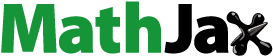
Abstract
Despite the existence of a powerful theoretical foundation for the development of multiscale models of infectious disease dynamics in the form of the replication–transmission relativity theory, the majority of current modelling studies focus more on single-scale modelling. The explicit aim of this study is to change the current predominantly single-scale modelling landscape in the design of planning frameworks for the control, elimination and even eradication of infectious disease systems through the exploitation of multiscale modelling methods based on the application of the replication–transmission relativity theory. We first present a structured roadmap for the development of multiscale models of infectious disease systems. The roadmap is tested on hookworm infection. The testing of the feasibility of the roadmap established a fundamental result which can be generalized to confirm that the complexity of an infectious disease system is encapsulated with a level of organization spanning a microscale and a macroscale.
1. Introduction
Even though there are still many challenges in the development of multiscale models of infectious disease dynamics [Citation1], we distinguish the recent formulation and development of the replication–transmission relativity theory [Citation3] as the most significant contribution to our current understanding of multiscale modelling of infectious disease dynamics. This theory summarized multiscale modelling of infectious disease dynamics in a form that significantly transformed mainstream thinking. It established the theoretical foundation for the development of multiscale models of infectious disease dynamics. We think that much of the hesitancy surrounding the application of the replication–transmission relativity theory in the development of multiscale models of infectious disease dynamics is caused by the uncertainty about how this theory can be applied to develop the multiscale models. In the context of mathematical modelling of infectious disease dynamics, we identify two scientific theories that inform our current understanding of infectious disease dynamics which are: (a) the transmission mechanism theory [Citation2] – which is about single-scale modelling of infectious disease dynamics and (b) the replication–transmission relativity theory [Citation3] – which is about multiscale modelling of infectious disease dynamics. For details about limitations of single-scale models of infectious disease dynamics as opposed to multiscale models, see [Citation2,Citation19]. The overall aim of this study is to change the current predominantly single-scale modelling landscape in the design of planning frameworks for the control, elimination and even eradication of infectious disease systems [Citation2] by stimulating interest from the broader international mathematical biology community to promote further exploitation of multiscale modelling framework based on the application of the replication–transmission relativity theory [Citation3] in understanding infectious disease dynamics. This overall aim is embodied in the following four specific objectives of this article:
clarifying some misunderstandings about the replication–transmission relativity theory as a biological relativity theory for living systems, particularly in the context of its relationship with Einstein's general relativity theory for physical systems [Citation4],
presenting a structured roadmap for the development of multiscale models of infectious disease dynamics based on the application of the replication–transmission relativity theory [Citation3],
testing the feasibility of the roadmap by applying it to the development of a multiscale model of hookworm infection as a paradigm and
illustrating the application of regular perturbation theory [Citation5] in the transformation of units of parameters in the reduction of scale order in the development of multiscale models of infectious disease dynamics.
The replication–transmission relativity theory [Citation3] states that at any level of organization of an infectious disease system there is no privileged or absolute scale which would determine disease dynamics, only interactions between the microscale and macroscale. The central idea of this biological relativistic theory is that at every level of organization of an infectious disease system, the interaction between the microscale and the macroscale is a pathogen replication–transmission multiscale cycle established through a positive feedback mechanism.
With so many multiscale modelling methods which are seemingly disjoint and mutually exclusive, our recent work has sought to bring some order in the discussion on the development of multiscale models of infectious disease dynamics based on the replication–transmission relativity theory [Citation3] by establishing what to include under the umbrella term ‘multiscale modelling of infectious disease dynamics’ and how to categorize various multiscale models [Citation6,Citation19]. The study established that there are five different generic categories of multiscale models that can be developed at the different levels of organization of an infectious disease system namely [Citation1,Citation6]: (a) individual-based multiscale models (IMSMs), (b) nested multiscale models (NMSMs), (c) embedded multiscale models (EMSMs), (d) hybrid multiscale models (HMSMs) and (e) coupled multiscale models (CMSMs) with each of these categories having several different classes of multiscale models. The intention was that it makes it not only easier to understand and compare different multiscale models but also makes it easier for researchers to know where to start when first creating their own multiscale model so that they do not end up re-inventing similar strategies under different names.
In the development of the different categories of multiscale models [Citation1,Citation6], the form of interaction between the microscale and the macroscale for these multiscale models necessarily assumes the replication–transmission relativity theory [Citation3]. A grouping of infectious disease systems for which multiscale models can be developed that belong to some of the categories of multiscale models that are developed by explicitly incorporating the microscale and the macroscale at any level of organization of an infectious disease system that includes nested multiscale models, the embedded multiscale models and the hybrid multiscale models is as follows:
Infectious disease systems with a replication cycle at microscale: For these infectious disease systems, pathogen load increases their numbers by replication at the microscale. Once the minimum infectious dose is consumed, then the infection at the level of organization of interest is sustained by pathogen replication. For these infectious disease systems, super-infection, that is, repeated infection of the host before it recovers from the initial infectious episode, plays an insignificant effect on the dynamics of the infectious disease system. From a mathematical point of view, the macroscale in this case influences the microscale through initial conditions of the microscale submodel variables (initial infection). Nested multiscale models or nested multiscale models in their hybrid form are more suitable to describe such infectious disease systems.
Infectious disease systems without a replication cycle at microscale: An important distinct property of such infectious disease systems is that pathogen load at the level of organization of interest does not increase through replication. It increases through super-infection, that is, repeated infection before the host recovers from the initial infectious episode. Therefore, when these infectious disease systems are considered at whole organism level, host behaviour is a major factor influencing the disease burden in a particular community because certain ways of behaving, particularly with regard to sanitation and hygiene will result in greater disease transmission. Such infectious disease systems are best modelled by embedded multiscale models [Citation6,Citation7] or embedded multiscale models in their hybrid form.
The roadmap for the development of multiscale models of infectious disease systems presented in this article focus on both nested multiscale models and embedded multiscale models.
The rest of this article is structured as follows. In Section 2, we describe the multilevel organization while in Section 3 we present the multiscale nature of infectious disease dynamics based on the replication–transmission relativity theory. We establish, for the first time, that the multiscale models for infectious disease systems developed based on the replication–transmission relativity theory [Citation3] are of two main different scale orders: multiscale models of scale order 2 and multiscale models of scale order 1. Based on this multiscale understanding, we present a structured four-stage roadmap for the development of multiscale models of infectious disease systems in Section 4. The central idea in the roadmap is to be able to establish that the multiscale models of scale order 1 are derived from multiscale models of scale order 2 through fast-and-slow time scale analysis. The feasibility of the roadmap is tested by applying it to the development of a multiscale model of the population biology of hookworm infection as a paradigm in Section 5. The multiscale model is further analysed in Section 6. The analytical results of the multiscale model of hookworm infection obtained in Section 6 are further confirmed by numerical results in Section 7. We finalize the paper with a discussion and concluding remarks in Section 8.
2. The levels of organization concept in the replication–transmission relativity theory
In the context of the replication–transmission relativity theory, infectious disease systems are considered as multilevel and multiscale complex systems [Citation1,Citation19]. The central idea of the replication–transmission relativity theory is that at every level of organization of an infectious disease system there is interdependence of scales within each level of organization while the levels are independent so that the scales within each level of organization can be analysed independently using multiscale modelling methods. Therefore, one of the key concepts introduced by the replication–transmission relativity in the development of multiscale modelling of infectious disease dynamics is the levels of organization concept. However, the replication–transmission relativity theory as a biological relativity theory for multiscale modelling in living systems is unique in that it draws from general relativity in physics [Citation4]. It draws from general relativity in physics [Citation17] because its formulation [Citation3] was based on the foundational idea that just as Newtonian gravity gave way to Einstein's general relativity in physical systems [Citation4], so too can the Newtonian models of biological processes give way to general relativistic models in living systems.
In the context of Einstein's general relativity theory [Citation17], from which the replication–transmission relativity is based on [Citation4], time is not a separate thing from space. It is part of a single concept called space–time which combines three dimensions of space and one dimension of time into a single four-dimensional manifold where the rules of geometry are different from the geometry of ordinary Euclidean space. However, the main idea put forward by the general relativity theory is that the four-dimensional space–time is a curved continuum. But, because of the levels concept introduced by the replication–transmission relativity, then the main distinction between the organization of space–time in Einstein's general relativity theory and in the replication–transmission relativity theory is that in Einstein's general relativity theory space–time is a curved four-dimensional continuum, while in the context of the replication–transmission relativity theory the curved four-dimensional continuum is discretized into four-dimensional regions of space–time called levels of organization.
Figure illustrates a conceptual representation of the seven main four-dimensional discrete and curved space–time regions called levels of organization of an infectious disease system based on the replication–transmission relativity theory which are [Citation1,Citation3,Citation19]: (a) the cell level – which consists of the within-cell scale as the microscale and the between-cell scale as the macroscale, (b) the tissue level – which consists of the within-tissue scale which as the microscale and the between-tissue scale as the macroscale, (c) the organ level or microcommunity level – which consists of the within-organ or within-microcommunity scale as the microscale and the between-organ or between-microcommunity scale as the macroscale, (d) the microecosystem level – which consists of the within-microecosystem scale as the microscale and the between-microecosystem scale as the macroscale, (e) the whole organism level – which consists of the within-whole organism scale as the microscale and the between-whole organism scale as the macroscale, (f) the macrocommunity level – which consists of the within-macrocommunity scale as the microscale and the between-macrocommunity scale as the macroscale and (g) the macroecosystem level – which consists of the within-macroecosystem scale as the microscale and the between-macroecosystem scale as the macroscale. The dotted lines in Figure indicate a multiscale cycle associated with pathogen dynamics within each curved four-dimensional level of organization of an infectious disease system, which can be a primary multiscale cycle, secondary multiscale cycle or tertiary multiscale cycle [Citation1,Citation19] depending on the level of organization under consideration.
Figure 1. A conceptual representation of the seven main levels of organization of an infectious disease system in the curved and discretized four-dimensional space–time which combines the three dimensions of space and one dimension of time into a single four-dimensional manifold based on the replication–transmission relativity theory. The seven main levels of organization of an infectious disease system are [Citation1,Citation3,Citation6,Citation19]: (a) the cell level – which consists of the within-cell scale as the microscale and the between-cell scale as the macroscale, (b) the tissue level – which consists of the within-tissue scale which as the microscale and the between-tissue scale as the macroscale, (c) the organ level or microcommunity level – which consists of the within-organ or within-microcommunity scale as the microscale and the between-organ or between-microcommunity scale as the macroscale, (d) the microecosystem level – which consists of the within-microecosystem scale as the microscale and the between-microecosystem scale as the macroscale, (e) the whole organism level – which consists of the within-whole organism scale as the microscale and the between-whole organism scale as the macroscale, (f) the macrocommunity level – which consists of the within-macrocommunity scale as the microscale and the between-macrocommunity scale as the macroscale and (g) the macroecosystem level – which consists of the within-macroecosystem scale as the microscale and the between-macroecosystem scale as the macroscale.
![Figure 1. A conceptual representation of the seven main levels of organization of an infectious disease system in the curved and discretized four-dimensional space–time which combines the three dimensions of space and one dimension of time into a single four-dimensional manifold based on the replication–transmission relativity theory. The seven main levels of organization of an infectious disease system are [Citation1,Citation3,Citation6,Citation19]: (a) the cell level – which consists of the within-cell scale as the microscale and the between-cell scale as the macroscale, (b) the tissue level – which consists of the within-tissue scale which as the microscale and the between-tissue scale as the macroscale, (c) the organ level or microcommunity level – which consists of the within-organ or within-microcommunity scale as the microscale and the between-organ or between-microcommunity scale as the macroscale, (d) the microecosystem level – which consists of the within-microecosystem scale as the microscale and the between-microecosystem scale as the macroscale, (e) the whole organism level – which consists of the within-whole organism scale as the microscale and the between-whole organism scale as the macroscale, (f) the macrocommunity level – which consists of the within-macrocommunity scale as the microscale and the between-macrocommunity scale as the macroscale and (g) the macroecosystem level – which consists of the within-macroecosystem scale as the microscale and the between-macroecosystem scale as the macroscale.](/cms/asset/681755fa-b089-431f-a285-d23c62e163e6/tjbd_a_2255066_f0001_oc.jpg)
For now, the idea of discretized space–time in living systems is new. However, the curved nature of space–time in living systems is not surprising because the mathematical point being made is a necessary one since anything, other than empty space, necessarily curves space–time. This is because anything, other than empty space, has a non-zero stress–energy–momentum tensor, even when it has no rest mass [Citation4]. The manner and degree to which anything curves space–time is determined by that anything's stress–energy–momentum tensor: a mathematical quantity that includes energy, momentum pressure and internal stresses [Citation4]. However, at this stage, the scientific community is used to this idea in relation to the structure of the universe and the way in which space–time is distorted by mass and energy to create phenomena such as gravitational lensing [Citation13,Citation14] according to Einstein's general relativity. As a result, it is usually assumed that, on smaller scales, such as in the case of living systems, the standard representations of space–time are sufficient. We are currently investigating to establish whether the space–time should also be incorporated in explicit terms in the submodels we use in multiscale models of infectious disease dynamics.
The implication of the replication–transmission relativity theory is that a level of organization is the smallest unit of decomposition of a complex system which can manifest some of the known properties of infectious disease systems as complex system [Citation19]. Therefore, in the context of the replication–transmission relativity theory, a level of organization consists of two scales, a microscale and a macroscale, whose aggregate dynamics manifests some of the properties of complex systems and is therefore characterized by
Hierarchy of scales – so that the scales at a particular level of organization are hierarchically organized in space–time in such a way that processes at those scales occur at different time scales.
Emergence – so that patterns emerge from interaction of processes of the scales of a level of organization.
Openness – so that it may be difficult to determine and fix the boundaries of processes of scales of a level of organization because living systems are open systems.
A history – so that the past dynamics of processes and mechanisms determines the present behaviour of the processes and mechanisms of scales of a level of organization.
Co-evolution – so that as the environment within the spatial scales at particular level of organization changes so does the organisms implicated in disease dynamics at that level of organization of the infectious disease system to ensure survival of the organisms (i.e. adaptation).
Self organized – so that there is spontaneous and robust overall order in the dynamics of processes of the scales of a level of organization.
Therefore, a level of organization is the smallest unit of decomposition of a complex system which can manifest some of the known properties of a complex system which include both (a) structural and functional organizational properties of scales of a complex system, and (b) dynamical behavioural properties of scales and processes within the scales of a complex system.
3. The multiscale nature of infectious disease systems
To be able to present the structured roadmap for the development of multiscale models of infectious disease systems, we need to understand first the multiscale nature of infectious disease systems in general. The multiscale nature of infectious dynamics considered in this study is based on the replication–transmission relativity theory [Citation3]. Our idea of the distinction between complex systems and simple systems in the context of their multiscale organization is that in complex systems each different scale is associated with a different process or mechanism while in simple systems multiple scales are associated with the same process or mechanism. In the case when one process or mechanism dominates at multiple scales in a system, then complexity usually does not emerge, so the corresponding system is simple and can be described using standard conservation laws or relationships. Complexity only emerges when at least two or more dominant processes or mechanisms are involved at two or more different scales such that each process or mechanism occurs at different scale in space–time. Based on this complex systems perspective to multiscale modelling of infectious dynamics, we now discuss the multiscale nature of infectious disease system. Since a level of organization is the smallest unit of decomposition of a complex system which can manifest all the known properties of a complex system, our description of the multiscale nature of infectious disease dynamics is focused on a level organization.
Although there is agreement among scientists that an infectious disease is a multilevel and multiscale complex system, a major source of confusion in multiscale modelling of infectious disease dynamics is what constitutes a level and what constitutes a scale. In this study, and consistent with the dictates of the replication–transmission relativity theory [Citation3], a level refers to a constructed organizational hierarchy in which: (a) the curved space–time is treated as being discrete and hierarchically structured into a microscale and a macroscale, and (b) it is assumed that this heterogeneity in space–time induces different responses by the pathogen leading to pathogen undergoing pathogen replication or reproduction at the microscale at an assumed fast time scale t, and pathogen transmission at the macroscale at an assumed slow time scale τ. Therefore, central to this multiscale nature is the idea that each of the two processes, that is, pathogen replication/reproduction and pathogen transmission progresses at an appropriate time scale determined by the spatial scale appropriate to that process. Further, the exchange of pathogen between the two heterogeneous scales (i.e. microscale and macroscale) is through two processes: (c) pathogen excretion/shedding – which involves pathogen movement from the microscale to the macroscale and (d) infection/super-infection by pathogen – which involves movement of pathogen from macroscale to microscale. These ideas about the multiscale nature of an infectious disease system at a particular level of organization of an infectious disease system are illustrated in Figure in the form of a multiscale cycle established through a positive feedback mechanism. In Figure , the dotted line also indicates a primary multiscale cycle associated with pathogen dynamics a curved four-dimensional level of organization of an infectious disease system [Citation19].
Figure 2. A conceptual diagram of a multiscale cycle of an infectious disease system based on the replication–transmission relativity theory [Citation3] in the curved and discretized four-dimensional space–time which combines the three dimensions of space and one dimension of time into a single four-dimensional manifold. The multiscale cycle is established through a positive feedback mechanism involving four disease processes across the microscale and the macroscale: (a) infection or super-infection by pathogen process – which involves the movement of the pathogen from the macroscale to the microscale, (b) pathogen replication or reproduction process at the microscale, (c) pathogen shedding or excretion – which involves movement of pathogen from microscale to macroscale, and (d) pathogen transmission at the macroscale.
![Figure 2. A conceptual diagram of a multiscale cycle of an infectious disease system based on the replication–transmission relativity theory [Citation3] in the curved and discretized four-dimensional space–time which combines the three dimensions of space and one dimension of time into a single four-dimensional manifold. The multiscale cycle is established through a positive feedback mechanism involving four disease processes across the microscale and the macroscale: (a) infection or super-infection by pathogen process – which involves the movement of the pathogen from the macroscale to the microscale, (b) pathogen replication or reproduction process at the microscale, (c) pathogen shedding or excretion – which involves movement of pathogen from microscale to macroscale, and (d) pathogen transmission at the macroscale.](/cms/asset/0fcfe8a7-8fdd-4ff8-bc77-ea6b689973c1/tjbd_a_2255066_f0002_oc.jpg)
From Figure , we note that considering the multiscale dynamics of an infectious disease system based on the replication–transmission relativity theory [Citation3], we establish that modelling the transition of the pathogen from the microscale where replication or reproduction often occurs to the macroscale where pathogen transmission often occurs through infection/super-infection, and then back to the microscale from the macroscale through pathogen shedding or excretion gives the spatial multiscale perspective to the multiscale dynamics of an infectious disease system. Similarly, the two processes which happen at these different spatial scales, that is, pathogen replication or reproduction at the microscale at fast time scale and pathogen transmission at the macroscale at slow time scale also give rise to the temporal multiscale perspective to multiscale dynamics of an infectious disease system. In line with the dictates of the replication–transmission relativity theory [Citation3], the multiscale dynamics of an infectious disease system at a typical level of organization as illustrated in Figure is conceptualized to involve four main processes [Citation1]: (a) infection/super-infection by pathogen process, (b) pathogen replication/reproduction process, (c) pathogen shedding/excretion process and (d) pathogen transmission process. Therefore, in the context of the replication–transmission relativity theory [Citation3] of infectious disease dynamics, the variety of multiscale models that can be developed at a particular level of organization of an infectious disease system are of two different scale orders, which are:
Multiscale models of scale order 2: These are multiscale models developed at a particular level of organization of an infectious disease system when the microscale and the macroscale are considered to be hierarchically organized in both space and time. When such multiscale models are written down in terms of ordinary differential equations where space–time is not explicitly incorporated into the multiscale model, model state variables at both the microscale and the macroscale vary at different time scales and parameters of both the microscale and the macroscale have different units. We refer to such multiscale models as multiscale models of scale order 2. Further, such multiscale models are not easy to analyse because of the time scale mismatch over different spatial scales.
Multiscale models of scale order 1: These are multiscale models developed at a particular level of organization of an infectious disease system when the microscale and the macroscale are considered to be hierarchically organized in space only. When such multiscale models are written down in terms of ordinary differential equations where space–time is not explicitly incorporated into the multiscale model, model state variables at both the microscale and the macroscale vary at same time scales and parameters of both the microscale and the macroscale have the same units. We refer to such multiscale models as multiscale models of scale order 1. Further, such multiscale models are easy to analyse because of there is no time scale mismatch over different spatial scales. For examples of these multiscale models see the published papers [Citation7,Citation9,Citation12,Citation15,Citation16].
In the context of multiscale nature of infectious disease dynamics conceptually illustrated in Figure , the method development of multiscale models which explicitly incorporate the microscale and the macroscale such as nested multiscale models, embedded multiscale models and hybrid multiscale models [Citation1,Citation6] involves up-scaling individual infectious to population infectiousness (which models pathogen excretion or shedding from microscale to macroscale) and down-scaling pathogen from population transmission to individual transmission (which models infection or super-infection by pathogen of the microscale). In nested multiscale models of infectious disease dynamics, the macroscale influences the microscale through initial infective inoculum dose of the microscale which involves the movement of pathogen from macroscale into the microscale. From a mathematical point of view, the macroscale in this case influences the microscale through initial conditions of the microscale submodel variables (initial infective inoculum dose) [Citation7,Citation8]. In embedded multiscale models of infectious disease dynamics, the macroscale influences the microscale continuously through super-infection (i.e. repeated infection before the host recovers from an infectious episode) which also involves the movement of pathogen from the macroscale to the microscale. From a mathematical point of view, the macroscale influences the microscale through scaled down macroscale variables and parameters [Citation7,Citation8]. As illustrated in Figure , the idea of scale based on the replication–transmission relativity theory [Citation3] is deeply entrenched to our understanding of space–time in infectious disease dynamics. In the following section, we use this present understanding of the multiscale nature of infectious disease to present a structured roadmap for the development of multiscale models of infectious disease systems.
4. The roadmap for the development of multiscale models of infectious disease systems
The roadmap for the development of multiscale models in this study is a four-stage process at host level of organization of the disease system as illustrated in Figure . The proposed roadmap can be used to develop multiscale models of infectious disease systems which have a replication cycle at microscale – which are nested multiscale models of scale order 1 if they incorporate the spatial scale only or of scale order 2 if they incorporate both the spatial scale and the time scale. The same roadmap can also be used to develop multiscale models for infectious disease systems which have no replication cycle at microscale – which are embedded multiscale models of scale order 1 if they incorporate the spatial scale only or of scale order 2 if they incorporate both the spatial scale and the time scale. The development of nested multiscale models ends with Stage III while the development of embedded multiscale models ends with Stage IV. At this stage, there are no known methods for the development of embedded multiscale. The best efforts by the scientific community have only so far produced embedded multiscale models of scale order 1 [Citation3,Citation7,Citation12,Citation15,Citation16]. But even then, the derivation of the embedded multiscale models of scale order 1 is not fully explained in current scientific literature to justify their multiscale nature. The general derivation of embedded multiscale is explained for the first time as illustrated in Figure . However, the application of this roadmap to the development of individual-based multiscale models is not appropriate because they do not explicitly incorporate the four disease processes (i.e. (a) infection or super-infection by pathogen process, (b) pathogen replication or reproduction process, (c) pathogen shedding or excretion process and (d) pathogen transmission process) at the microscale and macroscale of a level of organization of an infectious disease system as illustrated in Figure .
In what follows, we explain the four stages in the development of multiscale models of infectious disease systems.
STAGE I: Develop single-scale submodels for infectious disease system: This involves an understanding of the life cycle of the pathogen implicated in the transmission of the infectious disease system and then translating that understanding into variables of the infectious disease system at both the microscale and the macroscale. Consider a single host species, and single pathogen species/strain infectious disease system. Suppose that
(1)
(1) are the microscale variables at within-host scale which vary at fast time scale t, where
is the pathogen load in the first life stage in the infected host and
is the pathogen load in the last life stage in the infected host (also assumed to be the life stage that is excreted or shed into the macroscale or environment) with
being the pathogen load in the intermediate life stages at the microscale so that
(2)
(2) are the parameters associated with the microscale variables with units of per unit time different from macroscale parameters. Suppose also that
(3)
(3) are the variables at macroscale of the pathogen load which vary at slow time scale τ so that
is the pathogen load in the first life stage at macroscale and
is the pathogen load in the last life stage at macroscale (also assumed to be the infectious life stage) while
are the pathogen load in the intermediate life stages at macroscale. In addition, suppose that
(4)
(4) are the variables describing host populations at macroscale which vary at slow time scale τ so that
is the population of susceptible hosts and
is the population of infected hosts (also assumed to be the infectious) while
are the populations of infected hosts in the other intermediate infection categories at the macroscale so that
(5)
(5) are the demographic and epidemiological parameters at macroscale associated with demographic and epidemiological variables at macroscale, with units of per unit time different from microscale parameters. The variables at the microscale and macroscale are defined and summarized in Table .
Figure 3. The roadmap for the development of multiscale models of infectious disease systems based on the replication–transmission relativity theory [Citation3].
Based on these variables and parameters at the microscale and macroscale, the submodel for the between-host-scale in general form is
(6)
(6) Further, the submodel for at microscale in general form is
(7)
(7) These submodels are then integrated into a nested multiscale model of scale order 2 in Stage II.
STAGE II: Integrate the submodels into a nested multiscale model of scale order 2: This involves up-scaling individual infectiousness to population infectiousness so that the nested multiscale model of scale order 2 is formulated. From a mathematical point of view, microscale variables
become part of variables at macroscale as shown in the derived nested multiscale model of scale order 2 (Equation8
(8)
(8) ).
(8)
(8) This nested multiscale model of order 2 (Equation8
(8)
(8) ) does not incorporate super-infection and is therefore not suitable for analysing the dynamics of infectious disease systems with no pathogen replication cycle at microscale because it does not incorporate super-infection. It is also not easy to analyse nested multiscale model of order 2 or any multiscale model of scale order 2 because of time scale mismatch over different spatial scales of the variables. In the next stage, the nested multiscale model of scale order 2 (Equation8
(8)
(8) ) is converted into a nested multiscale model of scale order 1 and then further converted into an embedded multiscale model of scale order 1 by incorporating super-infection, which is a hallmark of the disease systems with no replication cycle at microscale.
STAGE III: Convert the nested multiscale model of scale order 2 into an embedded multiscale of scale order 1: This involves two steps. In the first step, the nested multiscale model of scale order 2 (Equation8
(8)
(8) ) is converted into a nested multiscale model of scale order 1 through fast-and-slow time scale analysis. This involves expressing the slow time-scale and the fast time-scale in terms of each other using the relationship
, where
and ϵ being a constant highlighting the fast time-scale t of the microscale submodel, compared to the slow time-scale τ of the macroscale submodel. By performing a fast-and-slow time scale analysis and then choosing a suitable value for ϵ which transforms the macroscale parameters so that they have the same units as the microscale parameters, the nested multiscale model (Equation8
(8)
(8) ) becomes a nested multiscale of scale order 1 of the form (Equation9
(9)
(9) )
(9)
(9) This first step completes the development of nested multiscale models of infectious disease systems with a replication cycle at microscale. For infectious disease systems with no replication cycle at microscale, this requires the development of embedded multiscale models by incorporating super-infection into the nested multiscale model of scale order 1. The second step involves incorporating super-infection and the corresponding excretion or shedding of the pathogen into the nested multiscale model of scale order 1 (Equation9
(9)
(9) ) so that it becomes an embedded multiscale model of scale order 1 (Equation10
(10)
(10) ).
(10)
(10) In mathematical terms, the development embedded multiscale model of scale order 1 (Equation10
(10)
(10) ) through incorporating super-infection and the corresponding excretion or shedding of pathogen involves down-scaling of macroscale variables and parameters and up-scaling of microscale variables and parameters as illustrated in Figure . This down-scaling and up-scaling of variables and parameters cannot be easily implemented on nested multiscale model of scale order 2 because of the time scale mismatch over different spatial scales of the variables. For an infectious disease system, the transition from one scale to another indicates or represents shifts in disease processes, that is pathogen replication or reproduction at the microscale and pathogen transmission at the macroscale. However, the greatest challenge is in methodological difficulties on specific implementation approaches for scaling (down-scaling and up-scaling) in space and time and in converting dimensions across spatial and temporal scales when coupling the submodels of a multiscale model [Citation1]. Down-scaling and up-scaling as illustrated in Figure are now possible because the nested multiscale model of scale order 1 has variables which vary at the same time scale and parameters of the same units. This embedded multiscale model of scale 1 (Equation10
(10)
(10) ) is much simpler to analyse and is more suitable now for use to study the multiscale dynamics of infectious disease systems. In the next stage, we implement Stage IV to show the derivation of the equivalent embedded multiscale model of scale order 2.
STAGE IV: Convert the embedded multiscale model of scale order 1 into an embedded multiscale model of scale order 2: This involves substituting back the variables and parameters in their original time scales and units of measurement.
(11)
(11) This embedded multiscale model of scale order 2 (Equation11
(11)
(11) ) is not easy to analyse. But since it is equivalent to the embedded multiscale model of scale order 1 (Equation10
(10)
(10) ), we study the multiscale dynamics of the infectious disease system using the embedded multiscale model of scale order 1 (Equation10
(10)
(10) ).
Table 1. A summary of the variables at the macroscale and microscale based on life cycle of pathogen at a particular level of organization of an infectious disease system.
In the following section, we test the feasibility of this roadmap for the development of multiscale models of infectious disease systems by applying it to the development of a multiscale model of the population biology of hookworm infection as a paradigm.
5. Application of the roadmap to the development of a multiscale model for hookworm disease system as a paradigm
Hookworm infection is caused by a soil transmitted nematode called hookworm. This disease is of public health importance because approximately a billion people in the world's tropical regions are infected by this intestinal parasite [Citation29]. The multiscale model for hookworm infection presented in this paper is derived from implementing the following four-stage process which constitute the roadmap for the development of multiscale models of infectious disease systems as follows.
5.1. STAGE I: development of single-scale submodels for hookworm infection
Taking into consideration the life cycle of the human ascariasis worm parasite [Citation30], the process-based multiscale model of the pathogen-centred disease system form in terms of its pathogen population dynamics can be established by integrating variables at the microscale and the macroscale is as follows. (a) At the macroscale, we have five variables ,
,
,
and
which occur as slow time scale τ. (b) At the microscale, we have four variables
which occur at fast time scale t. We summarize the description of these variables as follows:
At the microscale, we have the following variables:
– hookworm parasite population in the first life stage. This first life stage is introduced into the microscale from the macroscale through infection or super-infection. Following infection or super-infection, this first life stage migrates to the gastrointestinal tract where it can either die naturally at an assumed rate of
or molt at an assumed rate
, to form the second life stage or first intermediate life stage.
– hookworm parasite population in the second life stage. This second life stage is reduced either by natural death at an assumed rate
, or by undergoes a second molt at a rate
to form the population of hookworm in second intermediate life stage or third life stage.
– hookworm parasite population in the third life stage. This population of mature female hookworm parasites is assumed to have a natural death rate of
and progress to mature female worms an assumed rate
. The parameter
multiplying
models the assumption that a proportion of the immature hookworm parasites are females. These mature female hookworm parasites give rise to final life stage of hookworm parasite at microscale, which are the hookworm parasite eggs.
– hookworm parasite population in the fourth and final life stages which is also the population of hookworm parasite eggs laid by mature female hookworm parasites. These hookworm parasite eggs either degrade at an assumed rate
or are excreted or shed into the macroscale environment at an assumed rate
. Based on the four variables, the microscale submodel can be derived to become
(12)
(12) The four variables of the microscale submodel
(13)
(13) vary at fast time scale t. The units of eight microscale submodel parameters
(14)
(14) are per day.
At the macroscale, we have the following variables:
– population of susceptible humans.
– population infected humans.
– population of ascaris worm parasites in the first life stage at macroscale. This first life stage is introduced at macroscale by the excretion of hookworm parasite eggs from the microscale. This first life stage of hookworm parasite may deplete through degrading of the eggs at an assumed rate
, or the eggs may hatch at an assumed rate
to give rise to second life stage of hookworm parasite at macroscale.
– population of hookworm parasites in the second life stage. This population of hookworm parasites molts at an assumed rate of
to give rise to the last life stage of hookworm parasite at macroscale, which is infectious to the human host.
– population of hookworm parasites in the last life stage at macroscale. This population of hookworm parasites in this life stage may be depleted by having an average life span of
. Further, this last life stage of hookworm parasites initiates the reproductive cycle again by establishing its first life stage at microscale through super-infection. Taking into account all these variables, the macroscale submodel is derived to be
(15)
(15) Like in all other helminth infections, it is not easy to establish who-infects-who for hookworm infection. As a result, the transmission of this parasitic worm in the macroscale submodel model for hookworm infection (Equation15
(15)
(15) ) is modelled by
where
is a Holling-type I functional form used to model the probability of being infected with hookworm parasite in an environment with hookworm parasite load
. In general, any function
, with the specification that
representing the probability of being infected with hookworm parasite in an environment with hookworm parasite load
can be used to model the transmission of hookworm infection. However, since the function
is a probability function, it must have the following properties:
Property I: The probability of infection vanishes in the absence of pathogen [i.e.
] and approaches 1 as the macroscale worm parasite load becomes large [i.e.
].
Property II: The probability of infection
increases with the macroscale worm parasite load
, that is,
, where prime denotes derivative with respect to the argument.
In the context of the proposed roadmap for the development of multiscale models of infectious disease systems, any function,
, with the above properties can be used in place of that derived from Holling-type I functional form. The five variables of the between-host scale submodel
(16)
(16) are assumed to vary at slow time scale τ. The units of the 10 macroscale submodel parameters
(17)
(17) are per annum.
In the next section, we invoke Stage II of the roadmap for the development of multiscale models of infectious disease systems to derive the nested multiscale model of hookworm infection of scale order 2.
5.2. STAGE II: integrating the submodels of hookworm infection into a nested multiscale model of scale order 2
The submodels for hookworm infection can be integrated into the following nested multiscale model of scale order 2 by first substituting constant individual infectiousness at macroscale by variable individual infectiousness
from the microscale. This has a result of up-scaling this individual infectiousness to population infectiousness. This gives the following nested multiscale model of scale order 2 (Equation18
(18)
(18) ).
(18)
(18) The multiscale model (Equation18
(18)
(18) ) is in the form of (Equation8
(8)
(8) ) of Stage II of the roadmap for the development of multiscale models of infectious disease systems and so is a nested multiscale model of scale order 2. In what follows, we implement Stage III of the roadmap for the development of multiscale models of infectious disease systems.
5.3. STAGE III: converting the nested multiscale model of hookworm infection of scale order 2 into an embedded multiscale model of scale order 1
First, the nested multiscale model of scale order 2 (Equation18(18)
(18) ) is converted into a nested multiscale model of scale order 1 by expressing the slow time-scale and the fast time-scale in terms of each other using the relationship
, where
and ϵ being a constant highlighting the fast time scale t of the microscale submodel variables, compared to the slow time-scale τ of the macroscale submodel variables. By performing a fast-and-slow time scale analysis, the nested multiscale model of scale order 2 (Equation18
(18)
(18) ) becomes
(19)
(19) For the nested multiscale model of scale order 2 (Equation19
(19)
(19) ), choose
so that
(20)
(20) The macroscale parameters (Equation20
(20)
(20) ) of the nested multiscale model of scale order 2 (Equation18
(18)
(18) ) have units of per annum. The chosen value of
transforms the macroscale parameters into new parameters
(21)
(21) which have the same units as the microscale parameters of per day. The nested multiscale model of scale order 2 (Equation18
(18)
(18) ) becomes a nested multiscale model of scale order 1 (Equation22
(22)
(22) ):
(22)
(22) However, the nested multiscale model of scale order 1 (Equation22
(22)
(22) ) is not suitable for describing hookworm infection. This is because, for hookworm disease system, the number of worms within an infected human host increases through super-infection, that is, repeated infection of the human host before it recovers from the initial infectious episode. Therefore, an embedded multiscale model for hookworm infection is the best model to describe this disease system. We develop the embedded multiscale model of scale order 1 by up-scaling and down-scaling variables and parameters of the nested multiscale model of scale order 1 (Equation22
(22)
(22) ) as described in Stage III of the roadmap for the development of multiscale models of infectious disease systems. The down-scaling and up-scaling here is based on an approached developed in [Citation9] where the reciprocal influence between the macroscale (i.e. between-whole human scale) and the microscale (i.e within-whole human scale) are modelled in the following way.
Influence of macroscale on microscale: The influence of the macroscale on the microscale is modelled by down-scaling uptake of the hookworm parasite at macroscale through infection of humans at the macroscale at a rate
to super-infection at microscale at a rate
. However, this representation of super-infection is a refinement of the approach in [Citation9] because the approach in [Citation9] overestimates the number of new infections. Here the number of new infections are assumed to be a proportion
of the existing cumulative number of infections.
Influence of microscale on macroscale: The influence of the microscale on the macroscale is modelled by up-scaling microscale excretion or shedding of the hookworm parasite at microscale at a rate
to macroscale at a rate
.
Implementing these changes on the nested multiscale model of scale order 1 (Equation22(22)
(22) ) gives us the following embedded multiscale model of scale order 1 (Equation23
(23)
(23) ).
(23)
(23) The addition of 1 in the infected class of the embedded multiscale model of hookworm disease system of scale order 1 (Equation23
(23)
(23) ) is due to the fact that the infection of a single host happens through a single transition defined by
with probability
. The embedded multiscale model of scale order 1 (Equation23
(23)
(23) ) is analysed in this article. It must be noted that this embedded multiscale model of scale order 1 is obtained without making any approximations except that the units of the macroscale submodel parameters are transformed into the same units as the microscale submodel parameters through a fast-and-slow time scale analysis. Figure is a schematic diagram of the embedded multiscale model of hookworm disease system of scale order 1 (Equation23
(23)
(23) ).
Figure 4. The schematic diagram of the embedded multiscale model of hookworm disease system of scale order 1 (Equation23(23)
(23) ).
![Figure 4. The schematic diagram of the embedded multiscale model of hookworm disease system of scale order 1 (Equation23(23) {1.dSH(t)dt=ΛH−βHPH(t)SH(t)P0+PH(t)−μHSH(t),2.dIH(t)dt=βHPH(t)SH(t)P0+PH(t)−(μH+δH)IH(t),3.dPF(t)dt=[IH(t)+1]αhPh(t)−(μF+αF)PF(t),4.dPM(t)dt=αFPF(t)−(μM+αM)PM(t),5.dPH(t)dt=αMPM(t)−αHPH(t),6.dPf(t)dt=βHPH(t)[SH(t)−1][P0+PH(t)]ΦH[IH(t)+1]−(μf+αf)Pf(t),7.dPs(t)dt=αfPf(t)−(μs+αs)Ps(t),8.dPm(t)dt=ϕsαsPs(t)−μmPm(t),9.dPh(t)dt=αmPm(t)−(μh+αh)Ph(t).(23) ).](/cms/asset/723e4b5f-b5c9-44e3-9e89-8699a20be31d/tjbd_a_2255066_f0004_oc.jpg)
5.4. STAGE IV: converting the embedded multiscale model of hookworm infection of scale order 1 into an embedded multiscale model of scale order 2
From the embedded multiscale model of scale order 1 (Equation23(23)
(23) ), we can then work backwards to obtain the corresponding embedded multiscale model of scale order 2. This is achieved by replacing original parameters in their original units and variables varying at their appropriate time scales to get the embedded multiscale model of scale order 2 (Equation24
(24)
(24) )
(24)
(24) The embedded multiscale model of hookworm infection of scale order 2 (Equation24
(24)
(24) ) is not easy to analyse because of the time scale mismatch over different spatial scales of the variables. Since this multiscale model is equivalent to the embedded multiscale model of scale order 1 (Equation23
(23)
(23) ), we use the embedded multiscale model of scale order 1 (Equation23
(23)
(23) ) to analyse the multiscale dynamics of hookworm infection.
6. Analysis of the embedded multiscale model of hookworm infection of scale order 1
In this section, we present analytical results of the embedded multiscale model of hookworm infection of scale order 1 (Equation23(23)
(23) ). To obtain the reproductive number of the embedded multiscale model of hookworm infection of scale order 1 (Equation23
(23)
(23) ) we first obtain the disease-free equilibrium point by setting the left-hand side of this model equal to zero and also assume that
in the embedded multiscale model of hookworm infection of scale order 1 (Equation23
(23)
(23) ). Thus we get
(25)
(25) where
denotes the disease-free equilibrium of the embedded multiscale model of hookworm infection of scale order 1 (Equation23
(23)
(23) ). The local asymptotic stability of
can be established using the basic reproductive number. We calculate the basic reproduction number of the embedded multiscale model of hookworm infection of scale order 1 (Equation23
(23)
(23) ) by using the next-generation matrix approach [Citation18] as appropriate for environmentally transmitted infectious disease systems. In this case, the basic reproduction number of the embedded multiscale model of hookworm infection of scale order 1 (Equation23
(23)
(23) ) can be shown to be
(26)
(26) where
is the within-host scale partial reproductive number, and
is the between-host scale partial reproductive number. The reproductive number (Equation26
(26)
(26) ) of the embedded multiscale model of hookworm infection of scale order 1 (Equation23
(23)
(23) ) consists of two partial reproductive numbers. The two partial reproductive numbers are: (a) the microscale partial reproductive number and (b) the macroscale partial reproductive number. This implies that in multiscale dynamics of hookworm disease system the microscale and the macroscale influence each other through a positive feedback mechanism.
The existence and nature of the endemic equilibrium based on the embedded multiscale model of hookworm disease system of scale order 1 (Equation23(23)
(23) ) can be shown to dependent on the basic reproductive number (Equation26
(26)
(26) ). To derive the endemic equilibrium of the embedded multiscale model of hookworm disease system of scale order 1 (Equation23
(23)
(23) ) let
(27)
(27) be an endemic solution for the embedded multiscale model of hookworm disease system of scale order 1 (Equation23
(23)
(23) ). We then express
in terms of
as follows:
(28)
(28) In the expressions (Equation28
(28)
(28) ) for the endemic equilibrium
is given by
(29)
(29) Substitute Equation (Equation28
(28)
(28) ) in the equation for
which is given by
(30)
(30) Substituting for
in (Equation30
(30)
(30) ) by
from (Equation28
(28)
(28) ) and setting the left-hand side of (Equation30
(30)
(30) ) to zero, then the endemic equilibrium of of
can be obtained by solving the algebraic expression
(31)
(31) where
(32)
(32)
(33)
(33) Therefore,
(34)
(34) Note that C<0 for
while B is either positive or negative for
. We conclude that the embedded multiscale model of hookworm disease dynamics of scale order 1 (Equation23
(23)
(23) ) admits a single positive endemic equilibrium for
whenever
. Furthermore, we conclude that the macroscale endemic expressions for
are dependent on both microscale disease parameters and macroscale parameters and in turn, the microscale endemic expressions for
also dependent on both microscale and macroscale parameters. The obtained results here confirm the analytical results derived from the analysis of the reproductive number (Equation34
(34)
(34) ) that the microscale and the macroscale influence each other in a reciprocal way through a positive feedback mechanism.
7. Numerical study of the embedded multiscale model of hookworm infection of scale order 1
In this section, we present results from the numerical simulations of the embedded multiscale model of hookworm infection of scale order 1 (Equation23(23)
(23) ) that also confirm the analytical results obtained in the previous section. The parameters of the embedded multiscale model of hookworm infection of scale order 1 (Equation23
(23)
(23) ) are summarized in Table . The main limitation of this study is that using the embedded multiscale model of hookworm infection of scale order 1 (Equation23
(23)
(23) ) is that parameters used to evaluate it could not be chosen from a single epidemic country situation. Where demographic and epidemiological parameters specific to hookworm disease system could not be found, the demographic parameters marked [
] and the epidemiological parameters marked [
] were used and were chosen to be within ranges of values for developing countries for helminth infections.
Table 2. Table of parameter values for the embedded multiscale model of hookworm disease system of scale order 1 (Equation23(23)
(23) ).
The two sections that follow contain simulations for the embedded multiscale model of scale order 1 of hookworm infection (Equation23(23)
(23) ) based on the following initial conditions:
,
,
,
,
,
,
,
, and
. The disease parameter values are presented in Table .
7.1. Methods
In this section, we construct a nonstandard finite difference scheme (NSFDS) based on Mickens' techniques and guidelines [Citation26] for the embedded multiscale model of hookworm infection at scale order 1 (Equation23(23)
(23) ). Most numerical methods establish solutions through the linearization of the nonlinear terms in the system of differential equations. For infectious disease systems such as hookworm infection, the nonlinear terms need to be retained in the numerical simulations because they model important disease processes such as (a) super-infection by the worm parasite, which models continuous transition of the worm parasite from the macroscale to the microscale (b) sexual reproduction of the worms at microscale (if sexual reproduction is explicitly incorporated into the multiscale model), (c) worm parasite shedding or excretion, which models continuous transition of the worm parasite from the microscale to the macroscale, and (d) worm parasite transmission at macroscale. The NSFD scheme is constructed based on the two fundamental rules [Citation25–28] as follows:
Non local approximation as follows:
For the discretization of the derivatives we have
where
the non-negative denominator [Citation26,Citation27] function satisfying
(35)
(35) for example
as
with the time domain
which is partitioned as
that is
where ϕ depends on step size h>0 and is chosen as
. where M depends on the problem parameters.
We also perform numerical simulations of the scheme. NSFD scheme for the embedded multiscale model of hookworm infection of scale order 1 is as follows. Let be approximations of
respectively for
and h is the time step size in the NSFD scheme. The solution sequence
must be non-negative to satisfy the underlying biological property of the mathematical model. The NSFDM scheme to solve the embedded multiscale model of hookworm infection of scale order 1 (Equation23
(23)
(23) ) is designed so that it satisfies the conservation law property proposed by Mickens [Citation25,Citation28]. The approximation of the embedded multiscale model of hookworm infection of scale order 1 (Equation23
(23)
(23) ) becomes
(36)
(36) where
and
.
Rearranging the Equation (Equation36(36)
(36) ), we get
(37)
(37) where
.
Since the embedded multiscale model of hookworm infection of scale order 1 (Equation23(23)
(23) ) deals with the human population and the hookworm parasite load at both the microscale and the macroscale, the constructed numerical scheme must guarantee positive values. It is clear from the developed numerical scheme that non negatives values will be produced since the time step h>0, and
,
then the numerators and the denominators are positive. This implies that
(38)
(38) Therefore the positivity of the numerical solution is guaranteed. The solution
(39)
(39) is calculated using the NSFDM scheme (Equation36
(36)
(36) ) as follows.
Select the initial values
Calculate
using the value
Using
,
and
calculate
Using
,
and
calculate
Using
and
calculate
Using
and
calculate
Using
,
,
and
calculate
Using
and
calculate
Using
and
calculate
Using
and
calculate
7.2. Results
We now present results of the numerical simulations of the embedded multiscale model of hookworm infection of scale order 1 (Equation23(23)
(23) ). All the numerical simulations were performed using MATLAB 22 programming language.
Figure shows the evolution of macroscale variables and
for the embedded multiscale model of hookworm infection of scale order 1 (Equation23
(23)
(23) ) for different values of the microscale parameter
:
,
and
. The results show that as shedding rate of the hookworm parasite from the microscale (i.e. within-host scale) increase, there is a corresponding increase in the between-host scale variables
and
at macroscale. Since
represents the rate of shedding of worm eggs into the environment (i.e. the rate of contamination of the environment with worm eggs by infected individuals), these results indicate that modifying certain ways of human behaviour with regards to sanitation and hygiene through interventions such as health education or the provision of proper sanitary facilities such as toilets in rural communities will contribute to the control of hookworm transmission at macroscale.
Figure 5. The evolution of macroscale variables and
for different values of the microscale parameter
:
,
and
for the embedded multiscale model of hookworm infection of scale order 1 (Equation23
(23)
(23) ).
![Figure 5. The evolution of macroscale variables IH,PF,PM and PH for different values of the microscale parameter αh: αh=0.3202, αh=0.03202 and αh=0.03202 for the embedded multiscale model of hookworm infection of scale order 1 (Equation23(23) {1.dSH(t)dt=ΛH−βHPH(t)SH(t)P0+PH(t)−μHSH(t),2.dIH(t)dt=βHPH(t)SH(t)P0+PH(t)−(μH+δH)IH(t),3.dPF(t)dt=[IH(t)+1]αhPh(t)−(μF+αF)PF(t),4.dPM(t)dt=αFPF(t)−(μM+αM)PM(t),5.dPH(t)dt=αMPM(t)−αHPH(t),6.dPf(t)dt=βHPH(t)[SH(t)−1][P0+PH(t)]ΦH[IH(t)+1]−(μf+αf)Pf(t),7.dPs(t)dt=αfPf(t)−(μs+αs)Ps(t),8.dPm(t)dt=ϕsαsPs(t)−μmPm(t),9.dPh(t)dt=αmPm(t)−(μh+αh)Ph(t).(23) ).](/cms/asset/5825d304-16a4-4664-aca2-362d631ab6c1/tjbd_a_2255066_f0005_oc.jpg)
Figure shows the evolution of macroscale variables and
for the embedded multiscale model of hookworm infection of scale order 1 (Equation23
(23)
(23) ), for different values of the microscale parameter
and
. The results show that as the rate of transition
of hookworm parasite from the first life stage to the second life stage at microscale increases, there is also a corresponding increase of macroscale variables
. Since
represents the rate of transition of hookworm parasite from the first life stage to the second life stage at microscale, the results indicate that interventions such as drugs that kill the hookworm parasite in the first life stage will contribute towards the reduction of hookworm infection transmission at macroscale.
Figure 6. The evolution of macroscale variables and
for different values of the microscale parameter
and
for the embedded multiscale model of hookworm infection of scale order 1 (Equation23
(23)
(23) ).
![Figure 6. The evolution of macroscale variables IH,PF,PM and PH for different values of the microscale parameter αf:αf=0.25,αf=0.025 and αf=0.0025 for the embedded multiscale model of hookworm infection of scale order 1 (Equation23(23) {1.dSH(t)dt=ΛH−βHPH(t)SH(t)P0+PH(t)−μHSH(t),2.dIH(t)dt=βHPH(t)SH(t)P0+PH(t)−(μH+δH)IH(t),3.dPF(t)dt=[IH(t)+1]αhPh(t)−(μF+αF)PF(t),4.dPM(t)dt=αFPF(t)−(μM+αM)PM(t),5.dPH(t)dt=αMPM(t)−αHPH(t),6.dPf(t)dt=βHPH(t)[SH(t)−1][P0+PH(t)]ΦH[IH(t)+1]−(μf+αf)Pf(t),7.dPs(t)dt=αfPf(t)−(μs+αs)Ps(t),8.dPm(t)dt=ϕsαsPs(t)−μmPm(t),9.dPh(t)dt=αmPm(t)−(μh+αh)Ph(t).(23) ).](/cms/asset/680f0bf6-04aa-4b83-8ed0-0af6567901c7/tjbd_a_2255066_f0006_oc.jpg)
Figure shows the evolution of macroscale variables and
for different values of the microscale parameter
and
for the embedded multiscale model of hookworm infection of scale order 1 (Equation23
(23)
(23) ). Similarly, these results show that as the rate of transition
of hookworm parasite from the second life stage to the third life stage at microscale increases, there is also a corresponding of macroscale variables
. Since
also represents the rate of transition of hookworm parasite from the second life stage to the third life stage at microscale, the results indicate that interventions such as drugs that kill the hookworm parasite in the second life stage will also contribute towards the reduction of hookworm infection transmission at macroscale.
Figure 7. The evolution of macroscale variables and
for different values of the microscale parameter
and
for the embedded multiscale model of hookworm infection of scale order 1 (Equation23
(23)
(23) ).
![Figure 7. The evolution of macroscale variables IH,PF,PM and PH for different values of the microscale parameter αm:αm=100,αm=1000 and αm=10000 for the embedded multiscale model of hookworm infection of scale order 1 (Equation23(23) {1.dSH(t)dt=ΛH−βHPH(t)SH(t)P0+PH(t)−μHSH(t),2.dIH(t)dt=βHPH(t)SH(t)P0+PH(t)−(μH+δH)IH(t),3.dPF(t)dt=[IH(t)+1]αhPh(t)−(μF+αF)PF(t),4.dPM(t)dt=αFPF(t)−(μM+αM)PM(t),5.dPH(t)dt=αMPM(t)−αHPH(t),6.dPf(t)dt=βHPH(t)[SH(t)−1][P0+PH(t)]ΦH[IH(t)+1]−(μf+αf)Pf(t),7.dPs(t)dt=αfPf(t)−(μs+αs)Ps(t),8.dPm(t)dt=ϕsαsPs(t)−μmPm(t),9.dPh(t)dt=αmPm(t)−(μh+αh)Ph(t).(23) ).](/cms/asset/1a815650-9a50-42c5-b9ed-a7e018c879c9/tjbd_a_2255066_f0007_oc.jpg)
Figure shows the evolution of microscale variables and
for different values of the macroscale parameter
and
for the embedded multiscale model of hookworm infection of scale order 1 (Equation23
(23)
(23) ). The results show that as the contact rate between infected individuals and the contaminated environment at macroscale increases, then hookworm parasite burden also increases at microscale. Therefore, interventions that reduce contact of individuals with a contaminated environments such as putting on shoes is expected to reduce the severity of hookworm infection at microscale.
Figure 8. The evolution of microscale variables and
for different values of the macroscale parameter
and
for the embedded multiscale model of hookworm infection of scale order 1 (Equation23
(23)
(23) ).
![Figure 8. The evolution of microscale variables Pf,Ps,Pm and Ph for different values of the macroscale parameter βH:βH=0.1,βH=0.01 and βH=0.001 for the embedded multiscale model of hookworm infection of scale order 1 (Equation23(23) {1.dSH(t)dt=ΛH−βHPH(t)SH(t)P0+PH(t)−μHSH(t),2.dIH(t)dt=βHPH(t)SH(t)P0+PH(t)−(μH+δH)IH(t),3.dPF(t)dt=[IH(t)+1]αhPh(t)−(μF+αF)PF(t),4.dPM(t)dt=αFPF(t)−(μM+αM)PM(t),5.dPH(t)dt=αMPM(t)−αHPH(t),6.dPf(t)dt=βHPH(t)[SH(t)−1][P0+PH(t)]ΦH[IH(t)+1]−(μf+αf)Pf(t),7.dPs(t)dt=αfPf(t)−(μs+αs)Ps(t),8.dPm(t)dt=ϕsαsPs(t)−μmPm(t),9.dPh(t)dt=αmPm(t)−(μh+αh)Ph(t).(23) ).](/cms/asset/9e8cfbf8-2e18-46bb-9c49-2b2a776f8d43/tjbd_a_2255066_f0008_oc.jpg)
Figure shows the evolution of microscale variables and
for different values of the macroscale parameter
and
for the embedded multiscale model of hookworm infection of scale order 1 (Equation23
(23)
(23) ). These results show that decontamination of environment of infectious life stage of hookworm parasite at macroscale decreases hookworm parasite load at microscale. Therefore, health interventions such as decontamination of the environment through application disinfectants such as hydrogen peroxide is expected to reduce hookworm parasite at microscale for infected individuals.
Figure 9. The evolution of microscale variables and
for different values of the macroscale parameter
and
for the embedded multiscale model of hookworm infection of scale order 1 (Equation23
(23)
(23) ).
![Figure 9. The evolution of microscale variables Pf,Ps,Pm and Ph for different values of the macroscale parameter αH:αH=0.8,αH=0.2 and αH=0.002 for the embedded multiscale model of hookworm infection of scale order 1 (Equation23(23) {1.dSH(t)dt=ΛH−βHPH(t)SH(t)P0+PH(t)−μHSH(t),2.dIH(t)dt=βHPH(t)SH(t)P0+PH(t)−(μH+δH)IH(t),3.dPF(t)dt=[IH(t)+1]αhPh(t)−(μF+αF)PF(t),4.dPM(t)dt=αFPF(t)−(μM+αM)PM(t),5.dPH(t)dt=αMPM(t)−αHPH(t),6.dPf(t)dt=βHPH(t)[SH(t)−1][P0+PH(t)]ΦH[IH(t)+1]−(μf+αf)Pf(t),7.dPs(t)dt=αfPf(t)−(μs+αs)Ps(t),8.dPm(t)dt=ϕsαsPs(t)−μmPm(t),9.dPh(t)dt=αmPm(t)−(μh+αh)Ph(t).(23) ).](/cms/asset/396ddc16-27c2-4392-a6ee-5b1b539eaf7b/tjbd_a_2255066_f0009_oc.jpg)
Figure shows the evolution of microscale variables and
for different values of the macroscale parameter
and
for the embedded multiscale model of hookworm infection of scale order 1 (Equation23
(23)
(23) ). From the numerical results, we note as
increases at macroscale, the microscale variables
and
also increase. Since
represents the proportion of new infections, these results imply that interventions such as mass drug administration that aim to reduce the number of infected individuals at microscale can reduce the hookworm parasite load at microscale, and thus reduce disease severity for an infected individual.
Figure 10. The evolution of microscale variables and
for different values of the macroscale parameter
and
for the embedded multiscale model of hookworm infection of scale order 1 (Equation23
(23)
(23) ).
![Figure 10. The evolution of microscale variables Pf,Ps,Pm and Ph for different values of the macroscale parameter ΦH:ΦH=0.3,ΦH=0.1 and ΦH=0.03 for the embedded multiscale model of hookworm infection of scale order 1 (Equation23(23) {1.dSH(t)dt=ΛH−βHPH(t)SH(t)P0+PH(t)−μHSH(t),2.dIH(t)dt=βHPH(t)SH(t)P0+PH(t)−(μH+δH)IH(t),3.dPF(t)dt=[IH(t)+1]αhPh(t)−(μF+αF)PF(t),4.dPM(t)dt=αFPF(t)−(μM+αM)PM(t),5.dPH(t)dt=αMPM(t)−αHPH(t),6.dPf(t)dt=βHPH(t)[SH(t)−1][P0+PH(t)]ΦH[IH(t)+1]−(μf+αf)Pf(t),7.dPs(t)dt=αfPf(t)−(μs+αs)Ps(t),8.dPm(t)dt=ϕsαsPs(t)−μmPm(t),9.dPh(t)dt=αmPm(t)−(μh+αh)Ph(t).(23) ).](/cms/asset/d5273a92-6a1b-4b53-a918-b458185bcb8f/tjbd_a_2255066_f0010_oc.jpg)
The numerical results confirm the analytical results obtained in Section 6 which show that there is reciprocal influence between hookworm parasite reproduction at microscale and hookworm parasite transmission at macroscale through a positive feedback mechanism. The results also confirm the existence of multiscale cycle involving hookworm parasite reproduction at microscale and hookworm parasite transmission at macroscale as illustrated in our initial conceptual understanding of the multiscale nature of the dynamics of these infectious disease systems illustrated in Figure . Bridging the gap between worm parasite reproduction at within-host (i.e. microscale) and worm parasite transmission at between-host scale (i.e. microscale) is at the heart of the structured four stage roadmap for the development of embedded multiscale models of infectious disease systems.
8. Discussion and conclusion
This article provided a structured roadmap for the development of multiscale models of infectious disease dynamics that integrates the microscale and macroscale based on the application of the replication–transmission relativity theory [Citation3]. The central idea in the four stage roadmap is to develop, in a structured way, simple multiscale models of infectious disease dynamics that can be used to explore the full multiscale cycle of the microscale–macroscale pathogen dynamics. The roadmap for the development of such multiscale models begins with expressing the life cycle of the pathogen implicated in the transmission of the infectious disease system in mathematical terms into submodels of the full multiscale model and ends up with an embedded multiscale model of the infectious disease system. The feasibility of the roadmap was tested by being applied to the development of a multiscale model of the population biology of hookworm infection as a paradigm. To the best of our knowledge, this work represents the first effort in which the method for development of embedded multiscale models is presented. In addition, the fast-and-slow time scale analysis presented in this article is the first of its kind that concurrently results in the reduction of scale order and transforming the parameters of multiscale model into appropriate units. In our analysis of the embedded multiscale model of hookworm infection of scale order 1, key points at multiple scales (within-host scale and between-host scale) for intervention that can drastically impact on control, elimination and even eradication of infectious disease systems were identified. In particular, it reveals multiple intervention points that cannot be simultaneously addressed when using single-scale models of infectious disease dynamics. The testing of the feasibility of the structured four-stage roadmap for the development of multiscale models of infectious disease dynamics by applying it to the development of a multiscale model of hookworm infection also established a fundamental result which can be generalized to confirm that the complexity of an infectious disease system is encapsulated with a level of organization spanning a microscale and a macroscale. We anticipate that the structured roadmap will articulate an integrated, more refined infectious disease control and elimination theory based on the development of realistic multiscale models of infectious disease dynamics. However, the replication–transmission relativity theory has limitations in that it only describes infectious disease dynamics from a pathogen-centred perspective. Therefore, this theory offers opportunities for improvements and extensions. Our current work is focused developing a unified multiscale modelling framework of infectious disease dynamics that integrates both a pathogen-centred perspective and a host-centred perspective to the multiscale dynamics of infectious disease systems.
Acknowledgments
All sources of funding of the study must be disclosed
Disclosure statement
No potential conflict of interest was reported by the author(s).
Funding
The authors acknowledge with thanks financial support from South Africa National ResearchFoundation (NRF) Grant No. IPRR (UID 132 608).
References
- W. Garira, A primer on multiscale modelling of infectious disease systems, Infect. Dis. Model. 3 (2018), pp. 176–191.
- W. Garira and B. Maregere, The transmission mechanism theory of disease dynamics: its aims, assumptions and limitations, Infect. Dis. Model. 8(1) (2023), pp. 122–144.
- W. Garira, The replication-transmission relativity theory for multiscale modelling of infectious disease systems, Sci. Rep. 9(1) (2019), pp. 1–17.
- A. Einstein, The foundation of the general theory of relativity, Ann. Phys. 354(7) (1916), pp. 769–822.
- J. Kevorkian and J.D. Cole, Perturbation Methods in Applied Mathematics, (Vol. 34), Springer Science & Business Media, 2013.
- W. Garira, A complete categorization of multiscale models of infectious disease systems, J. Biol. Dyn.11(1) (2017), pp. 378–435.
- R. Netshikweta and W. Garira, An embedded multiscale modelling to guide control and elimination of paratuberculosis in ruminants, Comput. Math. Methods. Med. 2021 (2021), pp. 1–25.
- R. Netshikweta and W. Garira, A nested multiscale model to study paratuberculosis in ruminants, Front. Appl. Math. Stat. 8 (2022), pp. 817060.
- W. Garira, D. Mathebula, and R. Netshikweta, A mathematical modelling framework for linked within-host and between-host dynamics for infections with free-living pathogens in the environment, Math. Biosci. 256 (2014), pp. 58–78.
- R.M. Anderson and R.M. May, Population dynamics of human helminth infections: control by chemotherapy, Nature 297(5867) (1982), pp. 557–563.
- R.M. Anderson and R.M. May, Infectious Diseases of Humans: Dynamics and Control, Oxford University Press, 1992.
- R. Netshikweta and W. Garira, A multiscale model for the world's first parasitic disease targeted for eradication: Guinea worm disease, Comput. Math. Methods. Med. 2017 (2017), pp. 1–29.
- A. Einstein, Lens-like action of a star by the deviation of light in the gravitational field, Science84(2188) (1936), pp. 506–507. doi:10.1126/science.84.2188.506
- A.O. Petters, H. Levine, and J. Wambsganss, Singularity Theory and Gravitational Lensing, Birkhäuser, Boston (MA), 2001.
- W. Garira and F. Chirove, A general method for multiscale modelling of vector-borne disease systems, Interface. Focus. 10(1) (2020), pp. 20190047.
- W. Garira and D. Mathebula, A coupled multiscale model to guide malaria control and elimination, J. Theor. Biol. 475 (2019), pp. 34–59.
- H.A. Lorentz, A. Einstein, H. Minkowski, H. Weyl, and A. Sommerfeld, The Principle of Relativity: a Collection of Original Memoirs on the Special and General Theory of Relativity, Courier Corporation, 1952.
- M. Bani-Yaghoub, R. Gautam, Z. Shuai, P. Van Den Driessche, and R. Ivanek, Reproduction numbers for infections with free-living pathogens growing in the environment, J. Biol. Dyn. 6(2) (2012), pp. 923–940.
- W. Garira, The research and development process for multiscale models of infectious disease systems, PLoS. Comput. Biol. 16(4) (2020), pp. e1007734.
- CDC, Parasites – Hookworm, 2014. https://www.cdc.gov/parasites/hookworm/biology.html.
- G. Smith and G.A. Schad, Ancylostoma duodenale and necator americanus: effect of temperature on egg development and mortality, Parasitology 99(1) (1989), pp. 127–132.
- K.A. Pawelek, S. Liu, and M.U. Lolla, Modeling the spread of hookworm disease and assessing chemotherapy programs: mathematical analysis and comparison with surveillance data, J. Biol. Syst.24(01) (2016), pp. 167–191.
- M. Bradley, S.K. Chandiwana, D.A.P. Bundy, and G.F. Medley, The epidemiology and population biology of necator americanus infection in a rural community in Zimbabwe, Trans. R. Soc. Trop. Med. Hyg. 86(1) (1992), pp. 73–76.
- H.M. Gilles and P.A.J. Ball, (Eds.). Hookworm infections, Human Parasitic Diseases, Vol. 4, Elsevier Science, pp. 38–39, 1991.
- R.E Mickens, Nonstandard Finite Difference Models of Differential Equations, World Scientific, Singapore, 1994.
- R.E. Mickens, Advances in the Applications of Nonstandard Finite Difference Schemes, World Scientific, Singapore, 2005.
- R.E. Mickens, Nonstandard finite difference schemes for differential equations, J. Differ. Equ. Appl.8(9) (2002), pp. 823–847.
- R.E. Mickens, Dynamic consistency: a fundamental principle for constructing nonstandard finite difference schemes for differential equations, J. Differ. Equ. Appl. 11(7) (2005), pp. 645–653.
- D.W.T. Crompton, How much human helminthiasis is there in the world?, J. Parasitol. 85(3) (1999), pp. 397–403.
- A. Loukas, S.L. Constant, and J.M. Bethony, Immunobiology of hookworm infection, FEMS Immunol. Med. Microbiol. 43(2) (2005), pp. 115–124.