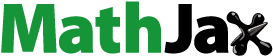
Abstract
Models with various complexity can asses soil C sequestration in agriculture. In this study, we updated the Introductory Carbon Balance Model (ICBM) with 28 years of additional data and included multiple long-term bare fallow experiments for old pool kinetics. We validated the model with data from a new long-term sister experiment. The new calibration included uncertainty in the estimation of below-ground C inputs to soil. The model now considers above- and belowground and external C inputs separately (ICBM/3). The underlying mathematical approach is the same, with two state variables and a climatic parameter, and such simple structure remained robust enough to describe soil organic C dynamics over six decades. We tested including an inert soil C pool in the model structure, but it did not decrease the observed variance. Similarly, an intercept in the functions for estimating belowground C input from crop yield was not useful. Results suggest that root C contributes more to the old organic C pool than aboveground C inputs. We also evaluated parameters interactions, in particular between C inputs and their transformation into more stable soil C interacted and decomposition kinetics. We also describe new functions for estimating the ICBM climatic parameter in a more user-friendly way.
Introduction
Soil organic carbon (SOC) is the primary terrestrial C pool, and its management is crucial to the global C budget in the biosphere [Citation1]. To manage it, several countries use models with different degrees of complexity to simulate changes in soil C stocks to determine their national contributions to global greenhouse gas balances [Citation2]. The robustness of such predictions depends on the accuracy of models and is unavoidably associated with uncertainties. Uncertainty in SOC models is related to imperfect knowledge of processes, model structure, errors in the data from long-term field experiments (LTEs) used for their calibration, and uncertainty in input data. All these components reduce the robustness of predictions. While structural uncertainty is inherent to specific model assumptions, other uncertainty sources can be estimated with stochastic calibrations [Citation3].
The annual C input to soil is the most important driver for changes in SOC stocks, and consequently, it is, in all models, one of the largest uncertainty sources [Citation4,Citation5]. This C input comes from exogenous organic amendments and aboveground (AG) and belowground (BG) crop residues; the latter comprises the root biomass left in the field at harvest plus root-derived C delivered to the soil during the growing season, usually referred to as rhizodeposition (RD) [Citation6]. Many SOC models estimate such inputs with empirical approaches (i.e. allometric functions) based on shoot-to-root (SR) ratios to estimate the C input from root biomass left in the field at harvest. These ratios consider shoots as the total AG biomass produced, estimated from grain yields using data on harvest index (i.e. the ratio of grain to total AG biomass; HI) for annual crops [Citation4]. Most models assume that a fraction of such C inputs is converted into more stabilized soil organic material (i.e. a humification coefficient; h) entering one or several SOC pools depending on model structure [Citation7].
Of the large uncertainties associated with C inputs, the most important are relative to estimating BG C inputs. For annual crops, pioneering work by Barber et al. [Citation8] and by Sauerbeck et al. [Citation9] indicated an RD coefficient of 1.0 (i.e. RD contributes as much as the root biomass to BG C inputs), an assumption commonly used thereafter [Citation10,Citation11]. Because these estimates are affected by traits of plants, pedo-climatic conditions and fluctuate over the growing season, RD coefficients reported in the literature vary from much lower than 0.5 to as high as 2.0 [Citation12,Citation13], evidentiating the high uncertainty around root C inputs estimation. We also know that field-based estimates of SR ratios for crops are highly variable within different crops, such as small-grain cereals and maize, with coefficients of variation typically of at least 50% [Citation6,Citation14].
Furthermore, SR ratios and HI data vary with genotypes and can change over time due to plant breeding; this can cause problems when modeling LTEs or assessing historical changes in C inputs [Citation15]. Finally, the most common allometric functions assume that SR ratios and HI data are linear with respect to production levels [Citation4]. However, some recent studies suggest that estimating BG C inputs using fixed values for root biomass by crop type may be better, independent from yields [Citation16,Citation17] or using HI values that change with crop yields [Citation18].
All these uncertainties affects the uncertainty of the humification (h) coefficients used in most SOC models, which are not measured but estimated with model calibration. The amount of C inputs to the soil and their decomposition (controlled in large part in compartmental SOC models by the h coefficients) interact directly in the models to determine the simulated SOC stocks over time. The change in SOC stocks over time in LTEs, the primary source of information to calibrate models, can result from many combinations of C inputs and humification. These direct interactions between the kinetics of decomposition and the C inputs, neither of which is ever precisely known, imply that many parameter combinations can explain the observed results (which is defined as equifinality) in the models [Citation19] and make it very difficult to determine both SR and h values.
Historically, commonly used values of h for AG crop residues (hs) vary between 0.05 and 0.15 [Citation20–22]. However, the h-values for roots (hr) were early recognized as higher than those for hs. For example, Hénin and Dupuis [Citation20] assumed the hr coefficient for maize and small-grain cereals were 1.50 and 1.80 times higher than the corresponding values for hs. Since then, several studies on LTEs and reviews have been quantifying this further, showing that total root-derived C (i.e. roots and RD) contributes from 1.3 up to almost four times more to SOC than AG [Citation11,Citation14,Citation23]. Following these studies, some models have recently assumed a higher root contribution to SOC than contributions from AG vegetation. For example, Dechow et al. [Citation24] modified the default h-value used for both shoots and roots (0.47) in the RothC model and found that using a lower range of values for hs (0.27–0.35) compared to hr (0.43–0.47) improved the model accuracy. On the other hand, in the AMG model, Clivot et al. [Citation25] considered hr values to be 26% to 77% higher than that for hs.
Sweden uses a relatively simple first-order two-component model, Introductory Carbon Balance Model (ICBM) [Citation26], to estimate SOC stock changes in the national reporting system [Citation27]. This approach relies on linear allometric functions for estimating plant C inputs from yields. It includes a parameter modifying SOC decomposition rates as a function of climate, edaphic properties, and crop conditions. The ICBM model is also used within farm-scale advisory tools such as Holos [Citation28] and other applications (Supplementary Material, ). Recent studies of the Ultuna LTE, used to calibrate the original version of ICBM, and its sister LTE at Lanna [Citation29,Citation30] showed that roots contribute more to SOC than AG crop residues. Based on these findings, some attempts have already been made to modify the ICBM model [Citation31,Citation32] by dividing the crop residue C input pool into AG and BG components, assuming hr 2.3 times the value of h (i.e. 0.125) in the original calibration. Kröbel et al. [Citation28] suggested the introduction of another third component accounting for organic amendments (i.e. ICBM/3 version), recognizing the need to calibrate and validate this new model structure.
Table 1. A summary of the treatments (•) and topsoil texture for the long-term field experiments used in the calibration and validation of ICBM/3B.
Furthermore, since its development, the original ICBM calibration still needs to be updated. More than two decades of new data are now available from the Ultuna LTE, and the sister experiment at Lanna, starting just after the original calibration, now has more than two decades of data. Moreover, throughout the years, we have further developed the functions for estimating the climatic parameter of ICBM, and such incremental improvements also need to be assessed and validated. The present study provides an update of the model, together with additional tools, such as the climatic module, that allow the model users to adapt this updated ICBM version to their workflow quickly and maintain the model being easily accessible. Given the expected equifinality of the model, we relied on a stochastic calibration approach, which should be more robust. Our objectives in the present study were as follows:
Calibrate the ICBM/3 version on the Ultuna LTE using more than two decades of new data within a stochastic Bayesian framework, including the new functions for estimating the climatic parameter. This version is referred to as ICBM/3B (B for Bayesian).
Test whether the longer time scale requires modifying ICBM/3B by including an additional inert SOC pool.
Test the robustness of a linear allometric approach to BG input estimations by adding an intercept in the allometric functions representing a minimal total BG C input independent of crop yield.
Analyze model uncertainties and equifinality, focusing on BG C inputs, including the available information for constraining SR ratios, hs, and hr from the literature.
Validate ICBM/3B on the Lanna sister LTE, the decomposition of the old SOC pool in ICBM/3B, and the new functions for estimating the climatic parameter on European bare-fallow LTEs.
Materials and methods
LTEs used for calibration and validation
The two Swedish LTEs are located along an east-southwest gradient in central Sweden. Experiment treatments involve the effect of three different types of mineral N fertilizer and organic amendments (farmyard manure FYM, composts, sewage sludge SLU, sawdust SAW, peat, small-grain cereal straw, and green manure GM) or a combination of both. The Swedish classical frame trial (FRAME-56) was initiated in 1956 in small plots (2 × 2 m, separated by metal frames) at Ultuna [Citation29], while its sister experiment (R3-0130) at Lanna started in 1996 in large (92 to 112 m2) plots [Citation30]. The latter includes several treatments in the former site; both LTEs also include a bare fallow treatment (). Both sites replicate all treatments four times in randomized block designs, and the organic amendments are applied every second year, corresponding to approximately 2 Mg C ha−1 yr−1 in the frame trial and 4 Mg ash-free dry mass ha−1 yr−1 at the Lanna site.
We used the Ultuna LTE (59.82oN, 17.65oE) time series for all model calibrations (). The field had been cultivated for centuries before the start of the experiment and is located on a clay loam, classified as a Typic Eutrochrept (USDA soil taxonomy) or Eutric Cambisol (FAO). The annual mean total precipitation is 542 mm, and the mean annual temperature is 5.8 °C. The organic amendments are added in the fall by carefully incorporating them into the topsoil down to a depth of 20 cm using a spade. The frequency of crops (number of years) between 1956 and 1999 was as follows: small-grain cereals (31), fodder rape (5), oilseed (3), fodder beet (2), and crop failure (3). Small-grain cereals were mostly spring barley (17) and oats (11), spring wheat (2), and winter wheat (1) were occasionally grown. Silage maize has been growing continuously since the year 2000. Every year at harvest, all AG plant biomass is cut at the soil surface, removed from the experimental plots, weighed, and sorted into crop products and AG crop residues; see Kätterer et al. [Citation29] for more details. The climatic data come from the Ultuna meteorological station, located at about 1 km distance from the experimental field.
Figure 1. Soil organic carbon (SOC) stock time series for the treatments of the Ultuna LTE, on which we based all model calibrations.

The Lanna LTE (58.34oN, 13.10oE) was used for validating the model and is located on an Aquic Haplocryept developed on a Quaternary silty clay deposit [Citation30]. The annual mean total precipitation is 636 mm, and the mean annual temperature 7.3 °C. The organic amendments are added in the fall by incorporating them through moldboard plowing into the topsoil to a maximum depth of 25 cm. The frequency of small-grain cereal crops (number of years) between 1996 and 2018 was as follows: oats (12), spring barley (8), winter wheat (2), and spring wheat (1). At this site, the stubble was left in the experimental plots after harvest, corresponding to 33% of the total amount of straw produced, measured for most of the years; see Kätterer et al. [Citation30] for more details. The climatic data for Lanna come from the Swedish Meteorological Institute (https://www.smhi.se/weather/Sweden-weather/obsevartions).
We also used data from an LTE bare-fallow network in Europe to specifically validate the decomposition rates of the old SOC pool and the climatic module of the ICBM model. From this network, consisting of six LTEs, we selected five, including Ultuna (year of initiation): Askov in Denmark (1956), Grignon and Versaille in France (1959 and 1928), and Kursk in Russia (1965). All sites have been kept free from vegetation, and we retrieved weather data records from https://www.ncdc.noaa.gov/cdo-web/; for details on these LTEs, see Barré et al. [Citation33].
SOC measurements and equivalent soil mass calculation
Measurements of SOC in the Ultuna and Lanna LTEs were made intermittently, more or less every second year, in the topsoil down to a fixed depth of 20 cm. For the time series of SOC data from the bare-fallow LTEs, measurements were taken ten times at Versaille and Grignon and six times at Kursk to a fixed depth of 25 cm, while measurements were available for almost every year at Askov to a fixed depth of 20 cm [Citation33]. The mass of soil sampled to a fixed depth changes over time proportional to dry soil bulk density changes. Consequently, we corrected the reference depth of 20 cm used in this study using an equivalent soil mass approach described in detail by Kätterer et al. [Citation29]. In short, treatment-specific bulk density values over time were estimated using linear functions based on the initial value measured at the start of the trial (1.44 Mg m−3) and those measured in the different treatments in 2009. The depth to which an equivalent amount of soil mineral mass is distributed was normalized for 20 cm depth in the control treatment. We then calculated soil carbon stocks by multiplying the bulk density and SOC concentration product with equivalent soil depth per treatment and year. The equivalent soil depth in the treatments at the Ultuna LTE varied between 19.5 and 27 cm [Citation29].
The ICBM/3B model
The model we calibrated is derived from the original ICBM presented by Andrén and Kätterer [Citation26]. The original ICBM model is a two-component first-order compartmental model with two state variables referred to as Young (Y) and Old (O) SOC pools, having specific decomposition rates (kY and kO, respectively), connected by a humification coefficient (h). The annual C input to soil enters through the Y pool, representing organic matter not yet humified with a fast decay rate, while the O pool represents the organic matter stabilized in the soil that decays much slower. In the original calibration, kY (here referred to as k1) was 0.8 year−1, and kO (here referred to as k2) was 0.0061 year−1 under standard climatic conditions at the calibration site in central Sweden [Citation26].
In order to consider C inputs from different organic materials having specific h-values, which determine the fraction that enters the O pool, we introduced three separate Y pools, all decaying in parallel (). These pools were AG crop residue inputs (Ys), total BG inputs including root tissues and rhizodeposits (Yr), and inputs from amendments (Ya), which are associated each with different humification coefficients (hs, hr, and ha, i, respectively, where i refers to each specific amendment in the experiment). In this application, Ya represented farmyard manure, sewage sludge, peat, and sawdust (). The basic mathematics of the ICBM model has been extensively introduced in the literature (Appendix A). In particular, we refer the reader to Appendix 1 in Kätterer and Andrén [Citation34], which thoroughly describes the equations. This model, from now on called the standard ICBM/3B model to distinguish it from the original version, adds two additional Y pools as described above, which are associated with different humification factors to account for different qualities of C inputs. This modification does not alter the mathematics foundations of the model, and, under constant input conditions, it is equivalent to considering an h value that is the weighted average of the individual h values of the various material added to the soil. However, it allows to decompose the different h values in calibration under non-steady input conditions, resulting in a more useful model when applied.
Figure 2. A schematic representation of the ICBM/3 model, adapted from Menichetti et al. [ Citation3].
![Figure 2. A schematic representation of the ICBM/3 model, adapted from Menichetti et al. [ Citation3].](/cms/asset/c0a65431-f1e9-4d9e-9137-e55204d62c72/tcmt_a_2304749_f0002_c.jpg)
The climatic functions
The climatic interactions with decomposition are implemented through a climatic parameter multiplying the two kinetic coefficients, k1, and k2. This parameter derives from the product of relative water content (rwat) and soil temperature (rtemp) calculated daily and averaged to an annual value since the model works in annual time steps. Its calculation involves daily climatic data and soil and crop properties. In this application, the climatic parameter was for convenience called rclim (please note that this corresponds to re_crop as defined in Bolinder et al. [Citation35]).
In this study, we updated the calculations of rwat and rtemp with the most recent functions we are using, as described in detail below. Specifically, we introduced a function with a peak in the middle at optimal moisture and with activity decreasing on both sides to represent the effect of soil moisture on biological decomposition activity at soil saturation. We also present in more detail the set of functions and information flow involved in the calculations of rclim (Appendix B), and we compiled the relative functions into an R package available on the GitHub repository of the corresponding author (address at the end of the Discussion section).
The effect of soil temperature is based on a quadratic response function [Citation27] with maximum () set to 30 °C and minimum (
) set to −3.8 °C.
This function presents a curve quite similar to the more conventional Arrhenius function [Citation36]. Its main limit of applicability is the lack of a protein denaturation mechanism, which varies depending on different soils but happens at relatively high temperatures (>35 °C [Citation37]), which are in general not reached in the soil in temperate climates. The daily mean soil temperature is calculated from the daily mean temperature based on an empirical relationship:
The effect of soil water content is more complicated to simulate since it is seldom available, and in general, it must be calculated from meteorological data. The response function for soil moisture is in this present model version adapted from Moyano et al. [Citation38]:
where
the optimal water content for decomposition, is calculated according to
and
the lower boundary water content for decomposition, is calculated according to
is the water content at field capacity,
is the water content at wilting point, and
= 0.5. However, this function needs, as input, besides soil hydrological constants, the soil water content. Soil water content is seldom available but can be calculated based on a simulated daily soil water balance once precipitation and evapotranspiration are known. For a specific soil layer of a given thickness, the water balance at a given time (
) can be calculated according to:
where
is the total precipitation in a given day
is the actual evapotranspiration on the same day, Pintercepted,t is the water intercepted by the crop and
is the excess water (lost by percolation). The
is calculated from reference evapotranspiration (
) through two coefficients according to
where
is the coefficient to calculate the potential evapotranspiration on the specific plot from the reference (
). The factor
is calculated from the green area index (GAI) according to
The intercepted water
is calculated using the GAI according to
The factor
is a coefficient to consider the effect of water stress on the crop, and it is calculated according to
Wilting point (
) and field capacity (
) are calculated according to pedotransfer functions developed for Sweden [Citation39].
The climatic parameter is calculated for each site and treatment separately and is normalized to the unit for the straw + N treatment (G) at the Ultuna site since this represents the most normal agricultural practice [Citation26,Citation40]. For this treatment, the updated calculations described above yielded a rwat × rtemp factor of 0.1057 for the time series 1956 to 2019. Thus, we were scaling all rclim values by dividing them by this factor, which implies that rclim became 1 for treatment G at Ultuna, and rclim for all other treatments at Ultuna, Lanna, and the long-term bare fallow sites have values relative to this normalized calibration site and treatment. Since rclim is multiplying the kinetic coefficients of the ICBM SOC pools, this implies that a higher value increases decomposition and that for a given amount of annual C inputs to soil, the steady-state SOC mass will be lower.
Testing possible modifications of the ICBM/3B model
Since the original ICBM calibration [Citation26], the trends in SOC stocks have been changing for most treatments. Consequently, it is expected that the decomposition rates have changed by adding two more decades of data, particularly in the bare-fallow treatment used initially for estimating the decomposition rate of the old SOC pool (kO), assuming a single exponential decaying pool may be less appropriate. Indeed, on very long time scales, the quality changes of SOC may not be well described by a single exponential law because the assumed exponential decay rate is slowing down over time [Citation33,Citation41]. Increasing the number of pools, adding, for example, a very slow or inert pool, can mitigate this problem. We also calibrated the model by introducing an inert pool to test if we needed to update the original model structure with only two SOC pools (Y and O) for time scales longer than four decades. This is a simple approach for increasing model complexity to represent a broader range of SOC quality. The size of the inert pool was treated as a parameter in the Bayesian framework.
The long-term experiment at Ultuna is located more or less in an urban area, and in order to avoid attracting people visiting the site to poach corn on the cobs, the maize was seeded late (between May 30 and June 23) and harvested relatively early (between September 2 and 29). Therefore, the maize at this site did not fully exploit the potential growing season. Some of the treatment plots are also extremely acidic. Consequently, maize yields are relatively low, varying between 1.9 and 8.4 and averaging 5.5 Mg dry matter ha−1 across all treatments from 2000 to 2019. Although maize primarily grows in southern Swedish regions, national average maize yields (2014–2018) usually are slightly higher than 10 Mg dry matter ha−1 (www.scb.se/JO0601). Therefore, we tested if an intercept in the allometric functions representing a minimal total BG C input could be more appropriate for these conditions, particularly for maize.
Where I is the mass of C inputs from roots each year, is the amount of roots exudates as a fraction of root mass, and we set it to 0.65 [Citation6],
is the average ratio of C in the total organic mass and was assumed between 0.40 and 0.51 with uniform prior,
and
represents each crop’s yields and shoot-to-root ratio, respectively, and
is the intercept. Units are in Mg C ha−1. The intercept was treated as a parameter in the Bayesian framework (i.e. it is zero in the standard ICBM/3B version).
Calibration and initialization of the ICBM/3B model
The model was calibrated within a Bayesian framework in JAGS language [Citation42] and ran in R [Citation43]. The technique is nowadays broadly adopted, and we refer to specific publications for details, such as Gelman et al. [Citation44] and Kruschke et al. [Citation45]. Shortly, the approach is a stochastic implementation of Bayesian statistics, achieved by running a large number of simulations with parameter sets sampled from their prior probability distributions and then updating such distribution based on the information from the data. The properties of the sampler used (Metropolis-Hastings) are Markovian (meaning that each new state is correlated only with the former), and this makes it so that the mean of each resulting chain (Markov chain Monte Carlo, shortly MCMC) converges to the optimal calibration. At the same time, the distribution of the MCMC represents the updated posterior probability distribution. Assuming that some distributions might be skewed, we generally relied on the mode rather than the mean (still reporting both) for estimating the best prediction. The climatic parameter rclim was left outside the Bayesian framework and utilized as an external driving variable. We also considered the RD coefficient (0.65) outside the Bayesian framework. We chose this because any uncertainty related to this parameter, being a multiplier of root biomass, will be included in the root biomass uncertainty since the two terms interact directly.
The initial ICBM calibration [Citation26] was based on a sequential approach, where different treatments at the Ultuna LTEs were used to infer specific model parameters. More specifically, the bare-fallow treatment, not receiving C inputs since the beginning of the experiment, was used to determine the decay rate of the old SOC pool (k2). This assumption implies that the decomposition of this pool was considered independent of C inputs, which may be questionable, particularly for some organic amendment treatments. In the present study, we relaxed this assumption, and instead of a sequential calibration, we calibrated all parameters simultaneously using all the treatments. We recognize that eventual modification of the kinetic coefficients can occur through SOC interactions with C inputs (e.g. priming). However, we assume they are not important enough to make the process of SOC decomposition radically different between treatments and that the Bayesian calibration can take care of minor modifications of the decay rates with its error model. This versatility is one of the advantages of the calibration method utilized in this study, together with estimating the uncertainty.
Model predictions were based on a combination of the whole MCMC parameter sets, uniformly resampled 5000 times over 25 independent chains. The model was running for all these 5000 × 25 sets, and the uncertainty intervals were drawn where the maximum and minimum of all these runs are present.
We initialized the model by including the proportion between the young and old (Y, O) SOC pools, which prior was assumed to be between 80% and 100% O (and the remaining Y), in the Bayesian framework. We assumed a fixed proportion for redistributing the C mass assigned to each Y pool (50% to the shoots, 50% to the roots, and none to the amendments). This way, the initialization of the SOC pools could be treated as any other parameters and calibrated within the Bayesian framework.
Since one of the study’s aims was to calibrate the model with more than two decades of new data, we conducted the calibration on two different time series. The first ended in 1999 (close to the original calibration, which ended in 1991), and the second ended in 2019. The latter allows us to assess the influence of silage maize since the shift from C3 to C4 crops that occurred in the year 2000.
Estimating model uncertainties: model priors
Initially, ICBM was designed in the beginning of the 1990s and aimed to produce a simple yet versatile first-order kinetic model for describing and predicting SOC stock changes [Citation26]. While recognizing that these simple approaches are not explicitly accounting for all the mechanisms of SOC formation and turnover [Citation46,Citation47], they have proven efficient in capturing a large amount of the variance in SOC decay [Citation41]. A large part of the model uncertainty is inherent to the structure common to all models in the same class (first-order, compartmental). On top of that, the assumption of a particular discretization carries some uncertainty, which we could include in the Bayesian framework as a parameter with a more abstract generalization of first-order models such as Q [Citation41]. However, model structural uncertainty was outside the scope of this study, and we focus here only on parameter uncertainty given the assumed model structure.
For the model version with a constant inert pool, we assumed its priors conservatively as a uniform distribution between 0 and 15 Mg SOC ha−1, considering that former estimates were between 2 and 7% of total SOC stocks [Citation33].
Priors for the decomposition rates and humification coefficients
In the present study, prior probability distributions for ICBM parameters were mainly taken from the literature [Citation26,Citation34,Citation48] concerning the mean values, but we introduced the uncertainties with different approaches for the SOC pool kinetics and the humification coefficients. We considered that the decomposition of the two SOC pools was relatively well known since k1 was estimated from empirical values from the literature and k2 was estimated from the bare-fallow treatment at Ultuna (see Andrén and Kätterer [Citation26]). We, therefore, utilized normal priors for the kinetic coefficients of the Y and O pools, with a standard deviation of 5% of the initial value.
For the priors regarding the BG humification coefficient (hr), we were considering the ratio of average contribution of total root-derived C compared to that of AG crop residues (hs) derived from studies in LTEs, mostly between 10 to 50 years duration [Citation11,Citation14,Citation29,Citation30]. Based on these studies, we considered that the upper limit of the priors for hr was four times higher than that for hs and assumed that both had uniform distributions. Similarly, since the previous humification coefficients for amendments (ha) were derived from inverse modeling, we also assumed a uniform distribution with wider priors, varying around ±100% of the formerly estimated values [Citation29,Citation30]. Priors for green manure and straw were considered the same as for shoots and connected with the same model parameter. The C content of organic matter was assumed between 40% and 51% (Appendix E), with uniform prior, contributing to the posteriors’ uncertainty estimate.
Priors used for the allometric functions
For the Ultuna calibration site, all AG plant biomass is cut at the soil surface and removed from experimental plots, and there is no need to have any priors concerning harvest index data. Thus, the AG crop residues that are not measured consist only of very short stubble parts below the soil surface, including stem bases, and its prior was estimated conservatively as a uniform distribution between 1 and 8% of total AG biomass. This estimate was based on results from an adjacent field trial, where BG stem bases accounted for between 2.5 and 3.8% of shoot biomass at harvest for wheat under different experimental treatments [Citation49].
In the present study, we used allometric functions assuming SR ratios relating linearly to crop yields, one of the most commonly used approaches [Citation6,Citation50], eventually adding a calibrated intercept (see “Testing possible modifications of the ICBM/3B model” section for an explanation of the rationale behind). However, BG estimations are possibly the most uncertain element of any SOC model. Therefore, we focused on estimating this approach’s uncertainty, conditional to the uncertainty in other parameters of the ICBM model. We were basing the priors for SR ratios on literature studies from Europe and North America evaluating SR ratios in field studies at or close to maturity and assuming uniform distributions. For small-grain cereals, it was based on Bolinder et al. [Citation6,Citation51], for maize on Amos and Walters [Citation12] and Bolinder et al. [Citation6,Citation14], while priors for root crops were set accordingly to Bolinder et al. [Citation52]. Priors for oilseed crops were based on the following studies: Ilola et al. [Citation53], Barraclough [Citation54], Pietola and Alakukku [Citation55], Gan et al. [Citation56], and Williams et al. [Citation57].
We used a fixed proportion for rhizodeposition (RD) of 0.65, suggested as an approximation by Bolinder et al. [Citation6], meaning that the plants are delivering RD C to the soil during the growing season in an amount equal to 65% of the total root biomass (i.e. 0.65 × root biomass measured at or close to maturity). This RD consists of root turnover (root hairs and fine roots), cell sloughing of epidermal root tissues, and root secretion of soluble low-molecular organic compounds (exudates) during the growing season. Our coefficient for RD is similar to the average value found for maize of 0.56 by Pausch et al. [Citation58]. Our approximation of 0.65 more or less also equals the mean value (0.66) from the studies on maize when considering only the measurements made about four months after planting in the review by Amos and Walters [Citation12], and it is similar to the value of 0.67 for wheat, maize, and soybean estimated at physiological maturity by Buyanovsky and Wagner [Citation59].
For the model version with an intercept () in the allometric functions, we assumed for this intercept a uniform prior between 0 and 5 Mg C ha−1; this lies within the range of general estimates for total root biomass [Citation60].
Results
Model calibration
The impact of 20 years of new data on the model
The present model calibration differs from the approach used in the original calibration in 1997 [Citation26], and differences are expected even when considering only the initial time series. These differences could be due to the calibration approach, which relaxes many assumptions made with the sequential calibration approach, but also to differences in the estimations of BG C inputs or the updated climatic functions. In particular, the decomposition of both the old and the young pool are different, with the young pool () decaying slower, and the old pool (
) decaying faster.
The ICBM mean annual rclim parameter in Ultuna showed high inter-annual variation, with values ranging from as low as 0.71 to as high as 1.32 (). The inter-annual variation followed the same general pattern for all treatments. There were minor differences between the treatments in the average values of rclim over the whole calibration period (1956 to 2019), from 1.00 for the straw + N reference treatment G (which is by definition scaled to unity) to the highest value of 1.05 for the bare-fallow treatment A. The rclim parameter tended to be higher when maize was grown (2000–2019), with an average value for all treatments of 1.11, compared to 0.97 during the first period ending in 1999.
The introduction of new information in the calibration with more than two decades of data slowed down compared to the calibration on the shorter time series and even more when compared with the original calibration from 1997, and in the same way, it sped up
(). The effect of the new data is particularly evident when comparing the posterior probability distributions of
and
When calibrating only on the bare fallow treatment, the differences between prior and posterior probability distributions become not noticeable, also because the uncertainty of the probability distributions increased greatly.
Figure 4. The prior and posterior probability distributions of the model parameters representing the kinetics of the two pools for the standard ICBM/3B model, calibrated on the time series until 1999 and 2019 and on all the plots or only on the bare fallow (BF) plot. Densities are estimated with kernel density estimation, N = 3750.

The model did not seem to require the introduction of an inert pool (), which was always calibrated close to zero.
Figure 5. The prior and posterior probability distributions of the intercept ( and inert pool parameters other than the kinetic terms for the ICBM/3B model (modified with intercept and inert, respectively) calibrated both on the time series until 1999 and 2019. Densities are estimated with kernel density estimation, N = 3750.

The model residuals in Ultuna for the model without an inert pool and intercept in the allometric functions () slightly increased when calibrating on the longer time series but with no difference in their general pattern.
Figure 6. Average residuals of the simulation in Ultuna considering the standard ICBM/3B version, calibrated both on the time series until 1999 and 2019.

Model residuals (measured minus predicted values) were generally well distributed around the mean (Appendix C), but the model presented some explicit biases toward different treatments, which could be related to processes specific to the treatments (). In particular, the model seemed to be overpredicting the calcium nitrate treatment C, and most of the amended treatments not receiving N inputs (B – control, H – Green manure, I – Peat and J – Farmyard manure), except for L (Sawdust), which was still pretty close to neutral, while N (Sawdust + N fertilizer) were also slightly overpredicted. Among the treatments without N addition the bare fallow treatment (A) and straw (F) were instead undepredicted.
The humification coefficients
The posterior mode values of the humification coefficients () for the amendments on the time series to 1999 were highest for peat (0.75), followed by that for sewage sludge and manure, and lowest for sawdust (0.27). The more recent data considering the whole time series to 2019, slowed down the humification coefficients slightly. The ratio of the humification coefficient for roots compared to shoots (i.e. hr/hs) was 2.6 and 4.0 when calibrating on the time series to 1999 and 2019, respectively. This difference was due to a lower value for hs in 2019 (0.09) than when the model was calibrated on the time series ending 1999 (0.14), whereas hr remained at 0.36 for both calibration periods. The posterior mode and mean values for all humification coefficients for both time series were similar ().
Figure 7. The prior and posterior probability distributions of the model parameters other than the kinetic terms for the standard ICBM/3B model calibrated both on the time series until 1999 and 2019. Densities are estimated with kernel density estimation, N = 3750.

Table 2. Results from the ICBM/3B calibration at the Ultuna long-term field experiment without an inert soil organic carbon pool and an intercept for minimal total belowground C inputs for maize.
The simulation of the bare fallow treatment
All the models considered simulated the Ultuna bare fallow treatment well, but when calibrating only on the bare fallow treatment, the model had a more accentuated initial decrease. This pattern was true for the standard and the model with an inert pool. This different model behavior is mainly due to the combination of a different initialization and a slower decomposition described by the parameter (), the most important in the bare fallow case since it describes the decomposition of the older organic matter. The
parameter when calibrating only on the bare fallow treatment was slower when calibrating until 1999 and when calibrating over the whole time series and was closer to the original value from 1997 (i.e. 0.0061).
Also in Lanna, although the bare fallow was not simulated well due to processes unaccounted for (most likely inputs above zero since the measurements record an increase in SOC), the different models had almost no difference in the residuals ().
Figure 8. The model simulation in Lanna, considering only the standard ICBM/3B model on the time series until 1999 and 2019 (the shaded area represents the uncertainty of the model calibrated until 1999) (panels A, C, E, G, I, J, L, and N) and the distributions of the RMSE in Lanna of all the calibrated model versions (panels B, D, F, H, J, K, M and O). The sewage sludge + metals treatment in Lanna was not considered for validation since it presents conditions that are far from the scope of the ICBM model.

The uncertainties of belowground C inputs estimation
Unsurprisingly there were strong interactions between the terms controlling the C inputs, particularly BG, and the humification factors. There was a relatively thin but elongated space of combinations of S:R and values for cereals with relatively good fitness () and a broader range for maize (). In both cases, there was a relatively vast region of high probability density with multiple local optima and little difference in the fitness of many different parameters’ combinations. This explains the broad posterior distributions of the S:R parameters ().
Model predictions and validation
The model represented, in general, the measured SOC in Ultuna relatively well, with an average RMSE of 3 Mg ha−1 when calibrated on the whole time series and 2.6 Mg ha−1 when calibrated for data until 1999. The less extreme treatments, as well as the treatments losing C, are represented particularly well by the model, while it struggles more with the treatments receiving amendments that lead to SOC accumulation (). The residuals were evenly distributed when considering the whole calibration, but biases were specific to each treatment (). The last decade presented a regular bias in many treatments (Appendix C).
Figure 11. The model simulation in Ultuna considering all three model versions (all calibrated on the time series until 2019).

Interestingly, the inert pool did not seem needed regardless of how long the calibration time series was, with its probability distribution resulting in both calibrations being very small and skewed toward zero. The intercept in the allometric functions seemed helpful to the model only to some extent but was still skewed toward zero ().
When considering the error of model predictions in the independent validation on the Lanna site (), the intercept improved the model’s performance only in the two mineral fertilization treatments. The minimal impact of the intercept on residuals was even more evident when the model was calibrated on the shorter time series.
The standard ICBM/3B model had mixed results in Lanna, representing relatively well most treatments but struggling in particular with the bare fallow and the sludge treatments ().
The four European long-term bare fallow sites considered were instead represented all pretty well except Kursk (), but the initialization had to be tuned for Versailles, with a larger initial proportion of young material at the start of the experiment to represent the land use change (from grassland to cropland). There was no appreciable difference between the model calibrations on the two time series.
Figure 12. The simulations from the standard ICBM/3B model, calibrated on the time series until 1999 and 2019, on four long-term bare fallow experiments in Europe [ Citation33]. Please notice for Versailles an additional simulation with a different initialization ratio (0.8) other than the one resulting from the calibration (0.952).
![Figure 12. The simulations from the standard ICBM/3B model, calibrated on the time series until 1999 and 2019, on four long-term bare fallow experiments in Europe [ Citation33]. Please notice for Versailles an additional simulation with a different initialization ratio (0.8) other than the one resulting from the calibration (0.952).](/cms/asset/ece9e96c-f560-4281-bb61-65796bd67caf/tcmt_a_2304749_f0012_c.jpg)
Discussion
Comparing the new model calibration with the former version of ICBM
The new model calibration presents some differences compared to the original model from 1997 that deserves some discussion. The original calibration used a stepwise parameterization process to derive rclim [Citation26]. Briefly, this was assuming h was constant (i.e. hs and hr were the same) and determined on only four of the treatments at Ultuna (B, C, F, and G), where a linear regression against yield differences provided the diverse rclim values under the assumption that higher plant biomass gives higher transpiration and thereby lower soil water content and rclim values. The principle used in our calibration estimating rclim values based on climatic response functions (i.e. rtemp and rwat) was introduced later [Citation27]. Using the climatic response functions showed that the bare-fallow in Ultuna still had the highest rclim value among treatments but did not provide as high a difference in rclim between the treatments as the former approach ( in Andrén and Kätterer [Citation26]). This is partly explained by the fact that all treatments constrained our Bayesian calibration and allowed the other parameters (k- and h-values and allometric functions) to explain the variance between treatments. In particular, a faster decay rate of the old SOC pool (k2) compensated the lower rclim value compared to the original calibration was compensated. The slightly lower rclim value for maize compared to other crops is mainly due to the shading of the maize plants.
Estimating the climatic parameter with climatic response functions has the advantage that this approach is more easily generalizable. For example, applied to more contrasting climatic conditions, such as the eight agricultural regions within the Swedish national inventory reporting system [Citation40], it provided rclim values ranging from 0.67 in the north to 1.30 in southern Sweden. Similarly, the approach has also helped describe climate’s influence on SOC decomposition at much broader scales [Citation35,Citation61], and the approach can be easily tuned to these broader ranges.
Another issue to consider is the equifinality that this class of models (first-order SOC models, driven by C inputs) presents [Citation19,Citation62]. The optima for the related parameters are, therefore, not well constrained. Because of that, slight differences in methods or assumptions can shift the optimal calibration values. The calibration approach adopted in the present study differs from the former version since it does not consider optima for single treatments to constrain parameters in sequence. Instead, in the current calibration approach, since all parameters are calibrated simultaneously on all treatments, the parameter values will represent optima between all the data points. With the former sequential approach, the SOC dynamics in the bare fallow treatment was assumed to be the most reasonable approximation of the old SOC kinetic coefficient, which was then used to define in all the other treatments (thus making one more assumption and reducing the amount of possible optimal parameter sets). The overall optima for the SOC decomposition rates could differ from the bare fallow optimum for several reasons, including substrate interactions such as priming effects), differences in microbial community composition, or effects of soil structure on biogeochemical processes.
Testing modifications of the ICBM/3B model
When considering the residuals of the three model versions, the only appreciable differences when introducing an intercept in the allometric functions occurred for the mineral fertilizer-only treatments (). The addition of an inert pool seemed to make very little difference in all treatments, confirmed by the small size of the calibrations assigned to the inert pool (). Besides, guessing the size of the “inert” pool is difficult since it represents a coarse approximation as a concept [Citation41] and is connected to the slower cycling part of SOC, which is likely dependent on local edaphic factors [Citation63,Citation64].
The standard ICBM/3B model, similar to the original ICBM, performed relatively well in Lanna concerning most treatments but failed to represent the high C input extremes (). In particular, the model struggled to represent sludges and, to a lesser extent, compost. The model assumes that all amendments have the same kinetic coefficient (i.e. k1), which remains a simplification. Furthermore, concerning sludge, its properties depend on the process used in the sewage treatment plants, which can be variable over time and from one plant to another, depending on the technical solutions adopted. In the lowest C inputs extreme (i.e. bare fallow), it is possible that the experiment at Lanna had C inputs from weeds since weeding was mechanical, contrary to the Ultuna bare-fallow plots, where weeding is done by hand and more frequently.
Testing the model representation for the decay of the old SOC pool
Despite the model’s being optimized on all treatments rather than just on the Ultuna bare-fallow as in the 1997 model version, the model performed well () in other long-term bare-fallow experiments [Citation33]. The model’s excellent performance in these experiments is not extremely surprising since the kinetics of old organic matter depends mainly on time, temperature, and moisture and very little on other edaphic factors [Citation41,Citation65]. Nevertheless, it represents a good validation of the general robustness of the kinetic coefficient of the old pool. In the absence of C inputs, the young pool rapidly more or less disappears, and the model becomes quickly equivalent to a one-compartment model represented by the old pool. Consequently, given the broad climatic gradient of the bare-fallow experiments, it is also a good validation of the climatic module since rclim is the only driver under such conditions. The model worked well for Grignon and Askov, while it struggled more for Versailles and Kursk. The Kursk site is a chernozem, a particularly rich soil where local processes have accumulated organic matter. These processes are probably not fully captured by our model. The site at Versaille is older than the other sites and has a different history. This site was a former grassland [Citation33], and the higher biomass productivity or at least root C inputs of this ecosystem, compared to cropland, seems to have influenced the quality of the initial SOC [Citation41]. When we tuned the model with an 80% initial size of the old pool (and therefore a 20% young pool), compared with the 98% old pool assumed by default in the other sites (i.e. set to the same initial proportion than Ultuna), the model represented the site in Versailles closely. This suggests that the only discrepancy of the Versailles site is not in the decomposition rate of the old pool but probably in the higher amount of young material at the beginning of the experiment, which can be represented by site-specific model starting conditions.
Model uncertainty
Model predictions residuals
Despite a relatively high uncertainty of model parameters (, ), model prediction boundaries were well constrained (, ). Such relatively narrow predictions’ uncertainty suggests that the model structure makes it robust and suitable for describing the SOC balance even if parameters are not precisely known since predictions are relatively insensitive to parameter uncertainty.
Most of the treatments not receiving N were overpredicted except for sawdust, calcium nitrate and bare fallow. All other underpredicted treatments receive some form of quickly available N. This pattern might be related to N-related processes but not to the C/N ratio (which does not correlate with the model residual). More likely, a more readily available N form might slightly slow down the SOC decomposition in a way not considered by the model by interacting with the soil microbiota. This effect is clear and well known for N-limited environments such as forests [Citation66], and it is mentioned in the literature for agricultural systems, although less clear [Citation67]. The introduction of maize seemed to increase the difficulties for the model, probably because of additional processes not captured by it, for example the already mentioned possible shading effect, or other similar effect on the micrometeorology of the plots, or effects related with inputs estimation (maize inputs are estimated lower than previous crops).
While in temperate agricultural systems [Citation68] the C:N ratio seems to drive decomposition with no effect of N fertilizers, N addition plays a more important role in determining SOC decomposition in poorer systems [Citation69]. In our case, long-term treatments without additional N added might have little bioavailable N compared with more conventional agricultural treatments and present some N limitations. Regarding the absolute error, the model struggled with treatments receiving amendments where the material is already partially decomposed and can present some very specific chemical quality, such as peat. The model considers the specificities of these amendments with different humification coefficients, but the amendments might also lead to differences in the chemistry of the remaining SOC (conceptualized by the O pool), which may explain the error.
Model equifinality and belowground C inputs
The strong interactions between the mass of C inputs and SOC decomposition are well recognized for this particular class of SOC model [Citation3,Citation19,Citation70,Citation71]. Decomposition rates are uncertain due to limitations in the measurement techniques on the relevant time scales. These results also confirm the high uncertainty in the estimation of C inputs, in particular due to the limitations when measuring BG biomass and fluxes, which constitutes a major limitation in SOC modelling [Citation4]. These uncertainties affect all SOC models and are a reason to keep the model as simple as possible since a more detailed model (with more pools) would face the same problem but only distribute the uncertainty among more parameters.
The equifinality caused by the interactions between the BG C input estimates and the kinetics of both SOC pools make the humification coefficient of roots and the SR ratios uncertain. The broad spread of the various chains in the traceplots of the relative parameter (Appendix D) also seem to be a consequence of such equifinality, which becomes visible when plotting the values of the cereals’ SR ratio and the coefficient for each element of the MCMC chains ( and ). This interaction is particularly important for small-grain cereals and maize, the dominant crops in the time series at Ultuna, representing 53 and 39%, respectively.
The mode of posteriors for the humification coefficients for crop residues at Ultuna ( and ) were within the range of those estimated in LTEs involving mostly small-grain cereals and maize, with values between 0.08 to 0.20 and 0.14 to 0.44 for AG and BG crop residues, respectively [Citation10,Citation29,Citation30,Citation72–75]. The ratio we obtained for the humification coefficient for roots compared to shoots (i.e. hr/hs) was also within the range of previously estimated values (i.e. 1.3 to 3.7) obtained in LTEs and reviews [Citation11,Citation14,Citation23,Citation29,Citation30]. Compared to similar studies using the RothC [Citation24] and AMG [Citation25] models, our calibration highlights the usefulness of attributing a higher C contribution of root C to the old SOC pool also in the ICBM model. For the organic amendments, humification coefficients were similar to those calculated earlier by Kätterer et al. [Citation29] using a first-order kinetics approach and linear approximation. For manure, hFYM calculated on the time series ending 1999 is almost identical to that used in the Hénin and Dupuis [Citation20] model (i.e. 0.30), whereas hFYM derived using the whole time series is similar to the average value (i.e. 0.26) in a recent review on data from Canadian LTEs [Citation76].
The mode of posterior SR ratios for the dominant crops, small-grain cereals and maize, were 4.8 and 4.6 for small-grain cereals when calibrated on the time series up to 1999 and 2019, respectively, and 12.1 for maize ( and ). This is close to the average SR ratio that was found for small-grain cereals (i.e. 5.6) in a review study on Scandinavian data [Citation77]. The relatively high SR ratio for maize is probably because its mean growth period at this site (see “The climatic functions” section) was only 95 ± 10 days, whereas the potential growing season in the area is about 140 days. However, the SR ratio from our calibration is similar to that reviewed for maize by Amos and Walters [Citation12], where SR ratios were between 11.1 and 14.5 for the measurements made 73 to 105 days after planting. Other recent studies on varieties used in Europe are also reporting SR ratios for maize well above 10.0 [Citation13,Citation78].
The impact of almost three decades of data on the calibration
The modifications in the two kinetic coefficients caused by two more decades of data align with the expectations since decomposition naturally slows down with time. Since the decomposition, initially well approximated by a single exponential decaying pool [Citation33], departs from such simple decomposition kinetics the more time passes, and in theory, the longer the time scale, the more pools would be useful. Although there is no real “inert” material in the soil [Citation41], the decay tends to slow down over time more than what is described by a single exponential law because the SOC quality changes gradually over time toward more recalcitrant material. This slowing down in the decomposition of the old SOC pool is why we introduced in this calibration the possibility of explaining part of the variance with a constant inert pool, a relatively simple modification that would allow the model to better represent this decrease in decomposition over time. However, this inert pool was not particularly crucial (), suggesting that a two-pool model can still capture most of the variance of SOC over time, even approaching the scale of a century. Residuals also were not particularly high, although they did increase from an RMSE of 2.6 to 3.0 Mg ha−1. Adding the last decades of data shifted the optimum toward reduced humification coefficients and slightly faster old pool decomposition following the slightly diminished C inputs from maize (Appendix F). Specifically, the average root C inputs, representing the largest organic contribution other than amendments, evolved from an average of roughly 0.6 to 0.32 Mg C ha−1 y−1.
The regular bias present in many of the treatments for the points of the last decade (Appendix C) might also be related to some other effect connected with maize. For example, since the experimental plots are adjacent and relatively small, in some treatments, plants might be shaded by the plants in another (maize grows much taller than all other crops previously grown in the experiment) in a more favorable position in the randomized block design.
When calibrating the model only on the bare-fallow treatments, the effect of the last two decades of data becomes clear (). The term is mostly not constrained by the data from the bare-fallow treatment since, with no inputs, the young material disappears quickly from the system, but the term
is definitely affected by the new data. It is not surprising that the decomposition of SOC in the bare-fallow seems to slow down. Still, given how well the standard ICBM/3B model calibrated on all treatments performed on all long-term bare-fallow experiments, this effect is small.
Description of the material attached to the study
We included as Supplementary Material:
The updated functions for calculating the climatic scaling are downloadable at https://github.com/ilmenichetti/reclim and possible to install in R through the package devtools.
A table describing all the applications of ICBM in the literature that we are aware of, with references
Conclusions
The ICBM model was calibrated with new data and considering a new structure (ICBM/3B), which allowed us to test if the model assumptions still hold on a longer time scale than the original model calibration. The assumptions still hold; in particular, the model does not need to consider more than two pools to represent the observed changes in SOC stocks. Introducing an intercept in the allometric functions was also not useful.
The model was calibrated with a multi-objective Bayesian approach, resulting in slightly different kinetic coefficients than the former sequential calibration approach, with a slightly faster decomposition for the old pool that nevertheless seems realistic. In particular, the calibration made evident the strong equifinality of the model due to the interactions between the decomposition of SOC and uncertain C inputs, which is common for compartmental SOC models. The lack of precise knowledge about BG C inputs is confirmed as the highest uncertainty for this class of SOC models. At the same time, the calibration pointed out the relative robustness of model predictions to model equifinality and uncertainty of BG C input estimates.
Keeping the model as simple as possible seems an effective way to deal with such equifinality since added complexity would only increase it without substantially increasing the model performance.
The ICBM model is one of the simplest compartmental SOC models available, and, nevertheless, it captures most of the SOC variance over time, making it useful for applied studies involving SOC decomposition. This simplicity is one of the main assets of the model. There is still a need to evaluate model performance with new experimental results and measurements of SOC dynamics. In particular, future work will focus on a refined regional multi-site calibration using data from other LTEs to test further and improve the generalizability of modeling results.
Supplemental Material
Download MS Word (50.4 KB)Acknowledgments
We are thankful to our Faculty for providing resources to maintain the Swedish long-term experiments and especially to Per Hillström, who carefully managed the Ultuna frame trial for the three recent decades, and to Karl-Gunnar Söderdahl, who took over the management in recent years. We are also grateful to the persons and institutions who provided data from the long-term bare fallow network sites (see Barré et al. [Citation33]). In particular, we acknowledge the inputs from Vladimir Romanenkov (Kursk site, Lomonosov Moscow State University, All-Russian Institute for Agrochemistry), Folkert van Oort and Sabine Houot (the two French sites, French National Institute for Agricultural Research and Agroparistech), and Bent T. Christensen (Askov Experimental Station, Dept. Agroecology, Aarhus University).
Disclosure statement
During the preparation of this work, the author(s) used Grammarly in order to improve the readability of the manuscript. After using this tool/service, the author(s) reviewed and edited the content as needed and take(s) full responsibility for the content of the publication.
Data availability statement
The data that support the findings of this study are available on request from the corresponding author, LM. The data are not publicly available due to being property of the author’s institution, which requires following particular policies for disclosing them.
Additional information
Funding
References
- Batjes NH. Total carbon and nitrogen in the soils of the world. Eur J Soil Sci. 1996;47(2):151–163. doi: 10.1111/j.1365-2389.1996.tb01386.x.
- Blujdea VN, Viñas RA, Federici S, et al. The EU greenhouse gas inventory for the LULUCF sector: I. Overview and comparative analysis of methods used by EU member states. Carbon Manag. 2016;6(5-6):247–259. doi: 10.1080/17583004.2016.1151504.
- Menichetti L, Kätterer T, Bolinder MA. A Bayesian modeling framework for estimating equilibrium soil organic C sequestration in agroforestry systems. Agric Ecosyst Environ. 2020;303:107118. doi: 10.1016/j.agee.2020.107118.
- Keel SG, Leifeld J, Mayer J, et al. Large uncertainty in soil carbon modelling related to method of calculation of plant carbon input in agricultural systems. Eur J Soil Sci. 2017;68(6):953–963. doi: 10.1111/ejss.12454.
- Pausch J, Kuzyakov Y. Carbon input by roots into the soil: quantification of rhizodeposition from root to ecosystem scale. Glob Chang Biol. 2017;24(1):1–12. doi: 10.1111/gcb.13850.
- Bolinder MA, Janzen HH, Gregorich EG, et al. An approach for estimating net primary productivity and annual carbon inputs to soil for common agricultural crops in Canada. Agric Ecosyst Environ. 2007;118(1-4):29–42. doi: 10.1016/j.agee.2006.05.013.
- Shibu ME, Leffelaar PA, Van Keulen H, et al. Quantitative description of soil organic matter dynamics – a review of approaches with reference to rice-based cropping systems. Geoderma. 2006;137(1-2):1–18. doi: 10.1016/j.geoderma.2006.08.008.
- Barber DA, Martin JK. The release of organic substances by cereal roots into soil. N Phytol. 1976;76(1):69–80. doi: 10.1111/j.1469-8137.1976.tb01439.x.
- Sauerbeck DR, Johnen BG. Root formation and decomposition during plant growth. In: Editorial staff of the International Atomic Energy Agency, editor. Soil organic matter studies, vol. I. Vienna: International Atomic Energy Agency; 1977. p. 141–148.
- Plénet D, Lubet E, Juste C. Évolution à long terme du statut carboné du sol en monoculture non irriguée du maïs (Zea mays L). Agron. 1993;13(8):685–698. doi: 10.1051/agro:19930802.
- Rasse DP, Rumpel C, Dignac M-F. Is soil carbon mostly root carbon? Mechanisms for a specific stabilization. Plant Soil. 2005;269(1-2):341–356. doi: 10.1007/s11104-004-0907-y.
- Amos B, Walters DT. Maize root biomass and net rhizodeposited carbon: an analysis of the literature. Soil Sci Soc Am J. 2006;70(5):1489–1503. doi: 10.2136/sssaj2005.0216.
- Hirte J, Leifeld J, Abiven S, et al. Below ground carbon inputs to soil via root biomass and rhizodeposition of field-grown maize and wheat at harvest are independent of net primary productivity. Agric Ecosyst Environ. 2018;265:556–566. doi: 10.1016/j.agee.2018.07.010.
- Bolinder MA, Angers DA, Giroux M, et al. Estimating C inputs retained as soil organic matter from corn (Zea mays L). Plant Soil. 1999;215(1):85–91. doi: 10.1023/A:1004765024519.
- Wiesmeier M, Hübner R, Dechow R, et al. Estimation of past and recent carbon input by crops into agricultural soils of southeast Germany. Eur J Agron. 2014;61:10–23. doi: 10.1016/j.eja.2014.08.001.
- Taghizadeh-Toosi A, Christensen BT, Glendining M, et al. Consolidating soil carbon turnover models by improved estimates of belowground carbon input. Sci Rep. 2016;6(1):32568. doi: 10.1038/srep32568.
- Taghizadeh-Toosi A, Cong W-F, Eriksen J, et al. Visiting dark sides of model simulation of carbon stocks in European temperature agricultural soils: allometric function and model initialization. Plant Soil. 2020;450(1-2):255–272. doi: 10.1007/s11104-020-04500-9.
- Fan J, McConkey B, Janzen H, et al. Harvest index-yield relationship for estimating crop residue in cold continental climates. Field Crops Res. 2017;204:153–157. doi: 10.1016/j.fcr.2017.01.014.
- Menichetti L, Kätterer T, Leifeld J. Parametrization consequences of constraining soil organic matter models by total carbon and radiocarbon using long-term field data. Biogeosciences. 2016;13(10):3003–3019. doi: 10.5194/bg-13-3003-2016.
- Hénin S, Dupuis M. Essai de bilan de la matière organique du sol. Ann Agron. 1945;15:17–29.
- Boiffin J, Kéli Zagbahi J, Sebillotte M. Système de culture et statut organique des sols dans le noyonnais: application du modèle de Hénin-Dupuis. Agronomie. 1986;6(5):437–446. doi: 10.1051/agro:19860503.
- Soltner D. Les grandes productions végétales: phytotechnie spéciale, Tome 1, Le sol et son amélioration. Collection Sciences et Techniques Agricoles, Sainte Gemmes sur Loire, France; 2000. p. 468.
- Johnson JM-F, Allmaras RR, Reicosky DC. Estimating source carbon from crop residues, roots and rhizodeposits using the national grain-yield database. Agron J. 2006;98(3):622–636. doi: 10.2134/agronj2005.0179.
- Dechow R, Franko U, Kätterer T, et al. Evaluation of the RothC model as a prognostic tool for the prediction of SOC trends in response to management practices on arable land. Geoderma. 2019;337:463–478. doi: 10.1016/j.geoderma.2018.10.001.
- Clivot H, Mouny J-C, Duparque A, et al. Modeling soil organic carbon evolution in long-term arable experiments with AMG model. Environ Model Softw. 2019;118:99–113. doi: 10.1016/j.envsoft.2019.04.004.
- Andrén O, Kätterer T. ICBM : the introductory carbon balance model for exploration of soil carbon balances. Ecol Appl. 1997;7(4):1226–1236. doi: 10.1890/1051-0761(1997)007[1226:ITICBM]2.0.CO;2.
- Andrén O, Kätterer T, Karlsson T. ICBM regional model for estimations of dynamics of agricultural soil carbon pools. Nutr Cycl Agroecosyst. 2004;70(2):231–239. doi: 10.1023/B:FRES.0000048471.59164.ff.
- Kröbel R, Bolinder MA, Janzen HH, et al. Canadian farm-level soil carbon change assessment by merging the greenhouse gas model Holos with the Introductory Carbon Balance Model (ICBM). Agric Syst. 2016;143:76–85. doi: 10.1016/j.agsy.2015.12.010.
- Kätterer T, Bolinder MA, Andrén O, et al. Roots contribute more to refractory soil organic matter than aboveground crop residues, as revealed by a long-term field experiment. Agric Ecosyst Environ. 2011;141(1-2):184–192. doi: 10.1016/j.agee.2011.02.029.
- Kätterer T, Börjesson G, Kirchmann H. Changes in organic carbon in topsoil and subsoil and microbial community composition caused by repeated additions of organic amendments and N fertilisation in a long-term field experiment in Sweden. Agric Ecosyst Environ. 2014;189:110–118. doi: 10.1016/j.agee.2014.03.025.
- Ericsson N, Porsö C, Ahlgren S, et al. Time-dependent climate impact of a bioenergy system – methodology development and application to Swedish conditions. GCB Bioenergy. 2013;5(5):580–590. doi: 10.1111/gcbb.12031.
- Hammar T, Ericsson N, Sundberg C, et al. Climate impact of willow grown for bioenergy in Sweden. Bioenerg Res. 2014;7(4):1529–1540. doi: 10.1007/s12155-014-9490-0.
- Barré P, Eglin T, Christensen B, et al. Quantifying and isolating stable soil organic carbon using long-term bare fallow experiments. Biogeosciences. 2010;7(11):3839–3850. doi: 10.5194/bg-7-3839-2010.
- Kätterer T, Andrén O. The ICBM family of analytically solved models of soil carbon, nitrogen and microbial biomass dynamics—descriptions and application examples. Ecol Modell. 2001;136(2-3):191–207. doi: 10.1016/S0304-3800(00)00420-8.
- Bolinder MA, Andrén O, Kätterer T, et al. Soil organic carbon sequestration potential for Canadian agricultural ecoregions calculated using the Introductory Carbon Balance Model. Can J Soil Sci. 2008;88(4):451–460. 2008doi: 10.4141/CJSS07093.
- Lloyd J, Taylor J. On the temperature dependence of soil respiration. Funct Ecol. 1994;8(3):315–323. doi: 10.2307/2389824.
- Ratkowsky DA, Olley J, Ross T. Unifying temperature effects on the growth rate of bacteria and the stability of globular proteins. J Theor Biol. 2005;233(3):351–362. doi: 10.1016/j.jtbi.2004.10.016.
- Moyano FE, Manzoni S, Chenu C. Responses of soil heterotrophic respiration to moisture availability: an exploration of processes and models. Soil Biol Biochem. 2013;59:72–85. doi: 10.1016/j.soilbio.2013.01.002.
- Kätterer T, Andrén O, Jansson P-E. Pedotransfer functions for estimating plant available water and bulk density in Swedish agricultural soils. Acta Agric Scand B Soil Plant Sci. 2006;56(4):263–276. doi: 10.1080/09064710500310170.
- Andrén O, Kätterer T, Karlsson T, et al. Soil C balances in Swedish agricultural soils 1990-2004, with preliminary projections. Nutr Cycl Agroecosyst. 2008;81(2):129–144. doi: 10.1007/s10705-008-9177-z.
- Menichetti L, Ågren GI, Barré P, et al. Generic parameters of first-order kinetics accurately describe soil organic matter decay in bare fallow soils over a wide edaphic and climatic range. Sci Rep. 2019;9(1):20319. doi: 10.1038/s41598-019-55058-1.
- Plummer M. JAGS: a program for analysis of Bayesian graphical models using Gibbs sampling JAGS: just another Gibbs sampler. In: Hornik K, Leisch F, Zeileis A, editors. Proceedings of the 3rd International Workshop on Distributed Statistical Computing (DSC 2003). Vienna; 2003.
- Core Team. R: A language and environment for statistical computing. Vienna, Austria: R Foundation for Statistical Computing; 2022.
- Gelman A, Carlin J, Stern B, et al.. Bayesian data analysis. 2nd ed. London: Chapman & Hall; 2004.
- Kruschke JK, Aguinis H, Joo H. The time has come: Bayesian methods for data analysis in the organizational sciences. Organ Res Methods. 2012;15(4):722–752. doi: 10.1177/1094428112457829.
- Coleman K. and Jenkinson, D. S. RothC-26.3 - a model for the turnover of carbon in soilA model for the turnover of carbon in soil. In Powlson DS, Smith P, Smith JU, editors. Evaluation of soil organic matter models, NATO ASI series. Berlin: Springer-Verlag; 1996. p. 237–246.
- Franko U, Oelschlägel B, Schenk S. Simulation of temperature-, water-and nitrogen dynamics using the model CANDY. Ecol Modell. 1995;81(1-3):213–222. doi: 10.1016/0304-3800(94)00172-E.
- Kätterer T, Eckersten H, Andrén O, et al. Winter wheat biomass and nitrogen dynamics under different fertilization and water regimes: application of a crop growth model. Ecol Modell. 1997;102(2-3):301–314. doi: 10.1016/S0304-3800(97)00065-3.
- Kätterer T, Hansson A-C, Andrén O. Wheat root biomass and nitrogen dynamics – effects of daily irrigation and fertilization. Plant Soil. 1993;151(1):21–30. doi: 10.1007/BF00010782.
- Calvo Buendia E, Tanabe K, Kranjc A, Baasansuren J, Fukuda M, Ngarize S, Osako A, Pyrozhenko Y, Shermanau P, Federici, S. 2019 Refinement to the 2006 IPCC Guidelines for National Greenhouse Gas Inventories. Switzerland; IPCC Task Force on National Greenhouse Gas Inventories; 2019.
- Bolinder MA, Angers DA, Dubuc J-P. Estimating shoot to root ratios and annual carbon inputs in soils for cereal crops. Agric Ecosyst Environ. 1997;63(1):61–66. doi: 10.1016/S0167-8809(96)01121-8.
- Bolinder MA, Kätterer T, Poeplau C, et al. Net primary productivity and belowground crop residue inputs for root crops: potato (Solanum tuberosum L.) and sugar beet (Beta vulgaris L). Can J Soil Sci. 2015;95(2):87–93. doi: 10.4141/cjss-2014-091.
- Ilola A, Elomaa E, Pulli S. Testing a Danish growth model for barley, turnip rape and timothy in Finnish conditions. AFSci. 1988;60(7):631–660. doi: 10.23986/afsci.72334.
- Barraclough PB. Root growth, macro-nutrient uptake dynamics and soil fertility requirements of a high-yielding winter oilseed rape crop. Plant Soil. 1989;119(1):59–70. doi: 10.1007/BF02370269.
- Pietola L, Alakukku L. Root growth dynamics and biomass input by Nordic annual field crops. Agric Ecosyst Environ. 2005;108(2):135–144. doi: 10.1016/j.agee.2005.01.009.
- Gan YT, Campbell CA, Janzen HH, et al. Carbon input to soil from oilseed and pulse crops on the Canadian prairies. Agric Ecosyst Environ. 2009;132(3-4):290–297. doi: 10.1016/j.agee.2009.04.014.
- Williams JD, McCool DK, Reardon CL, et al. Root:shoot ratios and belowground biomass distribution for pacific northwest dryland crops. J Soil Water Conserv. 2013;68(5):349–360. doi: 10.2489/jswc.68.5.349.
- Pausch J, Tian J, Riederer M, et al. Estimation of rhizodeposition at field scale: upscaling of a 14C labeling study. Plant Soil. 2012;364(1-2):273–285. doi: 10.1007/s11104-012-1363-8.
- Buyanovsky GA, Wagner GH. Crop residue input to soil organic matter on Sanborn field. In: Paul EA, Paustian KH, Elliott ET, et al. editor. Soil organic matter in temperate agroecosystems: long-term experiments in North America. Boca Raton (FL): CRC Press; 1997. p. 73–83.
- Bolinder MA, Kätterer T, Andrén O, et al. Estimating carbon inputs to soil in forage-based crop rotations and modeling the effects on soil carbon dynamics in a Swedish long-term field experiment. Can J Soil Sci. 2012;92(6):821–833. doi: 10.4141/cjss2012-036.
- Andrén O, Kihara J, Bationo A, et al. Soil climate and decomposer activity in Sub-Saharan Afrika estimated from standard weather station data: a simple climate index for soil carbon balance calculations. AMBIO. 2007;36(5):379–386. doi: 10.1579/0044-7447(2007)36[379:SCADAI]2.0.CO;2.
- Tang J, Riley WJ. Linear two-pool models are insufficient to infer soil organic matter decomposition temperature sensitivity from incubations. Biogeochemistry. 2020;149(3):251–261. doi: 10.1007/s10533-020-00678-3.
- Dungait JaJ, Hopkins DW, Gregory AS, et al. Soil organic matter turnover is governed by accessibility not recalcitrance. Glob Chang Biol. 2012;18(6):1781–1796. doi: 10.1111/j.1365-2486.2012.02665.x.
- Kleber M, Nico PS, Plante A, et al. Old and stable soil organic matter is not necessarily chemically recalcitrant: implications for modeling concepts and temperature sensitivity. Glob Chang Biol. 2011;17(2):1097–1107. doi: 10.1111/j.1365-2486.2010.02278.x.
- Farina R, Sándor R, Abdalla M, et al. Ensemble modelling, uncertainty and robust predictions of organic carbon in long-term bare-fallow soils. Glob Chang Biol. 2021;27(4):904–928. doi: 10.1111/gcb.15441.
- Fleischer K, Dolman AJ, van der Molen MK, et al. Nitrogen deposition maintains a positive effect on terrestrial carbon sequestration in the 21st century despite growing phosphorus limitation at regional scales. Global Biogeochem Cycles. 2019;33(6):810–824. doi: 10.1029/2018GB005952.
- Ladha JK, Reddy CK, Padre AT, et al. Role of nitrogen fertilization in sustaining organic matter in cultivated soils. J Environ Qual. 2011;40(6):1756–1766. doi: 10.2134/jeq2011.0064.
- Poeplau C, Don A. A simple soil organic carbon level metric beyond the organic carbon‐to‐clay ratio. Soil Use Manage. 2023;39(3):1057–1067. doi: 10.1111/sum.12921.
- Feng J, Zhu B. Global patterns and associated drivers of priming effect in response to nutrient addition. Soil Biol Biochem. 2021;153:108118. doi: 10.1016/j.soilbio.2020.108118.
- Cagnarini C, Renella G, Mayer J, et al. Multi-objective calibration of RothC using measured carbon stocks and auxiliary data of a long-term experiment in Switzerland. Eur J Soil Sci. 2019;70(4):819–832. doi: 10.1111/ejss.12802.
- Juston J. Environmental modeling: learning from uncertainty; Doctoral dissertation. Lund University; 2023. https://portal.research.lu.se/sv/publications/environmental-modelling-learning-from-uncertainty
- Larson WE, Clapp CE, Pierre WH, et al. Effects of increasing amounts of organic residues on continuous corn: II. Organic carbon, nitrogen, phosphorus and sulfur. Agron J. 1972;64(2):204–209. doi: 10.2134/agronj1972.00021962006400020023x.
- Barber SA. Corn residue management and soil organic matter. Agron J. 1979;71(4):625–627. doi: 10.2134/agronj1979.00021962007100040025x.
- Delas J, Molot C. Effet de divers amendements organiques sur les rendements du maïs et de la pomme de terre cultivés en sol sableux. Agron. 1983;3(1):19–26. doi: 10.1051/agro:19830103.
- Angers DA, Voroney RP, Côté D. Dynamics of soil organic matter and corn residues affected by tillage practices. Soil Sci Soc Am J. 1995;59(5):1311–1315. doi: 10.2136/sssaj1995.03615995005900050016x.
- Liang C, Hao X, Schoenau J, et al. Manure-induced carbon retention measured from long-term field studies in Canada. Agric Ecosyst Environ. 2021;321:107619. doi: 10.1016/j.agee.2021.107619.
- Palosuo T, Heikkinen J, Regina K. Method for estimating soil carbon stock changes in Finnish mineral cropland and grassland soils. Carbon Manag. 2016;6(5-6):207–220. doi: 10.1080/17583004.2015.1131383.
- Xu H, Vandecasteele B, Maenhout P, et al. Maize root biomass and architecture depend on site but not on variety: consequences for prediction of C inputs and spread in topsoil based on root-to-shoot ratios. Eur J Agron. 2020;119:126121. doi: 10.1016/j.eja.2020.126121.
Appendix A
The model has been adapted from Kätterer and Andrén [Citation34]. In order to have a climatic parameter variable over time, we started from the step version of the ICBM models.
The initial model:
For simplicity, we substitute with one single term for the flux between the pools:
Obtaining a more compact writing:
To consider the three input classes, we now introduce three Young pools with three humifications (but with the same kinetic ), R (Roots), S (Shoots), and M (Manure):
We therefore have also three fluxes:
Which all end up in the Old pool:
The total SOC is given by the sum of the Old and Young pools:
To which we can add a constant Inert pool ():
Appendix B
A diagram of the information flow in the climatic module.
Appendix C
All the residuals of the two calibrations. Empty points represent the calibration until 1999, and solid fill points until 2019.
Appendix D
The traceplots of the MCMCs of selected parameters.
Appendix E
The prior and posterior probability distribution of the C content of organic matter.
Appendix F
The C inputs from roots estimated by the Bayesian calibration.