Abstract
Purpose
Neurodegenerative motor diseases (NMDs) have devastating effects on the lives of patients and their loved ones, in part due to the impact of neurologic abnormalities on speech, which significantly limits functional communication. Clinical speech researchers have thus spent decades investigating speech features in populations suffering from NMDs. Features of impaired articulatory function are of particular interest given their detrimental impact on intelligibility, their ability to encode a variety of distinct movement disorders, and their potential as diagnostic indicators of neurodegenerative diseases. The objectives of this scoping review were to identify (1) which components of articulation (i.e. coordination, consistency, speed, precision, and repetition rate) are the most represented in the acoustic literature on NMDs; (2) which acoustic articulatory features demonstrate the most potential for detecting speech motor dysfunction in NMDs; and (3) which articulatory components are the most impaired within each NMD.
Method
This review examined literature published between 1976 and 2020. Studies were identified from six electronic databases using predefined key search terms. The first research objective was addressed using a frequency count of studies investigating each articulatory component, while the second and third objectives were addressed using meta-analyses.
Result
Findings from 126 studies revealed a considerable emphasis on articulatory precision. Of the 24 features included in the meta-analyses, vowel dispersion/distance and stop gap duration exhibited the largest effects when comparing the NMD population to controls. The meta-analyses also revealed divergent patterns of articulatory performance across disease types, providing evidence of unique profiles of articulatory impairment.
Conclusion
This review illustrates the current state of the literature on acoustic articulatory features in NMDs. By highlighting the areas of need within each articulatory component and disease group, this work provides a foundation on which clinical researchers, speech scientists, neurologists, and computer science engineers can develop research questions that will both broaden and deepen the understanding of articulatory impairments in NMDs.
Introduction
Neurodegenerative motor diseases (NMDs) are a heterogeneous group of diseases characterised by progressive neuronal loss in the central and peripheral nervous systems, affecting motor function (Duffy, Citation2013). Dysarthria, which is a speech motor disorder resulting from loss of articulator muscle strength or control, is a common symptom of NMDs (Duffy, Citation2013), and the consequent communication disturbances significantly impact patient quality of life (Hartelius, Elmberg, Holm, Lovberg, & Nikolaidis, Citation2008). The type and severity of dysarthria is, therefore, often examined in the assessment and differential diagnosis of neurologic disorders (Duffy, Citation2013; Kent, Kent, Weismer, & Duffy, Citation2000). Furthermore, a growing number of studies suggest that speech impairments may be among the most informative motor abnormalities for detecting the onset of neurological dysfunction or tracking the rate of disease progression (Robin et al., Citation2020). Exploring the efficacy of candidate speech acoustic biomarkers has thus become an active area of research for the purposes of improving early detection, disease classification, and progress monitoring during clinical trials (Carmichael, Citation2014; Gunduz, Citation2019; Gutz, Wang, Yunusova, & Green, Citation2019; Orozco-Arroyave et al., Citation2016; Tsanas, Little, McSharry, Spielman, & Ramig, Citation2012; Wang, Kothalkar, Cao, & Heitzman, Citation2016).
Aberrant acoustic speech features are loosely linked to their physiologic origins, which involve dysfunction of one or several of the four speech subsystems (i.e. articulatory, phonatory, respiratory, or resonatory). While phonatory, resonatory, respiratory, and prosodic deficits can significantly limit communicative capacity, impairments to articulatory function have a highly detrimental impact on speech intelligibility (De Bodt, Hernandez-Diaz Huici, & van De Heyning, Citation2002; Kent et al., Citation1989; Lee, Hustad, & Weismer, Citation2014; Rong, Yunusova, Wang, & Green, Citation2015; Turner, Tjaden, & Weismer, Citation1995; Weismer, Jeng, Laures, Kent, & Kent, Citation2001). Articulatory abnormalities are also thought to encode unique motor symptoms (e.g. hypokinesia, hyperkinesia, ataxia) that may correspond with underlying neuropathologies of movement disorders (Guenther, Citation2016). For instance, damage to the cerebellum, which is often found in speakers with ataxic dysarthria, has been associated with articulatory discoordination (Ackermann, Citation2008). Damage to the basal ganglia in speakers with hypokinetic dysarthria, on the other hand, can affect the size and speed of articulatory movement (Hirose, 1986). These presumed linkages between neuropathology and speech motor behaviour make articulatory features an appealing diagnostic target to further explore for neurologic disease. Indeed, prior work has already demonstrated the efficacy of articulatory movement features as diagnostic indicators of movement disorders such as bulbar amyotrophic lateral sclerosis (Green et al., Citation2013), apraxia of speech in neurodegenerative conditions (Takakura et al., Citation2019), and the nonfluent variant of primary progressive aphasia (Cordella, Dickerson, Quimby, Yunusova, & Green, Citation2017).
Given their diagnostic potential, speech features are increasingly being used to train machine learning models for detecting the presence of NMDs (Robin et al., Citation2020). Data-driven feature extractors (e.g. OpenSmile; Eyben, Wollmer, & Schuller, Citation2010) are capable of extracting thousands of features—many of which are uninterpretable—from a single speech recording and, therefore, might be viewed as the preferred method for feature discovery. However, domain knowledge (i.e. expert knowledge of a specific discipline) and feature interpretability have been increasingly recognised for reducing model bias, refining diagnostic model performance, and furthering scientific understanding (Childs & Washburn, Citation2019; Gunning & Aha, Citation2019; Tu, Berisha, & Liss, Citation2017). Moreover, recent work has found automatically extracted speech features to have low reliability for dysarthria assessment (Stegmann et al., Citation2020). To that end, the rationale for the current review paper is in the context of identifying domain-specific features that provide inference ability. Knowledge regarding impaired articulatory function has the potential to advance diagnostic model development by (1) providing guidance regarding features that may be the most likely candidates for detecting speech abnormalities in NMDs (Anand, Bell, & Hughes, Citation1995; Berisha, Sandoval, Utianski, Liss, & Spanias, Citation2013; Gupta et al., Citation2016) and (2) ensuring the inclusion of participants with characteristics that represent the full range of diversity in the target population. Taken together, there is a critical need to identify acoustic articulatory features to improve the diagnostic applications of artificial intelligence and scientific understanding of NMDs.
A proposed framework to guide the investigation of interpretable articulatory features
To guide the organisation of articulatory features for this review, we grouped features into five components: coordination, consistency, speed, precision, and repetition rate (Rowe, Gutz, Maffei, & Green, Citation2020; Rowe, Stipancic, Lammert, & Green, Citation2021; Rowe & Green, Citation2019). These groupings are intended to be comprehensive but are not exhaustive (i.e. they do not characterise all the variations of articulatory motor impairments) nor mutually exclusive (i.e. one articulatory feature could presumably represent multiple components). Our affiliation of a particular articulatory feature with a particular component was aligned with each author’s stated intention in the reviewed source articles. For example, Tykalova and colleagues (Citation2017) measured voice onset time as a measure of coordination, and Lam and Tjaden (Citation2016) measured vowel space area as a measure of articulatory precision (see ). The clinical validity of these components is supported by their widespread use in movement disorders clinics to characterise a wide diversity of movement disorders. As such, they have informed one of the most established speech motor impairment classification systems developed by Darley, Aronson, and Brown (DAB) (Darley, Aronson, & Brown, Citation1969a, Citation1969b)—a system based on the hypothesised relationships between atypical speech patterns and distinct neurological and/or pathophysiological deficits (Ackermann, Citation2008; Darley et al., Citation1969a, Citation1969b; Duffy, Citation2013; Guenther, Citation2016; Hirose, 1986; Kent et al., Citation2000). With the increasing amount of research on acoustic measures of speech function in dysarthria, the proposed five-component scheme for characterising articulatory motor impairments can help guide the interpretation of new and existing features within the context of common clinical descriptors for movement disorders.
Figure 1. Dysarthria subtypes for each neurodegenerative motor disease in the current review, their perceptual articulatory characteristics based on a widely used taxonomy of speech motor disorders (ordered from most severe to least severe, with * indicating more severely impaired than other dysarthria subtypes), and the corresponding areas of impairment based on our framework of articulatory components.
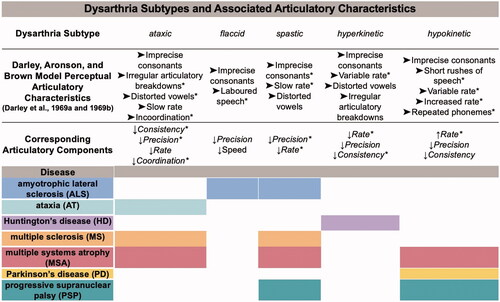
In this scoping review, we aimed to summarise four decades of literature on acoustic features of articulatory performance with the goals of identifying (1) which components of articulation (i.e. coordination, consistency, speed, precision, or repetition rate) are the most represented in the speech acoustic literature on NMDs; (2) which acoustic articulatory features demonstrate the most potential for detecting speech motor dysfunction in NMDs; and (3) which articulatory components are the most impaired within each NMD. Based on recommendations made by Munn and colleagues (2018), a scoping review is an appropriate review method for this paper because we sought to identify the available evidence on acoustic features in NMDs, clarify key concepts underlying acoustic-based articulatory features in the literature by categorising them into different components of articulation, and determine where the gaps are in the acoustic literature base in NMDs (Munn et al., Citation2018).
Method
Using a five-stage framework, Arksey and O’Malley (Citation2005) outline the process of a scoping review that offers transparency and permits the replication of the search strategy used in the review. This scoping review employs the five stages of the framework: A. Identifying the Research Questions; B. Identifying Relevant Studies; C. Study/Feature Selection; D. Charting the Data; and E. Collating, Summarising, and Reporting the Results (Arksey & O’Malley, Citation2005). This review also followed the Preferred Reporting Items for Systematic Reviews and Meta-Analyses (PRISMA) Extension for Scoping Reviews guidelines (Tricco et al., Citation2018).
Identifying the research questions
The primary focus of this review was to evaluate the range of acoustic articulatory features that has been used to investigate the most common NMDs. Our first research question (RQ1) focussed on the articulatory components that are most represented in the acoustic literature, our second research question (RQ2) focussed on the specific features that demonstrate the most potential for detecting speech motor dysfunction in NMDs, and our third research question (RQ3) focussed on the articulatory components that are the most impaired within each NMD and, therefore, may be important for differential diagnosis.
Identifying relevant studies
Studies were identified by searching the following six electronic databases: MEDLINE, CINAHL, PsycINFO, PubMed, IEEE Xplore, and Google Scholar (to identify sources within grey literature). The most recent search was conducted on December 1, 2020. The search strategy was based on the methodology (i.e. acoustic analysis), speech disorder (i.e. dysarthria), and population (i.e. neurodegenerative motor disease) of interest for this review. Thus, the main search terms and corresponding operational definitions included acoustics/speech acoustics, defined as any analysis of the speech signal waveform recorded with a microphone, including amplitude, duration, or spectral properties of the waveform; dysarthria/dysarthric speech, defined as speech difficulties due to weakened muscles; articulation, defined as features that represent imprecise consonants, distorted vowels, incoordination, irregular articulatory breakdowns, and reduced speed and range of articulatory movement; and neurodegenerative disease, defined as a heterogeneous group of disorders characterised by the progressive degeneration of the central or peripheral nervous systems related to movement generation.
When appropriate, the Medical Subject Heading (MeSH) terms were “exploded” (i.e. made broader than the scope of the review) to retrieve citations that carried more specific MeSH terms. Individual search terms were adapted for each database to accommodate differences in subject headings. Key search terms included (“acoustics” OR “speech acoustics”) AND (“dysarthria” OR “dysarthric speech”) AND (“neurodegenerative diseases”) AND (“articulation”). Reliability of the abstract selection (indexed by percent agreement) was based on two independent raters on 50% of the abstracts identified in the primary search. Percent agreement was 92.6% for the subset of abstracts. Discrepancies were resolved by consensus.
Study/feature selection
Using the search terms described above, a total of 1230 articles were identified. Since the terms were exploded and included conditions other than neurodegenerative disease, such as stroke or traumatic brain injury stringent selection criteria were determined and applied a priori. Studies were excluded if they (1) had no abstract; (2) were not human studies; (3) were not focussed on adults; (4) were not peer-reviewed; (5) were not in English; (6) did not use acoustic analyses (e.g. only used perceptual analyses); (7) did not investigate the articulatory subsystem (e.g. only examined phonatory features); (8) did not investigate NMDs (e.g. only investigated acquired disorders, non-progressive diseases, or diseases with initial symptoms that are not motor-based, such as dementia or Alzheimer’s disease); (9) were classified as a review, report, or book; or (10) were a methodology study (e.g. assessed the accuracy of a speech recognition algorithm). While studies were excluded if they were not written in English, we included studies with speakers of languages other than English, as recent work has reported similar deficits across multiple languages in individuals with dysarthria (Rusz, Hlavnicka, et al., Citation2021). Furthermore, including studies with languages other than English expands the generalisability of our findings and provides a wider representation of the current literature.
As mentioned previously, the results of our review were framed within the five proposed components of articulatory motor control (i.e. coordination, consistency, speed, precision, or repetition rate) (see ). Importantly, features were first identified as being from the articulatory subsystem (as opposed to the respiratory, resonatory, phonatory, or prosodic subsystem). Each feature was then assigned to a component based on the intended use by the author. If the specific terms in our classification scheme were not explicitly used in a source article, we relied on the presence of terms with similar meaning, such as “articulatory variability” for consistency or “articulatory distinctiveness” for precision. Several notable distinctions were made regarding whether features were primarily characteristic of the articulatory motor subsystem or another subsystem: (1) variability across repeated utterances (articulatory consistency) versus variability in continuous speech (often involved in rhythm) (Grabe & Low, Citation2008); (2) formant duration of consonant-vowel or diphthong transitions (articulatory speed) versus vowel duration in continuous speech (often involved in stress) (Crystal & House, Citation1982); and (3) rate of repeated utterances (repetition rate) versus speaking/articulatory rate (can be confounded by pausing or rhythm, respectively) (Allen, Citation1975; Grosjean & Collins, Citation1979). Speech acoustics are, nevertheless, at the confluence of multiple neural and biomechanic systems and co-dependency among performance measures is inevitable. For example, spectral coarticulation was classified as a coordination feature because it represents the appropriate temporal alignment of articulatory movements, but deficits in spectral coarticulation also have implications for precision. However, the extent to which a speech measure represents the status of one or more speech subsystem or divergent impairments within a subsystem (e.g. breathy versus strained voice) is a critical question for advancing speech diagnostics as well as explanatory models of speech. For the purposes of this review, the proposed framework offers an organisational structure for the literature on articulatory impairments. Additional research is needed to better understand the validity of specific acoustic features and their value for identifying distinct movement disorders and the underlying neurological and/or pathophysiological deficits.
Figure 2. Definitions of the five framework components of articulatory motor and examples of acoustic features that represent each component.
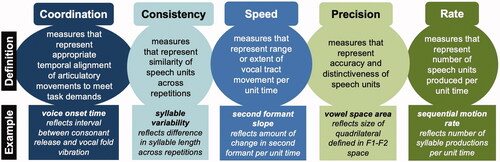
Based on our exclusion and inclusion criteria, 165 studies were identified as relevant to our research questions and were subsequently brought to full review. Since our first research question was focussed on the frequency of articulatory features in the literature, articles were excluded if they used feature sets that did not allow for assessment of (1) the individual features of interest (e.g. “blackbox” classification studies) or (2) the population of interest (e.g. aggregate data that included individuals with multiple diagnoses, undiagnosed speakers, or speakers of an unknown diagnosis). Since our second and third questions were focussed on the level of impairment of individual features within and across NMDs, additional studies/features were excluded if they (3) did not have a control group; (4) did not include sufficient statistics to calculate an effect size (e.g. no means and standard deviations); (5) only included features that had been investigated in one population; or (6) only included features that had been investigated in one study. The process of article selection followed the PRISMA Extension for Scoping Reviews guidelines (Tricco et al., Citation2018) (see ). Reliability of article selection (indexed by percent agreement) was based on two independent raters on 50% of the full articles. Percent agreement was 94.2% for the subset of articles. Discrepancies were resolved by consensus. Reliability of feature categorisation into the five components (indexed by percent agreement) was based on two independent raters on 100% of the features included in the study. Percent agreement was 90.6%. Discrepancies were resolved by consensus.
Charting the data
For each study, a brief quality assessment was performed by the first author. The key areas for quality assessment included specifying demographics (i.e. age and sex), describing clinical history (i.e. disease duration and speech severity), addressing incomplete outcome data (e.g. providing reasons for study attrition), documenting comorbidities (e.g. cognitive impairments or aphasia), and conducting appropriate statistical analyses (e.g. omnibus testing and correcting for multiple comparisons).
Collating, summarizing, and reporting the results
The fifth and final stage of the five-stage scoping review framework involves collating, summarising, and reporting the results. Demographic and clinical characteristics (i.e. medical diagnosis and speech diagnosis of the participants, sample size, age, sex, disease duration, and speech severity) were extracted from all 126 studies included for RQ1 and in the subset of 89 studies included for RQs 2 and 3, which can be found in . The data extraction for RQ1 included (1) diagnosis and (2) acoustic features investigated in study. The additional data extraction for RQs 2 and 3 included (1) statistics necessary for effect size calculations (i.e. means and standard deviations, t values, F values, and number per group); (2) speech stimuli used to derive acoustic features; (3) brief methodology used to extract acoustic features; and (4) pathophysiological significance of acoustic features, when available. Effect sizes were calculated for all comparisons regardless of significance. All effect sizes were then combined regardless of directionality. For most comparisons, speakers in the disease groups were more impaired than controls. There was, however, a subset of instances where the mean values for the disease group indicated that they were less impaired than controls, but these effect sizes were very small and were not statistically significant.
Table I. Demographics and clinical characteristics of participants across studies for RQ1 and RQs 2 and 3.
Result
This scoping review investigated a total of 126 studies for RQ1. See Supplementary Material for a complete list of the studies investigated for the review. Performance on a total of 24 acoustic features from 89 articles (a subset of the 126 included for RQ1) was analysed for the meta-analyses in RQs 2 and 3. Within the accepted studies, an initial total of nine NMDs were examined: ALS; Huntington’s disease (HD); cerebellar ataxia (CA); Friedreich’s ataxia (FA); spinocerebellar ataxia (SCA) variants 2/3/6/7/8; multiple sclerosis (MS); multiple system atrophy (MSA), both the unified syndrome as well as the parkinsonism variant; PD; and progressive supranuclear palsy (PSP). Because CA, FA, and the SCA variants were so infrequently studied, they were combined in this paper and are herein referred to as ataxia (AT). These seven movement disorders are known to be the most common NMDs worldwide (Erkkinen, Kim, & Geschwind, Citation2018; Schaffert & Carter, Citation2020) and have been shown to result in different perceptual clusters of articulatory characteristics (Darley et al., Citation1969a, Citation1969b) (see ).
Consistent with the high prevalence of PD (Parkinson’s Foundation, Citation2020), speakers with PD were the most investigated, while speakers with MSA, which has one of the lowest prevalence rates (Schrag, Ben-Shlomo, & Quinn, Citation1999), were the least commonly investigated (see and ). The findings for each research question are discussed below.
Figure 4. Pie chart illustrating the number of studies investigating each neurodegenerative motor disease. Studies were counted more than once if they investigated more than one disease. The number in parentheses represents the percentage of the 126 studies that investigated that specific disease.
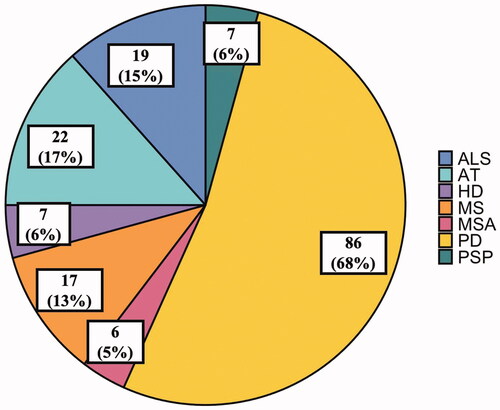
Research question 1. Which articulatory components (i.e. coordination, consistency, speed, precision, or repetition rate) are the most represented in the acoustic literature on NMDs?
Based on the 126 articles included for RQ1, precision was the most represented articulatory component in the acoustic literature on NMDs (190 occurrences) and consistency was the least represented (37 occurrences), with coordination, repetition rate, and speed similarly underrepresented (41, 48, and 48 occurrences, respectively) (see ). Importantly, precision was also represented by the greatest number of unique features (46), while the other components consisted of significantly fewer features (six for coordination, four for consistency, six for speed, and two for repetition rate) (see Tables 2a, 2b, and 3 in Supplementary Material). Additionally, the results demonstrated that the representation of articulatory components differed based on the NMD investigated. Indeed, precision was the most represented for the majority of NMDs (19 occurrences for ALS, 26 occurrences for MS, four occurrences for MSA, 125 occurrences for PD, and five occurrences for PSP) (see ). However, consistency and repetition rate were most frequently studied in individuals with AT (11 and 12 occurrences, respectively), and consistency and precision were most frequently studied, though only by one occurrence, in HD (three occurrences each) (see ).
Research question 2. Which acoustic articulatory features demonstrate the most potential for detecting speech motor dysfunction in NMDs?
A total of 63 unique acoustic articulatory features were included in this review, but 39 of those features did not meet the inclusion criteria for the meta-analysis (see Table IIa in Supplementary Material). Thus, 24 unique features from 89 articles were included for RQs 2 and 3 (see Tables IIb and III in Supplementary Material). Based on the features included in the meta-analysis, vowel dispersion/distance and stop gap duration exhibited a mean Cohen’s d effect size (across all disease groups) greater than 1.5 (d = 1.95 and d = 1.80, respectively) (see ). In contrast, second formant duration and first spectral moment coefficient exhibited a mean effect size smaller than .6 (d = 0.26 and d = 0.43, respectively) (see ).
Figure 6. Forest plot illustrating effect sizes (disease group compared to healthy controls) for each articulatory feature. The number of participants investigated in each disease is displayed above the corresponding dot. The total number of participants across all diseases is displayed in brackets to the right of the features. Positive effect sizes indicate that the disease group exhibited poorer performance compared to controls, while negative effect sizes indicate that the disease group performed better than controls.
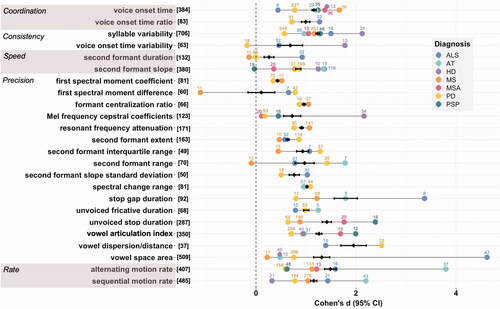
Research question 3. For each NMD, which articulatory components are the most impaired?
It should be noted that due to limited number of datapoints (e.g. only one study looked at speed features in speakers with MSA), we were unable to calculate confidence intervals for every effect size mean. However, based on inspection of the mean effect sizes and confidence intervals we were able to calculate, the findings revealed divergent patterns of articulatory performance within and across NMDs (see ). We considered the articulatory performance of each NMD population compared to both (1) the other components within the specific diagnosis group and (2) the articulatory performance of the other NMD populations. We found that speakers with ALS exhibited the greatest impairments in precision, speakers with AT exhibited the greatest impairments in repetition rate, speakers with HD exhibited the greatest impairments in consistency, and speakers with MS exhibited the greatest impairments in coordination. We also noted that speakers with MSA exhibited equal impairments in coordination, consistency, precision, and repetition rate; speakers with PD exhibited equal (and minimal) impairments across all components; and, compared to the other components within PSP, speakers with PSP exhibited primarily impaired consistency and precision.
Discussion
This study reviewed the current state of the literature on acoustic articulatory features in NMDs, with the overarching goals of determining (1) the articulatory components that are the most frequently investigated in NMDs, (2) the articulatory features that are the most effective in differentiating NMDs from healthy controls, and (3) the articulatory components that are most impaired in each NMD. Our findings revealed the depth and breadth of the present research base and laid the groundwork for identifying the domain knowledge necessary for improving diagnostic models for individuals with NMDs.
Comprehensively sampling across different articulatory components in NMDs (research question 1)
Identifying robust markers of abnormal articulatory function is a high research priority because of their (1) strong influence on speech intelligibility (De Bodt et al., Citation2002; Kent et al., Citation1989; Lee et al., Citation2014; Rong et al., Citation2015; Turner et al., Citation1995; Weismer et al., Citation2001), (2) hypothesised links to distinct neurological and/or pathophysiological deficits (Ackermann, Citation2008; Guenther, Citation2016; Hirose, 1986), and (3) previously demonstrated diagnostic efficacy for a number of movement disorders (Cordella et al., Citation2017; Green et al., Citation2013; Takakura et al., Citation2019). These markers thus have the potential to improve diagnostic models for speakers with NMDs. Our review, however, revealed expansive gaps in the literature on all of the articulatory components except precision.
The focus on precision in the acoustic literature is not unfounded, as deficits in precision have been documented in nearly every dysarthria subtype (Darley et al.,Citation1969a, Citation1969b). Furthermore, in contrast to the small subset of features representing other articulatory components, precision can be represented by a plethora of features assessing the distinctiveness of speech sounds within three types of targets: vowels, consonants, and consonant-vowel transitions. Within each type of target, the same articulatory configuration can be acoustically measured in multiple ways. For example, for consonants, the inability to maintain appropriate vocal tract constriction can be indexed by length of frication, length of stop gap, or spectral moment differences. It is important to note that despite—or perhaps in light of—the abundance of precision features, many of them lack standardisation, with the exception of the vowel space features. More research is, therefore, needed to test the reliability and validity of existing features. Particular focus should be given to consonant features, as previous work has suggested that consonants may have a disproportionate impact on intelligibility (Owren & Cardillo, Citation2006).
Compared to precision, the other four articulatory components were far less represented in the acoustic literature. The reasons for this underrepresentation may differ depending on the component. The paucity of investigation of coordination, for example, may arise from the many-to-one mapping of articulatory movements to acoustic output (e.g. uncoordinated lip movement during the production of /p/ will not necessarily alter the acoustic result) (Stevens, Citation1972), which limits feature interpretation and impedes the generation of valid acoustic features. Feature development for coordination may also be hindered by inadequate conceptual and operational definitions across and even within disciplines such as computer science, speech science, neurology, and linguistics.
While there were also far fewer features representing speed, repetition rate, and consistency, the lack of studies examining these articulatory components was surprising given their relative ease of measurement (e.g. second formant slope, syllables per second, and between-repetition variability in syllable length, respectively) and their diagnostic utility for different neurologic diseases. Prior work has shown that repetition rate, in particular, can distinguish speakers across a variety of aetiologies (e.g. ALS, AT, PD) from healthy controls with high sensitivity (Nishio & Niimi, Citation2006; Schalling, Hammarberg, & Hartelius, Citation2007; Tjaden & Watling, Citation2003; Ziegler & Wessel, Citation1996).
The disproportionate attention on precision is problematic because it does not provide a sufficient amount of information to distinguish how articulator motor impairments manifest in different diseases. For example, if one considered only precision when characterizing articulation in PD, it may be difficult to distinguish speakers with PD from other diseases since imprecision is common across many dysarthria subtypes (Darley et al., Citation1969a, Citation1969b). However, considering other abnormalities in addition to precision, such as repetition rate, may help to distinguish PD since speakers with this condition have been found to maintain a normal repetition rate through truncated movements (Blanchet & Snyder, Citation2009; Kim et al., Citation2009; Rowe et al., Citation2020). Developing phenotypes that elucidate the articulatory components that are impaired and those that are spared may thus be critical for improving diagnostic accuracy. Identifying similar multidimensional profiles for other NMDs, however, requires broadening and deepening our exploration of articulatory components across all NMD populations.
Identifying potential markers of speech motor dysfunction in NMDs (research question 2)
Our findings revealed several promising features of articulatory dysfunction within the existing literature on acoustic articulatory features. When examining NMDs as a whole, the meta-analysis for RQ2 demonstrated large effect sizes for a subset of features (e.g. vowel dispersion/distance, stop gap duration, alternating motion rate, unvoiced stop duration) (illustrated by the black diamonds in ), indicating their potential to detect articulatory deficits in dysarthria in general. The subset of features, however, differed depending on the disease type (e.g. vowel space area for ALS, sequential motion rate for AT) (illustrated by the coloured dots in ), which suggests that articulation may be differentially impaired across diseases.
Among the five components, precision exhibited the greatest range in effect size values across all acoustic features, in part because there were significantly more features representing precision compared to any other component. As previously mentioned, features of precision assess the distinctiveness of vowels, consonants, and consonant-vowel transitions. For vowels, reductions in vowel space (e.g. vowel articulation index or vowel space area) reflect the inability to achieve the full range of movement required for distinct vowel production. For consonants, reductions in duration (e.g. unvoiced fricative duration or unvoiced stop duration) or intensity (e.g. first spectral moment coefficient or spectral change range) of spectral energy reflect the inability to maintain sufficient articulatory closure and/or, in the case of spectral moments, inaccurate place and degree of constriction. For consonant-vowel transitions, reductions in formant “movement” (e.g. second formant extent or second formant interquartile range) reflect the inability to achieve appropriate vocal tract movement between sounds. The concept of “articulatory imprecision” is, therefore, employed broadly across different features and articulatory gestures. The overall findings suggest that features reflecting changes in vowel space and in duration of spectral energy are promising for detecting articulatory imprecision across disease types. Further research is, nevertheless, needed to validate acoustic features of precision using kinematic approaches and to determine whether our findings hold with a larger and more diverse sample.
A wide range of effect sizes was also noted across NMDs for nearly every feature, which implies that some features may be uniquely sensitive to specific disease types (see ). For example, our findings revealed that alternating motion rate is most noticeably impaired in speakers with AT, while vowel space area is particularly reduced in speakers with ALS. However, many features (i.e. voice onset time ratio, second formant standard deviation, spectral change range, resonant frequency attenuation, first spectral moment coefficient, first spectral moment difference, and formant centralisation ratio) have only been studied in two populations. Furthermore, based on our literature search, there are several features that, to our knowledge, have never been studied in certain diseases, such as second formant extent in individuals with AT. Additional work is, therefore, needed to examine performance on these features across more disease types.
Aside from further investigation into existing features, more focus is needed on developing new acoustic features reflecting components other than precision. For instance, while second formant slope has been shown to be highly sensitive to several NMD populations (Kent & Kim, Citation2003; Kim et al., Citation2009; Yunusova et al., Citation2012), other features representing speed that were not included in the meta-analysis, such as spectral change rate, may be more sensitive to speed in certain other populations. Dense sampling across multiple different features and disease types is integral to guiding engineers and speech scientists in selecting the features that best detect speech impairment in specific NMDs. Importantly, most findings are based on cross-sectional rather than longitudinal data. Thus, further work is needed to examine the stability of articulatory features over time, as such information is essential for determining the utility of speech as a diagnostic tool or outcome measure in a clinical trial.
Considering divergent articulatory patterns in the differential diagnosis of NMDs (research question 3)
The DAB model posits that each disease type has a different presumed neurological and/or pathophysiological basis (Darley et al., Citation1969a, Citation1969b), which hypothetically results in a unique articulatory phenotype. Evidence for such phenotypes emerged, to some degree, upon collapsing the meta-analysis results from RQ2 across features within each component for each NMD group (see ). While findings are preliminary since we were unable to adequately control for severity, our analyses revealed divergent patterns of articulatory performance based on disease type.
Many of the NMD populations exhibited articulatory characteristics consistent with those identified in the DAB model for their corresponding dysarthria subtype (see and ) (Darley et al., Citation1969a, Citation1969b). For speakers with ALS, impairments in precision, speed, and repetition rate align with the DAB characteristics of imprecise consonants / distorted vowels, slow rate, and labored speech, which Darley and colleagues observed in mixed spastic-flaccid dysarthria (Darley et al., Citation1969a, Citation1969b). Likewise, for speakers with HD, primary impairments in consistency align with the DAB characteristic of irregular articulatory breakdowns, which the authors observed in hyperkinetic dysarthria (Darley et al., Citation1969a, Citation1969b).
Among two articulatory deficits that the DAB model associates with ataxic dysarthria (i.e. incoordination and slow rate) (Darley et al., Citation1969a, Citation1969b), only rate was notably impaired in speakers with AT. Moreover, only coordination was notably impaired in speakers with MS, which the DAB model considers to be a mixed spastic-ataxic dysarthria (Darley et al., Citation1969a, Citation1969b). These findings provide support for the idea that coordination is a multidimensional construct that may not be well-represented by the single measure of coordination used in this study (i.e. voice onset time) (Caruso et al., Citation1988).
For speakers with PSP, primary impairments in precision and consistency align with the DAB characteristics of imprecise consonants / distorted vowels and variable rate, which are seen in mixed spastic-hypokinetic-dysarthria (Darley et al., Citation1969a, Citation1969b). Similarly, as speakers with MSA tend to present with a mixed spastic-ataxic-hypokinetic dysarthria (Darley et al., Citation1969a, Citation1969b), impaired precision and consistency may reflect the spastic and hypokinetic components, while impaired coordination and repetition rate may reflect the ataxic component.
Lastly, our finding of equal (and minimal) impairments across all components for speakers with PD was surprising, as we would have expected greater impairments in speed or precision based on the typical perceptual profile of hypokinetic dysarthria (Darley et al., Citation1969a, Citation1969b). The PD group in our meta-analysis, however, demonstrated a mild impairment on average, and prior work has found that in speakers with PD, phonatory features—which were not examined in this study—tend to be the leading deficit in the earlier stages of disease progression, with articulatory features becoming more impaired in the later stages (Ho et al., Citation1999).
Overall, these findings can only be viewed as very preliminary given the plethora of factors that were not controlled for (e.g. severity), as discussed in detail below. Thus, further work is needed to investigate the presence and validity of divergent articulatory impairment phenotypes across NMD populations. Nonetheless, our findings tentatively suggest that different speech motor subtypes may present with distinct articulatory patterns, which underscores the necessity to explore articulation more broadly across and within NMD populations.
Conclusion
Several limitations should be noted in this review. Namely, we did not control for sex, medication use, dysarthria severity, or task used in the study. While most of the studies included in the meta-analysis had age- and sex-matched control groups, we included studies with unequal sex ratios because a subset of the diseases examined do not occur equally across sexes. Indeed, many studies used sex ratios in their sample that represent the ratio of occurrence in the larger population. The greater number of males with ALS, PD, and PSP seen in is, therefore, relatively consistent with the higher global incidence of ALS, PD, and PSP in males than in females (Manjaly et al., Citation2010; Miller & Cronin-Golomb, Citation2010; Steele, Richardson, & Olszewski, Citation2014).
Additionally, we included studies that did not control for medication use. Of the 86 studies that examined PD, the vast majority (54 studies) reported that the participants were taking Levodopa, 13 studies reported that the participants were not taking any medication, and 19 studies did not report on medication use. However, recent work has demonstrated little effect of Levodopa on speech performance for speakers with PD, in both the early (Tykalova, Novotny, Ruzicka, Dusek, & Rusz, Citation2022) and later stages of disease progression (Cavallieri et al., Citation2021; Fabbri et al., Citation2017; Rusz, Tykalova, et al., Citation2021). Moreover, including participants regardless of medication time, type, or dosage provides a more representative sample of dysarthric characteristics in PD.
It is also important to note that certain parameters cannot be assessed in some speakers or can be invalidated due to the speaker’s speech severity (e.g. voice onset time cannot be assessed if the speaker has incomplete stop closures due to severe resonance problems). Consequently, a number of features may be underrepresented in speakers with conditions that tend to result in more resonatory dysfunction, for example. Additional research is critically needed to investigate the impacts of hypernasality and phonatory dysfunction on the acoustic signal and to identify more measures that are robust across disease severities.
In addition, we did not control for speech severity across all the disease groups (i.e. by only accepting articles with speakers in a specific severity range). While the range of intelligibility (i.e. 83-94%) and speech severity illustrated in indicates that the average severity level of all speakers was in the mild range, intelligibility and speech severity subscales were not recorded in every study or for all disease groups. The findings from our meta-analyses must, therefore, be interpreted with the knowledge that different effect sizes for any two disease groups (compared to controls) may be due to differences related to severity rather than disease. Finally, we included all tasks (e.g. word, sentence, passage, etc.) to extract the different acoustic features for the meta-analysis. Thus, we were not able to capture the task-specific manifestations of motor disease (e.g. impaired coordination may be more evident during the sequential motion rate task than during passage reading given the rapid sequencing required for this task).
Lastly, this work only considered features from the articulatory subsystem. Our focus on articulation was not intended to dismiss the diagnostic potential of other speech subsystems but rather to bring coherence to an inarguably important topic. Because our focus was on the articulatory subsystem, features representing consistency were derived from repetition tasks (as opposed to connected speech tasks, for which authors would often refer to the feature as a prosodic measure). Future work should, therefore, examine these features in the context of other tasks and review the landscape of resonatory, phonatory, respiratory, and prosodic features in individuals with NMDs.
Overall, our findings revealed a strong focus in the speech motor literature on acoustic features that represent precision and an underrepresentation of studies on features that represent coordination, consistency, speed, and repetition rate. In light of the need for research across all articulatory components to elucidate articulatory phenotypes, the restricted focus on precision is problematic. Furthermore, while the limited data in our meta-analysis precluded us from making specific recommendations regarding the most promising feature for each population, our results revealed phenotypic variability in articulatory impairments across speech motor subtypes. This finding motivates the need to employ more impairment-specific knowledge in algorithm development, which may significantly extend the impact such models have for individuals with NMDs. However, there remains a significant need to broaden and deepen our understanding of the articulatory phenotypes underlying NMDs. Further investigation across a more diverse representation of NMDs and in articulatory components that distinguish speech motor subtypes will be crucial to identifying the most effective features for detecting diagnosis-specific impairments.
Supplemental Material
Download MS Word (48.5 KB)Declaration interest
The authors report no conflicts of interest. The authors alone are responsible for the content and writing of the paper.
Additional information
Funding
References
- Ackermann, H. (2008). Cerebellar contributions to speech production and speech perception: psycholinguistic and neurobiological perspectives. Trends in Neurosciences, 31, 265–272. doi:10.1016/j.tins.2008.02.011
- Allen, G.D. (1975). Speech rhythm: Its relation to performance universals and articulatory timing. Journal of Phonetics, 3, 75–86. doi:10.1016/S0095-4470(19)31351-8
- Anand, S.S., Bell, D.A., & Hughes, J.G. (1995). The role of domain knowledge in data mining. Proceedings of 4th International Conference on Information and Knowledge Management, 37–43.
- Arksey, H., & O’Malley, L. (2005). Scoping studies: Towards a methodological framework. International Journal of Social Research Methodology, 8, 19–32. doi:10.1080/1364557032000119616
- Atlas of Multiple Sclerosis. (2020). Mapping multiple sclerosis around the world. Retrieved from https://www.msif.org/
- Berisha, V., Sandoval, S., Utianski, R., Liss, J., & Spanias, A. (2013). Selecting disorder-specific features for speech pathology fingerprinting. Proceedings of IEEE International Conference on Acoustics, Speech, and Signal Processing, 7562–7566.
- Blanchet, P.G., & Snyder, G.J. (2009). Speech rate deficits in individuals with Parkinson’s disease: A review of the literature. Journal of Medical Speech Language Pathology, 17, 1–7.
- Carmichael, J. (2014). Diagnosis of dysarthria subtype via spectral and waveform analysis. Computer Systems Science and Engineering, 1, 33–42.
- Caruso, A.J., Abs, J.H., & Gracco, V.L. (1988). Kinematic analysis of multiple movement coordination during speech in stutterers. Brain, 111(Pt. 2), 439–456.
- Cavallieri, F., Budriesi, C., Gessani, A., Contardi, S., Fioravanti, V., Menozzi, E., … Antonelli, F. (2021). Dopaminergic treatment effects on dysarthric speech: Acoustic analysis in a cohort of patients with advanced Parkinson’s disease. Frontiers in Neurology, 11, 1–7. doi:10.3389/fneur.2020.616062
- Childs, C.M., & Washburn, N.R. (2019). Embedding domain knowledge for machine learning of complex material systems. MRS Communications, 9, 806–820. doi:10.1557/mrc.2019.90
- Cordella, C., Dickerson, B.C., Quimby, M., Yunusova, Y., & Green, J.R. (2017). Slowed articulation rate is a sensitive diagnostic marker for identifying non-fluent primary progressive aphasia. Aphasiology, 31, 241–260. doi:10.1080/02687038.2016.1191054
- Crystal, T.H., & House, A.S. (1982). Segmental durations in connected speech signals: Preliminary results. Journal of the Acoustical Society of America, 72, 705–716. doi:10.1121/1.388251
- Darley, F.L., Aronson, A.E., & Brown, J.R. (1969a). Clusters of deviant speech dimensions in the dysarthrias. Journal of Speech, Language, and Hearing Research, 12, 462–496. doi:10.1044/jshr.1203.462
- Darley, F.L., Aronson, A.E., & Brown, J.R. (1969b). Differential diagnostic patterns of dysarthria. Journal of Speech, Language, and Hearing Research, 12, 246–269. doi:10.1044/jshr.1202.246
- De Bodt, M.S., Hernandez-Diaz Huici, M.E., & van De Heyning, P.H. (2002). Intelligibility as a linear combination of dimensions in dysarthric speech. Journal of Communication Disorders, 35, 283–292. doi:10.1016/S0021-9924(02)00065-5
- Duffy, J.R. (2013). Motor speech disorders: Substrates, differential diagnosis, and management. St. Louis, MO: Elsevier Health Sciences.
- Erkkinen, M.G., Kim, M.-O., & Geschwind, M.D. (2018). Clinical neurology and epidemiology of the major neurodegenerative diseases. Cold Spring Harbor Perspectives in Biology, 10, a033118–44. doi:10.1101/cshperspect.a033118
- Eyben, F., Wollmer, M., & Schuller, B.W. (2010). Opensmile: The Munich versatile and fast open-source audio feature extractor. Proceedings of IEEE International Conference on Multimedia, 1459–1462.
- Fabbri, M., Guimaraes, I., Cardoso, R., Coelho, M., Correia Guedes, L., Rosa, M.M., … Ferreira, J.J. (2017). Speech and voice response to a Levodopa challenge in late-stage Parkinson’s disease. Frontiers in Neurology, 8, 1–7. doi:10.3389/fneur.2017.00432
- Grabe, E., & Low, E.L. (2008). Durational variability in speech and the rhythm class hypothesis. In C. Gussenhoven & N. Warner (Ed.), Laboratory phonology (pp. 515–546). Berlin: De Gruyter Mouton.
- Green, J.R., Yunusova, Y., Kuruvilla, M.S., Wang, W., Pattee, G.L., Synhorst, L., … Berry, J.D. (2013). Bulbar and speech motor assessment in ALS: Challenges and future directions. Amyotrophic Lateral Sclerosis and Frontotemporal Degeneration, 14, 494–500. doi:10.3109/21678421.2013.817585
- Grosjean, F., & Collins, M. (1979). Breathing, pausing and reading. Phonetica, 36, 98–114. doi:10.1159/000259950
- Guenther, F. (2016). Neural control of speech. Cambridge, MA: The MIT Press.
- Gunduz, H. (2019). Deep learning-based Parkinson’s disease classification using vocal feature sets. IEEE Access, 7, 115540–115551. doi:10.1109/ACCESS.2019.2936564
- Gunning, D., & Aha, D. (2019). Darpa’s explainable artificial intelligence (XAI) program. AI Magazine, 40, 44–58.
- Gupta, R., Chaspari, T., Kim, J., Kumar, N., Bone, D., & Narayanan, S. (2016). Pathological speech processing: State-of-the-art, current challenges, and future directions. Proceedings of the IEEE international conference on acoustics, speech, and signal processing, 6470–6474.
- Gutz, S.E., Wang, J., Yunusova, Y., & Green, J. (2019). Early identification of speech changes due to amyotrophic lateral sclerosis using machine classification. Interspeech, 604–608.
- Hartelius, L., Elmberg, M., Holm, R., Lovberg, A.S., & Nikolaidis, S. (2008). Living with dysarthria: Evaluation of a self-report questionnaire. Folia Phoniatrica et Logopaedica, 60, 11–19. doi:10.1159/000111799
- Ho, A.K., Iansek, R., Marigliani, C., Bradshaw, J.L., & Gates, S. (1999). Speech impairment in a large sample of patients with Parkinson’s disease. Behavioural Neurology, 11, 131–137. doi:10.1155/1999/327643
- Kent, R.D., & Kim, Y. (2003). Toward an acoustic typology of motor speech disorders. Clinical Linguistics and Phonetics, 16, 427–445.
- Kent, R.D., Kent, J.F., Weismer, G., & Duffy, J.R. (2000). What dysarthrias can tell us about the neural control of speech. Journal of Phonetics, 28, 273–302. doi:10.1006/jpho.2000.0122
- Kent, R.D., Kent, J.F., Weismer, G., Martin, R.E., Sufit, R.L., Brooks, B.R., & Rosenbek, J.C. (1989). Relationships between speech intelligibility and the slope of the second-formant transitions in dysarthric subjects. Clinical Linguistics and Phonetics, 3, 347–358. doi:10.3109/02699208908985295
- Kim, Y., Weismer, G., Kent, R.D., & Duffy, J.R. (2009). Statistical models of F2 slope in relation to severity of dysarthria. Folia Phoniatrica et Logopaedica, 61, 329–335. Included in Review) doi:10.1159/000252849
- Lam, J., & Tjaden, K. (2016). Clear speech variants: An acoustic study in Parkinson’s Disease. Journal of Speech, Language, and Hearing Research, 59, 631–646. (Included in Review) doi:10.1044/2015_JSLHR-S-15-0216
- Lee, J., Hustad, K.C., & Weismer, G. (2014). Predicting speech intelligibility with a multiple speech subsystems approach in children with cerebral palsy. Journal of Speech, Language, and Hearing Research, 57, 1666–1678. doi:10.1044/2014_JSLHR-S-13-0292
- Longinetti, E., & Fang, F. (2019). Epidemiology of amyotrophic lateral sclerosis: An update of recent literature. Current Opinion in Neurology, 32, 771–776. doi:10.1097/WCO.0000000000000730
- Manjaly, Z.R., Scott, K.M., Abhinav, K., Wijesekera, L., Ganesalingam, J., Goldstein, L.H., … Al-Chalabi, A. (2010). The sex ratio in amyotrophic lateral sclerosis: A population based study. Amyotrophic Lateral Sclerosis and Frontotemporal Degeneration, 11, 439–442. doi:10.3109/17482961003610853
- Miller, I.N., & Cronin-Golomb, A. (2010). Gender differences in Parkinson’s disease: Clinical characteristics and cognition. Movement Disorders, 25, 2695–2703. doi:10.1002/mds.23388
- Munn, Z., Peters, M.D.J., Stern, C., Tufanaru, C., McArthur, A., & Aromataris, E. (2018). Systematic review or scoping review? Guidance for authors when choosing between a systematic or scoping review approach. BMC Medical Research Methodology, 18, 143–147. doi:10.1186/s12874-018-0611-x
- National Institute of Neurologic Disorders and Stroke. (2020). Progressive supranuclear palsy fact sheet. Retrieved from https://www.ninds.nih.gov/
- Nishio, M., & Niimi, S. (2006). Comparison of speaking rate, articulation rate and alternating motion rate in dysarthric speakers. Folia Phoniatrica et Logopaedica, 58, 114–131. doi:10.1159/000089612
- Orozco-Arroyave, J.R., Honig, F., Arias-Londono, J.D., Vargas-Bonilla, J.F., Daqrouq, K., Skodda, S., … Noth, E. (2016). Automatic detection of Parkinson’s disease in running speech spoken in three different languages. Journal of the Acoustical Society of America, 139, 481–500. doi:10.1121/1.4939739
- Owren, M.J., & Cardillo, G.C. (2006). The relative roles of vowels and consonants in discriminating talker identity versus word meaning. Journal of the Acoustical Society of America, 119, 1727–1739. doi:10.1121/1.2161431
- Parkinson’s Foundation. (2020). Statistics. Retrieved from https://www.parkinson.org/
- Pringsheim, T., Wiltshire, K., Day, L., Dykeman, J., Steeves, T., & Jette, N. (2012). The incidence and prevalence of Huntington’s disease: A systematic review and meta-analysis. Movement Disorders, 27, 1083–1091. doi:10.1002/mds.25075
- Robin, J., Harrison, J.E., Kaufman, L.D., Rudzicz, F., Simpson, W., & Yancheva, M. (2020). Evaluation of speech-based digital biomarkers: Review and recommendations. Digital Biomarkers, 4, 99–108. doi:10.1159/000510820
- Rong, P., Yunusova, Y., Wang, J., & Green, J.R. (2015). Predicting early bulbar decline in amyotrophic lateral sclerosis: A speech subsystem approach. Behavioural Neurology, 1, 1–11.
- Rowe, H.P., & Green, J.R. (2019). Profiling speech motor impairments in persons with amyotrophic lateral sclerosis: An acoustic-based approach. Interspeech, 4509–4513. (Included in Review)
- Rowe, H.P., Gutz, S.E., Maffei, M., & Green, J.R. (2020). Acoustic-based articulatory phenotypes of amyotrophic lateral sclerosis and Parkinson’s disease: Towards an interpretable, hypothesis-driven framework of motor control. Interspeech, 4816–4820. (Included in Review)
- Rowe, H.P., Stipancic, K.L., Lammert, A.C., & Green, J.R. (2021). Validation of an acoustic-based framework of speech motor control: Assessing criterion and construct validity using kinematic and perceptual measures. Journal of Speech, Language, and Hearing Research, 64, 4736–4718. (Included in Review) doi:10.1044/2021_JSLHR-21-00201
- Ruano, L., Melo, C., Silva, M.C., & Coutinho, P. (2014). The global epidemiology of hereditary ataxia and spastic paraplegia: A systematic review of prevalence studies. Neuroepidemiology, 42, 174–183. doi:10.1159/000358801
- Rusz, J., Hlavnicka, J., Novotny, M., Tykalova, T., Pelletier, A., Montplaisir, J., … Sonka, K. (2021). Speech biomarkers in rapid eye movement sleep behavior disorder and Parkinson disease. Annals of Neurology, 90, 62–75. doi:10.1002/ana.26085
- Rusz, J., Tykalova, T., Novotny, M., Zogala, D., Sonka, K., Ruzicka, E., & Dusek, P. (2021). Defining speech subtypes in de novo Parkinson disease: Response to long-term Levodopa therapy. Neurology, 97, 2124–2135.
- Schaffert, L.-N., & Carter, W.G. (2020). Do post-translational modifications influence protein aggregation in neurodegenerative diseases: A systematic review. Brain Sciences, 10, 232–230. doi:10.3390/brainsci10040232
- Schalling, E., Hammarberg, B., & Hartelius, L. (2007). Perceptual and acoustic analysis of speech in individuals with spinocerebellar ataxia (SCA). Logopedics Phoniatrics Vocology, 32, 31–46. (Included in Review) doi:10.1080/14015430600789203
- Schrag, A., Ben-Shlomo, Y., & Quinn, N.P. (1999). Prevalence of progressive supranuclear palsy and multiple system atrophy: A cross-sectional study. The Lancet, 354, 1771–1775. doi:10.1016/S0140-6736(99)04137-9
- Steele, J.C., Richardson, J.C., & Olszewski, J. (2014). Progressive supranuclear palsy: A heterogeneous degeneration involving the brain stem, basal ganglia and cerebellum with vertical gaze and pseudobulbar palsy, nuchal dystonia and dementia. Seminars of Neurology, 34, 129–150. doi:10.1055/s-0034-1377058
- Stevens, K.N. (1972). The quantal nature of speech: Evidence from articulatory–acoustic data. In P.B. Denes, & E.E. David Jr. (Eds.), Human communication: A unified view. (pp. 51–66). McGraw-Hill.
- Stegmann, G.M., Hahn, S., Liss, J., Shefner, J., Rutkove, S.B., Kawabata, K., … Berisha, V. (2020). Repeatability of commonly used speech and language features for clinical applications. Digital Biomarkers, 4, 109–122. doi:10.1159/000511671
- Takakura, Y., Otsuki, M., Sakai, S., Tajima, Y., Mito, Y., Ogata, A., … Nakagawa, Y. (2019). Sub-classification of apraxia of speech in patients with cerebrovascular and neurodegenerative diseases. Brain and Cognition, 130, 1–10. doi:10.1016/j.bandc.2018.11.005
- Tjaden, K., & Watling, E. (2003). Characteristics of diadochokinesis in multiple sclerosis and Parkinson’s disease. Folia Phoniatrica et Logopaedica, 55, 241–259. (Included in Review) doi:10.1159/000072155
- Tricco, A.C., Lillie, E., Zarin, W., O’Brien, K.K., Colquhoun, H., Levac, D., … Straus, S.E. (2018). PRISMA extension for scoping reviews (PRISMA-ScR): Checklist and explanation. Annals of Internal Medicine, 169, 467–473. doi:10.7326/M18-0850
- Tsanas, A., Little, M.A., McSharry, P.E., Spielman, J., & Ramig, L.O. (2012). Novel speech signal processing algorithms for high-accuracy classification of Parkinson’s disease. IEEE Transactions on Neural Systems and Rehabilitation Engineering, 59, 1264–1271.
- Tu, M., Berisha, V., & Liss, J. (2017). Interpretable objective assessment of dysarthric speech based on deep neural networks. Interspeech, 1849–1853. doi:10.21437/Interspeech.2017
- Turner, G.S., Tjaden, K., & Weismer, G. (1995). The influence of speaking rate on vowel space and speech intelligibility for individuals with amyotrophic lateral sclerosis. Journal of Speech, Language, and Hearing Research, 38, 1001–1013. (Included in Review) doi:10.1044/jshr.3805.1001
- Tykalova, T., Novotny, M., Ruzicka, E., Dusek, P., & Rusz, J. (2022). Short-term effect of dopaminergic medication on speech in early-stage Parkinson’s disease. NPJ Parkinson’s Disease, 8, 22–26. doi:10.1038/s41531-022-00286-y
- Tykalova, T., Rusz, J., Klempir, J., Cmejla, R., & Ruzicka, E. (2017). Distinct patterns of imprecise consonant articulation among Parkinson’s disease, progressive supranuclear palsy and multiple system atrophy. Brain and Language, 165, 1–9. (Included in Review).
- Wang, J., Kothalkar, P.V., Cao, B., & Heitzman, D. (2016). Towards automatic detection of amyotrophic lateral sclerosis from speech acoustic and articulatory samples. Interspeech, 1195–1199.
- Weismer, G., Jeng, J.-Y., Laures, J.S., Kent, R.D., & Kent, J.F. (2001). Acoustic and intelligibility characteristics of sentence production in neurogenic speech disorders. Folia Phoniatrica et Logopaedica, 53, 1–18. Included in Review) doi:10.1159/000052649
- Yunusova, Y., Green, J.R., Greenwood, L., Wang, J., Pattee, G.L., & Zinman, L. (2012). Tongue movements and their acoustic consequences in amyotrophic lateral sclerosis. Folia Phoniatrica et Logopaedica, 64, 94–102. Included in Review) doi:10.1159/000336890
- Ziegler, W., & Wessel, K. (1996). Speech timing in ataxic disorders: Sentence production and rapid repetitive articulation. Neurology, 47, 208–214. doi:10.1212/wnl.47.1.208