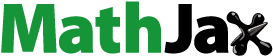
Abstract
The COVID-19 epidemic has been spreading around the world for nearly three years, and asymptomatic infections have exacerbated the spread of the epidemic. To analyse and evaluate the role of asymptomatic infections in the spread of the epidemic, we establish an improved COVID-19 infectious disease dynamics model. We fit the epidemic data in the four time periods corresponding to the selected 614G, Alpha, Delta and Omicron variants and obtain the proportion of asymptomatic persons among the infected persons gradually increased and with the increase of the detection ratio, the cumulative number of cases has dropped significantly, but the decline in the proportion of asymptomatic infections is not obvious. Therefore, in view of the hidden transmission of asymptomatic infections, the cooperation between various epidemic prevention and control policies is required to effectively curb the spread of the epidemic.
1. Introduction
Since its outbreak at the end of 2019, COVID-19 has had a severe impact on countries around the world. How to effectively control the spread of the epidemic and restore normal production and life is still an issue that governments need to consider. To control the spread of COVID-19, many measures have been taken, including non-pharmacological interventions, pharmacological interventions, vaccinations, and more. However, due to the continuous emergence of variants, the epidemic prevention measures did not achieve the expected effect.
There are a large number of contagious asymptomatic infections during the spread of COVID-19 [Citation1–4]. This feature determines the high concealment of the spread of COVID-19, and leads to an increase in the difficulty of epidemic prevention and control. There have been a large number of research results on asymptomatic infections of new coronary pneumonia, including age distribution, average proportion, and transmission intensity [Citation5–7]. In addition, some researchers have studied the spread of COVID-19 by establishing dynamic models including asymptomatic infections. Ruan et al. established a time-varying COVID-19 transmission compartment model including asymptomatic infected persons, simulated and reviewed the development process of the Wuhan epidemic, and obtained that the asymptomatic proportion of infected persons was about 20% [Citation8]. Rahul Subramanian et al. established a COVID-19 transmission model including asymptomatic infections, quantified asymptomatic infections in New York City, and obtained asymptomatic infections accounted for about 60% [Citation9]. Mohamed Amouch et al. proposed a new epidemiological mathematical model of the spread of COVID-19 disease, fitted the outbreak in Monaco, and obtained that the proportion of asymptomatic patients was 30% [Citation10]. However, these studies are all conducted for a specific variant, and cannot effectively reflect the changes caused by variant iterations.
In the more than two years since the first appearance of COVID-19, many countries and regions around the world have experienced repeated outbreaks. Taking England as an example, although multiple rounds of lockdown measures have been adopted to control the spread of the epidemic, such epidemic prevention measures have not been fully effective. When the epidemic prevention measures were gradually lifted, the epidemic rebounded again. We believe that asymptomatic infections have played a very important role in the rebound of the epidemic. The lockdown measures have effectively reduced the number of infected people to a certain extent, but because large-scale screening tests were not adopted, there were still a certain number of asymptomatic infections in the population. After the epidemic prevention measures are lifted, these undetected asymptomatic infections will cause the next round of outbreaks. If the number of asymptomatic infections can be effectively estimated in the early stage of the next outbreak, and certain epidemic prevention and control measures are taken, the spread of the epidemic can be delayed to a certain extent. We establish an improved SEIAR infectious disease dynamic model to assess the role of asymptomatic infections in the early stages of epidemic transmission.
The remainder of this article is organized as follows. In Section 2, we present the COVID-19 infectious disease model and analyse the existence and stability of equilibriums. In the third section, we use the established model to fit the actual epidemic data, and conduct a comparative analysis of the relevant kinetic parameters. In the last section, we conclude and discuss.
2. Model establishment and analysis
2.1 Model establishment
There are two typical characteristics of the spread of COVID-19. One is a large number of asymptomatic infected people with COVID-19, the other is that people with COVID-19 have a roughly 3–7 d exposure period and can show strong contagion two days before the patient shows symptoms [Citation11,Citation12]. Based on our knowledge of COVID-19, we have the following assumptions in our model:
(A1) We divide the exposure period into two parts: the early stage and the later stage, in which the early stage is not infectious and the later stage is infectious;
(A2) For symptomatic infections, we assume that symptomatic infections can be screened as long as they are tested, and that the detected symptomatic infections are completely isolated and no longer contagious;
(A3) Asymptomatic infections were assumed not to be tested, and deaths from illness in asymptomatic infections were not considered.
Based on the above assumptions, we establish a COVID-19 transmission compartment model including asymptomatic infected persons. The model divides the general population into susceptible (), pre-exposure patients (
), late-exposure patients (
), detected symptomatic infections (
), undetected symptomatic infections (
), asymptomatic infections (
) and recovered (
). Its dynamic flow chart is shown in Figure .
Figure 1. Flow chart of the COVID-19 transmission dynamic model containing seven compartments. The compartments represented by the red box are infectious, and the compartments represented by the blue box are not infectious.

The corresponding propagation dynamic equation is constructed as follows:
(1)
(1) The parameters in system (1) are explained as follows.
is the daily number of births and
is the daily natural mortality rate. The parameter
represents the basal transmission rate, and
represents the conversion rate from patients in the pre-exposure period to patients in the late-exposure period.
is the conversion rate from patients in the pre-exposure period to asymptomatic infected persons, and
represents the conversion rate from patients in the late exposure period to infected persons.
is the weight of the transmission intensity of the asymptomatic infection relative to the symptomatic infection, and
is the proportion of testing among the symptomatic infection and the value interval is [0,1].
is the recovery rate of detected symptomatic infections, undetected symptomatic infections, and asymptomatic infections, respectively.
is the mortality rate of detected symptomatic infections and undetected symptomatic infections, respectively. In order to maintain biological significance, the dynamic parameters above are positive real numbers.
2.2 Calculation of disease-free equilibrium and control reproduction number
The domain of the solution of the system (1) is
Obviously, there is always a disease-free equilibrium
in system (1), where
. Then we use the next generation matrix method to calculate the control reproduction number of system (1). The Jacobian matrices
and
at the disease-free equilibrium are obtained from system (1) as
The control reproduction number is the spectral radius of , therefore we get.
2.3 Existence of the endemic equilibrium
Theorem 2.3.
When , the system (1) has a unique positive equilibrium point
.
Proof. Using the equilibrium equation of system (1) at the endemic equilibrium, we can get
(2)
(2)
Furthermore, according to the equilibrium equation, we obtain
Using equation (2) we can simplify the above equation to
Therefore, we can get
where
Therefore, has a unique positive root when
. According to equation (2), we can know that there is a unique positive equilibrium
of system (1) at this time.
2.4 Stability
Theorem 2.4.1.
When , the disease-free equilibrium
of system (1) is locally asymptotically stable.
Proof. We refer to the method of proof for stability in [Citation13, Citation14]. Let the Jacobian matrix of system (1) at the disease-free equilibrium be
. The matrix
is detailed in the Appendix A. When
, assume that there is
satisfied
, and
. Expanding the characteristic equation, we can obtain.
(3)
(3) Where
Since is assumed, the characteristic equation (3) can be simplified to.
The necessary condition for the above formula to be established is
(4)
(4)
Furthermore, assumed , we can obtain.
(5)
(5)
Therefore, in the case of , it can be known from inequality (5) that
(6)
(6)
At this point, it can be seen that there is a contradiction between inequalities (4) and (6), so the initial assumption about is invalid, that is, all the characteristic roots of the characteristic equation
have negative real parts. That is to say, when
, the disease-free equilibrium
of system (1) is locally asymptotically stable.
Theorem 2.4.2.
When , the disease-free equilibrium
of system (1) is globally asymptotically stable.
Proof. Define function
For any
there is
, and
if and only if
.
Let be the solution of system (1) through any
. Clearly,
is bounded and
holds for any
. Referring to the construction method in [Citation15], define a function
on
as follows,
The derivative of with respect to
along
is
Hence when , we have
, which means that
is a Lyapunov function on
. And
if and only if
. Therefore, the largest invariant set of
is
. According to the LaSalle's Invariance Principle, when
, the disease-free equilibrium
of system (1) is globally asymptotically stable.
Theorem 2.4.3.
When , the disease-free equilibrium
of system (1) is unstable.
Proof. Similar to the proof method in Theorem 2.4.1, when , assumed that there is
satisfied
, and
. Expanding the characteristic equation, we can obtain.
(7)
(7) where
The characteristic equation (7) can be simplified to
(8)
(8)
To simplify the subsequent formulation, redefine the notation as
Equation (8) is now rewritten as.
The result of expanding the above formula is
Where
Define function
Under the new notation, the control reproduction number is
Therefore, when
, there is
In addition, there is , so equation (8) has at least one positive root. That is to say, the characteristic equation
has at least one positive root, that is, the assumption is true. This indicates that, when
, the disease-free equilibrium
of system (1) is unstable.
Theorem 2.4.4.
When , the system (1) is locally asymptotically stable at the endemic equilibrium
.
Proof. Let the Jacobian matrix of system (1) at the endemic equilibrium be
. The matrix
is detailed in the Appendix B. When
, assume that there is
satisfying
, such that
. Expanding the characteristic equation, we can obtain.
(9)
(9) where,
The characteristic equation (9) can be simplified to
Using equation (2), the above equation can be transformed into
The above equation can be simplified to
(10)
(10)
Furthermore, from the equilibrium equation we can obtain
Using equation (2) to replace the parameters of the above equation, we can obtain
and
Similar to the proof process of inequality (5), we get
taking the norm on the right-hand side of equation (10), we get
(11)
(11)
Since we assume , there is
, and the left-hand side of equation (10) satisfies
Therefore, taking the norm on the left-hand side of equation (10), we get
(12)
(12)
At this point we can see that the inequalities (11) and (12) are contradictory to the equation (10), so the initial assumption about is invalid, that is, all the eigenvalues of the characteristic equation
have negative real parts. That is, when
, the system (1) is locally asymptotically stable at the endemic equilibrium
.
3. Numerical fitting
3.1 Selection of fitting objects
The SARS-CoV-2 has mutated several times since it was first discovered in late 2019. Among them, there are 7 variants of concern that caused a global pandemic, including 614D, 614G, Alpha, Beta, Gamma, Delta and Omicron variants [Citation16]. 614D is an original variant and there is a lack of data on its infectors; although the Beta variant has strong immune evasion ability and its transmission is stronger than that of the Alpha variant, its epidemic process is quickly overtaken by the more transmissible variant; similarly, the Gamma variant, although more pathogenic, was rapidly replaced by the Delta variant in its epidemic process due to its weaker transmission; therefore, we do not consider the above three variants. This study focuses only on four of the major virus variants that are widely circulating worldwide, namely 614G, Alpha, Delta and Omicron variants.
For the 614G variant, we select the epidemic data from 1 June 2020 to 31 July 2020 in England for simulation, which was recorded as the first time period. Since then, the spread of COVID-19 in England has slowed until early November 2020, when a second nationwide lockdown began and lasted for about a month. The second national lockdown did play a relatively obvious role in curbing the spread of the epidemic, but due to the dual effects of the gradual relaxation of the lockdown policy and the emergence of the Alpha variant, England has experienced the third large-scale outbreak in a short period of time [Citation17]. Therefore, for the Alpha variant, we select the epidemic data from 1 December 2020 to 31 December 2020 in England for simulation, which was recorded as the second time period.
Since then, England has entered the third national lockdown and lasted for about two months, which has brought the epidemic in England under certain control. However, similarly, England began its third large-scale outbreak in May 2021 due to the dual effects of the gradual easing of the third lockdown policy and the emergence of the Delta variant. Since the Delta variant first appeared in England in mid-April 2021, it has replaced the Alpha variant as the predominant variant in England in just over a month. Therefore, for the Delta variant, we select the epidemic data from 1 June 2021 to 31 June 2021 in England for simulation, which is recorded as the third time period. Since then, the UK has been adopting a lax epidemic prevention policy, which has kept the daily new case data at a high position.
For the Omicron variant, we select the epidemic data from 18 March 2022 to 9 April 2022 in Shanghai, China for simulation, which is recorded as the fourth time period [Citation18]. There are two main reasons for choosing the epidemic data in Shanghai during this period as the research object. First, this round of epidemic in Shanghai is basically caused by single Omicron variant, which facilitates our comparative analysis. Secondly, this time period is the early stage of the outbreak in Shanghai, which is more suitable for the mathematical model. The relative time stage of the fitting time period selected relative to this round of epidemics are shown in Figure . The first three fitting time periods we selected are the early stages of three large-scale outbreaks in England, and the fourth time period is the early stages of the Shanghai outbreak in April 2022. We use the established model to fit the epidemic data in these four time periods, and comparatively analyse the role of asymptomatic infections in the spread of the epidemic.
Figure 2. Relative position map of the four data fitting time periods during the corresponding outbreak. a, b and c, The relative position map of the selected first, second and third data fitting time periods during the first three outbreaks of the corresponding epidemic in England. d, The relative position map of the selected fourth data fitting time period during the corresponding outbreak in Shanghai in April 2022.

3.2 Numerical fitting results
For the selected 614G, Alpha, Delta and Omicron variants, based on the established model, we use the least squares method to simulate the epidemic data in different periods. Among them, the epidemic data corresponding to the 614G, Alpha and Delta variants are all from the official website of the British government (https://coronavirus.data.gov.uk/), and the epidemic data corresponding to the Omicron variant are from the Shanghai Municipal Health Commission (https://wsjkw.sh.gov.cn/).
The fitting results of the epidemic data in the four time periods are shown in Figure , in which Figures (a–d) show the data fitting in the first to fourth time periods in turn. From the figure, we can see that the simulation results of the epidemic data in the four time periods are relatively satisfactory, which indicates that our model has good universality. Due to the small value and large fluctuation of the original data, the simulation results of the first time period and the fourth time period are not perfect in some parts, but they are generally acceptable. The related dynamic parameters obtained by fitting are shown in Table .
Figure 3. Simulation results of the daily number of newly confirmed cases of epidemic data in four time periods. a, Simulation results in the first time period, corresponding to the 614G variant. b, Simulation results in the second time period, corresponding to the Alpha variant. c, Simulation results in the third time period, corresponding to the Delta variant. d, Simulation results in the fourth time period, corresponding to the Omicron variant.

Table 1. Fitting results of dynamic parameters in model (1).
In addition, we performed a sensitivity analysis of the system dynamic parameters. Sensitivity analysis can be used to obtain the effect of parameters on the system and help to evaluate control measures. The sensitivity of the control reproduction number with respect to any parameter
is calculated as
Using the first set of parameter values obtained by data fitting, the sensitivity of the control reproduction number with respect to the corresponding parameters is obtained, as shown in Figure . From the figure, we can see that there are four parameters with elasticity values greater than 0, which indicates that the spread of the epidemic will expand when these parameters increase, and the spread of the epidemic will shrink when the remaining parameters with elasticity values less than 0 increase. We can also see the impact of control measures on the epidemic. Taking parameters with elastic values less than 0 as an example, such as the proportion of testing among the symptomatic infection (), the recovery rate of undetected symptomatic infections (
), and the recovery rate of asymptomatic infections (
), as long as the values of these three parameters are increased by control measures, the spread of the epidemic can be effectively delayed.
3.3 Horizontal comparison of fitting results
From the perspective of mathematical model simulation, we verify the characteristics of enhanced infectivity and weakened symptoms of infected persons of the four SARS-CoV-2 variants concerned in this study, as shown in Figure . We count the proportions of the fitting results of the three types of infected persons. From the circular distribution map of the types of infected people, we can see that the proportion of asymptomatic patients in the four time periods was 38.05%, 52.89%, 54.02% and 62.03% respectively, showing a clear upward trend. This result shows that the overall symptom level of the infected person is decreasing, which is consistent with the clinical statistics of the decrease in the severe rate of the infected person.
Figure 5. Horizontal comparison results of epidemic data simulation in four time periods. a, b, d and e, Changes in the proportion of the three types of infected persons. c, Model prediction results of the peak time of the epidemic in four time periods. f, Model predictions of maximum daily confirmed cases over four time periods.

In addition, we also compare the propagation speed and propagation strength of different variants. From the perspective of the model, we predict the subsequent development of the epidemic in four time periods. From Figures (c and f), we can see that the peak time of the epidemic development process caused by the variants of interest in this study gradually shortens, and the peak value of daily confirmed cases gradually increases, and there is even an order of magnitude difference. This indicates that the speed of transmission, as well as the intensity of transmission, of the four COVID-19 variants of interest in this study has increased. In addition, it can also be seen from the dynamic parameters obtained by fitting that the basic propagation rate has increased significantly.
3.4 The impact of the rate of participation in testing among symptomatic infected persons on the spread of the epidemic
Although the rate of testing among those with symptomatic infection is fixed in the model simulation, in practice, with the increase in the number of newly diagnosed patients every day, certain prevention and control measures will inevitably be taken to curb the spread of the epidemic. The most direct and effective prevention and control measure is to increase the detection intensity, which is reflected in the model as the increase of the parameter . Therefore, we explore the effect of the change of parameter
on the spread of the epidemic.
Based on the simulation results over four time periods, while keeping other parameters unchanged, re-prediction is carried out for the cases of = 20%, 40%, 60%, and 80%, respectively, as shown in Figures and . From the results, we can see that with the increase of
, the cumulative number of infected people has dropped significantly. In addition, the proportion of asymptomatic infections has declined to a certain extent, but the decline is not significant. As the proportion of people tested among symptomatic infections increases, the impact of symptomatic infection on the spread of the epidemic gradually decreases. Since the model assumes that asymptomatic infected people will not participate in the detection, although the transmission intensity is lower than that of symptomatic infected people, it will still cause a certain amount of transmission. Therefore, even if the proportion of symptomatic infected people participating in the test reaches 80%, there are still a certain proportion of asymptomatic infections.
Figure 6. The impact of the tested ratio ρ among symptomatic infected persons on the spread of the epidemic. a, The influence of parameter ρ changes on the cumulative amount of infected people, based on the fitting results of the first time period. b, The impact of parameter ρ changes on the proportion of different types of infected people, based on the fitting results of the first time period. c, The influence of parameter ρ changes on the cumulative amount of infected people, based on the fitting results of the second time period. d, The impact of parameter ρ changes on the proportion of different types of infected people, based on the fitting results of the second time period.

Figure 7. The impact of the tested ratio ρ among symptomatic infected persons on the spread of the epidemic. a, The influence of parameter ρ changes on the cumulative amount of infected people, based on the fitting results of the third time period. b, The impact of parameter ρ changes on the proportion of different types of infected people, based on the fitting results of the third time period. c, The influence of parameter ρ changes on the cumulative amount of infected people, based on the fitting results of the fourth time period. d, The impact of parameter ρ changes on the proportion of different types of infected people, based on the fitting results of the fourth time period.

In addition, we compare the differences between the different variants when the rate of detection in symptomatic infected individuals changed. As can be seen from Figure , when the detection ratio of symptomatic infections increases from 20% to 80%, the decline ratio of the total number of infected people decreases slowly with the variation of variants. However, the decline rates remain above 70%, indicating that increased testing efforts can still effectively reduce the total number of infected people. In addition, the proportion of asymptomatic infections decrease significantly with the change of variants. From Figure , we can see that for the Omicron variant, when the detection ratio increased from 20% to 80%, the proportion of asymptomatic infections drop by only about 10%. The specific data are shown in Table , where represents the percentage of decline in the total number of infected people, and
represents the percentage of decline in the number of asymptomatic infections. From the results of this part, we can see that in the process of epidemic prevention, only improving the detection of symptomatic infections cannot completely block the spread of the epidemic. It is necessary to cooperate with certain social isolation policies to effectively block hidden transmission caused by asymptomatic infections.
Figure 8. The impact of the tested ratio ρ among symptomatic infected persons on the spread of the epidemic. a, The reduction rate in the total number of infected people when the detection rate goes from 20% to 80%. b, The reduction rate in asymptomatic infections when testing rates go from 20% to 80%.

Table 2. The impact of parameter ρ changes on the spread of the epidemic in different time periods.
3.5 Model-based prediction results
We use the established model to predict the spread of the epidemic. Since the English government no longer publishes epidemic data from May 2022, we choose WHO Coronavirus (COVID-19) Dashboard (https://covid19.who.int/) as the source of epidemic data to fit and predict the development of the epidemic in the UK. As shown in Figure , we forecast the development of the epidemic in the UK from late 2022 to early 2023. Predictions suggest a larger outbreak in the UK in the winter of 2022, which would last for around four months. In order to avoid the collapse of the healthcare system and the negative impact on productive life, the UK should take the necessary measures to hinder the spread of the epidemic.
4. Conclusion
In this study, a SEIAR infectious disease dynamic model was established. We first analyse the local stability at the equilibrium point of the model system. As a result, when , the disease-free equilibrium
of the system is locally asymptotically stable; when
, the disease-free equilibrium
of the system is unstable and the endemic equilibrium
is locally asymptotically stable. In addition, we use the method of constructing the Lyapunov function to prove that the disease-free equilibrium
of the system is globally asymptotically stable when
. In addition, we use the model of the architecture to numerically fit the COVID-19 epidemic data. We select corresponding data for the four main variants, 614G, Alpha, Delta and Omicron, and performed fitting analysis. The comparative analysis of the fitting results shows that the proportion of asymptomatic infections among infected persons is gradually increasing, and the propagation speed and propagation strength of the variant are increased to a certain extent. Finally, we analyse the impact of the detection ratio of symptomatic infections on the spread of the epidemic. With the increase of the detection ratio, the cumulative cases drop significantly, but asymptomatic infections still maintain a certain proportion.
5. Discussion
In this paper, the epidemic data of the main epidemic variants of the novel coronavirus are integrated, and the proportion of asymptomatic infected people during the epidemic of different variants is analysed by mathematical model method, and a horizontal comparative analysis is carried out [Citation27].
Since asymptomatic infections are difficult to be actively detected, the transmission caused by asymptomatic infections is difficult to be interrupted under relatively loose epidemic prevention policies. Especially after the end of the last round of the epidemic, the epidemic prevention policy tends to be gradually loosened as the number of daily confirmed cases declines, which will cause the number of asymptomatic infections to accumulate again. It can also be seen from the comparison results of this study that in general, the pathogenicity of the SARS-CoV-2 variants is decreasing but the infectivity is increasing, which means that the role of asymptomatic infected persons in the spread of the epidemic has increased significantly. And due to increased infectivity, the absolute number of symptomatic infections will not necessarily decrease. This shows that epidemic prevention and control measures are still very important, otherwise there will be continuous challenges to the medical carrying capacity.
Large-scale social activities will also cause large-scale spread of the epidemic in the short term. Taking the Tokyo Olympics as an example, during the entire event period, not only the cities where the competition were held, but also other cities that did not hold the competition experienced outbreaks [Citation28]. During the Tokyo Olympics, Japan's epidemic prevention policy was relaxed, and social mobility increased. This made a large number of asymptomatic infections accumulated in the crowd, resulting in a full-scale outbreak of the epidemic. Therefore, when holding large-scale social activities, it is more necessary to strengthen the control of social mobility to reduce the probability of large-scale outbreaks in the short term.
In view of the increasing role of asymptomatic infections in the spread of the epidemic, non-pharmaceutical intervention (NPI) may play a more important role [Citation29]. Common NPI measures include wearing masks, maintaining social distance, etc. Compared with symptomatic infected individuals, asymptomatic infected individuals have lower viral load and weaker transmission ability, which enables NPI to better block the transmission caused by asymptomatic infected individuals. In addition, vaccination policies still need to be actively implemented, as vaccination is the most effective way to reduce the risk of transmission overall. Therefore, in the process of epidemic prevention and control, when the detection capacity is limited, NPI and vaccination are important measures and means to avoid the collapse of the medical system.
This paper also has some limitations. The model in this paper is only suitable for numerical fitting at the beginning of the outbreak, and does not consider more factors affecting the epidemic. In addition, reinfection due to decreased antibody levels in recovered people is not included in the model, so the model is only suitable for short-term outbreak data fitting.
Acknowledgments
We thank all the individuals who generously shared their time and materials for this study.
Disclosure statement
No potential conflict of interest was reported by the author(s).
Correction Statement
This article was originally published with errors, which have now been corrected in the online version. Please see Correction (http://dx.doi.org/10.1080/17513758.2023.2259761)
Additional information
Funding
References
- Y. Bai, L. Yao, T. Wei, et al., Presumed asymptomatic carrier transmission of COVID-19. JAMA 323(14) (2020), pp. 1406–1407.
- F. Ye, S. Xu, Z. Rong, et al., Delivery of infection from asymptomatic carriers of COVID-19 in a familial cluster. Int. J. Infect. Dis. 94 (2020), pp. 133–138.
- X. Pan, D. Chen, Y. Xia, et al., Asymptomatic cases in a family cluster with SARS-CoV-2 infection. Lancet Infect. Dis. 20(4) (2020 Apr), pp. 410–411.
- M. Samsami, J. Zebarjadi Bagherpour, B. Nematihonar, et al., COVID-19 pneumonia in asymptomatic trauma patients; report of 8 cases. Arch Acad Emerg Med. 8(1) (2020 Apr 6), pp. e46.
- J.J. Barboza, D. Chambergo-Michilot, M. Velasquez-Sotomayor, et al., Assessment and management of asymptomatic COVID-19 infection: A systematic review. Travel Med. Infect. Dis. 41 (2021 May-Jun), pp. 102058.
- W. He, G.Y. Yi, and Y. Zhu, Estimation of the basic reproduction number, average incubation time, asymptomatic infection rate, and case fatality rate for COVID-19: Meta-analysis and sensitivity analysis. J. Med. Virol. 92(11) (2020 Nov), pp. 2543–2550.
- C. Yi, W. Aihong, and Y. Bo, Epidemiological characteristics of infection in COVID-19 close contacts in ningbo city. Chinese J Epid 41(05) (2020), pp. 667–671. in Chinese.
- X. Huo, J. Chen, and S. Ruan, Estimating asymptomatic, undetected and total cases for the COVID-19 outbreak in Wuhan: A mathematical modeling study. BMC Infect. Dis. 21(1) (2021), pp. 476.
- R. Subramanian, Q. He, and M. Pascual, Quantifying asymptomatic infection and transmission of COVID-19 in New York city using observed cases, serology, and testing capacity. Proc. Natl. Acad. Sci. U.S.A. 118(9) (2021), pp. e2019716118.
- M. Amouch, and N. Karim, Modeling the dynamic of COVID-19 with different types of transmissions. Chaos, Solitons Fractals 150 (2021), pp. 111188.
- L. Ying-Ke, Z. Shi, L. Yi-Jun, et al., Epidemiological parameters and models of coronavirus disease 2019. Acta Phys. Sin. 69(9) (2020), pp. 0090202.
- Y. Ge, L. Martinez, S. Sun, et al., COVID-19 transmission dynamics among close contacts of index patients With COVID-19. JAMA. Intern. Med. 181(10) (2021), pp. 1343–1350.
- A. Alshorman, X. Wang, M.J. Meyer, et al., Analysis of HIV models with two time delays. J. Biol. Dyn. 11(sup1) (2017), pp. 40–64.
- S. Guo, Y. Xue, X. Li, et al. Global dynamics of a COVID-19 model with asymptomatic infections and quarantine measures. (2022). doi: 10.48550/arXiv.2209.05240.
- Y. Bai, X. Wang, and S. Guo, Global stability of a mumps transmission model with quarantine measure. Acta Mathematicae Applicatae Sinica, English Series 37 (2021), pp. 665–672.
- K. Koelle, M.A. Martin, R. Antia, et al., The changing epidemiology of SARS-CoV-2. Science 375(6585) (2022), pp. 1116–1121.
- H.S. Vöhringer, T. Sanderson, M. Sinnott, et al., Genomic reconstruction of the SARS-CoV-2 epidemic in England. Nature 600(7889) (2021), pp. 506–511.
- J. Cai, X. Deng, J. Yang, et al., Modeling transmission of SARS-CoV-2 Omicron in China. Nat. Med. 28 (2022), pp. 1468–1475.
- Office for National Statistics. Births, deaths and marriages: Life events in the UK including fertility rates, live and stillbirths, family composition, life expectancy and deaths [EB/OL]. [2022-07-22]. https://www.ons.gov.uk/peoplepopulationandcommunity/birthsdeathsandmarriages.
- Shanghai Municipal Bureau of Statistics. 2021 Shanghai Statistical Yearbook [EB/OL]. [2022-07-22]. http://tjj.sh.gov.cn/tjnj/20220309/0e01088a76754b448de6d608c42dad0f.html. (in Chinese).
- Z.C. Du, J. Gu, J.H. Li, et al., Estimating the distribution of COVID-19 incubation period by interval-censored data estimation method. Zhonghua Liu Xing Bing Xue Za Zhi 41(7) (2020 Jul 10), pp. 1000–1003. in Chinese.
- Comprehensive team of the joint prevention and control mechanism of the state council of the People's Republic of China in response to the novel coronavirus pneumonia outbreak. Notice on Issuing the New Coronavirus Pneumonia Prevention and Control Plan (Eighth Edition) [EB/OL]. [2022-05-11]. http://www.gov.cn/xinwen/2021-05/14/content_5606469.htm. (in Chinese).
- L. Shirong, Y. Gang, H. Fen, et al., Clinical characteristics of COVID-19 and analysis of the outcomes in 31 cases. J Wannan Med College 40(01) (2021), pp. 61–64. (in Chinese).
- Z. Yu, L. Yang, Y. Renxin, et al., Length of hospital stay of 365 patients with novel coronavirus pneumonia (COVID-19) based on a multilevel model analysis. Mod Prev Med 47(22) (2020), pp. 4210–4213 + 4224. in Chinese.
- A. Guo, J. Lu, H. Tan, et al., Risk factors on admission associated with hospital length of stay in patients with COVID-19: A retrospective cohort study. Sci. Rep. 11(1) (2021), pp. 7310.
- X. Rong, L. Yang, H. Chu, et al., Effect of delay in diagnosis on transmission of COVID-19. Math. Biosci. Eng. 17(3) (2020), pp. 2725–2740.
- Z. Gao, Y. Xu, C. Sun, et al., A systematic review of asymptomatic infections with COVID-19. J Microbiol, Immunol Inf 54(1) (2021), pp. 12–16.
- T. Yashio, A. Murayama, M. Kami, et al., COVID-19 infection during the Olympic and paralympic games Tokyo 2020. Travel Med Infect Dis. 44 (2021 Nov-Dec), pp. 102205.
- R.B. Bestetti, R. Furlan-Daniel, and L.B. Couto, Nonpharmaceutical public health interventions to curb the COVID-19 pandemic: A narrative review. J Inf Devel Countries 16(4) (2022), pp. 583–591.
Appendices
Appendix A
Jacobian matrix of system (1) at the disease-free equilibrium
.
Appendix B
The Jacobian matrix of system (1) at the endemic equilibrium
.