλjRunj+ωCvj−(γj+θjλi+μ)Cuj,(Cvj)′=λjNv+ηjλjRvnj−[γj+θj(1−ϵ)λi+ω+μ]Cvj,(Cuij)′=θiλjCui+θjλiCuj+θjηiλiCunij+θiηjλjCunji−(γij+μ)Cuij,(Cvij)′=θj(1−ϵ)λiCvj+θiλjCvi+θjηiλiCvnij+θiηjλjCvnji−[(θv(1−r2)+r2)γij+μ]Cvij,(Runi)′=γiCui−(ηiλi+λj+μ)Runi,(Rvni)′=θvγiCvi−(ηiλi+λj+μ)Rvni,(Runj)′=γjCuj+ωRvnj−(ηjλj+λi+μ)Runj,(Rvnj)′=γjCvj−[ηjλj+(1−ϵ)λi+ω+μ]Rvnj,(Runinj)′=γiCunji+γjCunij+(1−r1−r2)γijCuij−(ηiλi+ηjλj+μ)Runinj,(Rvninj)′=θvγiCvnji+γjCvnij+θv(1−r2)(1−r1)γijCvij−(ηiλi+ηjλj+μ)Rvninj,(Cunji)′=λiRunj+ηiλiRuninj+r2γijCuij−(γi+θiηjλj+μ)Cunji,(Cvnji)′=(1−ϵ)λiRvnj+ηiλiRvninj+r2γijCvij−(θvγi+θiηjλj+μ)Cvnji,(Cunij)′=λjRuni+ηjλjRuninj+r1γijCuij−(γj+θjηiλi+μ)Cunij,(Cvnij)′=λjRvni+ηjλjRvninj+θv(1−r2)r1γijCvij−(γj+θjηiλi+μ)Cvnij.(A.1) ), showing the prevalence of 19A, 35B and co-colonization and overall cohort-wide vaccination coverage, as a function of time for (a) no cohort vaccination(ϕ=0), (b) low coverage level of cohort vaccination (ϕ=0.4), (c) high coverage level of cohort vaccination (ϕ=0.8). Other parameter values used are as given in Table 2. The initial condition for no (ϕ=0), low (ϕ=0.4) and high (ϕ=0.8) coverage level of cohort vaccination are listed in items (i), (ii) and (iii), respectively, in Section D. The constituent reproduction numbers of the vaccination model for each of the settings (a)–(c) above are given by (a) Rvi=3.214, Rvj=1.1 (so that, Rv=3.214), (b) Rvi=2.222, Rvj=1.1 (so that, Rv=2.222) and (c) Rvi=1.23, Rvj=1.1 (so that, Rv=1.23). The rate parameters are in years.](/cms/asset/5ea5ad36-d416-4a52-a55c-550ad3c78d8a/tjbd_a_1884760_f0007_oc.jpg)
When the cohort vaccination program is implemented at the aforementioned low (40%) coverage level, the simulation results obtained show that prevalence of serotype 19A (35B) increases (slightly decreases) from the initial prevalence 7.3% (2.5%) and reaches 16.06% (0%), but the co-colonization remains constant at 0% for the entire 30 years period (Figure b).
For high coverage level of the cohort vaccination program (i.e. ), there is a decrease (increase) in the prevalence of the 19A (35B) from the initial prevalence 7.3% (2.5%) which reaches 0.89% (4.21%) at steady state, and the co-colonization increases to 0.013% (Figure c). In this setting, the presence of the phenomenon of serotype replacement in the cohort vaccination model against the two serotypes (19A & 35B) is observed (Figure c).
In summary, our simulations show that, while the use of cohort vaccination reduces the prevalence of 19A, high cohort vaccination coverage increases the prevalence of 35B. For high level of cohort vaccination coverage, the presence of the phenomenon of serotype replacement in the cohort vaccination model against the two serotypes of SP (EquationA.1(A.1)
(A.1) ) was illustrated. Furthermore, when there is no, or there is low, coverage of cohort vaccination, the phenomenon of competitive exclusion is observed, where 19A (the serotype with higher reproduction number) drives serotype 35B to extinction (Figures (a) and (b)). However, it is worth highlighting that the competitive exclusion was not observed in the simulations carried out in the presence of cohort vaccination with high coverage(Figure c).