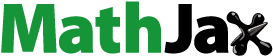
ABSTRACT
An intraguild predator–prey model including prey refuge and hunting cooperation is investigated in this paper. First, for the corresponding ordinary differential equation model, the existence and stability of all equilibria are given, and the existence of Hopf bifurcation, direction and stability of bifurcating periodic solutions are investigated. Then, for partial differential equation model, the diffusion-driven Turing instability is obtained. What is more, the existence and non-existence of the non-constant positive steady state of the reaction–diffusion model are established by the Leray–Schauder degree theory and some priori estimates. Next, some numerical simulations are performed to support analytical results. The results showed that prey refuge can change the stability of model and even have a stabilizing effect on this model, meanwhile the hunting cooperation can make such model without diffusion unstable, but make such model with diffusion stable. Lastly, a brief conclusion is concluded in the last section.
1. Introduction
The interaction between predator and prey has become increasingly complex due to long-term convolution. To avoid being hunted by predators and prevent their own species from extinction, the prey gradually adopts the collective defence, escape, hiding and other methods to reduce their mortality rate. One of two common ways is the prey hiding from predators through rapid escape, the other is that the prey can avoid predators by establishing various refuges (i.e. spatial refuges) to provide guarantee for population reproduction [Citation20, Citation21, Citation32, Citation51]. Although, the refuge reduces the mortality rate of the prey, the birth rate of the prey will also be reduced due to the lack of resources and harsh environment in refuges. Therefore, the refuge has both positive and negative effects on the prey [Citation20, Citation25].
In 1946, Crombic did one experiment on two species of beetles, in which the larvae of prey species were placed in glass tubes to avoid being eaten by predators, then obtained the coexistence of the two species. By experiment, he not only verified the existence of natural refuges for the prey but also introduced them into Lotka–Volterra model. Thus Crombic established the first predator–prey model with prey refuge:
where m is a fixed number of the prey being protected by the refuge. To easily establish the predator–prey system with prey refuge in mathematics, Taylor [Citation44] divided prey refuges into two types: one is that the prey enters the refuge in a fixed number [Citation6, Citation23], and the other is that the prey enters the refuge in a fixed proportion [Citation10]. In addition, Ruxton [Citation37] derived a continuous-time model under the assumption that the refuge term is proportional to the density of predator and showed that prey refuge term has a stabilizing effect. Many empirical and theoretical scholars have investigated the consequences of prey refuge and concluded that prey refuge has a stabilizing effect on the considered predator–prey interaction, and increasing refuge can prevent the prey from dying out [Citation9, Citation11–14, Citation24, Citation30, Citation50].
Recently, Kar [Citation24] investigated a prey–predator model incorporating prey–refuge and independent harvesting:
(1)
(1) where mx (
) is a refuge protecting of the prey and
is the prey available to the predator. Using the harvesting efforts as controls, the optimal harvest policy is obtained by Pontryagin's maximal principle [Citation24].
Based on the model (Equation1(1)
(1) ), a three-component model composed of single and double prey species is proposed in the reference [Citation40]:
(2)
(2) Here Holling type II function contains a constant proportion of prey shelters. The authors [Citation40] also considered competition among predators for food (prey) and shelters, and obtained the threshold values of some parameters, the feasibility and stability conditions of the equilibrium, and the ranges of important parameters allowing different types of bifurcations in such model.
Prey species seek the shelter, which makes it difficult for predator species to find food. So a series of adaptive characteristics for predators have been produced in the evolution process, such as sharp teeth, claws, fangs and other tools, using bait pursuit, collective hunting and other ways. For example, carnivores [Citation29], lions [Citation35], wolves [Citation41] and African wild dogs [Citation5] often capture and kill their prey together. Ants [Citation34], spiders [Citation47] and birds [Citation18] also seek out and attack their prey together. These behaviours have caused widespread attention.
Cosner [Citation4] first considered hunting cooperation in predator–prey model and proposed a functional response for the predators who hunt for food in a spatially linear formation and aggregate when they encounter a large group of prey. Berec [Citation2] discussed in detail how cooperative foraging did not always help to sustain the population but can have some destabilizing effect on the underlying systems too. In 2017, Alves and Hilker [Citation1] investigated a prey–predator model with hunting cooperation in a Holling type I function:
(3)
(3) where N and P are the densities of the prey and the predator respectively.
is Holling-type I function, i.e.
, here
is the attack rate per predator and prey. In the case of hunting cooperation, the function depends on both prey and predator densities. They assumed that cooperative predators benefit from their behaviour, the success of attacks on prey increases with predator density. They [Citation1] represented this assumption in the model (Equation3
(3)
(3) ) by replacing the constant attack rate λ with a density-dependent term:
(4)
(4) where a>0 is the predator cooperation in hunting and aP is the cooperation term. They showed that hunting cooperation is beneficial to the predator population by increasing the attack rate, identified a scenario in which the hunting cooperation produces Allee effects in predators and allowed the latter to persist when the prey does not sustain them in the absence of hunting cooperation. However, hunting cooperation can turn detrimental to predators when prey density drastically decreases due to the increased predation pressure, which decreases inhalation of the predator. Hunting cooperation can also destabilize the system and promote a sudden collapse of the predator [Citation8, Citation31].
In addition, Sapkota et al. [Citation39] proposed a three-species food chain model with hunting cooperation among the middle predator as follows:
(5)
(5) where
and
denote the prey, middle predator and top predator respectively. The top predator preys on the middle predator and the middle predator preys on the prey. The hunting cooperation among the middle predator affects interestingly on the numbers of the predator and prey. The linear stability of model (Equation5
(5)
(5) ) and the two-parameter numerical analysis on hunting cooperation are conducted [Citation39].
Under the same or similar nutrient level in an ecosystem, the relationship between two populations may be both predation and competition [Citation3, Citation49]. Consequently, Polis, Myers and Holt [Citation36] first proposed the concept of intraguild predation (IGP) system. Intraguild predation is the mixture of competition and predation. That is, IGP involves three species: shared resource, IG prey and IG predator (see Figure ) and describes an interaction in which two species compete for shared resources and also eat each other. The IG prey feeds on only the shared resource while the IG predator feeds on both the IG prey and the shared resource.
The first general model describing IGP was exploited by Holt and Polis [Citation22], which takes the following form:
(6)
(6) where
and
represent the densities of the shared resource, IG prey and IG predator, respectively.
and
are functional responses of the IG predator to the shared resource and IG prey, respectively,
is the functional response of the IG prey to the shared resource.
and
are density-independent death rates. The parameters
and
convert resource consumption into reproduction for the IG prey and IG predator, respectively;
is the benefit enjoyed by the IG predator from its consumption of the IG prey;
is recruitment of the shared resource.
In reality, according to Fick's law, species have spatial heterogeneity, and individuals move to areas with lower density to increase the chance of survival. Based on this fact, the importance of the spatial model has been recognized by scholars in various fields and has been continuously investigated in depth. In recent decades, many experiments showed that the spatial models can generate the rich spatiotemporal dynamics (see [Citation7, Citation15, Citation16, Citation26, Citation33, Citation38, Citation42, Citation43, Citation45, Citation48, Citation52, Citation53] and references therein). Specially, Tiwari et al. [Citation45] discussed a diffusive predator–prey system including mutually interfering predators, nonlinear harvesting in predators with Crowley–Martin function. They [Citation45] not only obtained the local and global stability of interior equilibrium and the existence and nonexistence of non-constant steady state solutions but also gave the conditions for Turing instabilities of the diffusive system. Han et al. [Citation16] proved the well-posedness of the temporal and spatiotemporal models. For the temporal model, they [Citation16] analysed its temporal dynamics. For the spatiotemporal model, they [Citation16] not only investigated its permanence, stability of non-negative constant steady states and Turing instability, but also gave the existence and non-existence of non-constant positive steady states by Leray Schauder degree theory.
Therefore, based on the above research, it has rich research significance by considering the intraguild predation relationship from a biological point of view. Because the relationship has both predation and competitive, and many scholars have done much work on the intraguild predation model in recent years. Thus we will also consider the intraguild predation model. Both the IG prey and the IG predator feed on shared resources, thus we will consider the effect of refuge on the shared resource to protect the population from extinction. Of course, the IG prey and IG predator can promote them to capture food with greater efficiency through cooperation, thus the hunting cooperation is also considered. The movement among the three populations makes it more realistic for the model to introduce self-diffusion. As a result, taking into account of prey refuge in a Holling-type I functional response of the IG prey to the shared resource and in a Holling-type II functional response of the IG predator to the shared resource, hunting cooperation in a Holling-type I functional response of the IG predator to the IG prey, we will investigate an intraguild predation model as follows:
(7)
(7) where
and
represent the density of the shared resource, IG prey and IG predator at the position x and time t, respectively.
is a fixed spatial bounded domain with smooth boundary
and ν is the outward unit normal vector of boundary
. Generally,
and
respectively are the self-diffusion rates of the three species; r and a respectively denote the birth rate and intraspecific competition rate of the shared resource.
and
are natural death rates of the three species;
and
are maximum numbers of different prey that can be eaten by the respective predator per unit time;
and
are a conversion rate of different prey captured by respective predator.
and
are fixed proportion of the shared resource by refuge, the remaining of the shared resource
and
are available to IG prey and IG predator, respectively. c is the same meaning as a of (Equation4
(4)
(4) ). All the parameters are the positive constants.
To investigate the properties of the corresponding ordinary differential model and the influence of diffusion in pattern formation as well as the influence of refuge effect and hunting cooperation, we denote and
Then model (Equation7
(7)
(7) ) can be rewritten as
(8)
(8) The remaining of this paper is organized as follows. In Section 2, the existence and stability of equilibria, the existence, direction and stability of the Hopf bifurcation of model (Equation9
(9)
(9) ) are discussed. In Section 3, the diffusion-driven Turing instability of model (Equation7
(7)
(7) ) is investigated, and the existence and non-existence of non-constant positive steady states are discussed by establishing positive upper and lower bounds and using Leray–Schauder degree theory. Numerical simulations are employed to confirm the theoretical results in Section 4. In Section 5, some discussions are given.
2. Stability and Hopf bifurcation of ODE model
In this section, we mainly consider the existence and stability of equilibria, the existence, direction and stability of the Hopf bifurcation of the ordinary differential equation (ODE) model corresponding to model (Equation8(8)
(8) ). The ODE model is as follows:
(9)
(9)
2.1. The existence and local stability of equilibria
It is obvious that (Equation9(9)
(9) ) have the following nonnegative constant steady states:
the trivial equilibrium
;
the semi-trivial equilibrium in the absence of IG prey and IG predator
if condition
holds, where
and
;
the semi-trivial equilibrium in the absence of IG predator
if condition
is true, where
,
and
, i.e.
;
the semi-trivial equilibrium in the absence of IG prey
if assumption
holds, where
,
and
;
the co-exist equilibrium
is expressed as follows:
and determined by the following equation:
(10)
(10) where
We can know that
and
can lead to
. From Descartes' Rule of Signs, it follows that the number of positive roots of Equation (Equation10
(10)
(10) ) is shown in Table .
Table 1. The number of positive roots of Equation (Equation10(10)
(10) )
Based on the above analysis, it can be seen that the existence of the co-exist equilibrium of model (Equation9(9)
(9) ) is very complicated. Therefore, we only discuss the second and fourth cases in this paper, that is, model (Equation9
(9)
(9) ) has only one co-exist equilibrium if the assumption
holds.
Next, we will use the standard linearization method to discuss the local stability of each equilibrium. The Jacobian matrix of the model (Equation9(9)
(9) ) at any non-negative equilibrium
is given by
(11)
(11) where
Lemma 2.1
The trivial equilibrium of model (Equation9
(9)
(9) ) is always unstable.
Proof.
The characteristic equation of model (Equation9(9)
(9) ) at the trivial equilibrium
is
Thus we can get that the corresponding eigenvalues are
So the trivial equilibrium
is unstable.
Lemma 2.2
If the condition and
hold, then the semi-trivial equilibrium
is locally asymptotically stable.
Proof.
Through the Jacobian matrix (Equation11(11)
(11) ), the characteristic equation of model (Equation9
(9)
(9) ) at the equilibrium
is
Thus one eigenvalue is
and other eigenvalues
,
if and only if
. Thus the semi-trivial equilibrium
is locally asymptotically stable.
Lemma 2.3
Under the case of conditions and
, the semi-trivial equilibrium
is locally asymptotically stable, where
.
Proof.
From matrix (Equation11(11)
(11) ), the characteristic equation of model (Equation9
(9)
(9) ) at equilibrium
takes the form
where
Therefore,
,
and
are the roots of the following equation:
where
. It has that
and
. Hence, the semi-trivial equilibrium
is locally asymptotically stable if
is true.
Lemma 2.4
If conditions and
hold, then the semi-trivial equilibrium
is locally asymptotically stable, where
and
, here
,
,
.
Proof.
From the Jacobian matrix (Equation11(11)
(11) ), the characteristic equation of model (Equation9
(9)
(9) ) at the semi-trivial equilibrium
takes
where
Further,
,
and
are the roots of following equation:
where
. It follows that
if and only if
. That is, when the assumption
holds, the semi-trivial equilibrium
is locally asymptotically stable.
Next, we analyse the local stability of the co-existence equilibrium . According to the matrix (Equation11
(11)
(11) ), the characteristic equation of model (Equation9
(9)
(9) ) at
is
(12)
(12) where
Assuming that
holds, we can conclude that
and
.
Lemma 2.5
All roots of Equation (Equation12(12)
(12) ) have negative real parts if and only if the conditions
and
are satisfied. Equation (Equation12
(12)
(12) ) has one negative root and a pair of complex roots with a positive real part if and only if the conditions
and
are true.
Based on Lemma 2.5, we obtain the following theorem.
Theorem 2.6
Assume that conditions and
are satisfied, the co-existence equilibrium
is locally asymptotically stable if
, but is unstable if
. Further, the co-existence equilibrium
loses stability when
passes through 0. That is, the Hopf bifurcation occurs at the co-existence equilibrium
when
.
Next, we investigate the existence of the Hopf bifurcation by considering the prey refuge as a bifurcation parameter. After some computations,
reduces to
(13)
(13) where
Without loss of generality, let
be the positive root of Equation (Equation13
(13)
(13) ). Then Equation (Equation12
(12)
(12) ) has a negative root denoted
and a pair of complex conjugates roots which are denoted
such that
.
Substituting into Equation (Equation12
(12)
(12) ) and separating the real and imaginary parts gives
(14)
(14)
(15)
(15) Because
, from Equation (Equation15
(15)
(15) ) we have
(16)
(16) Then we substitute (Equation16
(16)
(16) ) in Equation (Equation14
(14)
(14) ) and get
(17)
(17) So Equation (Equation17
(17)
(17) ) has a root
if and only if
. Furthermore, since
has no real root, u = 0 is the only root. Thereby, when
, the roots of (Equation12
(12)
(12) ) are
.
We will prove that . Differentiating Equation (Equation17
(17)
(17) ) with respect to
gives
(18)
(18) When
in (Equation18
(18)
(18) ), we have that from
It shows that the hypotheses of Hopf theorem are satisfied. Thus we obtain the following result.
Theorem 2.7
Suppose that the coefficients of Equation (Equation12(12)
(12) ) at
are functions of
and
at
satisfy the following conditions:
Thus Hopf bifurcation occurs at the co-existence equilibrium
when
.
2.2. Direction of Hopf bifurcation and stability of the bifurcating periodic solutions
In this part, we will derive an explicit algorithm to determine the direction of Hopf bifurcation and stability of the bifurcating periodic solutions for model (Equation9(9)
(9) ) by using the centre manifold theory and normal form method.
For the sake of convenience, we translate the equilibrium to the origin by the transformation
. We denote
by X, Y, Z to calculate conveniently, so the model (Equation9
(9)
(9) ) becomes
(19)
(19) where
Let
and
be the eigenvectors corresponding to eigenvalues
and
of
at
, respectively. We can obtain
and
,
where
Denote the matrix
with
Then we obtain
where
By the transformation
, system (Equation19
(19)
(19) ) becomes
(20)
(20) where
and
Thus the system (Equation20
(20)
(20) ) can be written as
(21)
(21) By the algorithm proposed by Hassard et al. [Citation17], we get
and
By solving the equations
and
, we have
. Moreover, we have
Then, we can compute the following values:
(22)
(22) which determine the direction of Hopf bifurcation and the stability of bifurcating periodic solutions. Based on the above analysis and the conclusions of Hassard et al. [Citation17], we have the following results.
Theorem 2.8
Suppose that assumption satisfies, Hopf bifurcation occurs at the co-existence equilibrium
of model (Equation9
(9)
(9) ) when
. If
, then the direction of Hopf bifurcation is supercritical (subcritical); if
, then the period of bifurcating periodic solutions increases (decreases); if
, then the bifurcating periodic solutions are stable (unstable).
3. Dynamics of the reaction–diffusion model
3.1. Linear stability and Turing instability
In this section, we consider the influence of the diffusion on the stability of the co-existence equilibrium and investigate the diffusion-induced spatially inhomogeneous steady state. In [Citation46], Turing concluded that the reaction–diffusion model may exhibit spatial patterns under the fact that the equilibrium is linearly stable in the absence of diffusion, but becomes linearly unstable in the presence of diffusion. Such instability is called a Turing instability or diffusion-driven instability.
Let be the eigenvalues of the operator
on Ω with the homogeneous Neumann boundary condition and
be the eigenspace corresponding to
in
. Let
be an orthonormal basis of
, and
,
. Then
(23)
(23) Suppose that
hold, let
. The linearization system of (Equation8
(8)
(8) ) at
is
. For each
is invariant under the operator
, and λ is an eigenvalue of
if and only if it is an eigenvalue of the matrix
for some
, in which there is an eigenvector in
. The characteristic polynomial of
is given by
where
here
and
are given in (Equation12
(12)
(12) ). If
, then it follows that
. Furthermore, we have
(24)
(24) where
if
. Recalling that
, we conclude that
for all
. From Routh–Hurwitz criterion, for each
,
of
have negative real parts.
In the following, we will prove that there exists a positive constant δ such that
(25)
(25) Let
, we have
Furthermore, it follows that
Applying the Routh–Hurwitz criterion, it follows that the three roots
of
have negative real parts. Thus there exists a positive constant
such that
. By continuity, we can find that there exists
such that the three roots
of
satisfy
. In turn,
Let
, then
, and Equation (Equation25
(25)
(25) ) holds for
. Hence, the spectrum of
lies in
. By Theorem 5.1.1 in reference [Citation19], we have the local stability of
.
Theorem 3.1
Suppose that and
are true, the co-existence equilibrium
of model (Equation8
(8)
(8) ) is local asymptotically stable if
, but is unstable if
.
The Routh–Hurwitz criterion implies that is unstable in model (Equation8
(8)
(8) ) if
or
. Consequently, to discuss whether
is stable (i.e.
) in model (Equation9
(9)
(9) ) but unstable in partial differential equation (PDE) model (Equation8
(8)
(8) ), we consider the following limits:
where
is given in (Equation12
(12)
(12) ). Suppose that
, we get
if
.
if
, i.e.
Combining with
large enough, we have
. Therefore, diffusion-driven Turing instability occurs when
is large enough. In addition, we define
and
.
Theorem 3.2
If the assumptions and
hold, then there exists an unbounded region
such that for any
,
is local asymptotically stable when
in reaction–diffusion model (Equation8
(8)
(8) ), but is unstable when
. That is, Turing instability occurs.
3.2. A-priori bounds for the positive steady state
The corresponding steady-state problem of (Equation8(8)
(8) ) is
(26)
(26) In the following, the generic constants
etc., will depend on the domain Ω and the dimension N. However, when Ω and N are fixed, we will not mention this dependence explicitly. For convenience, we denote the constants
collectively by Λ. The main purpose of this section is to give a-priori positive upper and lower bounds for the positive solutions of (Equation26
(26)
(26) ). First, we need two results as follows.
Lemma 3.3
Maximum Principle [Citation27]
Let and
.
(1) | If | ||||
(2) | If |
Lemma 3.4
Harnack Inequality [Citation28]
Let be a positive solution to
with
, subject to homogeneous Neumann boundary condition. Then there exists a positive constant
such that
Consequently, the results of upper and lower bounds can be given as follows:
Theorem 3.5
Upper Bound
Let k be a fixed positive number. Then there exist positive constants and
such that, when
the positive solution
to (Equation26
(26)
(26) ) satisfies
(27)
(27)
Proof.
First of all, we will prove that this estimates
(28)
(28) Applying Lemma 3.3 to the first equation of (Equation26
(26)
(26) ), it yields
. Define
, where
, then we get
Let
such that
. It follows from Lemma 3.3 that
(29)
(29) A simple application of Lemma 3.3 to the third equation of (Equation26
(26)
(26) ) obtains
for a positive constant
. This together with (Equation29
(29)
(29) ) gets
for a positive constant
. Similarly, we can prove that
and
for some positive constants
and
.
Define and
. Because of (Equation28
(28)
(28) ), by the regularity for elliptic equations we have that X, Y, Z belong to
, and
norms of them depend on known parameters
. Using the regularity of elliptic equations again, the estimate (Equation27
(27)
(27) ) follows.
Theorem 3.6
Lower Bound
Let k be a fixed positive number. Then there exist a positive constant such that, when
the positive solution
to (Equation26
(26)
(26) ) satisfies
(30)
(30)
Proof.
We suppose that (Equation30(30)
(30) ) does not hold. Then there exists a sequence
with
and the corresponding positive solutions
to (Equation26
(26)
(26) ) such that
According to (Equation27
(27)
(27) ), this implies that
(31)
(31) As
, we assume
as
. By the maximum principle, we get
. Integrating by parts yields
(32)
(32) The standard regularity theorem for elliptic equations yields that there exists a subsequence of
denoted by
, and three non-negative functions
, such that
in
as
. By (Equation31
(31)
(31) ), we find that
or
or
. Let
in (Equation32
(32)
(32) ), then we have
(33)
(33) Next, we will consider three cases as follows.
Case (i). .
Since , as
, then
on
, for all
. Integrating the differential equation for
over Ω by parts, we have
which is a contradiction to the second equation of (Equation32
(32)
(32) ).
Case (ii). .
Since , as
, then
on
, for all
, and
which is a contradiction to the third equation of (Equation32
(32)
(32) ).
Case (iii). on
, then the Hopf lemma gives X>0, Y >0.
(a) . Under the circumstances, X satisfies
which implies that
is a positive constant. If
, then Y meets
When
, we get
, which is a contradiction.
If or
, then
is a constant. By the third equation of (Equation32
(32)
(32) ), there exists
such that
(34)
(34) i.e.
. Furthermore, we assume that
as
. So Equation (Equation34
(34)
(34) ) implies
. Then from the first equation of (Equation33
(33)
(33) ), we get
which is a contradiction to Lemma 4.5 [Citation7].
(b) . If
, then X and Y satisfy
(35)
(35) By Lemma 4.4 [Citation7], (Equation35
(35)
(35) ) has no non-constant positive solution. Hence
is a constant vector and Z = 0, but it is a contradiction to Lemma 4.5 [Citation7].
If , set
, then
satisfy
(36)
(36) Similarly, there exists a subsequence
, denoted by
, and three non-negative functions
, such that
in
. Due to
, we obtain that
. In addition, we notice that
. Therefore, let
in (Equation36
(36)
(36) ), then we get
(37)
(37) If
, then
satisfies
, and
, which means that
. If
, an application of strong maximum principle and the Hopf boundary lemma to the third equation of (Equation37
(37)
(37) ) gives
on
. The above analysis shows that
on
for both cases
and
.
Notice that if
. The third equation of (Equation37
(37)
(37) ) means
. Then X satisfies a logistic-type elliptic equation
, which makes X to be a constant
, a contradiction to Lemma 4.5 [Citation7]. Therefore, the proof of this theorem is completed.
3.3. Non-existence of non-constant positive steady states
In this section, we apply the energy method to prove the non-existence of the non-constant positive steady states of (Equation26(26)
(26) ) by the effect of large diffusivity of the three species, which manifests that the three species can disperse fast. This means that the three populations will be spatially homogeneously distributed.
Theorem 3.7
If there exist three constants such that
and
, then the problem (Equation26
(26)
(26) ) has no non-constant solution.
Proof.
Assume that is a positive solution to (Equation26
(26)
(26) ) and denote
,
and
. Multiplying the first equation of (Equation26
(26)
(26) ) and then integrating on Ω by parts yields
By the similar method, we can get
From the above results and the ε-Young inequality, it follows that
where ε is a small enough positive number. Using the Poinc
re inequality, we get
Let
If
and
, then
constant,
constant and
constant. Hence, this proof is completed.
3.4. Existence of non-constant positive steady states
The previous part analysed the case that a non-constant positive steady states would not occur. In this part, by using the Leray–Schauder degree theory, we will discuss the existence of non-constant positive steady states of (Equation26(26)
(26) ), that is the stationary pattern can exist for the system (Equation7
(7)
(7) ) with self-diffusion.
Let be the Hilbert space as in Section 3.1, and define
Next, (Equation26
(26)
(26) ) can be written as follows:
(38)
(38) Thus
is a positive solution of (Equation38
(38)
(38) ) if and only if it meets
where
is the inverse of
with the homogeneous Neumann boundary condition. As
is a compact perturbation of the identity operator, for any
, the Leray–Schauder degree
is well-defined if
on
. Furthermore, simple computation yields
If
is invertible, the index of L at
is defined as index
, where κ is the sum of algebraic multiplicity of negative eigenvalues of
.
We refer to the decomposition (Equation23(23)
(23) ) in the following discussion of the eigenvalues of
. First of all, for each integer
,
is invariant under
, and λ is an eigenvalue of
if and only if
is an eigenvalue of the matrix
. Thus
is invertible if and only if this matrix is non-singular. Let
To calculate the index
, we cite the following lemma.
Lemma 3.8
[Citation7]
Suppose that the matrix is non-singular for all
. Then
where
.
The direct computation gives (39)
(39) where
here
are given in (Equation12
(12)
(12) ).
We find the dependence of W on . Let
and
be the three roots of
with
. It is known that
from (Equation39
(39)
(39) ), and according to the previous analyses,
can be obtained. Thus, due to
, one of
is real and negative, and the product of the other two is positive.
Consider the following limits:
If
holds, then the following lemma is true.
Lemma 3.9
Assume that holds, there exists a positive constant d such that when
, three roots
,
of
are all real and meet
Furthermore, it obtains
(40)
(40)
By determining the range of μ such that , we have the existence of non-constant steady states of (Equation26
(26)
(26) ).
Theorem 3.10
Suppose that and Λ are fixed and the condition
is true. If
for some
and the
is odd, then there exists a positive constant d such that (Equation26
(26)
(26) ) has at least one non-constant positive solution for
.
Proof.
By Lemma 3.9 and , there exists a positive constant d such that (Equation40
(40)
(40) ) satisfies if
, and
(41)
(41) By using the homotopic invariance of the topological degree, we prove that (Equation26
(26)
(26) ) has at least one non-constant positive solution for any
.
On the contrary, suppose that the conclusion is not true for some . Let
. By Theorem 3.7, we can get that
,
,
. Then fix
,
,
. Define
with
and
, and consider the following elliptic equation problem:
(42)
(42)
is a non-constant positive solution of (Equation26
(26)
(26) ) if and only if it is a solution of (Equation42
(42)
(42) ) with t = 1. It is obvious that
is the unique constant positive solution of (Equation42
(42)
(42) ) for any
. For any
,
is a non-constant positive solution of (Equation42
(42)
(42) ) if and only if
Obviously,
. According to Theorem 3.7,
has only the positive solution
in
. Then, we obtain
where
. From (Equation39
(39)
(39) ), we know that
By (Equation40
(40)
(40) ) and (Equation41
(41)
(41) ), it follows that
Hence, zero is not an eigenvalue of the matrix
for all
, and
, which is odd. It follows from Lemma 3.8 that
(43)
(43) Now, by Theorems 3.6 and 3.5, there exists a positive constant C such that, for all
, the positive solution of (Equation42
(42)
(42) ) satisfies
. Hence
on
for all
. By the homotopy invariance of the topological degree, we have that
(44)
(44) Nevertheless, both equation
and
have the unique positive solution
in
. From (Equation43
(43)
(43) ), we have that
which contradict (Equation44
(44)
(44) ). Thus we finish the proof of this theorem.
4. Numerical simulation
In this section, with the help of Matlab software, numerical simulations will be given to illustrate our analytic results obtained in previous sections. All values of parameters in model (Equation7(7)
(7) ) are given according to the meaning of parameters. What's more, because model (Equation7
(7)
(7) ) is not considered for the specific species a reasonable set of parameters are chosen as follows:
Since
and
represent the density of the shared resource, IG prey and IG predator at the time t, respectively, the initial values of system (Equation9
(9)
(9) ) are assumed to be
.
First of all, we mainly illustrate the stability and Hopf bifurcation for non-spatial model (Equation9(9)
(9) ) by using the ode45 function of Matlab software. According to Theorem 2.6, we obtain that model (Equation9
(9)
(9) ) has the positive equilibrium
and is locally asymptotically stable (see Figure ). Subsequently, we choose
of model (Equation9
(9)
(9) ) as a bifurcation parameter. Based on the parameter values given above, we can get
. Furthermore, when
,
is locally asymptotically stable (see Figure a), but
is unstable when
(see Figure b). That is, Hopf bifurcation takes place when
. That is, when we take measures to protect the shared resource, the dynamics of system is determined by the strength of protection. If the strength of protection is weak, periodic fluctuations occur in the system. Inverse, the system will keep well stability.
Figure 2. The co-existence equilibrium of model (Equation9
(9)
(9) ) is locally asymptotically stable: (a) shared resource, (b) IG prey, (c) IG predator. (a)
, (b)
and (c)
.

Figure 3. Local dynamics of model (Equation9(9)
(9) ) at the co-existence equilibrium
: (a)
, (b)
.

Next, by choosing the and fixing all values of the other parameters, we obtain that the dynamical behaviour of the model (Equation9
(9)
(9) ) at the positive equilibrium
without prey refuge and hunting cooperation is shown in Figure . From Figure , the shared resource, IG prey and IG predator take periodically if IG predators lack of hunting cooperation and the shared resource is not protected.
Figure 4. The dynamical behaviour of the model (Equation9(9)
(9) ) at
when
.

Based on Theorem 2.6, the dynamic behaviour of the system at the positive equilibrium without the effect of hunting cooperation is seen in Figure . Further, it can be concluded that the stability of model (Equation9
(9)
(9) ) at
is better with the increase of prey refuge.
Figure 5. The dynamical behaviour of model (Equation9(9)
(9) ) at
when c = 0: (a) shared resource, (b) IG prey, (c) IG predator. (a)
, (b)
and (c)
.

In addition, according to Lemma 2.5, the dynamical behaviour of model (Equation9(9)
(9) ) at the positive equilibrium
without prey refuge is seen in Figure . From Figure , the shared resource, IG prey and IG predator take periodically if the prey refuge of the shared resource is not considered. That is, we take measures to protect the resource, which is good for the long-term stability of the system.
Figure 6. The dynamics of model (Equation9(9)
(9) ) at
when
: (a) shared resource, (b) IG prey, (c) IG predator. (a)
, (b)
and (c)
.

While the effect of prey refuge is considered, i.e. , the model (Equation9
(9)
(9) ) becomes stable when c is very small (see Figure ), but becomes unstable when it gradually increases (see Figure ). From the viewpoint of ecology, the hunting cooperation of IG predator may change the stability of system. That is, the hunting cooperation may be beneficial for IG predator. However, when the intensity of cooperation is gradually increasing, it may be bad for the system, which shows that the effect of hunting cooperation makes the model always unstable.
Figure 7. The model (Equation9(9)
(9) ) with prey refuge becomes stable when hunting cooperation c is very small: (a) shared resource, (b) IG prey, (c) IG predator. (a)
. (b)
and (c)
.

Figure 8. The model (Equation9(9)
(9) ) with prey refuge becomes unstable when hunting cooperation c gradually increases: (a) shared resource, (b) IG prey, (c) IG predator. (a)
, (b)
and (c)
.

Finally, we will check the spatial dynamics of model (Equation7(7)
(7) ) by considering the diffusion. We will use pdepe function of Matlab software to solve PDE model and show intuitive results. We assume the spatial region Ω as an one-dimensional domain
for the convenience of simulation. We keep all values of all parameters with the same of the above and choose the values of diffusions
satisfying one condition of Theorem 3.1. Under this case, we can obtained the critical value
. Thus, according to Theorem 3.1, the co-existence equilibrium
is stable when
, which is shown in Figure , but is unstable when
, which is shown in Figure . That is, it also can be concluded that the stability of model (Equation9
(9)
(9) ) at
is better with the increase of prey refuge.
Figure 9. The model (Equation7(7)
(7) ) is stable when
: (a) shared resource, (b)IG prey, (c) IG predator. (a)
, (b)
and (c)
.

Figure 10. Unstable behaviour of model (Equation7(7)
(7) ) when
: (a) shared resource, (b) IG prey, (c) IG predator. (a)
. (b)
and (c)
.

To check Turing instability, we choose that the values are . It is obtained that
. According to Theorem 3.2, when
, the positive equilibrium
is local asymptotically stable as shown in Figure , but Turing instability appears as shown in Figure when
. Generally speaking, the diffusion often makes the Turing instability take place. However, the prey refuge for IG prey also change the stability of the model with and without diffusion.
Figure 11. Model (Equation7(7)
(7) ) is local asymptotically stable when
,
,
and
: (a) shared resource, (b)IG prey, (c) IG predator. (a)
, (b)
and (c)
.

Figure 12. Turing instability occurs in model (Equation7(7)
(7) ) when
,
,
, and
: (a) shared resource, (b) IG prey, (c) IG predator. (a)
, (b)
and (c)
.

Whether the hunting cooperation in PDE model has the same role in that ODE model? We choose that the new values of all parameters are as follows: When
and
, the effect of hunting cooperation on the model (Equation7
(7)
(7) ) is shown in Figure . From Figure , we know that the model (Equation7
(7)
(7) ) becomes more stable when the effect of hunting cooperation increases. That is, under the case diffusion, the hunting cooperation of IG predator can make the system stale.
Figure 13. Effect of hunting cooperation on model (Equation7(7)
(7) ) when
,
,
and
: (a) c = 0.45; (b) c = 1; (c) c = 2.5.

5. Conclusion
In this paper, we have investigated an intraguild prey–predator model with prey refuge and hunting cooperation. By choosing prey refuge as the Hopf bifurcation parameter, we obtained the condition of the existence of the Hopf bifurcation, and showed the direction of Hopf bifurcation and stability of the bifurcating periodic solutions by using the normal form theory and the centre manifold theorem. According to a lot of numerical simulations, we found that only hunting cooperation c always makes model (Equation9
(9)
(9) ) unstable, but model (Equation9
(9)
(9) ) becomes stable with the increase of prey refuge effect
. Moreover, our research also explains the reasons for prey refuge and hunting cooperation in real life from the perspective of Hopf bifurcation, which effectively verifies the reliability of the theoretical results. From an ecological point of view, hunting cooperation makes the ODE model with intraguild predation unstable, which increases the mortality rate of the three populations, and the possibility of extinction occurs in severe cases, while the refuge effect is within a certain range which makes the model stable. That is, maintaining the survival of various species and ensuring that the population does not become extinct. In addition, we study the diffusive model (Equation7
(7)
(7) ), which has the same positive equilibrium with the ODE model (Equation9
(9)
(9) ). It is shown that diffusion-driven Turing instability occurs under certain conditions. Finally, we also discuss the existence and non-existence of non-constant positive steady states of the system (Equation7
(7)
(7) ) by establishing positive upper and lower bounds by using Leray–Schauder degree theory. Biologically, the refuge effect causes Turing instability within a certain range, which shows the population inhomogeneously distributed within the spatial domain and exhibits various patterns. As the hunting cooperative effect increases, the model becomes more stable. If two factors are properly introduced together, then the system will become more stable, and the population is homogeneously distributed in space. When the shared resource or IG prey populations diffuse rapidly, they will break the original stable state and are spatially inhomogeneously distributed. While the three populations diffuse rapidly, they will not change the stable state, that is, the population is spatially inhomogeneously distributed.
From the viewpoint of human profit, the humans will capture some corresponding organisms to obtain certain economic benefits and the degree of economic impact on people often depends on the implementation of the harvest in nature. In addition, some behaviours of predator or prey species cannot be displayed immediately in ecology, that is, there is a time delay. To make the model more practical, we will consider time delay and linear harvesting into our model,
where E is the harvesting effort,
and
are the catchability coefficient of IG prey and IG predator, respectively. τ is the time delay due to the gestation of the IG predator. We leave this work in the future.
Disclosure statement
No potential conflict of interest was reported by the author(s).
Additional information
Funding
References
- M.T. Alves and F.M. Hilker, Hunting cooperation and Allee effects in predators, J. Theor. Biol. 419 (2017), pp. 13–22.
- L. Berec, Impacts of foraging facilitation among predators on predator-prey dynamics, Bull. Math. Biol. 72 (2010), pp. 94–121.
- L.B. Catano, A.A. Shantz, and D.E. Burkepile, Predation risk, competition, and territorial damselfishes as drivers of herbivore foraging on Caribbean coral reefs, Mar. Ecol. Progr. Ser. 511 (2014), pp. 193–207.
- C. Cosner, D.L. DeAngelis, J.S. Ault, and D.B. Olson, Effects of spatial grouping on the functional response of predators, Theor. Popul. Biol. 56 (1999), pp. 65–75.
- S. Creel and N.M. Creel, Communal hunting and pack size in African wild dogs, Lycaon pictus, Anim. Behav. 50 (1995), pp. 1325–1339.
- S. Devi, Nonconstant prey harvesting in ratio-dependent predator–prey system incorporating a constant prey refuge, Int. J. Biomath. 5 (2012), pp. 1250021.
- S.M. Fu, X. He, L.N. Zhang, and Z.J. Wen, Turing patterns and spatiotemporal patterns in a tritrophic food chain model with diffusion, Nonlinear Anal. Real World Appl. 59 (2021), pp. 103260.
- U. Ghosh, A.A. Thirthar, B. Mondal, and P. Majumdar, Effect of fear, treatment, and hunting cooperation on an eco-epidemiological model: Memory effect in terms of fractional derivative, Iran. J. Sci. Technol. Trans. Sci. 46 (2022), pp. 1541–1554.
- E. González-Olivares and R. Ramos-Jiliberto, Dynamic consequences of prey refuges in a simple model system: More prey, fewer predators and enhanced stability, Ecol. Model 166 (2003), pp. 135–146.
- X.N. Guan, W.M. Wang, and Y.L. Cai, Spatiotemporal dynamics of a Leslie–Gower predator–prey model incorporating a prey refuge, Nonlinear Anal. Real World Appl. 12 (2011), pp. 2385–2395.
- L.N. Guin and S. Acharya, Dynamic behaviour of a reaction-diffusion predator–prey model with both refuge and harvesting, Nonlinear Dyn. 88 (2) (2017), pp. 1501–1533.
- L.N. Guin and H. Baek, Comparative study between prey-dependent and ratio-dependent predator–prey models relating to patterning phenomenon, Math. Comput. Simulat. 146 (2018), pp. 100–117.
- L.N. Guin, R. Murmu, H. Baek, and K.H. Kim, Dynamical analysis of a Beddington–DeAngelis interacting species system with harvesting, Math. Probl. Eng. 2020 (2020), pp. 7596394.
- L.N. Guin, S. Pal, S. Chakravarty, and S. Djilali, Pattern dynamics of a reaction–diffusion predator-prey system with both refuge and harvesting, Int. J. Biomath. 14(1) (2021), pp. 2050084.
- R.J. Han, L.N. Guin, and B.X. Dai, Cross-diffusion-driven pattern formation and selection in a modified Leslie–Gower predator-prey model with fear effect, J. Biol. Syst. 28(1) (2020), pp. 27–64.
- R.J. Han, L.N. Guin, and B.X. Dai, Consequences of refuge and diffusion in a spatiotemporal predator–prey model, Nonlinear Anal. Real World Appl. 60 (2021), pp. 103311.
- B.D. Hassard, N.D. Kazarinoff, and Y.H. Wan, Theory and Applications of Hopf Bifurcation, Cambridge University Press, Cambridge-New York, 1981.
- D.P. Hector, Cooperative hunting and its relationship to foraging success and prey size in an avian predator, Ethology. 73 (1986), pp. 247–257.
- D. Henry, Geometric Theory of Semilinear Parabolic Equations, Springer-Verlag, New York, 1981.
- M.E. Hochberg and R.D. Holt, Refuge evolution and the population dynamics of coupled host–parasitoid associations, Evolut. Ecol. 9 (1995), pp. 633–661.
- R.D. Holt, Population dynamics in two-patch environments: Some anomalous consequences of an optimal habitat distribution, Theor. Popul. Biol. 28 (1985), pp. 181–208.
- R.D. Holt and G.A. Polis, A theoretical framework for intraguild predation, The Amer. Natur. 149 (1997), pp. 745–764.
- L.L. Ji and C.Q. Wu, Qualitative analysis of a predator–prey model with constant-rate prey harvesting incorporating a constant prey refuge, Nonlinear Anal. Real World Appl. 11 (2010), pp. 2285–2295.
- T.K. Kar, Modelling and analysis of a harvested prey–predator system incorporating a prey refuge, J. Comput. Appl. Math. 185 (2006), pp. 19–33.
- V. Křivan, Effects of optimal antipredator behavior of prey on predator-prey dynamics: The role of refuges, Theor. Popul. Biol. 53 (1998), pp. 131–142.
- X. Li, W.H. Jiang, and J.P. Shi, Hopf bifurcation and turing instability in the reaction–diffusion Holling–Tanner predator–prey model, IMA J. Appl. Math. 78 (2013), pp. 287–306.
- Y. Lou and W.M. Ni, Diffusion, self-diffusion and cross-diffusion, J. Differ. Equ. 131 (1996), pp. 79–131.
- Y. Lou and W.M. Ni, Diffusion vs cross-diffusion: An elliptic approach, J. Differ. Equ. 154 (1999), pp. 157–190.
- D.W. Macdonald, The ecology of carnivore social behaviour, Nature 301 (1983), pp. 379–384.
- J.N. McNair, The effects of refuges on predator–prey interactions: A reconsideration, Theor. Popul. Biol. 29 (1986), pp. 38–63.
- N. Mukherjee and M. Banerjee, Hunting cooperation among slowly diffusing specialist predators can induce stationary turing patterns, Physica. A. 599 (2022), pp. 127417.
- X.Y. Meng and J. Li, Dynamical behavior of a delayed prey–predator-scavenger system with fear effect and linear harvesting, Int. J. Biomath. 14 (2021), pp. 2150024.
- X.Y. Meng, N.N. Qin, and H.F. Huo, Dynamics of a food chain model with two infected predators, Int. J. Bifur. Chaos 31 (2021), pp. 2150019.
- M.W. Moffett, Foraging dynamics in the group-hunting myrmicine ant, Pheidologeton diversus, J. Insect Behav. 1 (1988), pp. 309–331.
- C. Packer, D. Scheel, and A.E. Pusey, Why lions form groups: Food is not enough, The Amer. Natur.136 (1990), pp. 1–19.
- G. Polis, C. Myers, and R. Holt, The ecology and evolution of intraguild predation: Potential competitors that eat each other, Ann. Rev. Ecol. Syst. 20 (1989), pp. 297–330.
- G.D. Ruxton, Short term refuge use and stability of predator–prey models, Theor. Popul. Biol. 47 (1995), pp. 1–17.
- M. Sambath, K. Balachandran, and L.N. Guin, Spatiotemporal patterns in a predator–prey model with cross-diffusion effect, Int. J. Bifur. Chaos 28(2) (2018), pp. 1830004.
- N. Sapkota, R. Bhatta, P. Dabney, and Z. Xie, Hunting co-operation in the middle predator in three species food chain model, preprint (2020), pp. 1–7. Available at arXiv, 2006.16525.
- S. Sarwardi, P.K. Mandal, and S. Ray, Dynamical behaviour of a two-predator model with prey refuge, J. Biol. Phy. 39 (2013), pp. 701–722.
- P.A. Schmidt and L.D. Mech, Wolf pack size and food acquisition, The Amer. Natur. 150 (1997), pp. 513–517.
- Y.L. Song, Y.H. Peng, and T.H. Zhang, The spatially inhomogeneous hopf bifurcation induced by memory delay in a memory-based diffusion system, J. Differ. Equ. 300 (2021), pp. 597–624.
- D.X. Song, Y.L. Song, and C. Li, Stability and turing patterns in a predator–prey model with hunting cooperation and Allee effect in prey population, Int. J. Bifur. Chaos 30 (2020), pp. 2050137.
- R.J. Taylor, Predation, Springer, Dordrecht, 1984.
- V. Tiwari, J.P. Tripathi, S. Abbas, J.S. Wang, G.Q. Sun, and Z. Jin, Qualitative analysis of a diffusive Crowley–Martin predator–prey model: The role of nonlinear predator harvesting, Nonlinear Dyn. 98 (2019), pp. 1169–1189.
- A.M. Turing, The chemical basis of morphogenesis, Bull. Math. Biol. 52 (1990), pp. 153–197.
- G.W. Uetz, Foraging strategies of spiders, Trends Ecol. Evolut. 7 (1992), pp. 155–159.
- C.H. Wang, S.L. Yuan, and H. Wang, Spatiotemporal patterns of a diffusive prey–predator model with spatial memory and pregnancy period in an intimidatory environment, J. Math. Biol. 84 (2022), pp. 12.
- P. Wanjugi and V.J. Harwood, The influence of predation and competition on the survival of commensal and pathogenic fecal bacteria in aquatic habitats, Envir. Microb. 15 (2013), pp. 517–526.
- C. Xiang, J.C. Huang, and H. Wang, Bifurcations in Holling–Tanner model with generalist predator and prey refuge, J. Differ. Equ. 343 (2023), pp. 495–529.
- A.A. Yakubu, Prey dominance in discrete predator–prey systems with a prey refuge, Math. Biosci. 144 (1997), pp. 155–178.
- T.H. Zhang, Y.P. Xing, H. Zang, and M.A. Han, Spatio-temporal dynamics of a reaction-diffusion system for a predator–prey model with hyperbolic mortality, Nonlinear Dyn. 78 (2014), pp. 265–277.
- W. Zuo and Y.L. Song, Stability and double-hopf bifurcations of a Gause–Kolmogorov-type predator–prey system with indirect prey-taxis, J. Dyn. Differ. Equ. 33 (2021), pp. 917–957.