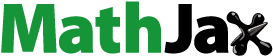
Abstract
We consider a Darwinian (evolutionary game theoretic) version of a standard susceptible-infectious SI model in which the resistance of the disease causing pathogen to a treatment that prevents death to infected individuals is subject to evolutionary adaptation. We determine the existence and stability of all equilibria, both disease-free and endemic, and use the results to determine conditions under which the treatment will succeed or fail. Of particular interest are conditions under which a successful treatment in the absence of resistance adaptation (i.e. one that leads to a stable disease-free equilibrium) will succeed or fail when pathogen resistance is adaptive. These conditions are determined by the relative breadths of treatment effectiveness and infection transmission rate distributions as functions of pathogen resistance.
1. Introduction
In the twenty-first century , the world had seen a large reduction in the incidence of communicable diseases and their associated mortality, primarily due to improved access to and development of novel treatments and preventive vaccines, as well as improving coverage of interventions targeting disease vectors [Citation20]. However, the evolution of resistance to treatment in pathogens and vectors remains a major hurdle to disease control and elimination (see [Citation19] and the World Health Organization (WHO) Global Malaria Programme at https://www.who.int/teams/global-malaria-programme/prevention/vector-control/insecticide-resistance. Increasing antimicrobial resistance has led to reduced efficacy of treatments, resulting in a higher disease burden and higher mortality from infectious diseases. Similarly, increasing insecticide resistance in disease vectors, especially mosquitoes, has reduced the effectiveness of preventive interventions such as insecticide-treated nets, resulting in higher incidences of vector-borne diseases and associated mortality.
There is a substantial body of literature in modelling resistance; however, most studies have focused on the transmission of resistant pathogens or vectors, not the evolution of resistance; subsequently they consider either the impact of interventions on the transmission of resistance or on the effect of resistance on intervention effectiveness [Citation5,Citation15]. The studies that have modelled the evolution of resistance have focused on discrete genotypes that only consider a limited number of phenotypes [Citation3,Citation14–16]. Our main goal here is to investigate the methodology of Darwinian dynamics (evolutionary game theory) [Citation18] as a tool to study the effectiveness of treatment in the presence of a pathogen's ability to develop resistance through evolutionary principles. Unlike most applications of adaptive dynamics [Citation6], we do not focus here on a limited number of pathogen mutants or strains or genotypes, utilize methods of optimizing nor assume a separation of ecological and evolutionary time scales [Citation7–9]. Instead we consider a pathogen with a continuously distributed phenotypic trait that confers resistance to a treatment and consider a model in which the host dynamics and the evolutionary dynamics of the mean trait are coupled. The model is based on the (so-called first order) methodology of evolutionary game theory in which the mean trait dynamics are governed by Lande's equation (sometimes referred to as Fisher's equation or the canonical equation of evolution) [Citation18]. In this paper we focus only on the existence and stability properties of disease-free and endemic equilibria and how they depend on the way treatment resistance and pathogen infectiousness depend on the evolving mean trait. To do this we extend a basic compartmental susceptible-infectious-recovered model to better understand the evolution of resistance to treatment and the impact of this evolution on treatment effectiveness. We assume in the model that
there is a constant recruitment/birth rate into the population;
the force of infection follows a mass action law;
infection leads to an additional disease-induced death rate; and
recovery from infection leads to lifelong immunity.
Since the recovered population does not influence the dynamics of the susceptible or infectious populations, we do not explicitly model the recovered class, and henceforth refer to the model as an SI model.
We consider a treatment that is fully effective in preventing disease-induced mortality and that further reduces the duration of the infectious period, in the absence of resistance. The evolution of resistance increases the fitness of the pathogen by reducing the effectiveness of the treatment in clearing the infection and thereby increasing the duration of infection. However, it also reduces the fitness of the pathogen since reducing treatment effectiveness leads to an increase in host death due to increased disease-induced mortality (and consequently leads to the loss of the pathogen). We furthermore assume that resistance has a cost to the pathogen in onward transmissibility from one host to another, leading to reduced infectivity of individuals infected with resistant pathogens.
We focus on a situation where the disease causing pathogen is controlled (asymptotically eliminated) by a certain level of a treatment in the absence of the pathogen's ability to evolve resistance to the treatment. Using the methodology of evolutionary game theory [Citation18], we derive a Darwinian SI model and using it to address the following questions. Under what conditions will this treatment level still work in eliminating the pathogen if it is capable of evolving resistance to the treatment? If this treatment level fails due to the pathogen's adaptation, will a higher treatment level succeed? Are there conditions under which no level of treatment will successfully eliminate an evolutionarily adapting pathogen?
We first describe the SI model with treatment in the absence of pathogen resistance, including the definition of the basic reproductive number, and the existence and stability of equilibrium solutions. We then derive a Darwinian version of this model that describes the evolution of the fitness of the pathogen in response to treatment. We analytically investigate the existence and stability of disease-free and endemic equilibria. We discuss the criteria for the existence and stability in terms of two important bifurcation parameters: the proportion of people treated and the ratio of the variance of treatment effectiveness to the variance of the infection transmission rate. We illustrate these results with time series plots, phase portrait plots and bifurcation diagrams. We summarize these results with a parameter map showing the criteria for the existence and stability of the equilibria.
The basic SI model we use in this study is described, as is commonly done, by ordinary differential equations. The method of Darwinian dynamics which we apply to the model is also available for discrete time (difference equation) models, which are also often used for modelling epidemics (for example, by A.-A. Yakubu, to whom this paper is dedicated).
2. An SI model with resistance to treatment
A basic disease model with two classes of individuals, susceptible S and infectious I, is [Citation4] (1)
(1) Here
is the constant rate at which susceptible individuals enter the population and
is the (per capita) natural death rate. The coefficient c>0 measures the transmission rate at which infectious individuals infect susceptible individuals (assumed to follow a mass action law) and cause them to enter the infectious class. Finally
is the (per capita) rate at which infectious individuals are removed from the population and, because complete immunity is assumed, do not re-enter the susceptible class. There exist two equilibria, a disease-free equilibrium
and, provided the basic reproduction number
satisfies
, an endemic equilibrium
A routine linearization analysis shows DFE is stable if
and is unstable if
when EE is stable. Thus, a standard transcritical bifurcation and exchange of stability occurs between these equilibria at
. In fact, it is shown in [Citation2] using Liapunov functions that DFE is globally asymptotically stable on
when
and EE is globally asymptotically stable on
when
.
We parse the infectious removal rate ρ into the sum of three rates: the natural death rate (which we assume equal to , the natural death rate of susceptible individuals), the death rate due to the disease
, and removal rate due to recovery from the infection
. We are interested in the effect of a treatment for the disease, which we assume is successfully administered to a fraction τ of the infectious class,
. This treatment introduces an additional recovery rate
to the
individuals treated and prevents death due to the disease. Adding together all the removals from the infectious class (see Table ), we get
which gives us the model equations
Table 1. Removal rates from the infectious class.
We consider the reproduction number as a function of the treatment fraction τ :
We are interested here in the scenario when the disease is endemic in the absence of the treatment, but is eliminated if the treatment reaches a sufficient fraction of the infectious class. To this end, we assume
inequalities which we can re-write as
(2)
(2) Note that these constraints imply
namely that the additional recovery rate provided by the treatment exceeds the death rate due to the disease. The treatment is successful in preventing an epidemic if
, i.e. if
(3)
(3) and fails if
.
3. A Darwinian SI model
To derive the fitness of the pathogen for the Darwinian SI model, we re-interpret I as the number of infections in the population, where each individual can have exactly zero or one infection. The individuals with no infection are susceptible, while the individuals with one infection are infectious. The derivation of the model in Section 2 remains exactly the same with this new interpretation, but c now needs to have the appropriate units to convert between individuals and infections. The number of infections increases when susceptible individuals get infected and decreases when infected individuals recover or die (because the death of the host also implies the death of the infection).
We suppose that the disease is caused by a pathogen that can develop resistance to the treatment by Darwinian processes. We hypothesize that resistance is conferred by a (continuous) phenotypic trait v that is subject to natural selection and that is normally distributed throughout the population at all time, with variance and mean u. Let
denote the probability distribution for v so that there are
infections with trait v when the mean population trait is u. The fraction of these infections that are successfully treated depends on the resistance of the pathogen and
is a function v.
We assume there is a trait v at which the pathogen has no resistance to the treatment. We denote this reference point by v=0 and assume that the treatment is a decreasing function of increasing pathogen resistance
In the model considered here, it is assumed that increased resistance to the treatment comes at a cost to the pathogen, namely at the cost of decreased infectiousness. Thus, the infection transmission rate c in model (Equation1(1)
(1) ) becomes a decreasing function
of
.
Consider the sub-class of infections with resistance v. The fraction of these infections successfully treated is
The removal rates from this sub-class are shown in Table . The total removal rate is the sum of the entries in Table , which equals
The removal rate from the susceptible class due to infections with trait v and the entry rate into this infectious sub-class is
. Thus, the change in this infectious class is
where
(4)
(4) We take this per capita rate as fitness of the pathogen with resistance v.
Table 2. Removal rates of infections with trait v.
The methodology of Darwinian dynamics provides a model for the dynamics of S, I, and the mean trait u [Citation1,Citation10,Citation11,Citation13,Citation18] :
where
is called the speed of evolution. Since
and
the expression (Equation4
(4)
(4) ) yields the model equations
(The primes denote differentiation with respect to a function's argument.)
In this paper, we assume both the treatment effectiveness and the infection transmission rates have Gaussian distributions as functions of the trait v. Thus, we replace and
, respectively, by
(5)
(5) where τ and c now denote positive constants that denote the treatment effectiveness and infection transmission rates for an pathogen with no resistance to the treatment (v=0). The coefficient
is the variance of the treatment effectiveness relative to the variance of the infection transmission rate (which we assume, by re-scaling the units for v if necessary, to be equal to 1). The resulting Darwinian SI model equations are
(6)
(6) The two parameters τ and
that define the distributions appearing in (Equation5
(5)
(5) ) play a central role in our analysis and findings below. τ is the maximal effectiveness of the treatment (which occurs for those infections with trait v=0, i.e. with no resistance to the treatment). The variance
measures how widely spread the treatment is effective; a larger value of
indicates that a larger distribution of infections are successfully treated.
Since the equations for I and u in (Equation6(6)
(6) ) have factors I and u on their right sides and because
(so that
when
we obtain the following lemma.
Lemma 3.1
For
the solution of (Equation6
(6)
(6) ) satisfies
for all
. Moreover for all t>0
(a) |
| ||||
(b) |
| ||||
(c) |
|
As a result of this lemma, we restrict our analysis of the dynamics of (Equation6(6)
(6) ) (with a focus on the existence and stability of equilibria) to
. In particular, we consider only solutions with non-negative trait components
. Note that a change in variables from u to
leaves the model equations (Equation6
(6)
(6) ) unchanged and results for
yield symmetric results for
.
From the right sides of (Equation6(6)
(6) ), with u fixed, we calculate the reproduction number
4. Equilibrium analysis of the Darwinian SI model
The equilibrium equations of (Equation6(6)
(6) ) are
(7a)
(7a)
(7b)
(7b)
(7c)
(7c) In the next section we see that these equations are analytically tractable and that there exist two types of disease-free and two types of endemic equilibria.
4.1. Existence
A disease-free equilibrium
is a solution of the equilibrium equations (Equation7
(7a)
(7a) ) with
and
and
that satisfy the equations
(8)
(8)
(9)
(9) Equation (Equation8
(8)
(8) ) implies
and equation (Equation9
(9)
(9) ) implies that either
or
is a root of the equation
(10)
(10) This results in two disease-free equilibria in
the non-adaptive disease-free equilibrium
and the equilibrium
with a positive equilibrium trait component
in either of two cases depending on the constraints
for the two distributional characteristics
and τ of the treatment effectiveness (Equation5
(5)
(5) ). Note that
when
where these two disease-free equilibria mathematically undergo a pitchfork bifurcation (taking into consideration the additional
with
replaced by
).
To find endemic equilibria we need to solve the equilibrium equations (Equation7a(7a)
(7a) ) for
and the equilibrium equations become (after a cancellation of I)
(11)
(11) The third equation implies either
or
is a root of the parenthetical factor on the right side of the equation.
In the first case we can solve the second equation for S after which we can solve the first equation for I. The result is the non-adaptive endemic equilibrium
in
provided
i.e. provided
In the second case the equilibrium equations reduce (after a cancellation of u) to the system of equations
By adding the last two equations we get an equation for u alone (with S and I absent) which we can solve for
(12)
(12) after which we can solve the third and first equations (in that order) for
(13)
(13) and
(14)
(14) to obtain the adaptive endemic equilibrium
provided this vector lies in
While it is clear that
it takes some manipulations with inequalities to show that
and
lies in
if and only if τ lies in the interval
Fixing all other parameters, we can view the various constraints required for the existence of the two disease-free equilibria
and the two endemic equilibria
and
in terms of the two parameters
and τ characterizing the effectiveness of the treatment as a function of pathogen resistance, as given by the assumed distribution (Equation5
(5)
(5) ). The relevant region in the
-plane, namely the infinite rectangle
and
, is divided in to a number of sub-regions in each of which there exist a specific set of equilibria. These regions are shown in the parameter maps in Figure , which also include information about the (local asymptotic) stability of the equilibria, a topic we take up in the next section.
4.2. Stability
The Jacobian associated with the equations (Equation6(6)
(6) ) is
(15)
(15) where
To apply the Linearization Principle to the disease-free equilibrium
we evaluate the Jacobian (Equation15
(15)
(15) ) at
and obtain
whose eigenvalues are the diagonal entries
Recalling that the assumptions (Equation2
(2)
(2) ) imply
we explore the signs of
and
and obtain the following result.
Theorem 4.1
The disease-free equilibrium is stable if
If either inequality is reversed,
is unstable.
To apply the Linearization Principle to the disease-free equilibrium we calculate the Jacobian (Equation15
(15)
(15) ) at
and obtain
where
The eigenvalues of this matrix are the diagonal entries:
Since
satisfies Equation (Equation10
(10)
(10) ) and hence the equation
we obtain
If
then
and
is unstable. If
then stability by linearization is determined by the sign of
which we treat as a function of τ and obtain the following result.
Theorem 4.2
The disease-free equilibrium is stable if
and unstable if either inequality is reverse.
To apply the Linearization Principle to the disease-free equilibrium (which, as we saw above, is positive if and only if
) we calculate the Jacobian (Equation15
(15)
(15) ) at
and obtain
which is block diagonal. The eigenvalues are the two eigenvalues
and
of the
submatrix
and the diagonal term
Once again recall that (Equation2
(2)
(2) ) imply
The trace-determinant criteria sufficient to guarantee that the eigenvalues of a
matrix lie in the left half complex plane are that the trace be negative, which it clearly is, and that the determinant
be positive, which it is since
. Thus, the stability by linearization of
is determined by the sign of
This leads to the the following result.
Theorem 4.3
The endemic equilibrium is stable if
or if
It is unstable if
Finally we consider the endemic equilibrium In the second equilibrium equation (Equation11
(11)
(11) ), we see that
and hence
. Using this fact, together with
which follows from the first equilibrium equation (Equation11
(11)
(11) ), we find that the Jacobian at
is
(16)
(16) As shown by the parameter maps in Figure , the endemic equilibrium
exists only in restricted regions of the
-plane that require, among other constraints, that
be not too large, namely, that
. Although we do not have a general analysis of this Jacobian for all parameter values for which
exists, we do have the following result for small
Theorem 4.4
For sufficiently small, the endemic equilibrium
is locally asymptotically stable.
Proof.
From
and
we get that
From the formulas (Equation12
(12)
(12) ) and (Equation13
(13)
(13) ) we have, after some algebraic manipulations, that
and since
we find that
Finally from these two limits and the formula (Equation14
(14)
(14) ) we get
Thus,
(17)
(17) The first column, third row entry in the Jacobian (Equation16
(16)
(16) ) tends to 0 as
. Therefore, its three eigenvalues are, for small
, approximately equal to the two eigenvalues
and
of the upper left
matrix
(18)
(18) and the real eigenvalue
.
If we re-write
and observe from (Equation13
(13)
(13) ) and (Equation14
(14)
(14) ) that
we find that
As
we see, by using (Equation17
(17)
(17) ), that the matrix (Equation18
(18)
(18) ) approaches the matrix
Since the trace of this matrix is negative and its determinant is positive, it follows that its two eigenvalues lie in the left half complex plane. Thus,
and
lie in the left half complex plane for
small.
4.3. Summary of equilibrium analysis
The existence and stability results for the equilibria of the Darwinian SI model (Equation6(6)
(6) ) that we obtained in the preceding sections are geometrically summarized by the two maps in the
-plane shown in Figure . The relevant infinite rectangle
in the
-plane is partitioned into regions that result from the constraints determining the existence and stability of the four types of equilibria. These regions are determined by the following curves and lines:
the vertical straight line at
the horizontal line at
the straight line L given by the equation
where
the curves given respectively by the equations
and
that describe τ as a function of
.
The horizontal line at , the line L, and the curves
and
all intersect at the point
where
The line L and the curve
intersect the horizontal line at
at
and
, respectively, where
is the solution of the equation
Within each region appearing in the rectangle R there exists a certain number of equilibria, as indicated by the tables in Figure . In each region there is exactly one stable equilibrium, with the exception of region IV. For parameter points
lying in region IV, we show in the Appendix that the asymptotic dynamics are disease-free in the sense that
, even though orbits do not equilibrate. As indicated in Figure the stability of
in regions A, B, and C is qualified in that it has been rigorously established only for
small and is conjectured to be true throughout these regions.
As one moves around on the parameter maps in Figure , one passes through various regions and by so doing loses or gains equilibria and/or equilibrium stability. These occurrences result from equilibrium bifurcations which can be displayed in bifurcation diagrams. For example, fixing the variance and varying τ from 0 to 1 one follows a vertical path on the left side of the parameter map in Figure , passing through regions D, C, B and A, along which the equilibrium counts change from 2 to 3 to 4 to 3. A sample bifurcation diagram for such a path is shown in Figure along with some other sample bifurcation diagrams along other vertical paths.
Figure 2. Four sample bifurcation diagrams showing the equilibrium components I and u plotted against the bifurcation parameter τ with model parameter values
and
The fours diagrams correspond to vertical paths in the parameter map in Figure (A) located at the displayed value of
. For the upper left diagram
and for the upper right diagram,
is between
and
in Figure For the lower left diagram
is between
and 1 and for the lower right diagram
in Figure .

5. Concluding remarks
As shown in Section 2, under the conditions (Equation2(2)
(2) ) on the parameters in the non-evolutionary SI model (Equation1
(1)
(1) ), any treatment level
will eliminate the disease pathogen (in the sense that the disease-free equilibrium is stable), but the treatment will fail (in the sense that there is a stable endemic equilibrium) if
. We can use the results of the equilibrium analysis of the Darwinian SI model (Equation6
(6)
(6) ), summarized in Figure , to determine the success or failure of the treatment when the disease pathogen can develop resistance to the treatment by means of evolutionary adaptation.
Note that the shaded regions in both maps correspond to disease-free asymptotic dynamics while the unshaded regions correspond to endemic asymptotic dynamics. This implies that the treatment is successful if and only if τ and are appropriately large; otherwise the disease becomes endemic.
Recall that τ in the Darwinian SI model (Equation6(6)
(6) ) is the treatment effectiveness against the disease pathogens who have no resistance to the treatment (i.e. trait v=0) and that the threshold treatment level
is defined by (Equation3
(3)
(3) ). It is therefore no surprise that a treatment
will also fail to eliminate the pathogen in the Darwinian model (Equation6
(6)
(6) ), as the parameter maps in Figure show (in which regions B, C, D and E correspond to stable endemic equilibria). That is to say, if the treatment fails to eradicate a non-evolving pathogen, then it will fail to eradicate an evolving pathogen.
It is also clear from the parameter maps in Figure that a treatment level that eliminates the pathogen in the non-evolutionary model will not necessary eliminate the pathogen in the Darwinian model. In order to successfully defeat an evolving pathogen (i.e. for there to be a stable disease-free equilibrium), not only τ but also the ratio of the variance of the treatment effectiveness to the ratio of the variance of the infection transmission rate,
, must be sufficiently large. More precisely, the pair
must lie in the shaded region shown in the parameter maps. This leads to three distinct possibilities, depending on the variance
of the treatment effectiveness:
implies any treatment level
leads asymptotically to a disease-free state;
implies a treatment level τ leads asymptotically to a disease-free state if and only if it meets a higher threshold (placing the point
above the curve
), namely
implies no treatment level will eradicate the disease causing pathogen and it will become endemic by evolutionary adaptation.
In the case (i), the distribution of treatment effectiveness as a function of pathogen resistance v is so wide that the treatment succeeds despite the evolutionary adaptation of the pathogen. In case (ii) in which the distribution is narrower, the treatment succeeds only if it meets a higher threshold than what it would need in the absence of evolutionary adaptation. Numerically calculated time series illustrating the adjusted threshold in case (ii) are shown in Figure . The orbits associated with those times series are shown in the three dimensional phase space, along with other sample orbits, in Figure . Finally, in case (iii) the distribution of treatment effectiveness is too narrowly constrained around those pathogens with little resistance to be successful in eradicating the disease.
Figure 3. Shown are the time series of and
for the solutions of the Darwinian SI model (Equation6
(6)
(6) ) with parameter values
and initial conditions
when (a)
and (b)
. The corresponding point
is shown as a solid dot in the (numerically calculated) parameter map. In case (a) the point is below the threshold curve
and, as predicted the endemic equilibrium
is stable. In this case the treatment is insufficient to eradicate the adapting disease agent which asymptotically attains a resistance level of approximately u=1. In case (b) the point is above the threshold curve
and as predicted the disease-free equilibrium
is stable. In this case the treatment eradicates the disease, even though it develops a level of resistance. In this example
,
, and
.

Figure 4. The phase portrait orbits of the time series solutions in Figure are displayed in three dimensional phase space, together with a selection of other orbits (with initial conditions denoted by open circles). The graphs (a) and (b) correspond to the graphs (a) and (b) in Figure . In (a) all orbits tend to an equilibrium point indicated by the solid black circle which, because it located in the interior of the positive octant, is an endemic equilibrium. In (b) all orbits tend to an equilibrium point indicated by the solid black circle which, because it located on the vertical coordinate plane I=0, is a disease-free equilibrium.

We also note that a decreased treatment level τ always results in a higher disease prevalence in endemic equilibria. (It is an elementary calculus exercise to show that
for the adaptive endemic equilibria
and
). Therefore, in this model a decreased treatment level τ always results in a higher disease prevalence
.
Although we applied this novel methodology to a relatively simple model to allow for tractability, the derivation of Darwinian dynamics described in Section 3 could in principle be applied to many other compartmental infectious disease models (in continuous or discrete time). The key requirement would be to identify a class that represents the number of infections, from which the fitness of the pathogen can be derived. This methodology could similarly be applied to mosquito population dynamics to model the evolution of insecticide resistance, that can then be combined with models of vector-borne disease dynamics. However, applying Darwinian dynamics to more complicated disease transmission models would likely result in systems of equations where analytical solutions of equilibria and their Jacobians may not be tractable, requiring a greater reliance on numerical simulations. Nevertheless, such models could provide greater understanding of the evolution of resistance in specific pathogens, and the role of the evolution of insecticide resistance in vector-borne disease control.
Disclosure statement
No potential conflict of interest was reported by the author(s).
Additional information
Funding
References
- P.A. Abrams, Modelling the adaptive dynamics of traits involved in inter- and intraspecific interactions: An assessment of three methods, Ecol. Lett. 4 (2001), pp. 166–175.
- P. Adda and D. Bichara, Global stability for SIR and SIRS models with differential mortality, Int. J. Pure. Appl. Math. 80(3) (2012), pp. 425–433.
- S. Barbosa, K. Kay, N. Chitnis, and I.M. Hastings, Modelling the impact of insecticide-based control interventions on the evolution of insecticide resistance and disease transmission, Parasites & Vectors11 (2018), pp. 1–21.
- F. Brauer, C. Castillo-Chavez, and Z. Feng Mathematical Models in Epidemiology, Texts in Applied Mathematics; Vol. 69 Springer, New York, 2019.
- T.S. Churcher, N. Lissenden, J.T Griffin, E. Worrall, and H. Ranson, The impact of pyrethroid resistance on the efficacy and effectiveness of bednets for malaria control in Africa, eLife 5 (2016), pp. e16090.
- F. Dercole and S. Rinaldi, Analysis of Evolutionary Processes: The Adaptive Dynamics Approach and Its Applications, Princeton University Press, New Jersey, 2008.
- U. Diekmann, Adaptive dynamics of pathogen-host interactions, in Adaptive Dynamics of Infectious Diseases: in Pursuit of Virulence Management, Cambridge Studies in Adaptive Dynamics, U. Dieckmann, J.A.J. Metz, M.W. Sabelis, and K. Sigmund, eds., Cambridge University Press, Cambridge, UK, 2002, Vol. 2, pp. 39–59 .
- R. Djidjou-Demasse, S. Alizon, and M.T. Sofonea, Within-host bacterial growth dynamics with both mutation and horizontal gene transer, J. Math. Biol. 82 (2021), pp. 1–30.
- S. Gupta, Pathogen evolution: the case of malaria, in Adaptive Dynamics of Infectious Diseases: in Pursuit of Virulence Management, Cambridge Studies in Adaptive Dynamics, U. Dieckmann, J.A.J. Metz, M.W. Sabelis, and K. Sigmund, eds., Cambridge University Press, Cambridge, UK, 2002, Vol. 2, pp. 347–361.
- R. Lande, Natural selection and random genetic drift in phenotypic evolution, Evolution 30 (1976), pp. 314–334.
- R. Lande, A quantitative genetic theory of life history evolution, Ecology 63 (1982), pp. 607–615.
- L. Markus, Asymptotically autonomous differential systems, in Contributions to the Theory of Nonlinear Oscillations III, Annals of Mathematics Studies, S. Lefschetz, ed., Princeton University Press, 1956, Vol. 36, pp. 17–29.
- B.J. McGill and J.S. Brown, Evolutionary game theory and adaptive dynamics of continuous traits, Annu. Rev. Ecol. Evol. Syst. 38 (2007), pp. 403–435.
- J. Mohammed-Awel and A.B. Gumel, Mathematics of an epidemiology-genetics model for assessing the role of insecticides resistance on malaria transmission dynamics, Math. Biosci. 312 (2019), pp. 33–49.
- A.M. Niewiadomska, B. Jayabalasingham, J.C. Seidman, L. Willem, B. Grenfell, D. Spiro, and C.Viboud, Population-level mathematical modeling of antimicrobial resistance: a systematic review, BMC Medicien 17 (2019), pp. 1–20.
- P. Selvaraj, E.A. Wenger, D. Bridenbecker, N. Windbichler, J.R. Russell, J. Gerardin, C.A.Bever, and M. Nikolov, Vector genetics, insecticide resistance and gene drives: An agent-based modeling approach to evaluate malaria transmission and elimination, PLoS. Comput. Biol. 16(8) (2020), pp. e1008121, https://doi.org/10.1371/journal.pcbi.1008121.
- H.R. Thieme, Asymptotically autonomous differential equations in the plane, Rocky Mountain J. Math. 24(1) (1994), pp. 351–380.
- T.L. Vincent and J.S. Brown, Evolutionary Game Theory, Natural Selection, and Darwinian Dynamics, Cambridge University Press, Cambridge, UK, 2005.
- World Health Organization, Global action plan on antimicrobial resistance, 2015, https://www.who.int/publications/i/item/9789241509763.
- World Health Organization, World health statistics 2021: monitoring health for the SDGs, sustainable development goals, 2021, https://apps.who.int/iris/handle/10665/342703.
Appendix
In the triangle in Figure (A) defined by the inequalities
(A1)
(A1) in the case when
the only equilibrium
is unstable.
Theorem A.1
Assume (EquationA1(A1)
(A1) ) and
. For solutions
of (Equation6
(6)
(6) ) with initial conditions
the following hold:
(a) | |||||
(b) |
| ||||
(c) |
Proof.
From Lemma 3.1 it follows that and
for all t>0.
From the first equation in (Equation6
(6)
(6) ) we see that
By standard comparison theorems
for all
where
is the solution of the initial value problem
Since
the result follows.
By (a), for any
there exists a
such that
From the third equation in (Equation6(6)
(6) )
Since
in (EquationA1
(A1)
(A1) ) we get
If we choose ε so that
(which we can do by (EquationA1
(A1)
(A1) )), then
for all
, i.e.
is eventually monotonically increasing and if we define
then either
or
. If
, then the first two equations of (Equation6
(6)
(6) ) constitute an asymptotically autonomous system
(A2)
(A2) for S and I with limiting system
(Note that right sides of (EquationA2
(A2)
(A2) ) converge uniformly as
for
in any compact set in
) This limiting system is an SI model of the form (Equation1
(1)
(1) ). As pointed out in Section 2 solutions globally equilibrate on
to an equilibrium
[Citation2]. Then we can invoke Theorem A.2 to conclude that
approaches the equilibrium
However, this is a contraction to the fact that on the triangular region (EquationA1
(A1)
(A1) ) the only equilibrium present in the system is
for which the u component equals 0. Thus,
cannot hold and it follows that
.
(c) Since the first two equations for S and I in the Darwinian model (Equation6
(6)
(6) ) are asymptotically autonomous system (EquationA2
(A2)
(A2) ) for
and
with the limiting equation
all of whose solutions equilibria to
Invoking Theorem A.2 below we conclude that that
.
Theorem A.2
(Theorem 1.3 in [Citation17] or Theorem 7 in [Citation12]) The omega limit set of any orbit of the asymptotically autonomous system either contains equilibria of the limiting system or is the union of periodic orbits of the limiting system.