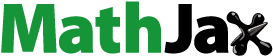
Abstract
In this paper, a compartmental model on the co-infection of pneumonia and HIV/AIDS with optimal control strategies was formulated using the system of ordinary differential equations. Using qualitative methods, we have analysed the mono-infection and HIV/AIDS and pneumonia co-infection models. We have computed effective reproduction numbers by applying the next-generation matrix method, applying Castillo Chavez criteria the models disease-free equilibrium points global stabilities were shown, while we have used the Centre manifold criteria to determine that the pneumonia infection and pneumonia and HIV/AIDS co-infection exhibit the phenomenon of backward bifurcation whenever the corresponding effective reproduction number is less than unity. We carried out the numerical simulations to investigate the behaviour of the co-infection model solutions. Furthermore, we have investigated various optimal control strategies to predict the best control strategy to minimize and possibly to eradicate the HIV/AIDS and pneumonia co-infection from the community.
1. Introduction
Infectious diseases caused by tiny microorganisms such as viruses, bacteria, fungi and parasites are clinically verified illnesses and have been reported as the leading cause of death in human beings throughout the world [Citation1,Citation2].
AIDS caused by human immunodeficiency virus (HIV) has been among the leading life-affecting disease which is expanding throughout nations in the world since 1981 [Citation3–5]. It remains a significant world health issue that impacts almost 70 million people worldwide and has been a significant cause of morbidity and mortality [Citation6]. HIV is transmitted through sexual intercourse, needle sharing, and direct contact with blood or other body fluids containing the virus and from mother to child during childbirth [Citation3,Citation7].
Pneumonia caused by various pathogenic microbial agents such as virus, bacteria, fungi and parasites is a major respiratory infectious disease identified as an inflammatory condition of the lungs [Citation8–10]. Among the pathogenic microbial agents which have potential in causing pneumonia infection, bacteria especially Streptococcus pneumoniae has been reported as the leading cause [Citation8–11]. The microbial agents enter the lungs, rapidly multiply its number, settle in the air passage called alveoli of the human being lung, the lung will be filled with fluid and pus and makes breathing difficult [Citation8,Citation10]. Pneumonia is commonly a highly transmitted disease and major causes of morbidity and mortality in both children and adults throughout nations in the world [Citation1,Citation11,Citation12].
Many scholars in different nations have proposed and analysed mathematical model of the phenomenon of the transmission and control of infectious diseases. Mathematical models of infectious diseases are used for examining the spreading dynamics and also for making predictions of quantitative measures of different controlling strategies and their effectiveness as we reviewed in the literature [Citation1–11,Citation13–40]. Even though, mathematical epidemiologists did not give attention like the common HIV/AIDS and TB co-infection and other co-infections, pneumonia is the most opportunistic infection for HIV/AIDS infected individuals; the co-infection of HIV/AIDS and pneumonia in one host is a common phenomenon. From few essential mathematical epidemiological research studies that have been studied by scholars on the transmission dynamics of HIV/AIDS and pneumonia co-infection, the existence of pneumonia and HIV co-infection has been delineating in the literature [Citation20,Citation21].
In this study, we have examined research papers that have been done on the transmission dynamics of different infectious diseases. Huo and Chen [Citation16] formulated a stage structure HIV/AIDS deterministic model to study the transmission of HIV/AIDS with treatment. The model analysis showed that ART at the asymptomatic initial stages of the HIV incidence or before-AIDS stage is the most effective to decrease its spreading rate. Omondi et al. [Citation17] analysed a sex-structured community infection model and discussed male and female HIV infection trends with heterosexual activities. The analysis of the study deduced that ART treatment has a considerable impact on the HIV/AIDS spreading rate. Saha et al. [Citation32] formulated and analysed a mathematical mode of HIV/AIDS with optimal control theory to prevent the transmission through pre-exposure prevention (PrEP) and limited treatment strategies. From the finding of the study, one can observe that the combined strategies effect is more powerful and effective than single strategies. Samanta et al. [Citation33] formulated and analysed a non-autonomous HIV/AIDS epidemic model with distributed time delay. Teklu [Citation27] constructed a mathematical model on COVID-19 in the presence of prevention and control strategies. The result emphasizes that the spreading of COVID-19 in the considered community will be minimized through applications of the intervention strategies presented on the study. Ahmed et al. [Citation22] constructed a mathematical model of HIV and COVID-19 co-infection to globally assess the COVID-19 pandemic situation in different countries affected by both diseases, such as South Africa, Brazil and many other countries. Teklu and Mekonnen [Citation20] proposed and analysed HIV/AIDS and pneumonia co-infection model with treatment at each infection stage and their model analysis verified that applying treatment mechanisms for both the single infections and co-infection models is the most effective strategy to minimize the co-infection transmission dynamics. Teklu and Rao [Citation21] constructed and examined a deterministic mathematical model on the co-existence of HIV/AIDS and pneumonia with control measures such as pneumonia vaccination and treatments of pneumonia and HIV/AIDS infections. Even though researchers stated in the literature [Citation20] and [Citation21] invested much effort in studying HIV/AIDS and pneumonia co-infection, they did not considered pneumonia protection, pneumonia vaccination, pneumonia treatment, HIV protection by using condom, and HIV treatment as prevention and control strategies simultaneously in their proposed co-infection model formulation and analysis. Moreover, based on the findings and limitations in the studies stated in [Citation20] and [Citation21], we are motivated and inspired to undertake this study and to fulfil the gap.
The remaining part of this study has structured in the sequence: the model is formulated in Section 2 and is analysed in Section 3, optimal control analysis and numerical simulation, and discussion and conclusion of the study are carried out in Sections 4, 5 and 6 respectively.
2. Model descriptions and formulation
In this study, we partitioned the total human population at a given time t denoted by into 11 distinct classes depending on their infection status: susceptible individuals to both pneumonia and HIV
, pneumonia protected individuals (by good hygiene, quitting smoking, and getting regular physical activity and eating healthy etc.)
, HIV protected individuals by using condom
, pneumonia vaccinated individuals
, pneumonia mono-infected individuals
, HIV unaware mono-infected individuals
HIV aware mono-infected individuals
, HIV unaware and pneumonia co-infected individuals
, HIV aware and pneumonia co-infected individuals
, individuals recovered from pneumonia
and HIV aware treated individuals
so that
. Since HIV/AIDS is a chronic infectious disease the susceptible individuals acquires HIV infection at the standard incidence rate given by
(1)
(1) where
are the modification parameters that increase infectivity and
is the HIV transmission rate.
Since pneumonia is an acute infectious disease, the susceptible individuals acquire pneumonia infection at the mass action incidence rate given by
(2)
(2) where
are the modification parameters that increase infectivity and
is the pneumonia transmission rate.
Assume: ,
,
and
where
are portions of the recruited individuals who are entering to susceptible class, pneumonia protected class, HIV protected class and pneumonia vaccinated class respectively. The susceptible class is increased by individuals from pneumonia vaccinated class in which those individuals who are vaccinated against pneumonia but did not respond to vaccination with waning rate of
and from pneumonia recovery class who lose their temporary immunity by the rate
, pneumonia vaccine may not be 100% efficient, so vaccinated individuals also have a chance of being infected with portion
of pneumonia serotype not covered by pneumonia vaccine where
, the human population distribution is homogeneous in each class, individuals who exactly apply protection measures such as good hygiene, quitting smoking, and getting regular physical activity and eating healthy and so on have fully protected from pneumonia infection, HIV-treated individuals do not transmit infection to others, individuals in each class are subject to natural death rate
, population of human being is variable, there is no dual-infection transmission simultaneously and no vertical HIV transmission.
In this section using parameters described in Table , model variables definitions given in Table , and the given assumptions the schematic diagram of the co-infection is given by Figure .
Figure 1. Schematic diagram of the pneumonia and HIV/AIDS co-infection transmission dynamics where and
are given in (1) and (2) respectively.

Table 1. Model parameter descriptions.
Table 2. Biological definitions of model variables.
Using Figure , the system of differential equations of the HIV/AIDS and pneumonia co-infection is given by
(3)
(3) with the corresponding initial conditions
(4)
(4) The sum of all the differential equations in (3) is
(5)
(5)
2.1. Two qualitative properties of the co-infection model
The model (3) is both biologically and mathematically meaningful if and only if all the model solutions are non-negative and bounded in the invariant region
(6)
(6)
Theorem 2.1 (Non-negativity of the model solutions)
Let us given the initial data in Equation (4), then the solutions ,
,
,
and
of the COVID-19 and HIV/AIDS co-infection model (3) are nonnegative for all time
.
Proof:
Appendix A
Theorem 2.2 (The Invariant region)
All the feasible non-negative solutions of the co-infection model (3) are bounded in the region (6).
Proof:
Appendix B
Note: Since the model (3) solutions are both non-negative and bounded in the region (6) the pneumonia and HIV/AIDS co-infection model (3) is both mathematically and biologically meaningful then we can consider the two mono-infection models, namely, HIV mono-infection and pneumonia mono-infection models. This is fundamental for the analysis of the full pneumonia and HIV/AIDS co-infection model.
3. Qualitative analysis of the models
Before analysing the pneumonia and HIV/AIDS co-infection model given in Equation (3), it is very crucial to gain some basic backgrounds about the pneumonia and HIV/AIDS mono-infection models.
3.1. Analysis of HIV/AIDS mono-infection model
In this section, we assume there is no pneumonia infection in the community, i.e.
in Equation (3), then the HIV/AIDS mono-infection model is given by
(7)
(7) where the total population is
the HIV mono-infection model force of infection is given by
, and initial conditions
,
,
,
and
. In a similar manner of the full co-infection model (3) in the region
, it is sufficient to consider the dynamics of the model (7) in
as biologically and mathematically well-posed.
3.1.1. Local stability of the model disease-free equilibrium point (DFE)
The disease-free equilibrium point of the HIV mono-infection system (7) is obtained by making its right-hand side as zero and setting the infected classes and treatment class to zero as we do have
,
. Hence the disease-free equilibrium point is given by
.
The local stability of the HIV mono-infection model (7) DFE is examined by its basic reproduction number denoted by , which is calculated by using the next-generation operator method stated by Van den Driesch and Warmouth [Citation24]. Applying the method given in [Citation24], the transmission matrix
and the transition matrix
, i.e. for the new infection and the remaining transfer respectively, are given by
and
Then we have calculated that
and
Then the effective reproduction number of the HIV mono-infection model (7) is defined as the largest eigenvalue in magnitude of the next generation matrix,
given by
Here
is defined as the total average number of secondary HIV unaware and HIV aware infection cases acquired from a typical HIV unaware or HIV aware individual during his/her effective infectious period in a susceptible population. The threshold result
is the effective reproduction number for HIV mono-infection.
Theorem 3.1:
The DFE point of the HIV mono-infection model given in Equation (7) is locally asymptotically stable (LAS) if , and it is unstable if
.
Proof:
The local stability of the disease-free equilibrium point of HIV mono-infection model (7) is studied by applying the Routh–Hurwitz stability criteria.
The Jacobian matrix of the HIV mono-infection model given in Equation (7) at the disease-free equilibrium point is given by
Then the corresponding characteristic equation of the Jacobian matrix
is given by
Then we have determined
, where
and
Then we have got
or
or
or
(8)
(8) For Equation (8), we can apply Routh–Hurwitz stability criteria stated in [Citation29] to determine that both eigenvalues are negative if
and hence we can conclude that since all the eigenvalues are negative whenever
the disease-free equilibrium point of the model (7) is locally asymptotically stable whenever
From Theorem 3.1, biologically one can conclude that HIV infection dies out from the population (whenever
) if the initial size of the sub-populations of the HIV mono-infection model given in Equation (7) is in the basin of attraction of the disease-free equilibrium point
.
3.1.2. Existence of endemic equilibrium point(s)
Let be an arbitrary endemic equilibrium point of the HIV mono-infection model (7) which can be determined by making the right-hand side of Equation (7) as zero. Then after a number of steps of computations, we have determined that
(9)
(9) where
,
, and
.
Now substitute and
which are given in Equation (9) into the HIV/AIDS force of infection
, then we have determined the result
,
(10)
(10) Then the non-zero solution of (10) is
, where
+
+
,
,
+
.
Therefore, the required non-zero solution (force of infection) is obtained as
Then we do have
whenever
. Thus the HIV/AIDS mono-infection model (7) has a unique positive endemic equilibrium point if and only if
.
Theorem 3.2:
The HIV/AIDS mono-infection model given in (7) has a unique endemic equilibrium point if and only if .
3.1.3. Global stability of disease-free equilibrium point
We now list two conditions which are sufficient to guarantee the global stability of the HIV only disease-free equilibrium point. Applying Castillo-Chavez et al. [Citation25], let us rewrite the dynamical system (7) as
,
, where
and
, with
denoting the total number of uninfected individuals and
denoting the total number of infected individuals. The conditions (
) and
below must met to guarantee global asymptotically stability.
for
,
is globally asymptotically stable.
for
, where
is a Metzler matrix (the off diagonal elements of
are nonnegative) and
is the region where the model makes biological sense.
Theorem 3.3:
The fixed point is a globally asymptotically stable equilibrium point of (7) provided
and the assumptions (
) and
are satisfied otherwise unstable.
Proof:
Consider
where
and
,
and
.
Then,
where
and
We get
implies that
in the region
whenever
. This implies that
is globally asymptotically stable whenever
.
3.2. Pneumonia mono-infection model analysis
The corresponding pneumonia mono-infection model of the system (3) is determined by making
=
, and it is given by
(11)
(11) with pneumonia infection initial conditions
,
,
, the total population is given by
+
and pneumonia force of infection given by
.
Here like the full model (3) and the HIV/AIDS sub-model (7) in the region , it is sufficient to consider the dynamics of model (11) in
be both biologically and mathematically meaningful.
3.2.1. Local stability of disease-free equilibrium point
Disease-free equilibrium point of the pneumonia mono-infection model (11) is obtained by making its right-hand side as zero and setting the infected class and isolation with treatment class to zero as and after some simple steps of calculations we have got
,
, and
. Hence the pneumonia mono-infection model (11), disease-free equilibrium point is given by
Here we apply the van den Driesch and Warmouth next-generation matrix approach [Citation24] to determine the pneumonia mono-infection model (11) effective reproduction number
. After long computations, we have got the transmission matrix given by
and the transition matrix given by
Then we have determined that
and
Then the spectral radius (effective reproduction number
) of
of the pneumonia mono-infection model (11) is
Theorem 3.4:
The disease-free equilibrium point (DFE) of the pneumonia mono-infection model (11) is locally asymptotically stable if
otherwise unstable.
Proof:
The local stability of the disease-free equilibrium of the system (11) at point
can be studied from its Jacobian matrix and Routh–Hurwitz stability criteria. The Jacobian matrix of the dynamical system at the disease-free equilibrium point is given by
Then the characteristic equation of the above Jacobian matrix is given by
where
and after some steps of computations, we have got
or
or
or
if
or
. Therefore, since all the eigenvalues of the characteristics polynomials of the system (11) are negative if
then disease-free equilibrium point of the pneumonia mono-infection model (11) is locally asymptotically stable.
3.2.2. Existence of endemic equilibrium point (s)
Before checking the global stability of the disease-free equilibrium point of the pneumonia mono-infection model (11), we shall find the possible number of endemic equilibrium point(s) of the model (11). Let be the endemic equilibrium point of pneumonia mono-infection and
be the pneumonia mono-infection mass action incidence rate (‘force of infection’) at the equilibrium point. To find equilibrium point(s) for which pneumonia mono-infection is endemic in the population, the equations are solved in terms of
at an endemic equilibrium point. Now setting the right-hand side of the equations of the model to zero (at steady state) gives
And
where
,
,
,
,
,
,
,
,
,
,
,
,
.
Then we have substituted
in the pneumonia force of infection given by
and we have computed the non-zero solution of
from the cubic equation
(12)
(12) where
(13)
(13)
if
.
It can be seen from (13) that (since the entire model parameters are non-negative). Furthermore,
whenever
. Thus the number of possible positive real roots the polynomial (12) can have depends on the sign of f1. This can be analysed using the Descartes’ rule of signs on the cubic
=
(with x =
). Hence, the following results are established.
Theorem 3.5:
The pneumonia mono-infection model (11) could have
a unique endemic equilibrium point if
either of the following holds:
.
.
two endemic equilibrium points if
, f1<0.
Here, the statement in (b) shows the happening of the backward bifurcation in the model (11), i.e. the locally asymptotically stable disease-free equilibrium point co-exists with a locally asymptotically stable endemic equilibrium point if ; examples of the existence of backward bifurcation phenomenon in mathematical epidemiological models, and the causes, can be seen in [Citation3,Citation21,Citation23,Citation27,Citation39]. The epidemiological consequence is that the classical epidemiological requirement of having the reproduction number
to be less than 1, even though necessary, is not sufficient for the effective control of the disease. The existence of the backward bifurcation phenomenon in sub-model (11) is now explored.
Theorem 3.6:
The pneumonia mono-infection model (11) exhibits backward bifurcation at whenever the inequality
holds, whenever H1 =
and
.
Proof:
Appendix C.
3.2.3. Global stability of the model disease-free equilibrium point (DFE)
We now list two conditions which are sufficient to guarantee the global stability of the pneumonia only disease-free equilibrium point. Following Castillo-Chavez et al. [Citation25], let us rewrite the dynamical system (11) as
where
and
, with
denoting the total number of uninfected individuals and
denoting the total number of infected individuals. The condition (
) and
below must met to guarantee global asymptotically stability.
for
,
is globally asymptotically stable.
for
, where
is a Metzler matrix (the off diagonal elements of
are nonnegative) and
is the region where the model makes biological sense.
Theorem 3.7:
The fixed point is a globally asymptotically stable equilibrium point of (11) provided
and the assumptions (H1) and (H2) are satisfied otherwise unstable.
Proof:
Consider
where
and
,
and
,
and
.
Then, , where,
,
, and
.
We get, .
Thus in the region
, whenever
that is whenever
, where
. This implies that
is globally asymptotically stable whenever
.
3.3. Pneumonia and HIV/AIDS co-infection model analysis
3.3.1. Disease-free equilibrium point
The disease-free equilibrium point of the dynamical system (3) whenever the state variable is given by
3.3.2. Effective reproduction number
Using similar approach to Sections 3.1.1 and 3.2.1, the largest (dominant) eigenvalue (spectral radius) of the matrix: , where
is the rate of appearance of new infection in compartment i,
is the transfer of infections from one compartment
to another and
is the disease-free equilibrium point. After some steps of calculations we have got
and
where
and we have computed as
After some computations and simplifications, the HIV/AIDS and pneumonia co-infection model effective reproduction number is given by
where
is the pneumonia effective reproduction number and
is the HIV/AIDS effective reproduction number.
3.3.3. Locally stability of the disease-free equilibrium (DFE)
The Jacobian matrix of the system (3) at the DFE given by is computed as
where
,
,
,
and
,
,
.
Then the eigenvalues of the matrix are
or
or
or
or
or
or
or
or
or
. Then after some calculations, we have determined that the last two eigenvalues of the quadratic equation as
and
whenever
. Thus, since all the 10 eigenvalues are negative, the disease-free equilibrium point of the full model (3) is locally asymptotically stable whenever
.
4. Analysis of the optimal control strategies
In this section, we analyse the optimal control strategies on the co-infection model (3) to identify the best control strategy that reduces the number of HIV/AIDS infected individuals, the number of pneumonia infected individuals and number of pneumonia and HIVAIDS Co-infected infected individuals. The objective is to find the optimal values of the controls
such that the associated state trajectories
are solution of the system (3) in the intervention time interval
with initial conditions as given in (4) and minimize the objective function. The control
represents the efforts on preventing HIV/AIDS infection using condom that helps to reduce contact rate HIV and
represents the efforts on preventing pneumonia infections that helps to reduce contact rate of pneumonia. Control to increase the recovery of pneumonia infected individuals which means to increase pneumonia recovered individuals
satisfies
, due to the efficacy of anti-pneumonia drug used for the treatment of pneumonia infected individuals and control on the treatment of HIV/AIDS to increase treated number of HIV/AIDS infected individuals
satisfies
, due to the efficacy of anti-HIV drugs used for the treatment of HIV/AIDS infected individuals.
Then the model (3) is now rewritten as
(17)
(17) with the corresponding initial conditions
(18)
(18) The optimal control problem is to minimize the objective functional
(19)
(19) The coefficients
and
are positive weight constants and
and
are the measure of relative costs of interventions associated with the controls
and
, respectively, and also balances the units of integrand. In the cost functional, the term
refers to the cost related to pneumonia infected class, the term
refers to the cost related to individuals mono-infected with HIV and aware, the term
refers to the cost related to co-infected individuals unaware of HIV infection and the term
refers to the cost related to co-infected individuals aware of HIV infection.
, measures the current cost at time t. The set of admissible control functions is defined by
(20)
(20) More precisely, we seek an optimal control pair
(21)
(21)
4.1. Characterization of optimal control
In this section, we present optimality conditions for the optimal control problem defined above and detail its properties. According to the Pontryagin’s Maximum Principle as stated in [Citation29], if is optimal for dynamical system (17) with initial value (18) and (21) with fixed final time
, then there exists a non-trivial absolutely continuous mapping
called the adjoint vector, such that
The Hamiltonian function is defined as
(22)
(22) where
stands for the right-hand side of model (16) which is the ith-state variable equation.
The control system
(23)
(23)
The adjoint system
(24)
(24)
And the optimality condition
(25)
(25) holds for almost all
.
Moreover, the transversality condition
(26)
(26) also holds true.
Furthermore, discussions and characterization of optimal controls and adjoint variables are carried out in Appendix D.
5. Numerical simulations
In this section, using values of the parameters given in Table and some initial population values we carried out, some numerical simulations regarding on the complete HIV/AIDS and pneumonia co-infection model (3) and the resulting optimal control based dynamical system consisting of the state equations (17) and the adjoint system (27). Applying ordinary differential equation solvers coded in MATLAB programming language, we have verified some basic qualitative results of the co-infection model (3) by performed numerical simulation of the models using some initial population and parameter values collected from the ministry of health of Ethiopia (EMOH) and others from the literature and some of them by reasonable assumptions given in Table .
Table 3. Parameter values used for numerical simulation.
5.1. Numerical simulations for the co-infection model (3)
In this section, using some initial population values, parameter values given in Table and applying the forward and backward Range–Kutta scheme we investigate the direction of the co-infection model bifurcation diagram and the behaviours of the co-infection model solutions whenever the corresponding effective reproduction number is greater than unity. We implement the numerical simulations in the maximum time level to be 5 years.
5.1.1. Backward bifurcation diagram
The diagram representation of the HIV/AIDS and pneumonia co-infection model bifurcation diagram given in Figure shows the phenomenon of the HIV/AIDS and pneumonia co-infection model backward bifurcation, which results in the co-existence of positive HIV/AIDS and pneumonia co-infection endemic and disease-free equilibrium points whenever . In such a case, the common conditions of HIV/AIDS and pneumonia co-infection minimization such as making
will not be necessarily effective, and the initial number of HIV/AIDS and pneumonia co-infected individuals also plays a crucial role.
5.1.2. Trajectories of the model (3) solutions whenever 

In this section, we have carried out numerical simulation of the complete co-infection model (3) by applying some initial population values and parameter values given in Table and the simulation illustrated by Figure shows the HIV/AIDS and pneumonia co-infection dynamical system (3) solutions are approaching to the complete model endemic equilibrium point whenever . Biologically it means that the outbreak of HIV/AIDS and pneumonia co-infection throughout the community is consistently present in the study area.
5.2. Optimal control problem numerical simulations
In this section, we investigate the effect of intervention strategies on the transmission of HIV/AIDs and pneumonia co-infection in a population of the study area. The optimal control problem stated in (17)–(21) is solved numerically using the forward and backward Range–Kutta scheme. To verify the qualitative analysis and to investigate the most effective controlling strategy to minimize the number of HIV/AIDs and pneumonia co-infection individuals in a population we implemented the numerical simulation of the co-infection model using some initial population values and parameter values illustrated in Table . We implement the numerical simulations in the maximum time level to be 5 years by considering the following possible illustrated optimal control strategies:
Single strategy at a time
A: use of HIV protection by condom only
B: use of pneumonia protection only
C: use of HIV/AIDS treatment only
D: use of pneumonia treatment only.
Double strategies simultaneously
E: use HIV protection + pneumonia protection only
F: use HIV/AIDS treatment + pneumonia treatment only
G: use HIV protection + HIV/AIDS treatment only
H: use pneumonia protection + pneumonia treatment only
I: use HIV protection + pneumonia treatment only
J: use pneumonia protection + HIV/AIDS treatment only.
Triple strategies simultaneously
K: use HIV protection + pneumonia protection + HIV/AIDS treatment only
L: use HIV protection + pneumonia protection + pneumonia treatment only
M: use HIV protection + pneumonia treatment + HIV/AIDS treatment only
N: use pneumonia protection + pneumonia treatment + HIV/AIDS treatment only.
All the possible mentioned strategies simultaneously
O: use all the four strategies simultaneously.
5.2.1. Impacts of single strategies on the co-infection
In this section, simulation is done when there is no control strategy in place and considering the following controlling strategies: Strategy A: use of HIV protection by condom, we present the simulation of optimal control system (17) with condom use as a protection against HIV infection by Figure (A). Strategy B: Use of pneumonia protection strategy, we present the simulation of optimal control system (17) with protection mechanism
as a protection against pneumonia infection by Figure (B). Strategy C: Use of HIV/AIDS treatment strategy, we present the simulation of optimal control system (17) with HIV/AIDS treatment mechanism
as a treatment against HIV infection by Figure (C). Strategy D: Use of pneumonia treatment strategy, we present the simulation of optimal control system (17) with pneumonia treatment mechanism
as a treatment against pneumonia infection by Figure (D).
From Figure , we observed that the protective strategies illustrated in B and C are more effective strategies compared to the treatment strategies illustrated in A and D. But we recommend that strategy B is the most effective strategy to tackle the co-infection problem in the community.
5.2.2. Impacts of double strategies on the co-infection
In this section, simulation is done when there is no control strategy in place and considering the following controlling strategies: Strategy E: use HIV and pneumonia protection strategies simultaneously, we present the simulation of optimal control system (17) with HIV and pneumonia protection strategies and
simultaneously as a protection against HIV and pneumonia infections respectively and is illustrated by Figure (E). Strategy F: Use HIV and pneumonia treatment strategies simultaneously, we present the simulation of optimal control system (17) with HIV and pneumonia treatment strategies
and
simultaneously as treatments against pneumonia and HIV infections respectively and is illustrated by Figure (F). Strategy G: Use HIV protection and HIV treatment strategies simultaneously, we present the simulation of optimal control system (17) with HIV protection and HIV treatment strategies
and
simultaneously as a control strategy against HIV and pneumonia co-infection and is illustrated by Figure (G). Strategy H: Use pneumonia protection and pneumonia treatment strategies simultaneously, we present the simulation of optimal control system (17) with pneumonia protection and pneumonia treatment strategies
and
simultaneously as a control strategy against HIV and pneumonia co-infection and is illustrated by Figure (H). Strategy I: Use HIV protection and pneumonia treatment strategies simultaneously, we present the simulation of optimal control system (17) with HIV protection and pneumonia treatment strategies
and
simultaneously as a control strategy against HIV and pneumonia co-infection and is illustrated by Figure (I). Strategy J: Use pneumonia protection and HIV treatment strategies simultaneously, we present the simulation of optimal control system (17) with pneumonia protection and HIV treatment strategies
and
simultaneously as a control strategy against HIV and pneumonia co-infection and is illustrated by Figure (J).
From Figure , we observed that the protective and treatment strategies illustrated in G, H, I and J are more effective strategies compared to other strategies illustrated in E and F. But we recommend that strategy J is the most effective strategy to tackle the co-infection problem in the community.
5.2.3. Impacts of triple strategies on the co-infection
In this section, simulation is done when there is no control strategy in place and considering the following controlling strategies: Strategy K: use both HIV and pneumonia protections and HIV treatment strategies simultaneously, we present the simulation of optimal control system (17) with both HIV and pneumonia protections and HIV treatment strategies ,
and
simultaneously as a control strategy against HIV and pneumonia co-infection and is illustrated in Figure (K). Strategy L: Use both HIV and pneumonia protections and pneumonia treatment strategies simultaneously, we present the simulation of optimal control system (17) with both HIV and pneumonia protections and pneumonia treatment strategies
,
and
simultaneously as a control strategy against HIV and pneumonia co-infection and is illustrated in Figure (L). Strategy M: Use both HIV and pneumonia treatments and HIV protection strategies simultaneously, we present the simulation of optimal control system (17) with both HIV and pneumonia treatments and HIV protection strategies
,
and
simultaneously as a control strategy against HIV and pneumonia co-infection and is illustrated in Figure (M). Strategy N: Use both HIV and pneumonia treatments and pneumonia protection strategies simultaneously, we present the simulation of optimal control system (17) with both HIV and pneumonia treatments and pneumonia protection strategies
,
and
simultaneously as a control strategy against HIV and pneumonia co-infection and is illustrated in Figure (N).
From Figure , we observed that the protections and treatment strategies illustrated in K and L are more effective strategies compared to the strategies illustrated in M and N. But we recommend that strategy K is the most effective strategy to tackle the co-infection problem in the community.
5.2.4. Impacts of combination of all strategies on the co-infection
Strategy O: Use all the four strategies HIV protection, pneumonia protection, pneumonia treatment and HIV treatment strategies ,
and
simultaneously, we present the simulation of optimal control system (17) with all strategies
,
and
simultaneously as a control strategy against HIV and pneumonia co-infection and is illustrated by Figure .
In this section, numerical simulation is carried out when there is no control strategy in place and when there are controls involving protection and treatment strategies for both pneumonia and HIV/AIDS single infections. Figure shows the result that all the protection and treatment strategies efforts are implemented, and the number of individuals co-infected with HIV/AIDS and pneumonia decreases drastically to 0 after 3. Using the result given in Figure , we also compared strategy O to each of other strategies and found out that the strategy shows a significant decline in the number of HIV/AIDS and pneumonia co-infected individuals and hence strategy O is the most effective strategies to tackle the co-infection spreading in the community.
6. Discussion and conclusion
In this proposed study, a new 11 mathematical model for the transmission dynamics of pneumonia and HIV/AIDS co-infection with pneumonia protection, pneumonia vaccination, HIV/AIDS protection by using condom, pneumonia treatment and HIV/AIDS treatment measures in a considered community is constructed and assessed the effects of the prevention and control measures such as pneumonia protection, pneumonia vaccination, HIV/AIDS protection, pneumonia treatment, and HIV/AIDS treatment for the pneumonia and HIV/AIDS co-infected individuals in a region where the model is both mathematically and biologically well-posed. Both analytical and numerical analyses of the pneumonia and HIV/AIDS co-infection model (3) were performed to assess the effect of various prevention and controlling strategies for the dynamics of both single infections and co-infection in a considered community.
In this study, the theoretical results are verified by the numerical results and we can summarize results as: the disease-free equilibrium points of all the mono-infection models (7) and (11) and the complete co-infection model (3) are locally asymptotically stable whenever each corresponding effective reproduction numbers are less than unity respectively also the study discusses the global stability of disease-free equilibrium points of the two-sub-models. Using Centre manifold criteria, we have shown the backward bifurcation of the pneumonia infection model and also the HIV/AIDS and pneumonia co-infection model which means there is co-existence of the disease-free equilibrium point(s) together with positive endemic equilibrium point(s) in the region where the co-infection model effective reproduction number is less than unity.
From the numerical simulations, we have observed the following results: from Figure of the numerical simulation, one can observe that the HIV/AIDS and pneumonia co-infection model exhibit the phenomenon of backward bifurcation means whenever the effective reproduction number is less than unity both positive endemic equilibrium and disease-free equilibrium points exist. Figure shows that the solutions of the co-infection model (3) converging to its endemic equilibrium point whenever its effective reproduction number {2.91, 1.23
Figure shows the impact of every single strategy separately to tackle the HIV/AIDS and pneumonia co-infection spreading dynamics. Figure shows the impact of every double strategy separately to tackle the HIV/AIDS and pneumonia co-infection spreading dynamics. Figure shows the impact of every triple strategy separately to tackle the HIV/AIDS and pneumonia co-infection spreading dynamics. Figure shows the impact of the combination of all strategies simultaneously to tackle the HIV/AIDS and pneumonia co-infection spreading dynamics. Compared to the other control strategies investigated and discussed applying all the protections and treatments controlling strategies simultaneously as stated in the discussion of Figure is the most effective strategy to decrease the HIV/AIDS and pneumonia co-infection transmission mechanisms in the community under the study.
The limitations of this study and the next potential researchers can incorporate and extend the model under this study will be: stochastic approach, fractional order derivative approach, environmental impacts, age and spatial structure, validate the model by applying appropriate real infection data.
Authors’ contributions
All authors have read and approved the final manuscript.
Math Classification
92-10 Mathematical modelling or simulation for problems pertaining to biology.
Acknowledgments
The authors thank Mr. Sitotaw Eshete for his valuable Wi-Fi contribution.
Disclosure statement
No potential conflict of interest was reported by the author(s).
Data availability statement
Data used to support the findings of this study are included in the article.
Additional information
Funding
References
- GT Tilahun, OD Makinde, D. Malonza Modelling and optimal control of pneumonia disease with cost-effective strategies. J Biol Dyn. 2017;11(Suppl. 2):400–426. doi:10.1080/17513758.2017.1337245
- M. Martcheva An introduction to mathematical epidemiology. Vol. 61. New York: Springer; 2015.
- A Nwankwo, D. Okuonghae Mathematical analysis of the transmission dynamics of HIV syphilis co-infection in the presence of treatment for syphilis. Bull Math Biol. 2018;80(3):437–492. doi:10.1007/s11538-017-0384-0
- R. Aggarwal “Stability analysis of a delayed HIV-TB co-infection model in resource limitation settings. Chaos Solit Fractals. 2020;140:110138. doi:10.1016/j.chaos.2020.110138
- M Aslam, R Murtaza, T Abdeljawad, et al. A fractional order HIV/AIDS epidemic model with Mittag–Leffler kernel. Adv Differ Equ. 2021;2021(1):1–15. doi:10.1186/s13662-021-03264-5
- JK Nthiiri, GO Lavi, A. Mayonge Mathematical model of pneumonia and HIV/AIDS coinfection in the presence of protection. Int J Math Anal. 2015;9(42):2069–2085. doi:10.12988/ijma.2015.55150
- EA Bakare, CR. Nwozo Bifurcation and sensitivity analysis of malaria–schistosomiasis co-infection model. Int J Appl Comput Math. 2017;3(1).
- MJOJ Otieno, O. Paul Mathematical model for pneumonia dynamics with carriers. 2013.
- S Saber, AM Alghamdi, GA Ahmed, et al. Mathematical modelling and optimal control of pneumonia disease in sheep and goats in Al-Baha region with cost-effective strategies. AIMS Math. 2022;7(7):12011–12049. doi:10.3934/math.2022669
- M Kizito, J. Tumwiine A mathematical model of treatment and vaccination interventions of pneumococcal pneumonia infection dynamics. J Appl Math. 2018; 2018. doi:10.1155/2018/2539465
- M Naveed, D Baleanu, A Raza, et al. Modeling the transmission dynamics of delayed pneumonia-like diseases with a sensitivity of parameters. Adv Differ Equ. 2021;2021(1):1–19. doi:10.1186/s13662-021-03618-z
- SJ. Aston Pneumonia in the developing world: Characteristic features and approach to management. Respirology. 2017;22(7):1276–1287. doi:10.1111/resp.13112
- CW Kanyiri, L Luboobi, M. Kimathi Application of optimal control to influenza pneumonia coinfection with antiviral resistance. Comput Math Methods Med. 2020;2020. doi:10.1155/2020/5984095
- FK Mbabazi, JYT Mugisha, M. Kimathi Global stability of pneumococcal pneumonia with awareness and saturated treatment. J Appl Math. 2020;2020. doi:10.1155/2020/3243957
- MI Ossaiugbo, NI. Okposo Mathematical modeling and analysis of pneumonia infection dynamics. Sci World J. 2021;16(2):73–80.
- H-F Huo, R. Chen Stability of an HIV/AIDS treatment model with different stages. Discrete Dyn Nat Soc. 2015;2015.
- EO Omondi, RW Mbogo, LS. Luboobi Mathematical analysis of sex-structured population model of HIV infection in Kenya. Lett Biomath. 2018;5(1):174–194. doi:10.30707/LiB5.1Omondi
- A Babaei, H Jafari, A. Liya Mathematical models of HIV/AIDS and drug addiction in prisons. Eur Phys J Plus. 2020;135(5):1–12. doi:10.1140/epjp/s13360-020-00400-0
- C Castillo-Chavez, B. Song Dynamical models of tuberculosis and their applications. Math Biosci Eng. 2004;1(2):361. doi:10.3934/mbe.2004.1.361
- SW Teklu, TT. Mekonnen HIV/AIDS-pneumonia co-infection model with treatment at each infection stage: mathematical analysis and numerical simulation. J Appl Math. 2021;2021. doi:10.1155/2021/5444605
- SW Teklu, KP. Rao HIV/AIDS-pneumonia codynamics model analysis with vaccination and treatment. Comput Math Methods Med. 2022;2022.
- I Ahmed, EFD Goufo, A Yusuf, et al. An epidemic prediction from analysis of a combined HIV-COVID-19 co-infection model via ABC-fractional operator. Alexandria Eng J. 2021;60(3):2979–2995. doi:10.1016/j.aej.2021.01.041
- AB Gumel, JM- Lubuma, O Sharomi, et al. Mathematics of a sex-structured model for syphilis transmission dynamics. Math Methods Appl Sci. 2018;41(18):8488–8513. doi:10.1002/mma.4734
- P Van den Driessche, J. Watmough Reproduction numbers and sub-threshold endemic equilibria for compartmental models of disease transmission. Math Biosci. 2002;180(1–2):29–48. doi:10.1016/S0025-5564(02)00108-6
- C Castillo-Chavez, Z Feng, W. Huang On the computation of R0 and its role on global stability, 2002. Math. la. asu. edu/chavez/2002/JB276. pdf. 2002.
- N Ringa, ML Diagne, H Rwezaura, et al. HIV and COVID-19 co-infection: A mathematical model and optimal control. Inform Med Unlocked 2022;31:100978.
- SW. Teklu Mathematical analysis of the transmission dynamics of COVID-19 infection in the presence of intervention strategies. J Biol Dyn. 2022;16(1):640–664. doi:10.1080/17513758.2022.2111469
- SW Teklu, BB. Terefe Mathematical modeling investigation of violence and racism coexistence as a contagious disease dynamics in a community. Comput Math Methods Med. 2022;2022.
- SW Teklu, BB. Terefe Mathematical modeling analysis on the dynamics of university students animosity towards mathematics with optimal control theory. Sci Rep. 2022;12(1):1–19. doi:10.1038/s41598-021-99269-x
- R Aggarwal, YA. Raj A fractional order HIV-TB co-infection model in the presence of exogenous reinfection and recurrent TB. Nonlinear Dyn. 2021;104(4):4701–4725. doi:10.1007/s11071-021-06518-9
- MU Nsuami, PJ. Witbooi A model of HIV/AIDS population dynamics including ARV treatment and pre-exposure prophylaxis. Adv Differ Equ. 2018;2018(1):1–12. doi:10.1186/s13662-017-1458-x
- S Saha, GP. Samanta Modelling and optimal control of HIV/AIDS prevention through PrEP and limited treatment. Physica A. 2019;516:280–307. doi:10.1016/j.physa.2018.10.033
- GP. Samanta Analysis of a nonautonomous HIV/AIDS epidemic model with distributed time delay. Math Model Anal. 2010;15(3):327–347. doi:10.3846/1392-6292.2010.15.327-347
- GP. Samanta Analysis of a nonautonomous HIV/AIDS model. Math Model Nat Phenom. 2010;5(6):70–95. doi:10.1051/mmnp/20105604
- GP. Samanta Permanence and extinction of a nonautonomous HIV/AIDS epidemic model with distributed time delay. Nonlinear Anal. Real World Appl. 2011;12(2):1163–1177. doi:10.1016/j.nonrwa.2010.09.010
- S Sharma, GP. Samanta Dynamical behaviour of an HIV/AIDS epidemic model. Diff Equ Dyn Syst. 2014;22(4):369–395. doi:10.1007/s12591-013-0173-7
- S Saha, P Dutta, G. Samanta “Dynamical behavior of SIRS model incorporating government action and public response in presence of deterministic and fluctuating environments. Chaos Solit Fractals. 2022;164:112643. doi:10.1016/j.chaos.2022.112643
- SW Teklu, BS. Kotola A dynamical analysis and numerical simulation of COVID-19 and HIV/AIDS co-infection with intervention strategies. J Biol Dyn. 2023;17(1):2175920. doi:10.1080/17513758.2023.2175920
- BS Kotola, SW Teklu, YF. Abebaw Bifurcation and optimal control analysis of HIV/AIDS and COVID-19 co-infection model with numerical simulation. PLoS One. 2023;18(5):e0284759. doi:10.1371/journal.pone.0284759
- SW Teklu, BB. Terefe COVID-19 and syphilis co-dynamic analysis using mathematical modeling approach. Front Appl Math Stat. 2023;8:1101029. doi:10.3389/fams.2022.1101029
Appendices
Appendix A. Proof of Theorem 2.1
Proof:
Let us consider ,
,
,
,
,
,
,
and
then for all t > 0, we have to show that
(t) > 0,
,
> 0,
>0,
>0,
>0,
>0,
>0,
and
> 0.
Define: = sup
,
,
>0,
>0,
> 0,
> 0
. Since all the state variables
,
,
,
,
, and
are continuous and we can justify that
. If
= +∞, then non-negativity holds. But, if 0 <
< +∞, we will have
or
or
or
or
or
or
or
or
or
or
.
From the first equation of the model (3), we do have
And integrate both sides using integrating factor, we have determined the constant value
where
and from the meaning of
, the solutions
,
, also the exponential function always is positive, then the solution
hence
.
Again from the second equation of the model (3), we do have .
And also using t integrating factor after some calculations we obtained that
where
, and from the meaning of
, the solution
hence
.
Similarly, hence
,
hence
,
hence
,
hence
,
hence
,
hence
,
hence
,
hence
, and
hence
.
Thus , and hence all the solutions of the pneumonia and HIV/AIDS co-infection model (3) are non-negative.
Appendix B. Proof of theorem 2.2
Proof: Let be an arbitrary non-negative solution of the system (3) with initial conditions given in Equation (4).
Now adding all the differential equations given in Equation (3), we do have the derivative of the total population which is given in Equation (5) as
Then by ignoring all the infection classes, we have determined that
and using the separation of variables whenever
we have obtained that
. Hence, all the positive feasible non-negative solutions of the co-infection model (3) entering into the region are given in Equation (6).
Appendix C. Proof of theorem 3.6
In this section, we apply the centre manifold theory [Citation19] to ascertain the local stability of the endemic equilibrium due to the convolution of the first approach (eigenvalues of the Jacobian matrix). To make use of the centre manifold theory, the following change of variables is made by symbolizing ,
,
, and
such that
. Furthermore, by using vector notation
, the COVID-19 mono-infection model (11) can be written in the form
with
, as follows:
(14)
(14) with
then the method entails evaluating the Jacobian of the system (14) at the DFE point
, denoted by
and this gives us
Consider,
and suppose that
is chosen as a bifurcation parameter. From
as
.
Solving for β2 we have got .
After some steps of the calculation, we have got the eigenvalues of
as
,
or
or
or
. It follows that the Jacobian
of Equation (14) at the disease-free equilibrium with
, denoted by
, has a simple zero eigenvalue with all the remaining eigenvalues have negative real part. Hence, Theorem 2.2 of Castillo-Chavez and Song [Citation19] can be used to analyse the dynamics of the model to show that the model (11) undergoes backward bifurcation at
.
Eigenvectors of : For the case
, it can be shown that the Jacobian of the system (14) at
(denoted by
has a right eigenvectors associated with the zero eigenvalue given by
as
(15)
(15) Then solving equation (15) the right eigenvectors associated with the zero eigenvalue are given by
Similarly, the left eigenvector associated with the zero eigenvalues at
given by
as
(16)
(16) where
.
Then solving Equation (16), the left eigenvectors associated with the zero eigenvalue are given by and
. After long steps of calculations, the bifurcation coefficients
and
are obtained as
where
=
and
.
Thus the bifurcation coefficient is positive whenever
.
Moreover
Hence, from in Castillo-Chavez and Song [Citation19] the pneumonia mono-infection model (11) exhibits a backward bifurcation at
and whenever
.
Appendix D. Discussion and characterization of optimal controls and adjoint variables
Theorem 3.8:
Let be the optimal control and
be the associated unique optimal solutions of the optimal control problem (17) with initial condition (18) and objective functional (19) with fixed final time
(20). Then there exists adjoint function
satisfying the following canonical equations:
(27)
(27)
with transversality conditions
(28)
(28) Moreover, the corresponding optimal controls
and
are given by
(29)
(29) From the previous analysis, to get the optimal point, we have to solve the system
With the Hamiltonian
where
and
.