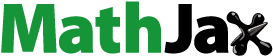
Abstract
In this paper, we investigate a reaction-diffusion model incorporating dynamic variables for nutrient, phytoplankton, and zooplankton. Moreover, we account for the impact of time delay in the growth of phytoplankton following nutrient uptake. Our theoretical analysis reveals that the time delay can trigger the emergence of persistent oscillations in the model via a Hopf bifurcation. We also analytically track the direction of Hopf bifurcation and the stability of the bifurcating periodic solutions. Our simulation results demonstrate stability switches occurring for the positive equilibrium with an increasing time lag. Furthermore, the model exhibits homogeneous periodic-2 and 3 solutions, as well as chaotic behaviour. These findings highlight that the presence of time delay in the phytoplankton growth can bring forth dynamical complexity to the nutrient-plankton system of aquatic habitats.
1. Introduction
Algal blooms occur in aquatic systems due to the massive growth of phytoplankton, leading to major water-quality problems and significant negative impacts on human health [Citation1–3]. Algal blooms are the consequences of the interplay of various hydrodynamics, chemical, and biological processes [Citation4]. Nutrient levels and zooplankton predation have been identified as key factors influencing the occurrence of algal blooms [Citation5,Citation6]. Vanni [Citation7] reported through experimental observations that even small changes in zooplankton size can have a tremendous impact on the phytoplankton community, while nutrient levels play a more significant role in the growth of phytoplankton population. Despite considerable research on phytoplankton growth dynamics, the specific process of phytoplankton bloom formation remains unclear. Previous studies have emphasized the need for in-depth investigations into nutrient-plankton interaction.
In recent years, extensive experimental studies and field observations have been conducted to study plankton dynamics. However, due to the complexity and nonlinearity of aquatic ecosystems, understanding the mechanisms behind phytoplankton growth solely through experimental and/or field observations remains challenging [Citation8]. Mathematical models serve as a powerful tool, providing quantitative insights into the complex dynamics of plankton communities, which have been alternatively employed by researchers [Citation9–11]. Notably, all the aforementioned researches have been conducted with an assumption that the growth process of phytoplankton is instantaneous. However, experimental evidence indicates that under high nutrient availability, a time lag occurs in the growth response of Isochrysis galbana [Citation12]. That is, the interaction between nutrient and phytoplankton will not be essentially instantaneous, suggesting that there is a delay in the growth of phytoplankton. Therefore, it is reasonable to consider the effect of time delay in the phytoplankton growth. Both experimental and field observations have demonstrated that changes in plankton population density usually exhibit oscillatory behaviour due to the existence of internal factors [Citation13,Citation14], supporting that the population density of phytoplankton in aquatic reservoirs is not constant but changes over time in realistic scenarios [Citation15]. In recent years, the effect of time delay has been widely studied in the dynamics of phytoplankton growth [Citation16–20]. Growing evidences suggest that plankton models with time delay exhibit rich and complex dynamical behaviours. Previous studies have demonstrated that the delay can destabilize a system via Hopf bifurcation and induce periodic solutions [Citation21–23], create stability switches [Citation24,Citation25], and even lead to chaotic dynamics [Citation26,Citation27].
Moreover, it is well established that nutrients and plankton can disperse in aquatic reservoirs due to currents and turbulent diffusion. Actually, both nutrient and plankton exhibit constant movement, leading to population densities that fluctuate spatially as well as temporally. Growing evidences suggest that plankton growth and distribution can be characterized by its spatial variation. Building on [Citation1,Citation28], a variety of ecological phenomena, including fundamental mechanisms of complex spatio-temporal plankton dynamics, can be modelled using appropriate partial differential equations. Segel and Jackson [Citation29] were the first to introduce spatial structure in population dynamics. Subsequently, numerous plankton models have been scrutinized by considering the effect of diffusion [Citation30–34]. Chakraborty et al. [Citation35] have investigated the spatial dynamics of a nutrient-phytoplankton model with the toxicity of phytoplankton. Their findings showed that in the presence of toxicity, the distributions of nutrient and phytoplankton in the aquatic ecosystem become inhomogeneous in space, and produce different patterns, such as stripes, spots, and mixtures, depending on the level of toxicity. They have also observed that the distributions of nutrient and phytoplankton show spatio-temporal oscillations for certain toxicity levels.
In recent years, numerous studies have been conducted on delay-induced dynamical behaviours in diffusive phytoplankton growth models [Citation36–38]. The findings of Dai et al. [Citation38] showed that the presence of delay not only triggers instability in a positive equilibrium but also promotes the emergence of patchiness through Hopf bifurcation. Moreover, the recent developments in dynamical model theory have considered delay-induced chaotic fluctuations of a dynamical model as highly desirable because fluctuations allow for easier control of such a system. Additionally, while various mechanisms, such as competition [Citation39], predation [Citation40], and resource and consumer digested delay [Citation41], contribute to chaotic dynamics, the phenomenon of delay-induced chaos in spatio-temporal plankton growth models has drawn considerable scientific attention. Gökce et al. [Citation42] demonstrated that delay is capable of switching the model from stable to unstable through Hopf bifurcation, resulting in spatio-temporal chaos. Despite the extensive discussion about the characteristics of delay-induced chaos and its presence in phytoplankton growth models, evidence for its existence in spatio-temporal phytoplankton growth models remains scarce.
It is worth noting that despite extensive investigations into the spatio-temporal dynamics of nutrient-plankton models, the influence of time delay involved in the growth of phytoplankton after the nutrient uptake on the spatial distributions of nutrient, phytoplankton, and zooplankton in aquatic ecosystems remains unclear. Therefore, in the present study, we aim to explore the role of such time delay in a diffusive model for the nutrient-plankton interactions in an aquatic reservoir, considering the release of toxic chemicals by certain phytoplankton species to mitigate predation pressure from zooplankton.
The remaining portion of this paper is organized as follows. In Section 2, we propose our model for the combined actions of delay and diffusion in a nutrient-plankton system of an aquatic ecosystem. In Section 3, we conduct mathematical analysis of the model's dynamics. Firstly, we obtain the equilibrium points of the temporal model in the absence of time delay. Then, we discuss the stability behaviour of the model in the presence of delay and diffusion. We also demonstrate the existence of Hopf bifurcation analytically and analyse the direction and stability of the bifurcating periodic solutions. In Section 4, we perform numerical simulations to illustrate the analytical findings and gain further insights into the dynamics of the delayed-diffusive nutrient-plankton model. Finally, in Section 5, we present the conclusions of this study.
2. The model formulation
One approach to the study of the dynamics of ecological community is via its food web and the coupling of interacting species with each other [Citation43]. We develop a mathematical model to explore the impact of time lag concealed in the growth of phytoplankton after the uptake of nutrient in a diffusive nutrient-plankton system of an aquatic ecosystem. The model encompasses three dynamic variables: nutrient concentration, phytoplankton biomass, and zooplankton biomass. The growth dynamics of the phytoplankton and zooplankton populations mainly depend on the availability of nutrient and phytoplankton, respectively. The aquatic system's nutrient concentration is determined by input and washout rates, as well as uptake by the phytoplankton population. For the phytoplankton population, its biomass is determined by nutrient uptake, grazing by zooplankton, and the mortality, either natural or due to nutrient scarcity. The predation of phytoplankton by zooplankton is described by a more appropriate functional form [Citation44]. Finally, the zooplankton biomass in the aquatic reservoir is influenced by the grazing of phytoplankton, toxin liberation by phytoplankton, and mortality.
Let ,
, and
denote the concentration of nutrient, biomass of phytoplankton, and the biomass of zooplankton, respectively, at any given time t>0 and position x. To build our model, we make the following ecological assumptions.
There is a constant input of nutrient into the aquatic system; the nutrients depleting naturally at a rate b and being taken up by phytoplankton through bilinear interaction.
The predation rate of zooplankton on phytoplankton follows the Holling type II functional form [Citation44],
; with c is denoting the maximum uptake rate of phytoplankton by zooplankton and h is representing the half-saturation constant.
The parameters m and k express the natural mortality rates of phytoplankton and zooplankton species, respectively. The phytoplankton species also experience mortality due to competition for available nutrient in the aquatic system; the parameter r signifies the strength of intraspecific competition among phytoplankton species owing to limited resources.
The biomass of phytoplankton is converted into the biomass of zooplankton at a rate d. Toxin-producing phytoplankton species release toxic chemicals at a rate ρ, leading to a decrement in the biomass of zooplankton at a rate
.
The growth response of phytoplankton following nutrient uptake is mediated by some time lag.
In the aquatic system, nutrient and plankton dispersal occur due to currents and turbulent diffusion.
The above assumptions yield the following delayed reaction-diffusion nutrient-plankton model: (1)
(1) In the above model, the parameter τ represents the time which is required by phytoplankton to absorb nutrient and reproduce. The constants
, and
denote the self-diffusion coefficients of nutrient, phytoplankton and zooplankton, respectively. Let
, and for
,
,
and
. All the parameters in model (Equation1
(1)
(1) ) are assumed to be positive, and their biological meanings are listed in Table .
Table 1. Biological meanings of parameters involved in model (Equation1(1)
(1) ).
3. Analytical results of model (1)
At first, we discuss about the existence of ecologically meaningful equilibria of model (Equation1(1)
(1) ) by ignoring the delay and diffusion factors. Then, we perform the linear stability and bifurcation analysis by taking the delay factor as a bifurcation parameter.
3.1. The existence of equilibria
Model (Equation1(1)
(1) ) does not have any trivial solution, but it has a non-trivial solution
, where
,
and
is the positive solution of the following quadratic equation:
The only one positive root of above equation is given by
Additionally, if the following conditions are satisfied:
then model (Equation1
(1)
(1) ) admits a unique positive equilibrium
whose components are given by
From an ecological point of view, the positive equilibrium
is very important as all the considered dynamical variables are present here. So, we will focus only on the dynamics of model (Equation1
(1)
(1) ) around this equilibrium point in the forthcoming sections.
3.2. Stability and bifurcation
Here, we will discuss about the stability behaviour of the positive equilibrium and the existence of Hopf bifurcation in model (Equation1
(1)
(1) ). As we have considered zero-flux boundary conditions, let
,
be the eigenvalues of the operator
on Ω with the homogeneous Neumann boundary condition. Following [Citation38], we represent model (Equation1
(1)
(1) ) as an abstract functional differential equation in the abstract space
. The linear form of model (Equation1
(1)
(1) ) around the equilibrium
is given by
(2)
(2) where
, and
Following [Citation49], we write the characteristic equation corresponding to Equation (Equation2
(2)
(2) ) as follows:
(3)
(3) Now, we define
(4)
(4) where
comprises of the eigenfunctions corresponding to the eigenvalues
. On substituting Equations (Equation4
(4)
(4) ) into (Equation3
(3)
(3) ), we get
for
Thus, an equivalent equation of Equation (Equation3(3)
(3) ) is obtained as follows:
(5)
(5) where
with
(H1) Let
. Then, we have the following result.
Theorem 3.1
If and (H1) holds, then the positive equilibrium
of model (Equation1
(1)
(1) ) without delay is locally asymptotically stable for any
.
Proof.
For , Equation (Equation5
(5)
(5) ) reduces to the following form:
(6)
(6) Since
, we have
in view of (H1). Obviously,
is not a solution of Equation (Equation5
(5)
(5) ). If
, then
and
for any
. Thus, Equation (Equation6
(6)
(6) ) has no positive roots, and hence Theorem 3.1.
Now, we consider the nutrient-plankton model (Equation1(1)
(1) ) with
. Following [Citation50], we assume that
is a root of Equation (Equation5
(5)
(5) ). Putting
into Equation (Equation5
(5)
(5) ), and separating real and imaginary parts, we get the following two equations:
(7)
(7) Squaring and adding both sides of the above equation, and simplifying, we get
(8)
(8) with
,
,
. Now, we present the following lemma.
Lemma 3.2
Let and (H1) holds. We have the following two cases.
(i) | If | ||||
(ii) | Let a |
Proof.
Let , then from Equation (Equation8
(8)
(8) ), we get
(9)
(9) Clearly,
in view of (H1). We denote by
. Evidently, for
, Equation (Equation9
(9)
(9) ) has no positive root. However, if there exists a
such that
, then equation
has the following two real roots:
For
,
, Equation (Equation9
(9)
(9) ) has two positive real roots if and only if
and
.
Now, we present the following lemma about the transversality condition that is required for the presence of Hopf bifurcation in model (Equation1(1)
(1) ).
Lemma 3.3
If the second condition of Lemma 3.2 holds, then
Proof.
Denoting the two positive roots of Equation (Equation9(9)
(9) ) by
and
, then the two positive roots of Equation (Equation8
(8)
(8) ) will be
and
. From Equation (Equation7
(7)
(7) ), we obtain the critical value of time delay as,
(10)
(10) Obviously, the right side of Equation (Equation10
(10)
(10) ) is an increasing function of s. Thus, we have
. Let
be a root of Equation (Equation5
(5)
(5) ) such that
(11)
(11) On differentiating both sides of Equation (Equation5
(5)
(5) ) with respect to τ, we obtain
(12)
(12) This yields,
(13)
(13) where
For convenience, we assume that
. Notably, the second point of Lemma 3.2 yields that
and
. Hence,
and
for
.
By Lemma 3.3, Hopf bifurcation occurs in model (Equation1(1)
(1) ) at the critical value
, i.e. the positive equilibrium
becomes unstable for
. We summarize these results in the following theorem.
Theorem 3.4
For model (Equation1(1)
(1) ), we find that
(i) | If Lemma 3.2 (i) holds, then the positive equilibrium | ||||
(ii) | If Lemma 3.2 (ii) holds, then there exists a non-negative integer n such that the positive equilibrium |
Now, we state the following results which are based on the centre manifold theorem and the normal form theory.
Theorem 3.5
The Hopf bifurcation in model (Equation1(1)
(1) ) is characterized by the signs of
and
as follows.
(i) | If | ||||
(ii) | If | ||||
(iii) | If |
The proof of this theorem is placed in Appendix.
4. Numerical simulations
In this section, we numerically investigate the influence of time delay on the dynamics of model (Equation1(1)
(1) ). For the numerical investigations of model (Equation1
(1)
(1) ), we adopt the following set of parameter values that is in accordance with the values mentioned in Table :
Unless specified otherwise, these parameter values are used consistently throughout the simulation section. Here, we choose
, and τ as control parameters in the nutrient-plankton model (Equation1
(1)
(1) ). Further, we choose the initial values for nutrient, phytoplankton, and zooplankton as 0.9, 0.9, and 22, respectively.
At first, we examine the dynamics of model (Equation1(1)
(1) ) in the absence of time delay (
). Figure illustrates the temporal and spatial variations in the biomass of phytoplankton and zooplankton populations. As depicted in the figure, the corresponding equilibrium point is asymptotically stable, implying that the plankton populations remain constant over time. Recall that the theoretical analysis indicates that Hopf bifurcation may occur when there is a time lag in the growth of phytoplankton after nutrient uptake. Moreover, stability switches may occur with gradual increments in the value of time delay. Numerically, we found that the natural mortality of phytoplankton species does not affect the model's dynamics much. Thus, in order to highlight the dynamical behaviours of stability switches, we choose m = 0.19, and present the number of stability switches experienced by the model by varying the parameters ρ and α (see Figure (a)). In the figure, region I represents the unstable domain of the positive equilibrium, while region X indicates stability if the positive equilibrium exists. In region II, the positive equilibrium loses its stability when τ exceeds the critical value
. Further, region III exhibits the emergence of stability switches. To provide a closer examination of the stability switches, an enlarged view of Figure (a) is presented in Figure (b).
Figure 1. Biomass distributions of (a) phytoplankton and (b) zooplankton populations in model (Equation1(1)
(1) ) over time and space for
.

Figure 2. (a) The number of stability switches of the positive equilibrium of model (Equation1(1)
(1) ) in the
plane. Stability switches occur once in region III, twice in region IV, three times in region V, four times in region VI, five times in region VII, six times in region VIII and seven times in region IX. (b) Enlarged diagram of regions V to X in (a).

Following Ref. [Citation38], we perform a sensitive analysis that is based on one-dimensional space solutions, Figure . The figures depict that model (Equation1(1)
(1) ) exhibits sensitivity to variations in the parameters α and ρ. Figure (a) illustrates that when the nutrient input rate is low (region I), model (Equation1
(1)
(1) ) does not exhibit any positive equilibrium point. However, as the value of α increases, model (Equation1
(1)
(1) ) experiences stability switches. Further, Figure (b) displays that when the toxin liberation rate is low, the biomass of phytoplankton oscillates. On further increasing the value of ρ, the dynamics of model (Equation1
(1)
(1) ) change from unstable to stable via Hopf bifurcation. However, once ρ enters region I, the positive equilibrium of model (Equation1
(1)
(1) ) does not exist. The results from Figure suggest that the nutrient input rate has the potential to generate as well as suppress the unstable coexistence of plankton populations, while a higher toxin liberation rate contributes to the stability of the system. However, very high toxin liberation rate can pose a threat to the entire ecosystem.
Figure 3. Sensitive analysis of model (Equation1(1)
(1) ) based on one-dimensional space solutions. The solid blue line represents the stable equilibrium, and the solid red line represents the maximal and minimal amplitudes of phytoplankton biomass. Figures show the effects of (a) nutrient input rate (α) on the model (Equation1
(1)
(1) ) with
and
, and (b) rate of toxin liberation (ρ) on the model (Equation1
(1)
(1) ) with
and
.

Further, we draw bifurcation diagrams of model (Equation1(1)
(1) ) in the ρ-τ plane for
(see Figure (a)) and
(see Figure (b)). These figures illustrate the existence of stability switches in the system before ρ enters the green zone (region I). Within the green zone, the positive equilibrium remains stable regardless of the value of time delay. However, if ρ falls within region II, the positive equilibrium does not exist for any value of τ. As our aim is to examine the effect of time lag on the dynamics of the nutrient-plankton system, we present the bifurcation diagram of model (Equation1
(1)
(1) ) with respect to the delay parameter τ, focusing solely on the biomass of the phytoplankton population (see Figure ). In Figure (a), we find that for
, the stability switch occurs once and periodic-2 solutions emerge as the value of time delay increases. The biomass distributions of phytoplankton over time and space at
, 10, and 18 are depicted in Figure , where the positive equilibrium is stable at
and 18 but unstable at
. Figure (b) shows that for
stability switches occur twice as the value of time delay gradually increases, indicating the emergence of transitions from stable to unstable via Hopf bifurcation. The biomass distributions at
3, 18, and 42 (stable) and
10, 36, and 50 (unstable) are plotted in Figure . Overall, our findings indicate that the nonlinear analysis is in agreement with the results predicted by the linear analysis (see Figures and ). The results indicate that delay in the growth of phytoplankton is the only reason for the rise in the instability around the positive equilibrium. Moreover, Figures and suggest that delay can generate as well as suppress oscillations around the coexistence equilibrium point.
Figure 4. Bifurcation diagrams of model (Equation1(1)
(1) ) with respect to ρ and τ for (a)
and (b)
. In the figures, the solid, dashed, dash-dot, and dotted curves represent the critical values of τ for j = 0, 1, 2 and 3, respectively.

Figure 5. Bifurcation diagrams of model (Equation1(1)
(1) ) with respect to τ for (a)
and
, and (b)
and
. In the figure, the solid blue line and the dotted red line represent the stable positive equilibrium and the maximum biomass of phytoplankton, respectively.

Figure 6. Biomass distribution of phytoplankton population in model (Equation1(1)
(1) ) over time and space for
,
, and different values of τ: (a)
, (b)
and (c)
.

Figure 7. Biomass distribution of phytoplankton population in model (Equation1(1)
(1) ) over time and space for
,
, and different values of τ: (a)
, (b)
, (c)
, (d)
, (e)
and (f)
.

Finally, we present a bifurcation diagram for a wide range of the delay parameter τ, focusing on the biomass of the phytoplankton population (see Figure (a)). The figure clearly shows that model (Equation1(1)
(1) ) exhibits periodic-1, 2, and 3 solutions as the value of time delay gradually increases. It can be noted in the figure that for a very large value of time delay, the model showcases chaotic dynamics. The periodic-1, 2, and 3 solutions at
, 30, and 56 are marked with green, blue, and yellow solid diamonds, respectively. A vertical black dashed line is drawn at
, indicating the entrance into the chaotic regime. The biomass distributions of the phytoplankton population over time and space at these critical values of time delay are presented in Figure (b–e).
Figure 8. (a) Bifurcation diagram of model (Equation1(1)
(1) ) with respect to τ for
and
. In the figure, the green, blue and yellow solid diamonds respectively represent the periodic−1, 2 and 3 solutions at
, 30 and 56; the black dashed line denotes chaotic solution at
. Biomass distributions of phytoplankton in model (Equation1
(1)
(1) ) over time and space at (b)
, (c)
, (d)
and (e)
.

5. Conclusions
The phenomenon of planktonic blooms has gathered significant attention from both ecologists and mathematicians in the recent decades [Citation2]. Mathematical models have been widely used to investigate this phenomenon. Huppert et al. [Citation51] investigated a simple mathematical model and found that high nutrient concentrations can trigger planktonic blooms in the aquatic reservoirs. Further, Steele and Henderson [Citation52] explored the role of predation in the plankton models. Additionally, the effect of time delay is inevitable in the aquatic environments. Researchers have also recognized the significance of nutrient and plankton diffusion on the spatial distribution of phytoplankton in the aquatic ecosystems [Citation53]. However, the dynamics induced by delay, such as oscillation, chaotic behaviour, and stability switches, in the phytoplankton growth models with diffusion require further exploration.
Here, we have investigated a nutrient-plankton model to examine the effects of time delay on the growth of phytoplankton and the interplay between nutrient and plankton populations. We also considered the effect of diffusion in the model to incorporate the spatial movements of nutrient and plankton populations in the aquatic ecosystem. Our theoretical results for the model without delay and diffusion showed that the positive equilibrium is locally asymptotically stable under some specific conditions on the parameters. In such a situation, the nutrient and plankton populations persist in the ecosystem. However, when the time delay involved in the growth of phytoplankton exceeds critical values, the positive equilibrium loses its stability, leading to the emergence of periodic solutions and oscillatory dynamics in the nutrient-plankton system. This indicates that the biomass of plankton populations cannot maintain a steady level. That is to say, time delay plays a significant role in inducing oscillations in the nutrient-plankton model. We also discussed the direction of Hopf bifurcation around the positive equilibrium and the stability of the bifurcating periodic solutions by employing the centre manifold theorem and the normal form theory. While many studies have demonstrated unstable coexistence of species in the ecosystems when the time delay exceeds a critical value [Citation46,Citation54,Citation55], our simulation results reveal that time delay can generate as well as suppress oscillations in the system. This phenomenon is known as stability switches in the plankton system [Citation41]. Obviously, these findings contribute to the control of plankton biomass in the aquatic reservoirs when the model exhibits stable coexistence of nutrient and plankton populations.
Furthermore, we explored the effects of time delay on the model's dynamics through bifurcation diagrams. Figures and illustrate the number of stability switches that occurred around the positive equilibrium. Unlike other nutrient-plankton models that only demonstrate a destabilizing role of time delay, our model exhibits both destabilizing as well as stabilizing effects of delay on the system's dynamics. Specifically, our model shows the occurrence of multiple stability switches around the positive equilibrium as the delay involved in the growth of phytoplankton increases. Figures and display the evolution of phytoplankton population concerning different time delay values. The results suggest that the time delay possesses the capability to generate as well as suppress oscillations in the plankton biomass, indicating the crucial role of time delay plays in the stability of nutrient-plankton system.
We observed that the system generates periodic-2 and 3 solutions, and ultimately becomes chaotic if there is a large time delay in the growth of phytoplankton following nutrient uptake. Thus, delay can be considered as a primary factor contributing to the chaotic behaviour of the plankton system. It is worth noting that the emergence of chaos may imply the unpredictability of biomass distributions of plankton populations over time and space. Therefore, the investigated nutrient-plankton model cannot accurately predict bloom events, but it contributes to enhancing our understanding of the influences of time delay and diffusion on the interplay between nutrient and plankton populations in the aquatic reservoirs. Our research provides important empirical evidence and specific insights into delay-induced oscillations within the context of the investigated nutrient-plankton model. Additionally, our findings offer valuable insights into the effect of delay on the emergence of chaos in the nutrient-plankton system.
Acknowledgments
All the authors thank the associate editor and anonymous reviewers for their valuable comments, which contributed to the improvement in the presentation of the paper. Qing Guo: Conceptualization, Methodology, Software, Writing – Original Draft. Lijun Wang: Writing – Review and Editing. He Liu: Writing – Review and Editing. Yi Wang: Writing – Review and Editing. Jianbing Li: Supervision, Resources, Writing – Review and Editing. Pankaj Kumar Tiwari: Writing – Review and Editing. Min Zhao: Writing – Review and Editing, Supervision, Resources, Funding acquisition. Chuanjun Dai: Software, Resources, Writing – Review and Editing.
Disclosure statement
The author declares that there are no conflicts of interest.
Additional information
Funding
References
- Medvinsky AB, Petrovskii SV, Tikhonova IA, et al. Spatiotemporal complexity of plankton and fish dynamics. SIAM Rev. 2002;44:311–370. doi: 10.1137/S0036144502404442
- Mukhopadhyay B, Bhattacharyya R. Modelling phytoplankton allelopathy in a nutrient-plankton model with spatial heterogeneity. Ecol Model. 2006;198:163–173. doi: 10.1016/j.ecolmodel.2006.04.005
- Hallegraeff GM. A review of harmful algal blooms and their apparent global increase. Phycologia. 1993;32:79–99. doi: 10.2216/i0031-8884-32-2-79.1
- Chen QW, Mynett AE. Modelling algal blooms in the Dutch coastal waters by integrated numerical and fuzzy cellular automata approaches. Ecol Model. 2006;199:73–81. doi: 10.1016/j.ecolmodel.2006.06.014
- Hecky RE, Kilham P. Nutrient limitation of phytoplankton in freshwater and marine environments: a review of recent evidence on the effects of enrichment. Limnol Oceanogr. 1988;33:796–822.
- Dacey JWH, Wakeham SG. Oceanic dimethylsulfide: production during zooplankton grazing on phytoplankton. Science. 1986;233:1314–1316. doi: 10.1126/science.233.4770.1314
- Vanni MJ. Effects of nutrients and zooplankton size on the structure of a phytoplankton community. Ecology. 1987;68:624–635. doi: 10.2307/1938467
- Dai CJ, Yu HG, Guo Q, et al. Dynamics induced by delay in a nutrient-phytoplankton model with multiple delays. Complexity. 2019;2019:3879626.
- Edwards AE, Brindley J. Zooplankton mortality and the dynamical behaviour of plankton population models. Bull Math Biol. 1999;61:303–339. doi: 10.1006/bulm.1998.0082
- Mandal A, Tiwari PK, Pal S. A nonautonomous model for the effects of refuge and additional food on the dynamics of phytoplankton-zooplankton system. Ecol Complex. 2021;46:100927. doi: 10.1016/j.ecocom.2021.100927
- Guo Q, Wang Y, Dai CJ, et al. Dynamics of a stochastic nutrient-plankton model with regime switching. Ecol Model. 2023;477:110249. doi: 10.1016/j.ecolmodel.2022.110249
- Caperon J. Time lag in population growth response of Isochrysis galbana to a variable nitrate environment. Ecology. 1969;50:188–192. doi: 10.2307/1934845
- Fussmann GF, Ellner SP, Shertzer KW, et al. Crossing the Hopf bifurcation in a live predator-prey system. Science. 2000;290:1358–1360. doi: 10.1126/science.290.5495.1358
- Huisman J, Thi NNP, Karl DM, et al. Reduced mixing generates oscillations and chaos in the oceanic deep chlorophyll maximum. Nature. 2006;439:322–325. doi: 10.1038/nature04245
- Sherratt JA, Smith MJ. Periodic travelling waves in cyclic populations: field studies and reaction-diffusion models. J R Soc Interface. 2008;5:483–505. doi: 10.1098/rsif.2007.1327
- Yuan Y. A coupled plankton system with instantaneous and delayed predation. J Biol Dyn. 2012;6:148–165. doi: 10.1080/17513758.2010.544409
- Sharma A, Sharma AK, Agnihotri K. The dynamic of plankton-nutrient interaction with delay. Appl Math Comput. 2014;231:503–515. doi: 10.1016/j.amc.2014.01.042
- Chen MX, Wu RC, Liu B, et al. Hopf-Hopf bifurcation in the delayed nutrient-microorganism model. Appl Math Model. 2020;86:460–483. doi: 10.1016/j.apm.2020.05.024
- Agnihotri K, Kaur H. The dynamics of viral infection in toxin producing phytoplankton and zooplankton system with time delay. Chaos Solit Fract. 2019;118:122–133. doi: 10.1016/j.chaos.2018.11.018
- Das K, Ray S. Effect of delay on nutrient cycling in phytoplankton-zooplankton interactions in estuarine system. Ecol Model. 2008;215:69–76. doi: 10.1016/j.ecolmodel.2008.02.019
- Chen SS, Shi JP, Wei JJ. Time delay-induced instabilities and Hopf bifurcations in general reaction-diffusion systems. J Nonlinear Sci. 2013;23:1–38. doi: 10.1007/s00332-012-9138-1
- Song YL, Wei JJ. Local Hopf bifurcation and global periodic solutions in a delayed predator-prey system. J Math Anal Appl. 2005;301:1–21. doi: 10.1016/j.jmaa.2004.06.056
- Bentounsi M, Agmour I, Achtaich N, et al. The Hopf bifurcation and stability of delayed predator-prey system. Comput Appl Math. 2018;37:5702–5714. doi: 10.1007/s40314-018-0658-7
- Thakur NK, Ojha A, Tiwari PK, et al. An investigation of delay induced stability transition in nutrient-plankton systems. Chaos Solit Fract. 2021;142:110474. doi: 10.1016/j.chaos.2020.110474
- Wang BB, Zhao M, Dai CJ, et al. Dynamics analysis of a nutrient-plankton model with a time delay. Discrete Dyn Nat Soc. 2016;2016:9797624.
- Shu HY, Hu X, Wang L, et al. Delay induced stability switch, multitype bistability and chaos in an intraguild predation model. J Math Biol. 2015;71:1269–1298. doi: 10.1007/s00285-015-0857-4
- Adak D, Bairagi N, Hakl R. Chaos in delay-induced Leslie-Gower prey-predator-parasite model and its control through prey harvesting. Nonlinear Anal Real World Appl. 2020;51:102998. doi: 10.1016/j.nonrwa.2019.102998
- Holmes EE, Lewis MA, Banks JE, et al. Partial differential equations in ecology: spatial interactions and population dynamics. Ecology. 1994;75:17–29. doi: 10.2307/1939378
- Segel LA, Jackson JL. Dissipative structure: an explanation and an ecological example. J Theor Biol. 1972;37:545–559. doi: 10.1016/0022-5193(72)90090-2
- Zhao QY, Liu ST, Niu XL. Dynamic behavior analysis of a diffusive plankton model with defensive and offensive effects. Chaos Solit Fract. 2019;129:94–102. doi: 10.1016/j.chaos.2019.08.015
- Han RJ, Dai BX. Spatiotemporal pattern formation and selection induced by nonlinear cross-diffusion in a toxic-phytoplankton-zooplankton model with allee effect. Nonlinear Anal Real World Appl. 2019;45:822–853. doi: 10.1016/j.nonrwa.2018.05.018
- Upadhyay RK, Volpert V, Thakur NK. Propagation of turing patterns in a plankton model. J Biol Dyn. 2012;6:524–538. doi: 10.1080/17513758.2012.655327
- Dai CJ, Zhao M, Yu HG, et al. Delay-induced instability in a nutrient-phytoplankton system with flow. Phys Rev E. 2015;91:032929. doi: 10.1103/PhysRevE.91.032929
- Agmour I, Baba N, Bentounsi M, et al. Mathematical study and optimal control of bioeconomic model concerning harmful dinoflagellate phytoplankton. Comput Appl Math. 2021;40:129. doi: 10.1007/s40314-021-01509-3
- Chakraborty S, Tiwari PK, Misra AK, et al. Spatial dynamics of a nutrient–phytoplankton system with toxic effect on phytoplankton. Math Biosci. 2015;264:94–100. doi: 10.1016/j.mbs.2015.03.010
- Raw SN, Sahu SR. Strong stability with impact of maturation delay and diffusion on a toxin producing phytoplankton–zooplankton model. Math Comput Simulat. 2023;210:547–570. doi: 10.1016/j.matcom.2023.03.023
- Liang Y, Jia Y. Stability and Hopf bifurcation of a diffusive plankton model with time-delay and mixed nonlinear functional responses. Chaos Solit Fract. 2022;163:112533. doi: 10.1016/j.chaos.2022.112533
- Dai CJ, Zhao M, Yu HG. Dynamics induced by delay in a nutrient-phytoplankton model with diffusion. Ecol Complex. 2016;26:29–36. doi: 10.1016/j.ecocom.2016.03.001
- Huisman J, Weissing FJ. Biological conditions for oscillations and chaos generated by multispecies competition. Ecology. 2001;82:2682–2695. doi: 10.1890/0012-9658(2001)082[2682:BCFOAC]2.0.CO;2
- Moroz IM, Cropp R, Norbury J. Chaos in plankton models: foraging strategy and seasonal forcing. Ecol Model. 2016;332:103–111. doi: 10.1016/j.ecolmodel.2016.04.011
- Song ZG, Zhen B, Xu J. Species coexistence and chaotic behavior induced by multiple delays in a food chain system. Ecol Complex. 2014;19:9–17. doi: 10.1016/j.ecocom.2014.01.004
- Gökçe A, Yazar S, Sekerci Y. Stability of spatial patterns in a diffusive oxygen–plankton model with time lag effect. Math Comput Simulat. 2022;194:109–123. doi: 10.1016/j.matcom.2021.11.006
- Hastings A, Powell T. Chaos in three-species food chain. Ecology. 1991;72:896–903. doi: 10.2307/1940591
- Holling CS. Some characteristics of simple types of predation and parasitism1. Can Entomol. 1959;91:385–398. doi: 10.4039/Ent91385-7
- Ruan SG. Persistence and coexistence in zooplankton-phytoplankton-nutrient models with instantaneous nutrient recycling. J Math Biol. 1993;31:633–654. doi: 10.1007/BF00161202
- Rehim M, Zhang ZZ, Muhammadhaji A. Mathematical analysis of a nutrient-plankton system with delay. SpringerPlus. 2016;5:1–22. doi: 10.1186/s40064-016-2435-7
- Garcés E, Vila M, Masó M, et al. Taxon-specific analysis of growth and mortality rates of harmful dinoflagellates during bloom conditions. Mar Ecol Prog Ser. 2005;301:67–79. doi: 10.3354/meps301067
- Javidi M, Ahmad B. Dynamic analysis of time fractional order phytoplankton–toxic phytoplankton–zooplankton system. Ecol Model. 2015;318:8–18. doi: 10.1016/j.ecolmodel.2015.06.016
- Wu JH. Symmetric functional differential equations and neural networks with memory. Trans Am Math Soc. 1998;350:4799–4838. doi: 10.1090/tran/1998-350-12
- Ruan SG, Wei JJ. On the zeros of transcendental function with applications to stability of delay differential equations with two delays. Dyn Contin Discrete Impuls Syst A: Math Anal. 2003;10:863–874.
- Huppert A, Blasius B, Stone L. A model of phytoplankton blooms. Am Nat. 2002;159:156–171. doi: 10.1086/324789
- Steele JH, Henderson EW. The role of predation in plankton models. J Plankton Res. 1992;14:157–172. doi: 10.1093/plankt/14.1.157
- Tian CR. Delay-driven spatial patterns in a plankton allelopathic system. Chaos. 2012;22:013129. doi: 10.1063/1.3692963
- Li L, Liu Z. Global stability and Hopf bifurcation of a plankton model with time delay. Nonlinear Anal Theory Methods Appl. 2010;72:1737–1745. doi: 10.1016/j.na.2009.09.014
- Meng XY, Li J. Stability and Hopf bifurcation analysis of a delayed phytoplankton-zooplankton model with allee effect and linear harvesting. Math Biosci Eng. 2020;17:1973–2002. doi: 10.3934/mbe.2020105
- Hassard BD, Kazarinoff ND, Wan YH. Theory and applications of Hopf bifurcation. New York: Cambridge University Press; 1981.
Appendix. The proof of Theorem 3.5
We will track the direction of Hopf bifurcation around the positive equilibrium and the stability of the bifurcating periodic solutions by using the normal formal theory and the centre manifold theorem of differential equations [Citation56]. Let and
. In space
, Equation (Equation2
(2)
(2) ) can be rewritten as
(A1)
(A1) where
,
and
with
for
. Hence,
is the bifurcation point of Equation (EquationA1
(A1)
(A1) ).
On linearizing Equation (EquationA1(A1)
(A1) ) about
, we get
(A2)
(A2) Note that Equation (Equation5
(5)
(5) ) has a pair of purely imaginary roots
when
and other roots have negative real parts. The solution operator of Equation (EquationA2
(A2)
(A2) ) is a semigroup whose infinitesimal generator is given by
Hence, Equation (EquationA2
(A2)
(A2) ) can be rewritten in the abstract form as,
(A3)
(A3) where
Define
, where
Define
where
.
We also define
Further, we define
Thus, we have
(A4)
(A4) where
For
, we define a bilinear form
on
as follows:
where
,
,
,
. Note that
for
. Thus, we have
where
is the bilinear form defined on
, and satisfies the following equation:
Now, we define an adjoint operator
as follows:
Let
and
be the eigenfunctions of the operators A and
corresponding to the eigenvalues
and
, respectively. Thus, one can obtain
By a direct calculation, we get
We choose an M such that
. Thus, we have
This yields the decomposition
by
with
One can rewrite the solution of Equation (EquationA1
(A1)
(A1) ) as follows:
(A5)
(A5) where
. On the centre manifold
, we have
(A6)
(A6) At
satisfies the following relation:
(A7)
(A7) Next, we define
For our convenience, we rewrite Equation (EquationA7
(A7)
(A7) ) in the following form:
(A8)
(A8) where
(A9)
(A9) From the above, we obtain
Now, we will compute
and
. From Equation (EquationA7
(A7)
(A7) ), we have
(A10)
(A10) where
(A11)
(A11) Further, we obtain
After differentiating both sides of Equation (EquationA6
(A6)
(A6) ) and comparing the coefficients with Equation (EquationA10
(A10)
(A10) ), we get
(A12)
(A12) where
. By the definition of
and Equation (EquationA12
(A12)
(A12) ), we have
(A13)
(A13) Denote by
and
. Obviously,
and
satisfies the following relations:
(A14)
(A14) where
Thus, we have
From these, one can compute the value of
. Finally, we will compute the values of following quantities:
The signs of
,
and
will determine the properties of Hopf bifurcation. Particularly,
signifies the direction of the Hopf bifurcation; for
, the Hopf bifurcation is supercritical (subcritical). Further,
determines the stability of the bifurcation periodic solutions; the solutions are stable (unstable) when
. Finally,
characterizes the period of the bifurcating periodic solutions; the period increases (decreases) when
.