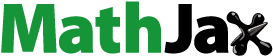
Abstract
Populations are generally censused daily, weekly, monthly or annually. In this paper, we introduce a discrete-time nutrients-phytoplankton-oysters (NPO) model that describes the interactions of nutrients, phytoplankton and oysters in a bay ecosystem. We compute the threshold parameter for persistence of phytoplankton with or without oysters. When
, then both phytoplankton and oysters populations go extinct. However, when
, we show that the model may exhibit two scenarios: (1) a locally asymptotically stable equilibrium with positive values of nutrients and phytoplankton with oysters missing, and (2) a locally asymptotically stable interior equilibrium with positive values of nutrients, phytoplankton and oysters. We use sensitivity analysis to study the impact of human and environmental factors on the model. We use examples to illustrate that some human activities and environmental factors can force the interior equilibrium to undergo a Neimark–Sacker bifurcation which generates phytoplankton blooms with oscillations in oysters population and nutrients level.
Mathematics Subject Classification:
1. Introduction
Estuaries, for example the Chesapeake Bay (the largest estuary in the US), provide diverse habitats for wildlife and aquatic life while shielding adjacent communities against flooding, decrease waterways and pollution. Furthermore, estuaries provide opportunities for commercial and recreational activities that support local economies [Citation2]. The observed decrease in the Chesapeake Bay's health is associated with the increase of the human population in its watershed [Citation3]. The large population causes waste, utilization of the resources and the consequent changes of the character of the land, water and air of the bay [Citation1]. For example, loss of habitat, overfishing and disease have depleted oyster population in the Chesapeake Bay. Excess nutrients cause algae blooms that block sunlight which endangers aquatic species. The excess nutrients and sedimentation threaten bay water quality [Citation1].
Interactions of nutrients and phytoplankton have been investigated in several research papers using continuous-time systems of ordinary differential equations models. For example, Fan and Glibert in [Citation12] and Hood et al. in [Citation14] used continuous-time models to study the impact of nutrients on the formation of phytoplankton blooms. A continuous-time model of nutrients-phytoplankton (NP) with phytoplankton bloom was analysed by Huppert et al. in [Citation15, Citation16]. In [Citation8–10, Citation13, Citation19, Citation21], nutrients-phytoplankton-zooplankton (NPZ) continuous-time models were studied. The authors demonstrated that a wide range of dynamical behaviours from equilibrium to oscillatory dynamics can be generated in NPZ models. Moreover, the stability analysis of a more general type of continuous-time nutrients-phytoplankton-oysters models was studied by Saijnders and Bazin [Citation20]. Ziyadi et al. in [Citation25], used sensitivity analysis in a continuous-time nutrients-phytoplankton-oysters (NPO) model to investigate the effect of human and environmental factors on the NPO model dynamics.
Populations are typically censused at discrete-time intervals, for example, daily, weekly, monthly or annually. To capture the discrete nature of population surveillance data, in this paper, unlike in [Citation25], we introduce a discrete-time nutrients-phytoplankton-oysters model that describes nutrients, phytoplankton and oysters interaction dynamics in a bay ecosystem. The model is a system of three difference equations. In our NPO model, we use the threshold parameter, , to study persistence of phytoplankton with or without oysters in the system. In particular, we obtain that when
, then both phytoplankton and oysters go extinct. However, when
, we show that the model is capable of exhibiting two scenarios: (1) oyster population goes extinct or the occurrence of a locally asymptotically stable equilibrium point with positive values of nutrients level and phytoplankton biomas with oysters missing, and (2) the nutrients, phytoplankton and oyster persist or the occurrence of a locally asymptotically stable interior equilibrium with positive values of nutrients, phytoplankton and oysters. As in [Citation25], we use sensitivity analysis to study the impact of human and environmental factors on the discrete-time NPO model.
This paper is organized as follows: In Section 2, we introduce the discrete-time mathematical model that describes interaction dynamics of nutrients, phytoplankton and oysters in a bay ecosystem. We establish the boundedness of nutrients level and all populations in Section 3. In Section 4, we give the condition for persistence of nutrients in the model. In addition, we give an illustrative example that shows that increasing model parameters, such as nutrients flow, can force persistence of the phytoplankton population. Furthermore, we perform sensitivity analysis. In Section 5, we give conditions for persistence of both nutrients and phytoplankton in the model. Also, we give an illustrative example that shows that increasing model parameters, such as nutrients flow, can force persistence of oysters population. In Section 6, we present illustrative examples showing that changing model parameters such as increasing the flow rate of nutrients or increasing the survival of phytoplankton can lead to the occurrence of Neimark–Sacker bifurcation. Finally, we summarize our results in the Discussion section.
2. Discrete-time nutrients-phytoplankton-oysters model
In this section, we construct the discrete-time NPO model. However, we first list the model variables and parameters in Tables and , respectively.
Table 1. List of model variables.
Table 2. Table of model parameters.
In [Citation25], Figure was used to construct a continuous-time ordinary differential equations model for the interactions of nutrients, phytoplankton and oysters in a bay ecosystem.
Next, we use Figure together with the variables and parameters of Tables and to introduce a discrete-time NPO model in three steps. First, we introduce a discrete-time equation that describes the nutrients level of our discrete-time NPO model.
2.1. (i) Nutrients level equation
The nutrients level at time in the bay ecosystem is modelled by Equation (Equation1
(1)
(1) ),
(1)
(1) for
, where
,
and
. In the unit time interval, first term of Equation (Equation1
(1)
(1) ) represents the proportion of nutrients flow that was not consumed by phytoplankton, and second term models nutrients not consumed by phytoplankton and not lost to sinking and denitrification. As in the continuous-time NPO model of [Citation25], when there are no phytoplankton and
, then the nutrients level is asymptotically constant in model (Equation1
(1)
(1) ).
Figure 1. Interactions of nutrients, phytoplankton and oysters in a bay ecosystem [Citation25].
![Figure 1. Interactions of nutrients, phytoplankton and oysters in a bay ecosystem [Citation25].](/cms/asset/770d4e00-eb9e-4b06-a3f5-343f18127786/tjbd_a_2242720_f0001_oc.jpg)
In step 2, we introduce the discrete-time equation that describes the phytoplankton biomass at time .
2.2. (ii) Phytoplankton biomass equation
The phytoplankton biomass at time in the bay ecosystem is modelled by Equation (Equation2
(2)
(2) ),
(2)
(2) for
, where
,
and
. In the unit time interval, first term of Equation (Equation2
(2)
(2) ) represents gain of phytoplankton from nutrients uptake while escaping from oysters filtration, and second term represents phytoplankton not consumed by oysters and not lost to sinking and predation. As in the continuous-time NPO model of [Citation25], the phytoplankton population goes extinct when
and the nutrients level is very low.
In step 3, we introduce the discrete-time equation that describes the oysters biomass at time .
2.3. (iii) Oysters biomass equation
The oysters biomass at time in the bay ecosystem is modelled by Equation (Equation3
(3)
(3) ),
(3)
(3) for
where
, and
. In the unit time interval, first term of Equation (Equation3
(3)
(3) ) represents the proportion of oysters gained by filtration of phytoplankton, and second term models the oysters not lost to mortality, harvest, disease and respiration at time
. Also, as in the continuous-time NPO model of [Citation25], the oyster population goes extinct when
and there are no phytoplankton.
From Equations (Equation1(1)
(1) ), (Equation2
(2)
(2) ) and (Equation3
(3)
(3) ), our discrete-time NPO model is the following system of three difference equations.
(NPO)
(NPO) where
, with non-negative initial conditions
,
and
. NPO model predicts the vectors of population levels of nutrients, phytoplankton and oysters at time
,
, from knowledge of their respective levels at time t,
, where
. The unit of time depends on the specific application. Baseline values for the discrete-time NPO model parameters are given in Table .
3. Boundedness of orbits
In this section, we establish the well-posedness of the discrete-time NPO model. That is, we show that the discrete-time NPO model has no unbounded growth for all non-negative initial conditions.
Let
(4)
(4) for
. The set of density sequences generated by Model (Equation4
(4)
(4) ) is equivalent to the set of iterates of the map
defined by
Lemma 3.1
In Model (Equation4(4)
(4) ), if
,
and
, then
,
and
for all
. Furthermore, every non-negative initial condition has a bounded orbit in Model (Equation4
(4)
(4) ).
Proof.
Clearly, ,
and
whenever
,
and
.
The inequalities for
imply, by induction argument, that
where
is the solution of the initial value problem
Because
, this linear difference equation has a globally attracting equilibrium
, i.e.
. Thus,
which implies
is bounded. Let
be a bound for
, i.e.
for all
.
From for
, we conclude, by an analogous argument, that
is bounded.
Finally, by an analogous argument used for , one can argue that
is bounded.
Hence, the orbit of every non-negative initial condition is bounded in Model (Equation4(4)
(4) ).
4. Nutrients-only equilibrium point: stability and sensitivity analysis
Next, we compute the nutrients-only equilibrium point of the NPO model. Then, we establish conditions for its stability and perform local and global sensitivity analysis.
Model NPO has a nutrients-only equilibrium point
4.1. 
: stability
To study the stability of , we introduce the threshold parameter
Note that
.
is equivalent to
and
is equivalent to
.
When γ is sufficiently ‘small’, then , and
is locally asymptotically stable. However, when γ is ‘large’, then
, and
is unstable. Using baseline parameter values of Table ,
. When
, the Model NPO has an only oysters-free equilibrium point. We summarize this stability result in the following theorem.
Theorem 4.1
In Model NPO, the phytoplankton-, oysters-free boundary fixed point, , is locally asymptotically stable and small initial nutrients, phytoplankton and oysters levels lead to extinction of phytoplankton and oysters when
. However,
is unstable when
.
Proof.
The Jacobian matrix of the NPO model evaluated at is
The eigenvalues of
are:
,
and
. Since
and
, the stability of
is determined by
.
Hence,
implies
is locally asymptotically stable and
implies
is unstable.
Corollary 4.2
In Model NPO, the phytoplankton-, oysters-free boundary equilibrium point, , is globally asymptotically stable and independently of positive initial population numbers, both phytoplankton and oysters go extinct when
.
Proof.
Since and
,
Since
this implies, for arbitrary
, there exists a time
such that
for all
. Since
it follows that
and we can choose ε so small that
(5)
(5) Since
which for
implies
and, by (Equation5
(5)
(5) ), that
.
Since , then
such that
,
. Hence,
.
By Theorem 4.1, is a locally asymptotically stable boundary equilibrium point of Model NPO. Hence,
is globally stable when
.
4.2. 
: sensitivity analysis
Next, we use the local sensitivity analysis index to estimate the relative change in a state variable caused when the value of a specific model parameter changes. Consequently, as in [Citation7, Citation23–26], we use the following definition of normalized forward sensitivity index to carry out the local sensitivity analysis and compute normalized sensitivity indices.
Definition 4.3
[Citation7]
The normalized forward sensitivity index of a variable, u, that depends differentiably on a parameter, q, is defined as:
We summarize the computations of the normalized sensitivity indices of equilibrium nutrients level at in Table .
Table 3. Normalized sensitivity indices of equilibrium nutrients level at to parameters evaluated at baseline parameter values of Table and their order of importance.
Sensitivity indices of Table indicate that increasing (respectively, decreasing) nutrients flow, γ, by will increase (respectively, decrease) equilibrium nutrients level at
by
. Increasing (respectively, decreasing) escapement rate of nutrients,
, will increase (respectively, decrease) nutrients equilibrium level at
, by
.
Sensitivity indices of Table suggest that nutrients level in the nutrients-only equilibrium has two important parameters: (1) flow rate of nutrients, γ and (2) escapement rate of nutrients, . This is consistent with the sensitivity analysis results in [Citation25] for the continuous-time NPO model that nutrients level in the phytoplankton- and oyster-free only equilibrium also has two important parameters: (1) nutrients flow and (2) nutrients loss [Citation25].
Now, we use the global sensitivity analysis at to measure the effect generated by one specific model parameter on a model variable, while all the other model parameters vary [Citation5, Citation6, Citation18]. Similar to the global sensitivity approach used in [Citation25], we adopt the ANOVA (ANalysis Of VAriance) decomposition-based method called Sobol' sensitivity method [Citation22]. As in [Citation25], we perform the global sensitivity analysis using Sobol' method with the free software tool, Global Sensitivity Analysis Toolbox (GSAT) [Citation4]. GSAT is performed using the Matlab software. We compute Sobol' sensitivity indices of type first-order and total-order. The samplings of our model parameter values are based on quasi-random sequences and are used to run 200,000 simulations. The ranges of model parameter are given in Table .
Sobol' sensitivity results of Table indicate that the escapement rate of nutrients, , is the most important parameter and contributes about
of the nutrients equilibrium level at
total variance. There is no large difference between first and total Sobol' index for each parameter. Therefore, there is no significant interaction between the parameters. However, phytoplankton biomass and oysters biomass at the fixed point
are not sensitive to changes in parameter values, and therefore remain fixed at zero. This is in contrast to the Sobol' sensitivity results for the continuous-time NPO model [Citation25] where nutrients flow and nutrients loss are the most important parameters and contribute respectively about
and
to the total variance of the nutrients level in the nutrients-only equilibrium.
4.3. 
: sensitivity analysis
Now, we use sensitivity analysis to study the impact of parameter changes on .
Table 4. First-order and total-order Sobol sensitivity indices of equilibrium nutrients level at , related to changes in parameters with ranges listed in Table and their order of importance.
Sensitivity indices of Table show that increasing (respectively, decreasing) the escapement rate of nutrients, , by
will increase (respectively, decrease)
by
. Increasing (respectively, decreasing) survival rate of phytoplankton,
, will increase (respectively, decrease)
, by
.
Note that, because the list of parameters for the continuous- and discrete-time NPO models are not identical, the threshold parameter is different for the two models [Citation25]. However, in both models when
, nutrients persist while phytoplankton and oysters population go extinct. However, when
both nutrients and phytoplankton persist with or without oysters.
Table 5. Normalized sensitivity indices of , to parameters evaluated at baseline of Table and their order of importance.
Sensitivity indices of Table show that the threshold parameter has two important parameters: (1) escapement rate of nutrients,
, (2) survival rate of phytoplankton,
. While sensitivity analysis of in [Citation25] for the continuous-time NPO model show that
has two important parameters: (1) removal of phytoplankton by sinking and predation and (2) the maximum nutrients uptake by phytoplankton. In Table , we summarize our results on the global sensitivity analysis of
.
Table 6. First-order and total-order Sobol sensitivity indices related to changes in parameters with ranges listed in Table and their order of importance.
Sobol' sensitivity results of Table show that escapement rate of nutrients, , contributes
and the survival rate of phytoplankton,
, contributes
of variance of
. Also, there is a large difference between first and total Sobol' indices for nutrients flow, γ, nutrients escapement rate,
, phytoplankton survival rate,
and nutrients uptake by phytoplankton, α. Hence, each of these parameters interact strongly with the others.
Sobol' sensitivity results of Table illustrate that has two important parameters: (1) escapement rate of nutrients and (2) survival rate of phytoplankton. While Sobol' sensitivity results in [Citation25] for the continuous-time NPO model showed that
has two important parameters: (1) removal of phytoplankton by sinking and predation and (2) the maximum nutrients uptake by phytoplankton.
4.4. Illustrative example: 
and 

Now, we use the following specific example to illustrate the stability of the nutrients-only equilibrium point, , in Model NPO.
Example 4.1
In Model NPO, let ,
,
,
,
and
.
In Example 4.1, . As predicted, by Corollary 4.2, Figure shows that
is globally asymptotically stable.
To study the impact of high nutrients inflow on Figure , we increase γ from to
and keep all the other parameters fixed at their current values in Example 4.1. Then
increases from
to
. Figure shows that
is unstable and the initial condition
limits on an only oysters-free equilibrium point
. Thus, high nutrients flow promotes the growth of phytoplankton population.
5. Oysters-free equilibrium point: stability
By Corollary 4.2, implies
is globally asymptotically stable and Model NPO has no equilibrium points with positive levels of phytoplankton and oysters. To ensure persistence of phytoplankton, we only consider
. In this case, Model NPO has an only oysters-free equilibrium point with positive level of phytoplankton,
; where
and
are positive the solutions of
(6)
(6) That is, at
,
(7)
(7) where
and
are positive.
Figure 3. and Model NPO has an unstable nutrients-only equilibrium point,
and an asymptotically stable only oysters-free equilibrium
, where
,
,
,
,
and
.

Let
Notice that
. Clearly,
and
for all
. Hence, the graph of
is increasing and concave down in
. Also,
and
for all
. Hence, the graph of
is increasing and concave up in
. Since,
and
,
implies
. Since
and
, using the monotonicity and concavity of the graphs of
and
, we obtain that the graphs of
and
intersect at exactly one point,
, in
, whenever
(see Figure ). That is
implies Model NPO has a unique oysters-free equilibrium point,
, with positive level of nutrients and phytoplankton. We summarize this in the following result.
Theorem 5.1
When , Model NPO has a unique oysters-free equilibrium point,
, where
and
are the unique positive solutions of (Equation6
(6)
(6) ) and (Equation7
(7)
(7) ).
5.1. 
: stability
In this section, the asymptotic stability of the oysters-free equilibrium will be analysed. We claim the following result:
Theorem 5.2
Let and
, where
and
are solutions of (Equation6
(6)
(6) ) and (Equation7
(7)
(7) ), and the following two inequalities are satisfied.
,
Then, the oysters-free equilibrium point, , is asymptotically stable and small initial nutrients, phytoplankton and oysters levels lead to extinction of oysters. However,
is unstable and persistence of nutrients, phytoplankton and oysters occur, when either inequality (i) or (ii) is not satisfied.
Proof.
To study stability of the oysters-free equilibrium point, , we compute the eigenvalues of the Jacobian matrix of the model evaluated
, where
and
are solutions of (Equation6
(6)
(6) ) and (Equation7
(7)
(7) ). The Jacobian matrix of NPO model evaluated at
is
Hence,
has three eigenvalues
,
and
, where
and
are the eigenvalues of the matrix
.
Since the model parameters are considered to be positive, then
which means that
is equivalent to the condition (i). By the Jury stability criteria [Citation11, Citation17],
where
is the trace of A and
is determinant of A.
, then
Which means that
are equivalent to condition (ii). When
then
is asymptotically stable.
is unstable when either of the conditions is not satisfied. Hence the result of the Theorem.
5.2. Illustrative example: 
and 

Now, we use the following specific example to illustrate the stability of the oysters-free equilibrium point, , in Model NPO.
Example 5.1
In Model NPO, let ,
,
,
,
and
.
In Example 5.1, and the Jacobian matrix of NPO model evaluated at
,
has three eigenvalues,
,
and
. Note that,
and
. So conditions (i) and (ii) of Theorem 5.2 are satisfied. As predicted by Theorem 5.2, Figure shows that the only oysters-free equilibrium point,
, is locally asymptotically stable.
To study the impact of high nutrients flow on Figure , we increase γ from to
and keep all the other parameters fixed at their current values in Example 5.1. Then
increases form
to
. The eigenvalues of the Jacobian matrix evaluated at
,
, are
,
and
. Note that
. So, condition (i) of Theorem 5.2 is not satisfied. As predicted by Theorem 5.2, Figure shows that
is unstable and the initial condition
limits at the interior equilibrium point
. Thus, high nutrients flow promotes phytoplankton growth which serves as food for the survival of the oysters.
6. Interior equilibrium point: stability
To find the interior equilibrium point of Model NPO, , we solve
(8)
(8) for
.
Figure 6. and Model NPO has an unstable oysters-free equilibrium point,
and a stable interior equilibrium point at
, where
,
,
,
,
and
.

Then
Thus,
That is,
By substituting for O in the third equation of (Equation8
(8)
(8) ), we obtain
Let
Notice that
and
for all P>0 and
. When
, then
and
for all
. Consequently the graphs of
and
intersect at exactly one point,
, in
whenever
(see Figure ). That is
implies Model NPO has a unique interior equilibrium point
where
(9)
(9) We summarize this in the following results.
Figure 7. When ,
,
,
,
and
then, the functions
and
intersect at P = 4.6189. Hence, Model NPO has an interior equilibrium point
.

Theorem 6.1
When , Model NPO has a unique interior equilibrium point,
, with positive levels of nutrients, phytoplankton and oysters, where
is the solution of (Equation9
(9)
(9) ) in
.
6.1. Illustrative example: 
and Neimark–Sacker bifurcation
An analysis of the local stability of the interior equilibrium point, is not tractable, so we show, by numerical examples, that
can be either stable or unstable.
We evaluate the Jacobian matrix of the model evaluated at ,
.
where
,
and
are solutions of (Equation9
(9)
(9) ).
is locally asymptotically stable if
for all the eigenvalues, λ, of
. Otherwise,
is unstable.
Now, we use the following two specific examples to illustrate the stability of the interior equilibrium point, , of Model NPO.
Example 6.1
In Model NPO, let ,
,
,
,
and
.
In Example 6.1, and the Jacobian matrix of NPO model evaluated at
,
, has three eigenvalues,
,
and
. Note that,
and
. Figure shows that the interior equilibrium point,
, is locally asymptotically stable. That is, small initial nutrients, phytoplankton and oysters values close to
levels lead to the persistence of nutrients, phytoplankton and oysters in Model NPO at
.
To study the impact of high nutrients flow on , we increase γ from to
and keep all the other parameters fixed at their current values in Example 6.1. Then
increases from
to
,
. Figure illustrates the emergence of a Neimark–Sacker closed curve. As γ increase to
, the interior equilibrium point undergoes a Neimark–Sacker bifurcation.
High flow of nutrients into a bay ecosystem is capable of forcing phytoplankton blooms with oscillations in oysters via Neimark–Sacker bifurcation.
Figure 8. Model NPO has a locally asymptotically stable interior equilibrium point, , where
,
,
,
,
and
.

Example 6.2
In Model NPO, let ,
,
,
,
and
.
Figure 9. Neimark–Sacker bifurcation closed curve in N-P, N-O and P-O planes at , where the other parameters are fixed at their current values in Example 6.1.

In Example 6.2, and the Jacobian matrix of NPO model,
has three eigenvalues,
,
and
. Note that,
and
. Figure shows that the interior equilibrium point,
, is locally asymptotically stable. That is, small initial nutrients, phytoplankton and oysters close to
levels lead to the persistence of nutrients, phytoplankton and oysters in Model NPO at
.
To illustrate the instability of in Example 6.2. and Figure , we increase
from
to
and keep all the other parameters fixed at their current values in Example 6.2. Then
increases from
to
,
. Figure illustrates the emergence of a Neimark–Sacker bifurcation.
7. Discussion
In this paper, we introduced a discrete time mathematical model and used it to study the dynamics of interactions of nutrients, phytoplankton and oysters occurring in a bay ecosystem. We established that when the threshold level , then extinction of both populations of phytoplankton and oysters is attained and the discrete time model stabilizes with a positive value of nutrients. Also, we showed that when
, the discrete time model is capable of exhibiting: (a) an equilibrium point where only nutrients and phytoplankton persist while oysters go extinct and (b) an equilibrium point where nutrients, phytoplankton and oysters are all persistent (interior equilibrium point). However, when
, both equilibrium points with oysters only missing and interior equilibrium point are given implicitly as solutions of systems (Equation6
(6)
(6) ) and (Equation8
(8)
(8) ), respectively. Furthermore, we presented a set of conditions in Theorem 5.2 that guarantee the local stability of the oysters-free only equilibrium point.
Figure 11. A Neimark–Sacker bifurcation closed curve emerges in N-P, N-O and P-O planes at , where the other parameters are fixed at their current values in Example 6.2.

We performed sensitivity analysis on the nutrients-only equilibrium point. Results of the local sensitivity analysis are the following:
Increasing nutrients flow, γ, by
will increase nutrients level at equilibrium by
.
Increasing escapement rate of nutrients,
, will increase nutrients level at equilibrium by
.
Results of the global sensitivity analysis using Sobol' method at the phytoplankton- and oysters- free equilibrium point are the following:
Escapement rate of nutrients,
, is the most important parameter and contributes about
of the total variance at the the nutrients equilibrium level.
Sensitivity analysis of both the oysters-free only equilibrium and the interior equilibrium was not performed due to the lack of explicit formulas of the oysters-free only equilibrium. We used examples to illustrate that human activities that lead to increase nutrients flow rate and increase phytoplankton survival in the bay ecosystem can force the interior equilibrium point to undergo a Neimark–Sacker bifurcation which generates phytoplankton blooms with corresponding oscillations in oysters population and nutrients level. Similar oscillatory bifurcations were also observed in the continuous time model for nutrients-phytoplankton-oysters interactions [Citation25], where increasing either nutrients flow or phytoplankton removal rates forced the interior equilibrium point to undergo Hopf bifurcation.
The discrete-time and continuous-time NPO model have similarities that we summarize below:
The nutrients level is asymptotically constant when there are no phytoplankton and
.
The phytoplankton population goes extinct when
and the nutrients level is very low.
The oyster population goes extinct when
and there are no phytoplankton.
The threshold parameter
formulas are different in the two models. However, in both models when
, then nutrients persist while phytoplankton and oysters population go extinct, and when
, then both nutrients and phytoplankton persist with or without oysters.
Results of local sensitivity indices for the nutrients-only equilibrium is consistent in both models.
Dynamics behaviours of discrete-time and continuous-time NPO model can range from equilibrium to oscillatory dynamics via bifurcation.
However, results of Sobols' sensitivity indices of the nutrients-only equilibrium showed that the flow of nutrients contribute to the total variance in the continuous-time NPO model while it does not contribute in the discrete-time NPO model.
This discrete-time NPO model is a first step in using difference equations to model interactions of nutrients, phytoplankton and oysters in a bay ecosystem. For simplicity, the model does not take into account seasonality, temperature, light which play a role in the feeding patterns of phytoplankton and oyster populations. Including some of these factors in the discrete-time NPO model is an open question.
Acknowledgement
I am very thankful to Dr. Abdul-Aziz Yakubu for helpful discussions during the early stages of the project, particularly in the formulation of the model.
Disclosure statement
No potential conflict of interest was reported by the author(s).
Additional information
Funding
References
- Chesapeake Bay: Introduction to an ecosystem, Available at https://www.chesapeakebay.net/documents/0633_001.pdf.
- Chesapeake Bay program, Bay 101, Available at https://www.chesapeakebay.net/discover/bay-101.
- Chesapeake Bay program, population, Available at https://www.chesapeakebay.net/state/population.
- Global sensitivity analysis toolbox, Available from: http://www.mathworks.com/matlabcentral/fileexchange/40759-global-sensitivity-analysis-toolbox.
- F. Cannavó, Sensitivity analysis for volcanic source modeling quality assessment and model selection, Comput. Geosci. 44 (2012), pp. 52–59.
- J. Cariboni, D. Gatelli, R. Liska, and A. Saltelli, The role of sensitivity analysis in ecological modelling, Ecol. Modell. 203 (2007), pp. 167–182.
- N. Chitnis, J.M. Hyman, and J.M. Cushing, Determining important parameter in the spread of malaria through the sensitivity analysis of mathematical model, Bull. Math. Biol. 70 (2008), pp. 1272–1296.
- A.M. Edwards and M. Bees, Generic dynamics of a simple plankton population model with a non-integer exponent of closure, Chaos Solit. Fractals 12 (2001), pp. 289–300.
- A.M. Edwards and J. Brindley, Oscillatory behavior in a three-component plankton population model, Dyn. Stab. Syst. 11 (1996), pp. 347–370.
- C.A. Edwards, T.A. Powell, and H.P. Batchelder, The stability of an NPZ model subject to realistic levels of vertical mixing, J. Mar. Res. 58 (2000), pp. 37–60.
- S.N. Elaydi, Discrete Chaos, Chapman & Hall/CRC, 2000.
- C. Fan and P. Glibert, The importance of reduced nitrogen in a natural prorocentrum minimum bloom – a model approach, Ecol. Sci. 22 (2003), pp. 199–204.
- P. Franks, NPZ models of plankton dynamics: Their construction, coupling to physics, and application, J. Oceanogr. 58 (2002), pp. 379–387.
- R.R. Hood, X. Zhang, P.A. Glibert, M.R. Roman, and D.K. Stoecker, Modeling the influence of nutrients, turbulence and grazing on Pfiesteria population dynamics, Harmful. Algae. 5 (2006), pp. 459–479.
- A. Huppert, B. Blasius, and L. Stone, A model of phytoplankton bloom, Am. Nat. 159 (2002), pp. 156–171.
- A. Huppert, B. Blasius, R. Olinky, and L. Stone, A model of seasonal phytoplankton blooms, J. Theor. Biol. 236 (2005), pp. 276–290.
- E.R. Lewis, Network Models in Population Biology, Springer-Verlag, Berlin and Heidelberg, 1977.
- A.M. Lutambi, M.A. Pennya, T. Smith, and N. Chitni, Mathematical modelling of mosquito dispersal in a heterogeneous environment, Math. Biosci. 241 (2013), pp. 198–216.
- S.G. Ruan, Oscillations in plankton models with nutrients recycling, J. Theor. Biol. 208 (2001), pp. 15–26.
- P.T. Saijnders and M.J. Bazin, On the stability of food chaines, J. Thoer. Biol. 52 (1975), pp. 121–142.
- J.E. Truscott and J. Brindley, Equilibria, stability and excitability in a general class of plankton population models, Phil. Trans R. Soc. Lond. 347 (1994), pp. 703–718.
- X.Y. Zhang, M.N. Trame, L.J. Lesko, and S. Schmidt, Sobol sensitivity analysis: A tool to guide the development and evaluation of systems pharmacology models, CPT Pharmacometrics Syst. Pharmacol. 4 (2015), pp. 69–79.
- N. Ziyadi, Local and global sensitivity analysis of ℜ0 in a male and female human papillomavirus (HPV) epidemic model of moroccans, Int. J. Evol. Equ. 9 (2014), pp. 337–356.
- N. Ziyadi, A male–female mathematical model of human papillomavirus (HPV) in African American population, Math. Biosci. Eng. 14 (2017), pp. 339–358.
- N. Ziyadi, K. Clark, C. Fan, and A. Nkwanta, Local and global sensitivity analysis of a nutrients-phytoplankton-oysters mathematical model of a bay ecosystem, Int. J. Biomath. Syst. Biol. 2 (2016). https://biomathsociety.in/issue3/paper2.pdf
- N. Ziyadi and A.-A. Yakubu, Local and global sensitivity analysis in a discrete-time SEIS epidemic model, Adv. Dyn. Syst. Appl. 11 (2016), pp. 15–33.