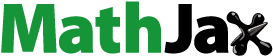
ABSTRACT
Symptomatic and asymptomatic individuals play a significant role in the transmission dynamics of novel Coronaviruses. By considering the dynamical behaviour of symptomatic and asymptomatic individuals, this study examines the temporal dynamics and optimal control of Coronavirus disease propagation using an epidemiological model. Biologically and mathematically, the well-posed epidemic problem is examined, as well as the threshold quantity with parameter sensitivity. Model parameters are quantified and their relative impact on the disease is evaluated. Additionally, the steady states are investigated to determine the model's stability and bifurcation. Using the dynamics and parameters sensitivity, we then introduce optimal control strategies for the elimination of the disease. Using real disease data, numerical simulations and model validation are performed to support theoretical findings and show the effects of control strategies.
1. Introduction
Infectious or communicable diseases are disorders produced by fungi and bacteria, viruses, or parasites. Various infections can be transferred from individual to individual, or from some contaminated water, food being exposed to organisms in the environment. Symptoms and signs are depending on the organisms causing the infection, but fatigue, fever, etc are common symptoms. Hepatitis A and B, Tuberculosis, flu, SARS, and influenza are some common examples. Coronaviruses are zoonotic diseases transmitted between animals and humans. The novel Coronavirus or SARS-CoV-2, initially, started in a seafood market in Wuhan, China. The pandemic initially began with a hospital admitting patients diagnosed with pneumonia. The sickness of patients was associated with the wet animal and seafood market [Citation1]. The infection of SARS-CoV-2 virus transmits via multiple ways, e.g. due to human interaction via exposure to spoil surfaces directly and by respiratory droplets inhalation as well as from an infected person, however, the pandemic rises due to human interaction [Citation2]. The various infection phases of the novel disease are asymptomatic and symptomatic. Especially, the asymptomatically infected individuals are crucial as they do not exhibit symptoms but transmit the infection. Therefore, a small amount of asymptomatically infected individuals will lead to a major disaster. Various pharmaceutical and non-pharmaceutical control strategies have been exercised for the control of the novel disease of the Coronavirus. For example, imposing mandatory and strict lockdown, maintaining social distancing, avoiding crowds, and masks use especially in public places, and imposing the ban on any type of gathering (social and religious) [Citation3,Citation4]. Similarly, the detected cases are placed in isolation for quick interventions [Citation4]. Coronavirus has affected many countries around the world, including China, India, Canada, France, Germany, the United States, Pakistan, Oman, the United Arab Emirates, Africa, Southeast Asia, Europe, and the Western Pacific. As a result, many people suffered and died [Citation5–7].
Mathematical modelling and optimal control of infectious disease is an emerging area that plays a significant role in exploring disease dynamics and predicting control measures [Citation8–13]. The temporal dynamics of infectious diseases can be well understood with the aid of mathematical models. Usually, the models represent the interacting components arising in epidemiology and population biology [Citation14–16]. These models can help to understand the dynamics and propose control strategies for disease eradication which are useful for health control authorities. With these guidelines, different steps are easily taken to control infection. Several models have been created to depict the time-based behaviour of contagious diseases. For example, a mathematical model has been presented to discuss the stability analysis with optimal vaccination using the concept of the classical SIR model in Ref. [Citation17]. A model representing the dynamics of hepatitis B has been studied by Khan et al. [Citation18]. Other mathematical models can be found in the literature for investigating the epidemiology of infectious diseases (see for detail, Refs [Citation19–21]). Since, the epidemiology of infectious diseases is characterized by dynamic and evolving scenarios, so optimal control theory to design adaptive controls that can respond to change the conditions of disease transmissibility, the emergence of new variants and population behaviour. By continuously updating and optimizing control measures based on real-time data and feedback, optimal control theory enables a more proactive and agile approach to disease management [Citation22,Citation23].
The spreading and control of SARS-CoV-2 was difficult but in fact still a burning issue, and therefore the attention of various researchers has been attracted (see for more detail, Refs [Citation24–33]). Since it is very much clear that the newly reported disease of SARS-CoV-2 virus has a complex nature. Especially, the different infection phases are very significant because the asymptomatically infected individuals have no symptoms and transmit the disease to others. It could be also noted that some of the individuals having no history of the asymptomatic stage while direct leads to the symptomatic stage. In this case, the probability-based transmission co-efficient is more reasonable because a susceptible population leads to asymptomatic and symptomatic at the same time. Moreover, vaccines for SARS-CoV-2 are now available and provide protection up to a certain degree of realism. Thus, we focus to develop an epidemiological model that contains the various infected groups with probability-based transmission and vaccination of susceptible in the form of an extraordinarily complex dynamical system to investigate the time dynamics and optimal control of the novel disease.
By assuming both symptomatic and asymptomatic individuals as sources of disease transmission, in this paper, we develop an epidemiological model to study the pandemic trend and optimal control of the novel disease. In more detail, the different populations are divided into four compartments, s, c, a, and r, which denote susceptible, symptomatically and asymptomatically infected, and recovered populations. When susceptible individuals interact successfully with infected individuals, they will enter both symptomatic and asymptomatic compartments. Due to the fact that susceptible individuals move to both infected compartments simultaneously, we use the probability-based transmission co-efficient. We then discuss the biological and mathematical feasibility of the considered epidemic problem. A detailed sensitivity analysis will be performed for every parameter in the model as well as the role that it plays in disease transmission and control, as well as the model equilibria will be examined. In addition, we analyse the bifurcation analysis of the model by using linear stability theory. The proposed model exhibits backward bifurcation based on the centre manifold theory. To eliminate the contagious infection of novel Coronavirus transmission, we designed an optimal control mechanism using optimal control theory, based on the temporal dynamics and parameter sensitivity of the model. Using real data of the novel disease, we validate the model and parameterize the parameters involved in its epidemic process. As a result, we use the estimated value for long-run numerical simulations to support our theoretical findings and show how the control measure affects disease transmission. This article is structured as follows. In Section 2, we discuss the detailed model formulation with positivity and boundedness. Section 3 shows how we derive the threshold quantity and calculate the equilibria in order to test the sensitivity of the model parameters and show how backward bifurcations occur. In Section 4, we proposed an optimal control mechanism for the presentation of Coronavirus disease. Section 5 presents the model calibration to support our theoretical results and the validity of the model. In the final Section 6, we provide a brief conclusion.
2. Formulation of the model
We present the model formulation to describe the time dynamics of the novel Coronavirus (SARS-CoV-2) disease. Since the symptomatically and asymptomatically infected individuals are very significant in the transmission of the novel disease of Coronavirus, therefore due to the characteristics of the novel disease, we distribute the entire community in the vulnerable or susceptible (those who are at risk), symptomatic, asymptomatic, and removed/recovered individuals, who are respectively characterized by , and
. The susceptible individuals
at time t are those who are yet not infected but have a chance to catch the infection at time
, where
is a small increment in t. The asymptomatic individuals
have no symptoms while becoming the source of the disease transmission. Similarly, the individuals showing symptoms of SARS-CoV-2 are known as symptomatic individuals, and those who become healthy after getting infected are the recovered population. We assume the newborn and immigrants from other compartments are susceptible because there is still no evidence of vertical transmission, although the immigrants may or may not be infected. Further, we assume a homogeneously mixed population, then the susceptible individuals enter the asymptomatic and symptomatic compartment after successful interaction with symptomatically and asymptomatically infected individuals. Two types of recovery for infected individuals are natural and based on treatment. The asymptomatic individuals enter the symptomatic compartment after producing symptoms of the SARS-CoV-2 virus. Moreover, the individuals leave the asymptomatic and symptomatic population group if they are fully recovered or die. Based on the effectiveness of the novel Coronavirus vaccination, those susceptible individuals which are successfully vaccinated lead to the recovered compartment. The complete transition of the novel disease is presented in the flowchart as presented in Figure .
Therefore, we illustrate the time dynamics of disease as follows:
(1)
(1) with the initial compartmental population sizes
(2)
(2) Here, the inflow of newborns is denoted by Π, and β is the disease transmission coefficient. It is assumed that γ represents the reduced rate of spreading, whereas the rate ν is indicative of the vaccination. Moreover, the natural as well as the SARS-CoV-2 related death rates are symbolized by
,
and
, respectively. Similarly,
and
denote the recovery of asymptomatic (natural) and symptomatic individuals (due to treatment). Also, it is assumed that p is the probability of those who lead to the asymptomatic population group while q denotes the probability of those who recover from symptomatic directly.
We assume that ,
and
to shorten our calculations. We also assume that all parameters and constants used in the model (Equation1
(1)
(1) )–(Equation2
(2)
(2) ) have non-negative values because of the population dynamical problem. In the upcoming section, we will prove the well-posedness of the proposed epidemic problem.
2.1. Positivity and bounded-ness
To prove the well-posedness of the model, it is essential to investigate all the state variables having non-negative values.
Theorem 2.1
For all non-negative initial conditions, the solutions, , of the proposed model are non-negative and uniformly bounded that is
, whenever the time grows without bounds. Further, the proposed epidemic problem is well-defined and positively invariant in the feasible biological region given by
(3)
(3)
Proof.
Let be solution of the epidemiological system (Equation1
(1)
(1) ), then by integrating the first equation, we obtain
(4)
(4) According to the second equation in the epidemiological model (Equation1
(1)
(1) ), it can be written as
which leads to
(5)
(5) In a similar fashion, the last two equations of the model look like
Integrating, we then obtain
(6)
(6) It could be noted from Equations (Equation4
(4)
(4) ), (Equation5
(5)
(5) ) and (Equation6
(6)
(6) ) that the solutions
of system (Equation1
(1)
(1) ) satisfying the initial conditions (Equation2
(2)
(2) ) remain non-negative for every
.
To proceed further, let denote the sum of the compartmental population, then
which yields that
(7)
(7) From Equation (Equation7
(7)
(7) ), it is observed that
becomes less than or equal to
whenever time grows. Thus it is follows that the total population in the region
is bounded by
with growing time (
), therefore, the solution trajectories of the model satisfying the initial conditions are bounded.
Since the model state variables are non-negative and implies that the epidemic problem (Equation1
(1)
(1) )–(Equation2
(2)
(2) ) is well-posed. Further, we assume that
, then from Equation (Equation7
(7)
(7) ), we conclude that
, thus every solution of the proposed epidemic problem with initial conditions in
remains in Ω and is well-defined dynamical system and positively invariant.
3. Steady states analysis and reproductive number
Here, we discuss the steady states of the model and find the reproductive number. First, we calculate the SARS-CoV-2 virus-free equilibrium of the model and find the threshold parameter to perform the sensitivity analysis. Further, we use the threshold parameter to calculate the endemic state of the model and its detailed temporal dynamics.
3.1. SARS-CoV-2 virus-free equilibrium and reproductive number
The model reported by the system (Equation1(1)
(1) ) is examined for equilibrium. We calculate the infection-free and disease-endemic states of the proposed model by assuming that
is the disease-free state as given by
, where
,
. We calculate the reproductive quantity by following Driessche and Watmough [Citation34] and Diekmann et al. [Citation35], and accordingly used by Khajanchi et al. [Citation36]. For this, let us assume that
, then
where
Calculating the dominant eigenvalue ρ of the matrix
implies
(8)
(8) For the shake of simplicity, let us assume that
,
and
, then the threshold quantity becomes
.
3.2. Sensitivity analysis of the reproductive number
We discuss the sensitivity analysis of the proposed model and its associated impact on the threshold parameter. The reproduction number plays an essential role to study the robustness of model prediction as the initial transmission of any epidemic is directly associated with this quantity. Because a small change in the value of the most sensitive parameter can result in a great effect on any epidemiological process [Citation30]. The normalized sensitivity analysis helps to figure out the most sensitive parameter with respect to the threshold quantity. We carry out the normalized sensitivity to determine the most sensitive parameters about the persistence, reduction, and prevalence of SARS-CoV-2 virus transmission.
Definition 3.1
Let Φ be any epidemic parameter and is the threshold quantity, the normalized forward sensitivity index of Φ is defined as
(9)
(9)
To calculate the association of the model parameters with the basic reproductive quantity and its relative effect, we calculate the following partial derivatives:
It is evident from the above relationships that certain parameters exert a positive influence on the basic reproductive number, while others have a negative influence. The + and − signs of the indices respectively describe the direct and inverse relation of the model parameters with the reproductive quantity. If the parameters that directly affect the threshold quantity are decreased or increased, the value of the threshold quantity will also decrease or increase accordingly. But in contrast, the basic reproductive number will decrease or increase with the increase or decrease in the value of parameters which are in inverse relation. We calculate the sensitivity indices and their relative effect with the aid of the normalized sensitivity index formula given in the Equation (Equation9
(9)
(9) ) to compute the parameters which are more sensitive to the novel disease of Coronavirus transmission and its prevention. In this case, we use the parameter values as:
,
,
,
,
,
, p = 0.26, q = 0.79,
,
and
. We accordingly calculate the indices with sensitivity as given in Table .
Table 1. Sensitivity indices and its relative impact on the basic reproductive number ().
The parameters which have in direct proportion with the basic reproductive number are , while the set of those parameters which have inverse relation is listed as
. We observe that an increase or decrease in the value of β, for example, by 10 % would increase or decrease the value of
by 10.0 %. In a similar fashion, if we perturb the value of γ, q, and p by 10 %, increase or decrease the value of
by 2.8 %, 0.36 % and 2.02 %, respectively. On the other hand, the sensitivity indices of the parameters ν,
, and
reveal that if their values are increased by 10 % would collectively decrease
by 13.94
The parameters that significantly affect reproduction number are β, γ, , and ν. In the case of the novel Coronavirus disease, the disease-spreading coefficient is the most influential epidemic parameter. Furthermore, the normalized sensitivity index of the vaccination rate indicates vaccination is crucial to reducing Coronavirus disease propagation. In addition, the parameters
and
are also essential for decreasing the reproductive number by increasing the recovery of the infected population. Consequently, we recommend control measures to slow the progress of β and γ and to strengthen the capacity of ν,
, and
.
3.3. Endemic state of the model
We use this the threshold quantity () to calculate the endemic state of the epidemiological model (Equation1
(1)
(1) ) by assuming
, where
(10)
(10) It can be observed clearly from the Equation (Equation10
(10)
(10) ) that the endemic states of the model exist whenever
. Thus we state a lemma as follows.
Lemma 3.2
The proposed epidemiological model has disease endemic states whenever . Otherwise, the proposed epidemic problem has only a disease-free equilibrium.
3.4. Stability analysis and existence of bifurcation
We investigate the model stabilities and existence of bifurcation to show the dynamics of the epidemic problem that is under consideration. For this, we state the results in the following.
Theorem 3.3
The infection-free state () of the epidemiological model (Equation1
(1)
(1) ) is stable locally asymptotically, whenever
.
Proof.
To determine the local dynamics of the epidemic problem at , we compute the Jacobian matrix
of the model (Equation1
(1)
(1) ) around infection-free equilibrium as
(11)
(11) Clearly,
have two eigenvalues such that
and
. For the rest of the eigenvalues we take the following matrix in reduced form
It could be noted from the matrix A, that
and the determinant of the matrix (A) gives
Clearly, the
and the
whenever
, which implies that Routh-Hurwitz criteria satisfied and the matrix A having negative eigenvalues or having negative real parts of the eigenvalues, thus all the eigenvalues of
at
are negative.
Now to discuss the bifurcation analysis of the proposed model, we assume the bifurcation parameter is , and at
, the value of the bifurcation parameter,
gives
(12)
(12) then regarding the existence of bifurcation, we establish the following result.
Theorem 3.4
The proposed SARS-CoV-2 virus epidemiological model undergoes a backward bifurcation at .
Proof.
The Jacobian matrix of the considered model evaluated at and at the disease-free equilibrium which looks like
Calculated the eigenvalues of the matrix,
, we obtain
We observed that three of the eigenvalues are negative or having negative real parts and one zero eigenvalue. Thus, the proposed epidemiological model has hyperbolic solution which greentree the existence of bifurcation. To find the direction of bifurcation, we assume that
and
are the left and right eigenvector associated with the zero eigenvalue, then
and
To proceed further, let us assume that
represent the right-hand side of the k-th equation of the proposed model and
be the state variables whose derivative is described by the k-th equation for k = 1, 2, 3, 4. Following centre manifold theory and the results stated by Theorem 4.1 in Ref. [Citation37], the local bifurcation at around the bifurcation parameter
is then investigated by calculating the two constants given as
where
and the first zero in
correspond to the SARS-CoV-2 virus-free equilibrium of the model. To calculate the above constants for the proposed model, let as assume that
,
,
and
. Thus, after some algebraic manipulation, we arrive at
It should be noted that both the co-efficient,
and
, thus the system exhibits the phenomenon of backward bifurcation.
Theorem 3.5
If , then the proposed model (Equation1
(1)
(1) ) at the disease endemic state (
) is stable.
Proof.
In a similar fashion, the linearized matrix of the system (Equation1(1)
(1) ) at the endemic state looks like
Clearly,
has one negative eigenvalue i.e.
. We take the reduced matrix to find the other eigenvalues as follows
Calculating the auxiliary equation
, we obtain
(13)
(13) where
Moreover
We noted that the above coefficients A, B, and C of the auxiliary Equation (Equation13
(13)
(13) ) are positive, and if AB−C is positive then it ensures that the characteristic equation has all negative roots and so the proposed model around disease endemic state is stable.
Theorem 3.6
The disease-free state () of the epidemiological system (Equation1
(1)
(1) ) is stable globally asymptotically, if and only if
.
Proof.
We investigate the global analysis of the considered problem as stated by Equation (Equation1(1)
(1) ) around
by using the concept of Lyapunov function theory. On the basis of this, we define a real-valued function
is given by
(14)
(14) Thus, the temporal differentiation of Equation (Equation14
(14)
(14) ) and making the substitution of the model (Equation1
(1)
(1) ) gives that
(15)
(15) Further, the substitution of the components of the disease-free equilibrium and then a little re-arrangement leads to the following assertion
(16)
(16) It can be noted from Equation (Equation16
(16)
(16) ), that
is negative and consequently equal to zero at (
), which satisfied the properties of Lyapunov function. Hence
is a Lyapunov function and therefore the invariance principle [Citation38] then implies that the proposed epidemiological model (Equation1
(1)
(1) ) is globally stable at the infection-free state.
Theorem 3.7
The endemic disease equilibrium () of the system (Equation1
(1)
(1) ) is globally asymptotically stable, if and only if
.
Proof.
We discuss the global analysis of the model around the endemic equilibrium. For this, we define a function given by
(17)
(17) Taking the temporal differentiation of
and making use of Equation (Equation1
(1)
(1) ) gives
From the above equation, we observe that
, and consequently equal to zero around the endemic state
, which justifies the axioms of Lyapunov function, so
is a Lyapunov function, and hence according to the LaSalle's invariance principle [Citation38], the proposed problem (Equation1
(1)
(1) ) is globally stable at the disease endemic state (
).
4. Optimal control analysis
Optimal control theory plays a pivotal role in the epidemiology of infectious diseases by providing a rigorous framework for designing effective control strategies and guiding decision-making processes. Optimal control theory enables researchers and policymakers to identify control measures that maximize the desired public health outcomes. By formulating the epidemiological problem as an optimization task, one can seek control strategies that minimize disease burden, reduce transmission rates, limit the number of cases, or achieve other specific objectives. This approach ensures that limited resources and interventions are utilized in the most efficient and effective manner, ultimately leading to improved public health outcomes. Moreover, optimal control theory takes into account the inherent constraints and limitations of resource availability. With the finite availability of control measures in the form of vaccines, treatment facilities, healthcare personnel, and other resources, it becomes essential to allocate them optimally to achieve the greatest impact. By incorporating resource constraints into the optimization framework, optimal control theory aids in determining the optimal distribution and utilization of resources to curb the spread of infectious diseases.
In addition, the epidemiology of infectious diseases is characterized by dynamic and evolving scenarios. Optimal control theory allows for the design of adaptive control strategies that can respond to changing conditions, such as variations in disease transmissibility, the emergence of new variants, or alterations in population behaviour. By continuously updating and optimizing control measures based on real-time data and feedback, optimal control theory enables a more proactive and agile approach to disease management. Similarly, optimal control theory provides a framework to explore trade-offs and balance competing objectives in disease control. It allows for the quantification and comparison of the costs and benefits associated with different control measures, considering both short-term and long-term perspectives. This analysis assists policymakers in making informed decisions by identifying cost-effective interventions that yield the greatest health benefits within the available resources. Optimal control theory facilitates the assessment of the potential impact of different control strategies. By mathematically modelling the epidemiological dynamics and incorporating various intervention measures, researchers can simulate different scenarios and predict their outcomes. This predictive capability enables the evaluation of the effectiveness and efficiency of different interventions before their implementation, helping to inform policy decisions and prioritize interventions based on their expected impact.
We formulate an optimal control problem to mitigate the spread of SARS-CoV-2 virus transmission by assuming a set of three control measures ,
and
that minimize the infected population over a given time frame. Physically,
represents the non-pharmaceutical control intervention strategy, implementing personal protection (using mask, avoiding gathering and keeping social distances etc.) that minimizes the spreading of novel Coronavirus transmission and slow down the intensity of β and γ. To optimize the interaction of infected and susceptible, we assume that
and introduce the control factor,
. This implies that whenever
, the control is fully responsive, while having no response at
. By full response, we mean that the interaction between susceptible and infected individuals vanishes. Moreover, the current scenario justifies that there is sufficient treatment available for those individuals which are clinically ill, therefore, we incorporate treatment as a control measure for clinically infected individuals by introducing the term
to minimize the infected population by keeping in view the normalized sensitivity indices of
and
, where the controlling attempt
represents the treatment intensity lies between zero and unity. In addition, the sensitivity index of vaccination parameter reveals that vaccination plays a vital role in the reduction of novel Coronavirus, and vaccine for the prevention of SARS-CoV-2 virus are available which provide safety up to a certain degree of realism, so to incorporate vaccination is a control measure, we incorporate
to maximize the number of recovered population. Thus, the clear goal of our control strategies is to enhance the response of susceptible and recovered by increasing the intensities of the control function
and
to overcome the novel Coronavirus spreading, while reducing the infected population with the aid of controlling effect of
. More precisely, the goal of our control mechanism is to maximize the recovered and to minimize the infected population, thus we define the following control set
(18)
(18) To describe the control problem, we formulate the objective functional to be minimized is as
(19)
(19) subject to the control system, an extended version of the proposed model, which takes the following form
(20)
(20) with
(21)
(21) In the above, the quantities
respectively represent the weight constants of asymptomatic, symptomatic, and the weight constants associated with the personal protection, treatment and vaccination against SARS-CoV-2 virus disease. The quadratic term
represent the cost corresponding to the control of susceptible and infected individuals interaction by personal protection, while
and
are the costs associated with the treatment and vaccination control measures. Basically, the cost correspond to the control measure
comes from the implementation of personal protection, educating the health official and public to properly wear mask, use sanitizer and keeping social distances etc., while the cost associated with the other two controls directly come from the treatment and vaccination of the individuals. The costs is assumed to be in proportion to the square of the control measure they are associated with. Thus, the objective of our control problem is to find the optimal value
for the control measure such that
First, we prove the existence analysis for the proposed optimal control problem and then investigate the optimality system. For this, we assume that
and
then the control system (Equation20
(20)
(20) ) can be re-written as
To proceed further, we also define that
, then for any
and
, we have
where
, is the Lipschitz constants implies that the function
is Lipschitz continuous, which ensure the existence analysis of the control system (Equation20
(20)
(20) ). In addition, we give the existence analysis for optimal value of the control measure in the result given below.
Theorem 4.1
For the objective functional J and the control subject to the control system, there exists optimal control value
, such that
.
Proof.
The integrand of the objective functional J is convex on the closed bounded control set U. Since, the model is linear in the controls function and is bounded by a linear system in the model state variables [Citation39], which shows that the optimal control exist.
We now find the optimality system with the aid of Pontryagin's maximum principle by following Pontryagin et al. [Citation40], thus we define the Hamiltonian for the proposed control system as (22)
(22) In the next theorem, we use the Hamiltonian H to present the characterization of adjoint variables and optimal control measures.
Theorem 4.2
For the given control measures and associated states s, a, c, r of the control system (Equation20
(20)
(20) ), there exists
, i = 1, 2, 3, 4, satisfying
(23)
(23) Moreover, the terminal conditions and optimal values of the control measures are
(24)
(24) and
(25)
(25)
Proof.
The adjoint system (Equation23(23)
(23) ) directly comes from the Pontryagin's Principle [Citation40], such that
with zero final time conditions.
In addition to get the optimal value of the controls measures, we solve the following equations
To get the compact form of the control measures, we then use the bound on the controls, we get the desired characterization of the control measures.
5. Numerical simulations
The numerical simulations of the proposed SARS-CoV-2 epidemiological model (Equation1(1)
(1) ) will be performed to present the graphical visualization of the analytical findings. We first present the graphical representation of the sensitivity for reproductive number and then the parameterizations of the model from real data of the novel disease of the Coronavirus. To visualize the results and to verify the theoretical results, we use the estimated values of the parameters retrieved from real data of SARS-CoV-2 virus reported cases.
5.1. Parameterization
For parameterization purposes, reported data of confirmed SARS-CoV-2 infected cases in Oman are used between January 2021 to May 2021. We estimate the value of parameters with the aid of Ordinary Least Square (OLS). The procedure of OLS is applied to minimize the cost representing the daily registered cases as well as the computed given in Equation (Equation26(26)
(26) ), with the corresponding relative error in the goodness of fit
(26)
(26) In Equation (Equation26
(26)
(26) ),
is used for the cumulative reported cases, while
represents the cumulative simulated cases. In order to estimate the model parameters, we first calculate the values of Π and µ. In 2021, Oman's average life expectancy was 78.16 years, while the total population was 4.52 million. Assuming that the limiting population is
in the absence of disease, the birth rate is
, where
per day, so we can obtain the death rate and birth rate as
and
per day, respectively. Then, we solve the proposed model using the fourth-order Runge–Kutta method in order to estimate the model's other epidemic parameters. Using the optimization algorithm, we update the parameter values to obtain a better agreement with the real data after evaluating the error. The process is repeated until the desired parameter value is reached, which meets the convergence criteria. Here, Figure shows the comparison between real data and model solution, while Table shows the associated values. The parameters are all measured in days.
Table 2. The fitted and assumed value of the epidemic parameters using (OLS) approach.
5.2. Sensitivity analysis
The main purpose of this section is to provide graphical representations of the sensitivity analysis of the basic reproductive number that was conducted using Equation (Equation9(9)
(9) ) as demonstrated in Table . As shown in Figure (a), we plot the most influential parameters along with their sensitivity indices on x-axis. It is shown in Figure (b) that β and γ have positive incidences and are the most influential parameters associated with the basic reproductive number. This contour plot describes the relationship between the reproductive number and β and γ. The graphical representation of the sensitivity analysis reveals that the substantial diminishing of the parameters with positive indices while improving the parameters with non-positive indices can lead to a decrease in the basic reproductive number to control the outbreak of the SARS-CoV-2 virus epidemic.
Figure 3. The plots represent the normalized sensitivity indices and contour plot of the epidemic parameters related with the reproductive number. In this case the parametric values are: ,
,
,
,
,
, p = 0.26, q = 0.79,
,
,
. (a) Normalized Sensitivity Indices, (b) Contour plot of
as a function of β and γ.

5.3. Validation of the model
To present the time dynamics of novel disease with the aid of the proposed epidemiological model, we use a numerical procedure of the fourth-order Runge–Kutta (RK) method [Citation41,Citation42] and consequently obtain the dynamics of the compartmental population of the model (Equation1(1)
(1) ) as given in Figure which respectively demonstrate the dynamics of susceptible, asymptomatic, symptomatic and the recovered compartments. We use the estimated parametric values and obtain the value of the reproductive number is 2.98. In this case, since
, the dynamics of various compartmental population of the model attain their associated endemic position which proves that the model stability at the disease endemic state as shown in Figure . This reveals that the infected population persists (see Figure (b,c)).
Figure 4. The graph visualizing the temporal dynamics of the compartmental model (Equation1(1)
(1) ), which shows that infected population will always exist whenever
. (a) Susceptible Population, (b) Asymptomatic Population, (c) Symptomatic Population and (d) Recovered Population.

On the other hand, to show the model dynamics at the infection-free state, we assume the value of model parameters as , v = 0.01,
,
,
,
, p = 0.36, q = 0.59,
,
and
. We calculate the reproductive number is
which is less than one. Therefore, we perturb the initial sizes of the various compartments s, a, c, r of the proposed model from
, so that the solutions curves reach disease-free equilibrium irrespective of its initial sizes in the long run as shown in Figure . This investigates that the model is stable around disease-free equilibrium
. The biological interpretation reveals that in the case of disease-free equilibrium, each solution curve of s, i.e. susceptible population will tend to its equilibrium position analyses that susceptible individuals will always exist as shown in Figure (a). The dynamics of asymptomatic and symptomatic compartments are depicted in Figure (b,c), which describes that the solution curves will tend to zero, whenever
implies that the infected population vanishes. Moreover, the dynamics of recovered individuals are shown in Figure (d) which shows that there will be always a recovered population. Thus, we conclude that the eradication of the novel disease continuously depends on the value of the threshold quantity. In addition, the system exhibits regular and irregular periodic oscillation, if we vary the value of bifurcation parameter from 2 to 6 as shown in Figure , which implies that in this case there exist an unstable equilibrium.
Figure 5. The graph visualizing the temporal dynamics of the compartmental population of model (Equation1(1)
(1) ) around disease-free equilibrium, where the parameters values are as:
,
,
,
,
,
, p = 0.36, q = 0.59,
,
,
and
. (a) Susceptible Population, (b) Asymptomatic Population, (c) Symptomatic Population and (d) Recovered Population.

5.4. Optimal control implementation
To visualize the control effect graphically, we use a similar procedure of numerical analysis with the help of fourth-order Runge–Kutta method. First, we solve the state system (Equation20(20)
(20) ) with initial compartmental population sizes by using the forward RK procedure, while for the solution of adjoint system, we use backward-RK method and the transversality conditions. Moreover, we used the parametric values as reported by Table and while the value of weight constants are assumed as:
,
,
and
and
. In addition, the time units are taken to be 0 to 100. By using the Matlab software, executing the proposed algorithm of Runge–Kutta method, we obtain the graphs as demonstrated in Figure , which describe the temporal dynamics of the susceptible, asymptomatic, symptomatic and recovered population with the implementation (blue dashed) and without the implementation (red dashed) of the control measure. The visualization clearly illustrate our objective in implementation: to reduce the number of infected population and to maximize the non-infected individuals (recovered & susceptible) as shown in Figure ( a–d), respectively. We noted that collectively, the implementation of the optimal control measures,
, i = 1, 2, 3 slow down the intensity of the transmission co-efficient β and γ, and strengthen the recovery of infected individuals which leads to the elimination of the current pandemic of novel disease. The difference between the two cases: with the implementation and without the implementation of the control measures are clearly visible and one can eliminate the contagious infection of Coronavirus, if we can apply the proposed control strategies in a true sense. Particularly, the disease transfer coefficient and rate of vaccination are very important in the reduction of the value of
. So using non-pharmaceutical and pharmaceutical interventions (face mask, avoiding gathering, vaccination, treatment and social distancing) are the best possible control measure to prevent the pandemic of the novel Coronavirus disease.
Figure 7. The graphs visualizing the time dynamics of the compartmental population of the proposed model with the optimal control measures implementation and with out the implementation of the control measure. (a) Susceptible Population, (b) Asymptomatic Population, (c) Symptomatic Population and (d) Recovered Population.

6. Conclusion
This study presented an epidemiological analysis and analysed the pandemic trend of SARS-CoV-2 virus transmission with optimal control strategies. We discussed the feasibility of the epidemic problem. It has been demonstrated that the considered epidemiological system is well-posed and biologically meaningful. The basic reproductive number of the model was also determined by examining the equilibria. Specifically, we discussed the positivity and boundedness of the model and calculated the infection-free state to obtain the infection-free reproduction number. Using normalized sensitivity analysis, we quantified the most sensitive parameters and their impact on disease prevention using normalized sensitivity analysis. Furthermore, we calculated the endemic equilibrium and performed a detailed stability analysis. A backward bifurcation phenomenon has been observed in the proposed model. To verify the analytical results, the proposed model was parameterized based on real data of the novel Coronavirus and simulated the problem. The disease will die out when
, but persists if
. We calculated the basic reproduction number as 1.406 using the estimated parameter values, which indicates that SARS-CoV-2 is endemic with the current control measures and immunization programme. Likevise, the sensitivity analysis shows that the transmission coefficient and vaccination got the highest sensitivity indices among other epidemic parameters, so vaccination and non-pharmaceutical interventions should be combined as an optimal control mechanism. As a result, using optimal control theory, we developed a control mechanism based on normalized sensitivity and stability and proposed a variety of non-pharmaceutical and pharmaceutical intervention strategies in the form of vaccinations, treatments, and personal protection. Combining these three control interventions can help eradicate novel Coronavirus contagious infections. To show the significance of the model and the work done, all theoretical results and the effects of controls are visualized.
While the model proposed yielded some interesting results, we will incorporate the stochastic nature by using stochastic differential equations in the future to capture more valuable information. Various fractional operators will also be used to discuss the dynamics of SARS-CoV-2 disease using the proposed model's associated fractional version.
Acknowledgments
The authors would like to thank the editor and reviewers for their excellent comments, which helped improve the quality of the manuscript.
Disclosure statement
No potential conflict of interest was reported by the author(s).
Additional information
Funding
References
- Rothan HA, Byrareddy SN. The epidemiology and pathogenesis of coronavirus disease (covid-19) outbreak. J Autoimmun. 2020;109:102433. doi: 10.1016/j.jaut.2020.102433
- Bai Y, Yao L, Wei T, et al. Presumed asymptomatic carrier transmission of covid-19. Jama. 2020;323(14):1406–1407. doi: 10.1001/jama.2020.2565
- Tang B, Bragazzi NL, Li Q, et al. An updated estimation of the risk of transmission of the novel coronavirus (2019-ncov). Infect Dis Model. 2020;5:248–255.
- Lin L, Hou Z. Combat covid-19 with artificial intelligence and big data. J Travel Med. 2020;27(5):taaa080. doi: 10.1093/jtm/taaa080
- Lu H. Drug treatment options for the 2019-new coronavirus (2019-ncov). Biosci Trends. 2020;14(1):69–71. doi: 10.5582/bst.2020.01020
- Bassetti M, Vena A, Giacobbe DR. The novel Chinese coronavirus (2019-ncov) infections: challenges for fighting the storm. Eur J Clin Investig. 2020;50(3):e13209. doi: 10.1111/eci.v50.3
- Al Harthi S, Al Osali M, Al Ismaili R, et al. Clinical characteristics of confirmed cases of covid-19 admitted at Al Nahdha Hospital Oman: a cross-sectional descriptive study. Cureus. 2021;13(8).
- Rihan FA, Alsakaji HJ, Rajivganthi C. Stochastic SIRC epidemic model with time-delay for covid-19. Adv Differ Equa. 2020;2020(1):502. doi: 10.1186/s13662-020-02964-8
- Din A, Li Y. Stationary distribution extinction and optimal control for the stochastic hepatitis b epidemic model with partial immunity. Phys Scr. 2021;96(7):074005. doi: 10.1088/1402-4896/abfacc
- Saha S, Samanta G. Modelling the role of optimal social distancing on disease prevalence of covid-19 epidemic. Int J Dyn Control. 2021;9(3):1053–1077. doi: 10.1007/s40435-020-00721-z
- Fadaei Y, Rihan FA, Rajivganthi C. Immunokinetic model for covid-19 patients. Complexity. 2022;2022:1–13. doi: 10.1155/2022/8321848
- Omame A, Onyenegecha IP, Raezah AA, et al. Co-dynamics of covid-19 and viral hepatitis b using a mathematical model of non-integer order: impact of vaccination. Fractal and Fract. 2023;7(7):544. doi: 10.3390/fractalfract7070544
- Kalula A, Mureithi E, Marijani T, et al. Optimal control and cost-effectiveness analysis of age-structured malaria model with asymptomatic carrier and temperature variability. J Biolog Dyn. 2023;17(1):2199766. doi: 10.1080/17513758.2023.2199766
- Kermack WO, McKendrick AG. Contributions to the mathematical theory of epidemics–i. 1927. Bull Math Biol. 1991;53(1-2):33–55.
- De Vries G, Hillen T, Lewis M, et al. A course in mathematical biology: quantitative modeling with mathematical and computational methods. SIAM; 2006. doi: 10.1137/1.9780898718256
- Agarwal P, Singh R. Modelling of transmission dynamics of Nipah virus (Niv): a fractional order approach. Phys A Stat Mech Appl. 2020;547:124243. doi: 10.1016/j.physa.2020.124243
- Zaman G, Kang YH, Jung IH. Stability analysis and optimal vaccination of an sir epidemic model. BioSystems. 2008;93(3):240–249. doi: 10.1016/j.biosystems.2008.05.004
- Khan T, Zaman G, Chohan MI. The transmission dynamic and optimal control of acute and chronic hepatitis b. J Biolog Dyn. 2017;11(1):172–189. doi: 10.1080/17513758.2016.1256441
- Kamyad AV, Akbari R, Heydari AA, et al. Mathematical modeling of transmission dynamics and optimal control of vaccination and treatment for hepatitis b virus. Comput Math Methods Med. 2014;2014:1–15. doi: 10.1155/2014/475451
- Nwankwo A, Okuonghae D. A mathematical model for the population dynamics of malaria with a temperature dependent control. Differ Equ Dyn Syst. 2019;1–30.
- Omame A, Okuonghae D, Umana R, et al. Analysis of a co-infection model for HPV-TB. Appl Math Model. 2020;77:881–901. doi: 10.1016/j.apm.2019.08.012
- Kang S, Hou X, Hu Y, et al. Dynamic analysis and optimal control considering cross transmission and variation of information. Sci Rep. 2022;12(1):18104. doi: 10.1038/s41598-022-21774-4
- Teklu SW, Terefe BB. Mathematical modeling analysis on the dynamics of university students animosity towards mathematics with optimal control theory. Scient Rep. 2022;12(1):11578. doi: 10.1038/s41598-022-15376-3
- W. C. C. for Infectious Disease Modelling, M. C. for Global Infectious Disease Analysis, A. L. J. I. for Disease, E. Analytics, and I. C. London: Impact of non-pharmaceutical interventions (npis) to reduce covid-19 mortality and healthcare demand. 2020.
- Kucharski AJ, Russell TW, Diamond C, et al. Early dynamics of transmission and control of covid-19: a mathematical modelling study. Lancet Infect Dis. 2020;20(5):553–558. doi: 10.1016/S1473-3099(20)30144-4
- Kuniya T. Prediction of the epidemic peak of coronavirus disease in japan. J Clin Med. 2020;9(3):789. doi: 10.3390/jcm9030789
- Stutt RO, Retkute R, Bradley M, et al. A modelling framework to assess the likely effectiveness of facemasks in combination with ‘lock-down’ in managing the covid-19 pandemic. Proc R Soc A. 2020;476(2238):20200376. doi: 10.1098/rspa.2020.0376
- Samui P, Mondal J, Khajanchi S. A mathematical model for covid-19 transmission dynamics with a case study of india. Chaos Solit Fractals. 2020;140:110173. doi: 10.1016/j.chaos.2020.110173
- Saha S, Samanta G, Nieto JJ. Epidemic model of covid-19 outbreak by inducing behavioural response in population. Nonlinear Dyn. 2020;102(1):455–487. doi: 10.1007/s11071-020-05896-w
- Rihan F, Alsakaji H. Dynamics of a stochastic delay differential model for covid-19 infection with asymptomatic infected and interacting people: case study in the uae. Res Phys. 2021;28:104658.
- Saha S, Samanta G, Nieto JJ. Impact of optimal vaccination and social distancing on covid-19 pandemic. Math Comput Simul. 2022;200:285–314. doi: 10.1016/j.matcom.2022.04.025
- Saha S, Dutta P, Samanta G. Dynamical behavior of sirs model incorporating government action and public response in presence of deterministic and fluctuating environments. Chaos Solit Fractals. 2022;164:112643. doi: 10.1016/j.chaos.2022.112643
- Rihan FA, Udhayakumar K, Sottocornola N, et al. Stability and bifurcation analysis of the caputo fractional-order asymptomatic covid-19 model with multiple time-delays. Int J Bifurc Chaos. 2023;33(02):2350022. doi: 10.1142/S0218127423500220
- Van den Driessche P, Watmough J. Reproduction numbers and sub-threshold endemic equilibria for compartmental models of disease transmission. Math Biosci. 2002;180(1-2):29–48. doi: 10.1016/S0025-5564(02)00108-6
- Diekmann O, Heesterbeek JAP, Metz JA. On the definition and the computation of the basic reproduction ratio r 0 in models for infectious diseases in heterogeneous populations. J Math Biol. 1990;28(4):365–382. doi: 10.1007/BF00178324
- Khajanchi S, Bera S, Roy TK. Mathematical analysis of the global dynamics of a HTLV-I infection model considering the role of cytotoxic t-lymphocytes. Math Comput Simul. 2021;180:354–378. doi: 10.1016/j.matcom.2020.09.009
- Castillo-Chavez C, Song B. Dynamical models of tuberculosis and their applications. Math Biosci Eng. 2004;1(2):361–404. doi: 10.3934/mbe.2004.1.361
- Verhulst F. Nonlinear differential equations and dynamical systems. Springer Science & Business Media; 2006.
- Fleming W, Rishel R, Marchuk G, et al. Deterministic and stochastic optimal control. 1975. (Applications of mathematics).
- Pontryagin LS. Mathematical theory of optimal processes. CRC press; 1987.
- Atkinson K, Han W, Stewart DE. Numerical solution of ordinary differential equations. Wiley; 2011.
- Kovalnogov VN, Simos TE, Tsitouras C. Runge–Kutta pairs suited for sir-type epidemic models. Math Methods Appl Sci. 2021;44(6):5210–5216. doi: 10.1002/mma.v44.6